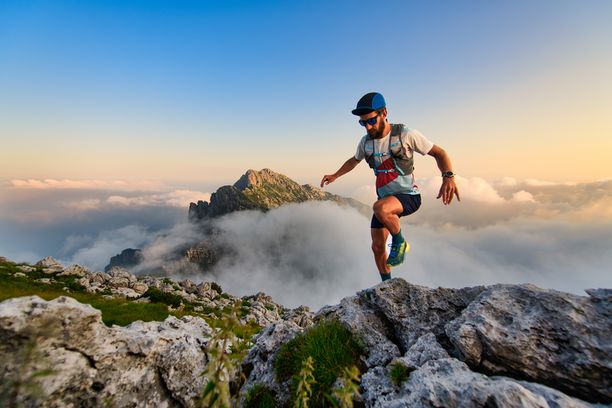
94% of researchers rate our articles as excellent or good
Learn more about the work of our research integrity team to safeguard the quality of each article we publish.
Find out more
ORIGINAL RESEARCH article
Front. Cardiovasc. Med., 10 October 2024
Sec. General Cardiovascular Medicine
Volume 11 - 2024 | https://doi.org/10.3389/fcvm.2024.1454321
Background: Traditional prognostic models for ST-segment elevation myocardial infarction (STEMI) have limitations in statistical methods and usability.
Objective: We aimed to develop a machine-learning (ML) based risk score to predict in-hospital mortality, intensive care unit (ICU) admission, and left ventricular ejection fraction less than 40% (LVEF < 40%) in STEMI patients.
Methods: We reviewed 1,863 consecutive STEMI patients undergoing primary percutaneous coronary intervention (pPCI) or rescue PCI. Eight supervised ML methods [LASSO, ridge, elastic net (EN), decision tree, support vector machine, random forest, AdaBoost and gradient boosting] were trained and validated. A feature selection method was used to establish more informative and nonredundant variables, which were then considered in groups of 5/10/15/20/25/30(all). Final models were chosen to optimise area under the curve (AUC) score while ensuring interpretability.
Results: Overall, 128 (6.9%) patients died in hospital, with 292 (15.7%) patients requiring ICU admission and 373 (20.0%) patients with LVEF < 40%. The best-performing model with 5 included variables, EN, achieved an AUC of 0.79 for in-hospital mortality, 0.78 for ICU admission, and 0.74 for LVEF < 40%. The included variables were age, pre-hospital cardiac arrest, robust collateral recruitment (Rentrop grade 2 or 3), family history of coronary disease, initial systolic blood pressure, initial heart rate, hypercholesterolemia, culprit vessel, smoking status and TIMI flow pre-PCI. We developed a user-friendly web application for real-world use, yielding risk scores as a percentage.
Conclusions: The STEMI-ML score effectively predicts in-hospital outcomes in STEMI patients and may assist with risk stratification and individualising patient management.
The advent of timely reperfusion strategies for patients with ST elevation myocardial infarction (STEMI) has yielded improvement in prognosis, including mortality (1). Whilst the overall outcomes following STEMI have improved over time, it remains a notable health problem with significant morbidity and mortality (2). The in-hospital mortality rate associated with STEMI ranges from 8.4%–33.5%, and complications include heart failure, cardiogenic shock, malignant arrhythmias, ventricular free wall rupture and tamponade (2, 3). Early identification of patients who are at the highest risk of poorer outcomes after STEMI remains key in both mitigating these outcomes and the burden to the healthcare system.
The Thrombolysis In Myocardial Infarction (TIMI) and Global Registry of Acute Coronary Events (GRACE) scores are commonly used risk assessment tools for acute coronary syndrome, including STEMI (4, 5). However, treatment strategies for STEMI have evolved significantly since the creation of these scores and their applicability in this contemporary setting remains uncertain. The GRACE risk score encompasses both STEMI and non-ST-segment elevation myocardial infarction (NSTEMI) patients, thus limiting its suitability as a predictive model specifically for STEMI patients, who remain a higher risk cohort (5–7). Whilst the TIMI risk score targets STEMI patients, it has been developed from a cohort of patients managed predominantly with thrombolytic therapy, as opposed to the contemporary gold standard of primary percutaneous reperfusion (4, 8, 9). Moreover, these traditional risk scoring models may fail to capture nonlinear effects of significance and oversimplify intricate interrelations among variables (10).
Consequently, there remains a need for sophisticated predictive models that accurately predict outcomes in patients after STEMI, including mortality, intensive care unit (ICU) admission and left ventricular impairment, to allow for effective risk stratification, resource allocation and optimal patient management. Machine-learning (ML) is a branch of computer science dedicated to predicting outcomes in intricate datasets through various algorithms that iteratively learn from the data (11, 12). In contrast to conventional statistical methods, which dependent on predefined assumptions regarding data behaviour and preselected variables, ML algorithms empower the model to develop by recognizing and integrating underlying data patterns (13, 14). ML models have consistently exhibited significantly better performance when compared to traditional methods for predicting risks (10).
The aim of our study is to develop a highly interpretable and effective machine-learning based risk prediction algorithm for mortality, ICU admission and left ventricular ejection fraction less than 40% (the STEMI-ML Score).
We reviewed all consecutive patients presenting to our tertiary Australian centre for primary percutaneous coronary intervention (pPCI) or rescue PCI (following thrombolysis) for STEMI from July 2010 to December 2019. The study received approval from the Northern Sydney Local Health District Human Research Ethics Committee. All patients were treated with aspirin prior to PCI, unless intolerant of oral medications and all patients were given intra-arterial, therapeutic heparin at the start of the procedure. The use of glycoprotein IIb/IIIa inhibitors was at the discretion of the proceduralist.
Patient electronic medical records were analysed to identify key demographic, clinical, biochemical, imaging, and procedural details. At the onset of coronary angiography, invasive hemodynamic parameters such as heart rate (HR) and aortic systolic blood pressure (SBP) were recorded and labelled as “starting HR” and “starting SBP”. Individual coronary angiograms were analysed to determine the presence and maturity of collaterals, which were then graded according to the Rentrop classification as grade 0 (absence of collateral filling), grade 1 [filling of side branches of the infarct-related artery (IRA)], grade 2 (partial filling of the epicardial vessel of the IRA) and grade 3 (complete filling of the IRA). Patients with Rentrop grade 0 or 1 collaterals were labelled as having poor collaterals, and patients with Rentrop grade 2 or 3 collaterals were labelled as having robust collaterals. Left ventricular function was evaluated using transthoracic echocardiogram after STEMI, or if unavailable, ventriculography at the time of the index procedure. The time at the onset of continuous chest pain to the time of acquisition of the first angiographic image during percutaneous coronary intervention was defined as the ischaemic time.
The primary outcomes were in-hospital mortality, intensive care unit (ICU) admission and left ventricular ejection fraction less than 40% (LVEF < 40%). In the included sites, STEMI patients are routinely admitted to the Coronary Care Unit (CCU) for ongoing care. There were no specific criteria for ICU admission. Generally, patients who require significant inotropic/vasopressor support (e.g., noradrenaline or adrenaline), intubation/mechanical ventilation, mechanical circulatory support (e.g., intra-aortic balloon pump or extracorporeal membrane oxygenation) or significant clinical instability prohibiting ongoing care in CCU are transferred to ICU.
Data were separated into two subsets, with 80% used as training data for feature selection and training of model parameters and 20% used as test data to evaluate model performance. The methodology of this machine-learning process is shown in Figure 1. We used eight supervised-learning classification models to create risk prediction algorithms for the outcome variables of in-hospital mortality, ICU admission and LVEF < 40%. These models include three linear, logistic regression (LR) based models [least absolute shrinkage and selection operator (LASSO or L1), ridge (L2) and Elastic Net (EN)] and five non-linear, models [decision tree (DT), support vector machine (SVM), random forest (RF), AdaBoost (AB) and gradient boosting (GB)].
We oversampled the training dataset for each model by the minority class using SMOTENC, which is an extension of SMOTE (15). Missing values for each included feature were imputed by the median value and features were standardised by subtracting the mean and scaling to a variance of 1. Feature selection for LR was performed in a sequential forward fashion and a subset of features were selected in a greedy fashion, where the next best feature to add in the subset is based on the AUC cross validation score. The best 5/10/15/20/25/30(all) features as determined by the coefficient magnitude were used as features for other models. This particular process of extracting, ranking, and grouping features was utilised to promote model interpretability and usability.
Model hyperparameters were chosen using 5-fold cross validation with AUC as the scoring metric. After parameter tuning, each model was evaluated on the test set and the final model was chosen based on the AUC score. The final models were then calibrated by fitting a sigmoid regressor and isotonic regressor, and optimal calibration was evaluated using the the Brier score and calibration plot. The threshold for significance was established at 5% for all statistical tests. We performed our analyses with Python (version 3.7), and the methodology code can be found on: https://github.com/harisritharan/stemi_risk_prediction/blob/master/stemi%20model%20building.ipynb.
To facilitate real-world use of our models, a pragmatic and user-friendly web application was built using Python Dash. Within this web application, the top-performing models for in-hospital mortality, ICU admission, and LVEF < 40% are incorporated, allowing users to manually select variables to generate a risk assessment score presented as a percentage.
The total study cohort included 1,863 patients; 128 (6.9%) patients died in-hospital, 292 (19.0%) patients required ICU admission and 373 (20.8%) patients had LVEF < 40%. The mean age of patients was 64.9 ± 13.7 years and 77.1% were male. Additional study cohort characteristics are detailed in Table 1.
From the 8 ML algorithms applied, the model obtained by EN had the best performance for the outcome of in-hospital mortality when including 30 features (AUC 0.81), 25 features (AUC 0.81), 20 features (AUC 0.81), 15 features (AUC 0.80), 10 features (AUC 0.79) and 5 features (AUC 0.79). The changes in model performance across the 8 ML algorithms based on the number of included features is shown in Figure 2A.
Figure 2. Predictive performance of models by number of variables for in-hospital mortality (A), ICU admission (B) and LVEF < 40% (C).
When balancing model performance with ease of interpretability and useability, the model obtained by EN including 5 features was chosen as the final model (AUC 0.79, accuracy 0.74, precision 0.18, recall 0.68, f1 score 0.28). The performance of this EN model and its comparison against the performance of other models including 5 features derived by the remaining 7 ML algorithms is detailed in Supplementary Table S1; Supplementary Figure S1.
The results of the final model found that higher age and pre-hospital cardiac arrest were associated with increased in-hospital mortality. In contrast, the presence of robust collateral recruitment, a family history of coronary disease before age 50 and higher starting SBP were associated with lesser in-hospital mortality. The coefficients of the EN model were used to build the in-hospital mortality component of the STEMI-ML score (Figure 3A).
Figure 3. Included coefficients and weighting in final models for in-hospital mortality (A), ICU admission (B) and LVEF < 40% (C).
The model obtained by RF had the best performance for the outcome of ICU admission when including 30 features (AUC 0.82). The model obtained by GB performed best when including 25 features (AUC 0.83) and 15 features (AUC 0.82). The model obtained by AB had the best performance when including 20 features (AUC 0.82), and the model obtained by L2 had the best performance when including 10 variables (AUC 0.81). However, the model obtained by EN performed best when including 5 features (AUC 0.78). The changes in model performance across the 8 ML algorithms based on the number of included features is shown in Figure 2B.
When balancing model performance with ease of interpretability and useability, the model obtained by EN including 5 features was chosen as the final model (AUC 0.78, accuracy 0.76, precision 0.32, recall 0.67, f1 score 0.44). The performance of this EN model and its comparison against the performance of other models including 5 features derived by the remaining 7 ML algorithms is detailed in Supplementary Table S2; Supplementary Figure S2.
The results of the final model found that pre-hospital cardiac arrest and a higher starting HR were associated with increased ICU admission. However, pre-existing diagnosis of hypercholesterolemia, a family history of coronary disease before age 50 and higher starting SBP were associated with decreased ICU admission. The coefficients of this EN model were used to build the ICU admission component of the STEMI-ML score (Figure 3B).
Out of the 8 ML algorithms, the model obtained by AB had the best performance for the outcome of LVEF < 40% when including 30 features (AUC 0.76), 25 features (AUC 0.76), 15 features (AUC 0.75) and 10 features (AUC 0.76). The model obtained by RF had the best performance when including 20 features (AUC 0.76). However, when including 5 features (AUC 0.74), the model obtained by EN had the best performance and this was marginally lower than the AB and RF models that included more features. The changes in model performance across the 8 ML algorithms based on the number of features included is shown in Figure 2C.
When balancing model performance with ease of interpretability and useability, the model obtained by EN including 5 features was chosen as the final model (AUC 0.74, accuracy 0.72, precision 0.44, recall 0.64, f1 score 0.52). The performance of this EN model and its comparison against the performance of other models including 5 features derived by the remaining 7 ML algorithms is detailed in Supplementary Table S3; Supplementary Figure S3.
The results of the final model found that the left anterior descending coronary artery as the culprit vessel was associated increased likelihood of LVEF < 40%. However, higher starting SBP, current smoking status, TIMI flow 2 pre-PCI and the presence of robust collateral recruitment were associated with decreased likelihood of LVEF < 40%. The coefficients of this EN model were used to build the LVEF < 40% component of the STEMI-ML score (Figure 3C).
Finally, we developed a web-based application for the individual probability for in-hospital mortality, ICU admission and LVEF < 40% in STEMI patients. This web application is shown in Figure 4 and is available at: https://stemi-ml-score.onrender.com.
We present dedicated machine-learning based risk prediction models for in-hospital mortality, ICU admission and LVEF < 40% in STEMI patients. These models are practical in their design and use data that is routinely collected during and in the lead up to PCI; thereby allowing for risk prediction immediately post-PCI. A criticism of existing prediction models is the large number of variables included and the limited useability in this context. We have pragmatically included five variables in all our models and have still achieved highly effective predictive capabilities with AUC greater than 0.7 and comparable if not superior efficacy to existing risk scores (16, 17). Moreover, we have developed a user-friendly, web-based application, the “STEMI-ML Score”, to assist clinicians at the bedside in risk stratifying patients in real-time: https://stemi-ml-score.onrender.com.
The presence of robust coronary collaterals has previously been reported as a protective factor for in-hospital mortality and left ventricular function in STEMI patients, however existing risk models do not consider it as one of their exposure variables (18–20). This significant impact of robust coronary collaterals on in-hospital outcomes is again reflected in our study, where it features in our final models for mortality and left ventricular impairment. The inclusion of variables such as coronary collateral circulation presents a major strength of our study and methodology with a machine-learning approach, wherein a larger number of variables can be considered, and key novel signals can be appreciated.
Whilst patients with a family history of coronary disease are at increased risk of developing a myocardial infarction, observational studies have conversely demonstrated lower in-hospital mortality and adverse clinical events in this group. A retrospective, observational study of 2,123,492 STEMI admissions demonstrated significantly lower odds of in-hospital mortality in patients with a family history of coronary artery disease compared to patients without [1.4% vs. 8.1%; aOR 0.42, 95% confidence interval (CI): 0.41–0.44; P < 0.001] (21). The purported mechanism for this difference was the influence of family history on heightened patient awareness of cardiovascular health and increased patient focus on pharmacological and non-pharmacological modalities of cardiovascular risk reduction (21). Our final models for in-hospital mortality and ICU admission find family history of coronary disease before age 50 to be a significant protective factor.
Several studies have investigated the cholesterol paradox in patients with acute coronary syndromes and NSTEMI or STEMI alone (22, 23). While hypercholesterolemia is a well-known risk factor for STEMI, studies have demonstrated lower risk of adverse events following STEMI in patients with hypercholesterolemia (22, 24). A retrospective, observational study including 11,543 STEMI patients and 8,470 NSTEMI patients demonstrated lower adverse events in patients with higher low-density lipoprotein-C and triglyceride levels (24). Similarly, the smoker's paradox has also been investigated in several studies, with a lower risk of adverse events noted especially in current smokers following STEMI (25, 26). This smoker's paradox may be explained by the younger age and fewer cardiovascular risk factors in smokers compared with non-smokers (27). Both the cholesterol paradox and smoker's paradox are demonstrated in our final models, with hypercholesterolemia a protective factor for ICU admission and current smoking a protective factor for left ventricular impairment.
Higher age and pre-hospital cardiac arrest are well-established predictors of poor outcomes in STEMI patients and are seen in our models for in-hospital mortality and ICU admission (28–30). A higher starting SBP is also an established protective predictor of outcomes in STEMI patients and features in all our final models (31). However, a higher starting heart rate has been associated with poorer outcomes in STEMI patients and was similarly a predictor for ICU admission in our study (32). Left ventricular impairment with an ejection fraction less than 40% was seen in 20.8% of patients in our study, and a culprit lesion in the left anterior descending artery (LAD) was a significant predictor of this outcome, likely mediated by the large myocardial territory supplied by the LAD.
Our study has some important limitations. Whilst our model was validated on an internal test dataset, external validation on another dataset would be ideal and necessary prior to consideration of widespread use. External validation also would be ideal from the perspective of validating the generalisability of our study, which involved a single centre only. Furthermore, our dataset exhibited an imbalance in the outcome variables, which is a common challenge encountered in the construction of machine-learning-based risk prediction algorithms for medical applications where the outcomes may be less common. To address this issue, we employed oversampling of the minority class during the training of each model, and thereby mitigated the impact of this imbalance on the algorithm's performance. Finally, the exposure variables included in the analysis were not comprehensive, and missing variables, although imputed and uncommon, may influence the outcomes.
We present a highly interpretable and effective machine-learning based risk prediction algorithm to predict in-hospital mortality, ICU admission and LVEF < 40% in STEMI patients: the STEMI-ML Score. This may assist in the risk stratification, individualised monitoring, and management of STEMI patients.
The original contributions presented in the study are included in the article, further inquiries can be directed to the corresponding author.
The studies involving humans were approved by Northern Sydney Local Health District Human Research Ethics Committee. The studies were conducted in accordance with the local legislation and institutional requirements. The ethics committee/institutional review board waived the requirement of written informed consent for participation from the participants or the participants’ legal guardians/next of kin because Retrospective study with large number of patients, many of whom where follow-up and consent is not feasible. The project itself was deemed to have low to negligible risk and a waiver of consent was granted.
HS: Writing – review & editing, Writing – original draft, Validation, Project administration, Methodology, Investigation, Formal Analysis, Data curation, Conceptualization. HN: Writing – review & editing, Writing – original draft, Methodology, Formal Analysis, Conceptualization. JC: Writing – review & editing, Writing – original draft, Investigation, Data curation. RB: Writing – review & editing, Writing – original draft, Supervision, Project administration, Methodology, Conceptualization. UA: Writing – review & editing, Writing – original draft, Supervision, Project administration, Methodology, Data curation, Conceptualization.
The author(s) declare that no financial support was received for the research, authorship, and/or publication of this article.
The authors declare that the research was conducted in the absence of any commercial or financial relationships that could be construed as a potential conflict of interest.
All claims expressed in this article are solely those of the authors and do not necessarily represent those of their affiliated organizations, or those of the publisher, the editors and the reviewers. Any product that may be evaluated in this article, or claim that may be made by its manufacturer, is not guaranteed or endorsed by the publisher.
The Supplementary Material for this article can be found online at: https://www.frontiersin.org/articles/10.3389/fcvm.2024.1454321/full#supplementary-material
1. Ahuja KR, Saad AM, Nazir S, Ariss RW, Shekhar S, Isogai T, et al. Trends in clinical characteristics and outcomes in ST-elevation myocardial infarction hospitalizations in the United States, 2002–2016. Curr Probl Cardiol. (2022) 47(12):101005. doi: 10.1016/j.cpcardiol.2021.101005
2. Ratwatte S, Ng ACC, Hyun K, Philip R, Boroumand F, Weber C, et al. Pre-hospital and in-hospital ST-elevation myocardial infarction from 2008 to 2020 in Australia. Int J Cardiol Cardiovasc Risk Prev. (2023) 19:200214. doi: 10.1016/j.ijcrp.2023.200214
3. Choudhury T, West NE, El-Omar M. ST elevation myocardial infarction. Clin Med (Lond). (2016) 16(3):277–82. doi: 10.7861/clinmedicine.16-3-277
4. Morrow DA, Antman EM, Charlesworth A, Cairns R, Murphy SA, de Lemos JA, et al. TIMI risk score for ST-elevation myocardial infarction: a convenient, bedside, clinical score for risk assessment at presentation: an intravenous nPA for treatment of infarcting myocardium early II trial substudy. Circulation. (2000) 102(17):2031–7. doi: 10.1161/01.CIR.102.17.2031
5. Fox KA, Dabbous OH, Goldberg RJ, Pieper KS, Eagle KA, Van de Werf F, et al. Prediction of risk of death and myocardial infarction in the six months after presentation with acute coronary syndrome: prospective multinational observational study (GRACE). Br Med J. (2006) 333(7578):1091. doi: 10.1136/bmj.38985.646481.55
6. Elbarouni B, Goodman SG, Yan RT, Welsh RC, Kornder JM, Deyoung JP, et al. Validation of the global registry of acute coronary event (GRACE) risk score for in-hospital mortality in patients with acute coronary syndrome in Canada. Am Heart J. (2009) 158(3):392–9. doi: 10.1016/j.ahj.2009.06.010
7. Fox KA, Fitzgerald G, Puymirat E, Huang W, Carruthers K, Simon T, et al. Should patients with acute coronary disease be stratified for management according to their risk? Derivation, external validation and outcomes using the updated GRACE risk score. BMJ Open. (2014) 4(2):e004425. doi: 10.1136/bmjopen-2013-004425
8. Morrow DA, Antman EM, Parsons L, de Lemos JA, Cannon CP, Giugliano RP, et al. Application of the TIMI risk score for ST-elevation MI in the national registry of myocardial infarction 3. JAMA. (2001) 286(11):1356–9. doi: 10.1001/jama.286.11.1356
9. Morrow DA, Antman EM, Snapinn SM, McCabe CH, Theroux P, Braunwald E. An integrated clinical approach to predicting the benefit of tirofiban in non-ST elevation acute coronary syndromes. Application of the TIMI risk score for UA/NSTEMI in PRISM-PLUS. Eur Heart J. (2002) 23(3):223–9. doi: 10.1053/euhj.2001.2738
10. Liu W, Laranjo L, Klimis H, Chiang J, Yue J, Marschner S, et al. Machine-learning versus traditional approaches for atherosclerotic cardiovascular risk prognostication in primary prevention cohorts: a systematic review and meta-analysis. Eur Heart J Qual Care Clin Outcomes. (2023) 9(4):310–22. doi: 10.1093/ehjqcco/qcad017
11. Quer G, Arnaout R, Henne M, Arnaout R. Machine learning and the future of cardiovascular care: JACC state-of-the-art review. J Am Coll Cardiol. (2021) 77(3):300–13. doi: 10.1016/j.jacc.2020.11.030
12. Chiarito M, Luceri L, Oliva A, Stefanini G, Condorelli G. Artificial intelligence and cardiovascular risk prediction: all that glitters is not gold. Eur Cardiol. (2022) 17:e29. doi: 10.15420/ecr.2022.11
13. Al'Aref SJ, Anchouche K, Singh G, Slomka PJ, Kolli KK, Kumar A, et al. Clinical applications of machine learning in cardiovascular disease and its relevance to cardiac imaging. Eur Heart J. (2019) 40(24):1975–86. doi: 10.1093/eurheartj/ehy404
14. Vidal-Perez R, Vazquez-Rodriguez JM. Role of artificial intelligence in cardiology. World J Cardiol. (2023) 15(4):116–8. doi: 10.4330/wjc.v15.i4.116
15. Blagus R, Lusa L. SMOTE for high-dimensional class-imbalanced data. BMC Bioinformatics. (2013) 14:106. doi: 10.1186/1471-2105-14-106
16. D'Ascenzo F, Biondi-Zoccai G, Moretti C, Bollati M, Omedè P, Sciuto F, et al. TIMI, GRACE and alternative risk scores in acute coronary syndromes: a meta-analysis of 40 derivation studies on 216,552 patients and of 42 validation studies on 31,625 patients. Contemp Clin Trials. (2012) 33(3):507–14. doi: 10.1016/j.cct.2012.01.001
17. Yanqiao L, Shen L, Yutong M, Linghong S, Ben H. Comparison of GRACE and TIMI risk scores in the prediction of in-hospital and long-term outcomes among East Asian non-ST-elevation myocardial infarction patients. BMC Cardiovasc Disord. (2022) 22(1):4. doi: 10.1186/s12872-021-02311-z
18. Allahwala UK, Weaver JC, Nelson GI, Nour D, Ray M, Ciofani JL, et al. Effect of recruitment of acute coronary collaterals on in-hospital mortality and on left ventricular function in patients presenting with ST elevation myocardial infarction. Am J Cardiol. (2020) 125(10):1455–60. doi: 10.1016/j.amjcard.2020.02.023
19. Cui K, Lyu S, Song X, Yuan F, Xu F, Zhang M, et al. Effect of coronary collaterals on prognosis in patients undergoing primary percutaneous coronary intervention for acute ST-segment elevation myocardial infarction: a meta-analysis. Angiology. (2018) 69(9):803–11. doi: 10.1177/0003319718768399
20. Hara M, Sakata Y, Nakatani D, Suna S, Nishino M, Sato H, et al. Impact of coronary collaterals on in-hospital and 5-year mortality after ST-elevation myocardial infarction in the contemporary percutaneous coronary intervention era: a prospective observational study. BMJ Open. (2016) 6(7):e011105. doi: 10.1136/bmjopen-2016-011105
21. Agarwal MA, Garg L, Lavie CJ, Reed GL, Khouzam RN. Impact of family history of coronary artery disease on in-hospital clinical outcomes in ST-segment myocardial infarction. Ann Transl Med. (2018) 6(1):3. doi: 10.21037/atm.2017.09.27
22. Wang TY, Newby LK, Chen AY, Mulgund J, Roe MT, Sonel AF, et al. Hypercholesterolemia paradox in relation to mortality in acute coronary syndrome. Clin Cardiol. (2009) 32(9):E22–8. doi: 10.1002/clc.20518
23. Reddy VS, Bui QT, Jacobs JR, Begelman SM, Miller DP, French WJ. Relationship between serum low-density lipoprotein cholesterol and in-hospital mortality following acute myocardial infarction (the lipid paradox). Am J Cardiol. (2015) 115(5):557–62. doi: 10.1016/j.amjcard.2014.12.006
24. Sia CH, Zheng H, Ho AF, Bulluck H, Chong J, Foo D, et al. The lipid paradox is present in ST-elevation but not in non-ST-elevation myocardial infarction patients: insights from the Singapore myocardial infarction registry. Sci Rep. (2020) 10(1):6799. doi: 10.1038/s41598-020-63825-8
25. Venkatason P, Salleh NM, Zubairi Y, Hafidz I, Ahmad WA, Han SK, et al. The bizzare phenomenon of smokers’ paradox in the immediate outcome post acute myocardial infarction: an insight into the Malaysian national cardiovascular database-acute coronary syndrome (NCVD-ACS) registry year 2006–2013. Springerplus. (2016) 5:534. doi: 10.1186/s40064-016-2188-3
26. Gupta T, Kolte D, Khera S, Harikrishnan P, Mujib M, Aronow WS, et al. Smoker’s paradox in patients with ST-segment elevation myocardial infarction undergoing primary percutaneous coronary intervention. J Am Heart Assoc. (2016) 5(4):e003370. doi: 10.1161/JAHA.116.003370
27. Redfors B, Furer A, Selker HP, Thiele H, Patel MR, Chen S, et al. Effect of smoking on outcomes of primary PCI in patients with STEMI. J Am Coll Cardiol. (2020) 75(15):1743–54. doi: 10.1016/j.jacc.2020.02.045
28. Doan TN, Prior M, Vollbon W, Rogers B, Rashford S, Bosley E. Survival after resuscitated out-of-hospital cardiac arrest in patients with paramedic-identified ST-segment elevation myocardial infarction treated with primary percutaneous coronary intervention. Prehosp Emerg Care. (2022) 26(6):764–71. doi: 10.1080/10903127.2021.1992054
29. Newell MC, Henry JT, Henry TD, Duval S, Browning JA, Christiansen EC, et al. Impact of age on treatment and outcomes in ST-elevation myocardial infarction. Am Heart J. (2011) 161(4):664–72. doi: 10.1016/j.ahj.2010.12.018
30. Strauss M, Gitt AK, Becker T, Kleemann T, Schiele R, Darius H, et al. Prehospital cardiac arrest: a marker for higher mortality in patients with acute myocardial infarction and moderately reduced left ventricular function: results from the MITRA plus registry. Clin Res Cardiol. (2008) 97(10):748–52. doi: 10.1007/s00392-008-0673-6
31. Park JS, Cha KS, Shin D, Lee DS, Lee HW, Oh JH, et al. Prognostic significance of presenting blood pressure in patients with ST-elevation myocardial infarction undergoing percutaneous coronary intervention. Am J Hypertens. (2015) 28(6):797–805. doi: 10.1093/ajh/hpu230
32. Jensen MT, Pereira M, Araujo C, Malmivaara A, Ferrieres J, Degano IR, et al. Heart rate at admission is a predictor of in-hospital mortality in patients with acute coronary syndromes: results from 58 European hospitals: the European hospital benchmarking by outcomes in acute coronary syndrome processes study. Eur Heart J Acute Cardiovasc Care. (2018) 7(2):149–57. doi: 10.1177/2048872616672077
Keywords: acute coronary syndrome, myocardial infarction, ST-elevation myocardial infarction, machine-learning, risk score
Citation: Sritharan HP, Nguyen H, Ciofani J, Bhindi R and Allahwala UK (2024) Machine-learning based risk prediction of in-hospital outcomes following STEMI: the STEMI-ML score. Front. Cardiovasc. Med. 11:1454321. doi: 10.3389/fcvm.2024.1454321
Received: 24 June 2024; Accepted: 27 September 2024;
Published: 10 October 2024.
Edited by:
Dragos Cretoiu, Carol Davila University of Medicine and Pharmacy, RomaniaReviewed by:
Chun Chin Chang, Taipei Veterans General Hospital, TaiwanCopyright: © 2024 Sritharan, Nguyen, Ciofani, Bhindi and Allahwala. This is an open-access article distributed under the terms of the Creative Commons Attribution License (CC BY). The use, distribution or reproduction in other forums is permitted, provided the original author(s) and the copyright owner(s) are credited and that the original publication in this journal is cited, in accordance with accepted academic practice. No use, distribution or reproduction is permitted which does not comply with these terms.
*Correspondence: Hari P. Sritharan, aGFyaS5zcml0aGFyYW5Ac3lkbmV5LmVkdS5hdQ==
Disclaimer: All claims expressed in this article are solely those of the authors and do not necessarily represent those of their affiliated organizations, or those of the publisher, the editors and the reviewers. Any product that may be evaluated in this article or claim that may be made by its manufacturer is not guaranteed or endorsed by the publisher.
Research integrity at Frontiers
Learn more about the work of our research integrity team to safeguard the quality of each article we publish.