- 1Beijing University of Chinese Medicine, Beijing, China
- 2Xiyuan Hospital, China Academy of Chinese Medical Sciences, Beijing, China
Background: The escalating global economic burden of ischemic stroke poses a significant public health challenge amid global aging trends. The broad therapeutic efficacy of new antidiabetic drugs may offer new options in the prevention and treatment of ischemic stroke. Consistent conclusions regarding the relationship between novel antidiabetic agents and the risk of ischemic stroke remain elusive, and the causal relationship deserves further investigation.
Materials and methods: Three novel antidiabetic drug targets were selected, and cis-expression quantitative trait loci (cis-eQTL) were screened as instrumental variables. Genetic association data for ischemic stroke were obtained from the Genome-wide Association Study (GWAS) database. Mendelian randomization (MR) analysis, facilitated by R software, calculated MR estimates for each single nucleotide polymorphism (SNP), and meta-analysis was performed using five methods. To ensure robustness, sensitivity analyses, heterogeneity analyses, horizontal pleiotropy analyses, and co-localization analyses were conducted for significant MR associations.
Results: Three eQTLs for antidiabetic drug genes served as instrumental variables, utilizing a GWAS dataset comprising 34,217 cases and 406,111 controls for ischemic stroke. Genetic variants in glucagon-like peptide-1 receptor agonists (GLP-1 RA) targets exhibited a positive correlation with ischemic stroke risk (OR 1.06, 95% CI 1.04–1.08, P = 0.000), while genetic variation in dipeptidyl peptidase 4 inhibitors (DPP-4i) targets showed a negative association with ischemic stroke risk (OR 0.93, 95% CI 0.89–0.97, P = 0.003). Sensitivity analyses supported robust conclusions, revealing no heterogeneity or horizontal pleiotropy.
Conclusion: This study found that GLP-1 RA and DPP-4i were associated with an increased risk of ischemic stroke by MR analysis. Although sensitivity analyses provide support for this result, it contradicts previous knowledge. Therefore, the results of this study still need to treated with caution. Updated and more in-depth GWAS data and high-quality real-world data are expected to validate the results.
1 Introduction
Ischemic stroke, constituting approximately 80% of all stroke cases (1), exhibits an age-associated surge in incidence. Projections suggest a global increase to 89.32 per 100,000 people by 2030 (2). While strides have been made in mitigating ischemic stroke lethality, survivors contend with enduring disabilities impacting daily life (3). This scenario amplifies the escalating global economic burden, posing a substantial public health challenge amid global aging trends (4). The current gold standard for acute ischemic stroke treatment involves intravenous thrombolysis and thrombectomy. However, the efficacy is confined to a small subset of patients due to restricted treatment limitations (5). Concurrently, secondary brain tissue damage induced by ischemia-reperfusion persists as a significant concern (6). Therefore, primary prevention before the onset, encompassing strategies like antiplatelet agents, statins, and blood pressure control, emerges as the most effective means to curtail the harm and disease burden of ischemic stroke (7).
Disturbed glucose metabolism stands out as a crucial risk factor for ischemic stroke (8), emphasizing the significance of maintaining moderate blood glucose levels in primary prevention (9, 10). In recent years, emerging novel antidiabetic agents such as glucagon-like peptide-1 receptor agonists (GLP-1 RA), sodium-glucose co-transporter 2 inhibitors (SGLT-2i), and dipeptidyl peptidase 4 inhibitors (DPP-4i) have demonstrated robust therapeutic effects across endocrine, cardiovascular, and renal domains (11–13). Their diverse therapeutic effects present potential options in ischemic stroke prevention and treatment. However, conclusive conclusions about the relationship between novel antidiabetic drugs and ischemic stroke risk remain elusive, warranting in-depth exploration of their effects and causality (14–16).
Hence, this study employs Mendelian randomization (MR) analysis to delve into the causal relationship between the use of novel antidiabetic drugs and the risk of ischemic stroke. Leveraging genetic variants associated with drug targets as instrumental variables, MR simulates a randomized controlled trial setting, offering insights into the reuse potential and risk of novel antidiabetic drugs in ischemic stroke. The outcomes hold promise as a reference for clinical practice.
2 Materials and methods
2.1 Identification of target data for novel antidiabetic drugs
Novel antidiabetic drugs, including GLP-1 RA, SGLT-2i, and DPP-4i, were selected for this study. Genes encoding the target proteins of these drugs were meticulously identified from the Drugbank (v5.0) and ChEMBL (v29.0) databases (17, 18). The chromosomal locations, start, and termination sites of these genes were precisely determined through the National Center for Biotechnology Information (NCBI).
2.2 Identification of cis-expression quantitative trait loci (eQTL) data associated with novel antidiabetic drug targets
Given the proximity of cis-eQTL to target genes in drug development studies (19, 20), we extracted cis-eQTL with full statistical significance (false discovery rate <0.05 ± 1Mb per probe) from the eQTLGen consortium database (21). This approach aims to analyze the genetic underpinnings of complex traits through blood gene expression.
2.3 Genetic association data screening for ischemic stroke
Genetic association data for ischemic stroke were obtained from the European Ischemic Stroke Cohort, the largest GWAS meta-analysis of the disease to date (22), pooled by the MEGASTROKE Consortium. The pooled data were adjusted for unknown confounders such as sex and ethnicity to avoid possible bias. The type of stroke included any type of ischemic stroke, and a total of 34,217 cases and 406,111 controls were included (23). All ischemic stroke diagnoses were confirmed by clinical symptoms and imaging criteria. All participants gave informed consent, and the local research ethics committee and institutional review board approved the study.
2.4 MR analysis
The TwoSampleMR R software package (v 4.3.2) was used for MR analysis. To ensure the reliability of the results, stringent criteria were used to filter low-quality genetic tools. Based on the key assumption that MR analyses are established (24), the criteria for screening SNPs in the exposure data were as follows (25): (1) genome-wide SNPs with significance (P < 5 × 10−6), (2) exclusion of weak instrumental variables with an F-statistic <10, (3) linkage disequilibrium (LD) testing to ensure independence of selected instrumental variables (r2 < 0.1 within a 1,000 kb range) (26, 27), and (4) removal of SNPs with incompatible or palindromic allele frequencies. Subsequently, drug target-associated SNPs were further screened based on gene chromosomal loci ±100 kb range and filtered based on eaf >0.01. Finally, the ending data were extracted and merged based on the filtered instrumental variables.
MR estimates for each SNP were calculated using the Wald ratio method, and meta-analysis of MR estimates was performed using inverse variance weighted (IVW), MR-Egger, weighted median, simple mode, and weighted mode (28). IVW assumes that each genetic variant exists independently and can only influence the outcome through the exposure of interest (29). However, in the presence of pleiotropy, causality may be biased. Methods such as MR Egger and weighted median may avoid the bias caused by pleiotropic effects and confounders in genetic variants, but may yield wider confidence intervals (30, 31). The IVW method has been reported to be slightly more accurate than the other methods under practical conditions (28). Therefore, the results of this study are mainly based on the IVW method, with the other four methods as its complement. MR estimates with P < 0.05 were considered statistically significant. All estimates were expressed as odds ratio (OR) with a 95% confidence interval (CI) per standard deviation increase in the corresponding exposure.
2.5 Sensitivity and co-localization analysis
The MR-PRESSO test was used to test for horizontal pleiotropy. If horizontal pleiotropy was found between instrumental variables, outliers were removed and the MR analysis was re-executed (32). Sensitivity analyses were conducted via the leave-one-out method. This method calculates the meta effect of the remaining SNPs by progressively eliminating each SNP in order to observe the potential impact of each SNP on the study results (33). Cochran's Q statistic was utilized to assess potential heterogeneity. The method infers the presence of heterogeneity in the sample by calculating the estimated difference between the causal effect estimate and the estimated difference in the instrumental variable (34). The presence of horizontal pleiotropy can cause the results of MR analyses to be untenable, so we performed the MR Egger intercept test in addition to the MR-PRESSO test (30). The above analysis was done using TwoSampleMR R package (v 4.3.2).
Subsequently, co-localization analysis was executed with the colocR package and default prior. For the eQTL dataset, a priori probabilities of 1E-04 for cis-eQTL and ischemic stroke associations were set, with the prior probability of a single variant affecting both traits at 1E-05. Significant co-localization was determined at PH4 >0.80, and genes strongly co-localized with ischemic stroke were considered as potential target molecules. The overall design of the study is shown in Supplementary Figure 1.
3 Results
3.1 Selection of genetic tools for novel antidiabetic drugs
We identified three major genes encoding proteins experimentally modified by novel antidiabetic drugs. To generate genetic tools for substituting novel antidiabetic drug targets, we selected cis-eQTL within ±100 kb of each gene's genomic location (Table 1). The eQTL of the three antidiabetic drug genes with the highest significance were chosen as tool variables based on their P values. GWAS data for ischemic stroke were obtained from the ebi-a-GCST005843 dataset (22), comprising 34,217 cases and 406,111 controls from the MEGASTROKE Consortium, encompassing a total of 7,537,579 SNPs.
3.2 MR analysis
As shown in Table 2, GLP1R were significantly associated with ischemic stroke risk according to the Inverse variance weighted method (OR 1.06, 95%CI 1.04–1.08, P = 0.000). Additionally, DPP4 were significantly associated with ischemic stroke risk (OR 0.93, 95%CI 0.89–0.97, P = 0.003). Conversely, SLC5A2 was not statistically significantly associated with ischemic stroke risk (OR 1.23, 95%CI 0.97–1.55, P = 0.077). The scatter plot (Figure 1) visualized the causal relationship. Each point in the graph represents a SNP, and the short lines of the cross at each point reflect its 95% CI. The abscissa is the effect of the SNP on the exposure, and the ordinate is the effect of the SNP on the outcome. The slash lines of different colors represent the MR fitting results of different calculation methods. A slope greater than 0 indicates that the exposure factor is a disadvantage of ischemic stroke. For the fitting results of different methods, the results of IVW are generally the main ones. All five pooling methods concurred on the ischemic stroke risk of GLP1R and the protective effect of DPP4.
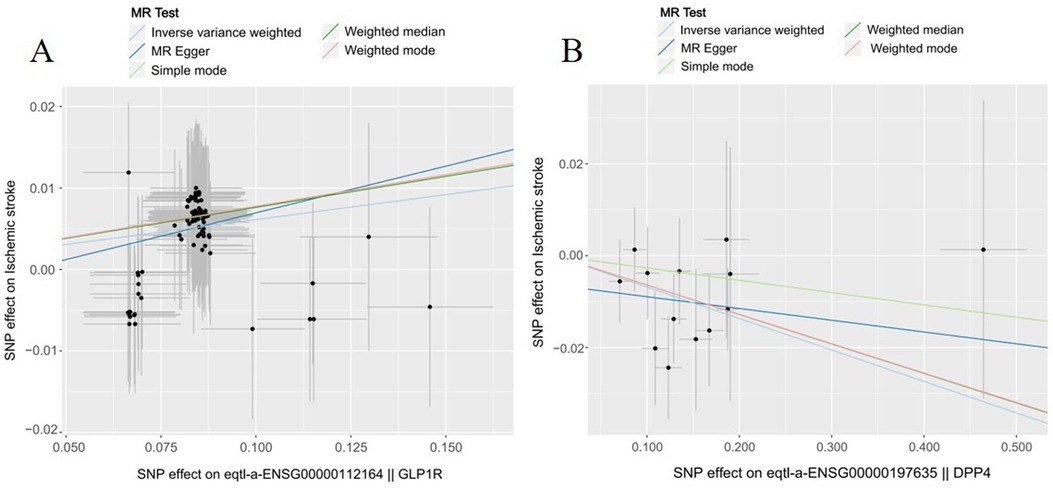
Figure 1. The effect of genetic variation in novel antidiabetic drug targets on ischemic stroke. (A) GLP1R (B) DPP4.
3.3 Sensitivity analysis and co-localization analysis
We performed the sensitivity analysis by the “leave-one-out” method (Supplementary Figure 2). The results showed that excluding each SNP individually did not affect the results of the MR pooling analysis, which indicated that the stability of the MR analysis.
To further verify the robustness of MR analysis, we analyzed the heterogeneity of MR by Cochran's Q statistic, and the MR-PRESSO method and Egger intercept test verified the horizontal pleiotropy of MR. As shown in Table 3, the results all indicated that there was no heterogeneity or horizontal pleiotropy between GLP1R and DPP4 gene and ischemic stroke risk (P > 0.05).
Co-localization analyses were conducted to ascertain the probability of shared causal genetic variants between SNPs associated with ischemic stroke and novel antidiabetic drug eQTL. Results revealed a 6.5% probability of the GLP1R gene having a shared genetic effect between eQTL and ischemic stroke risk, while the DPP4 gene exhibited a 10.7% probability of shared genetic effect between eQTL and ischemic stroke risk (Figure 2).
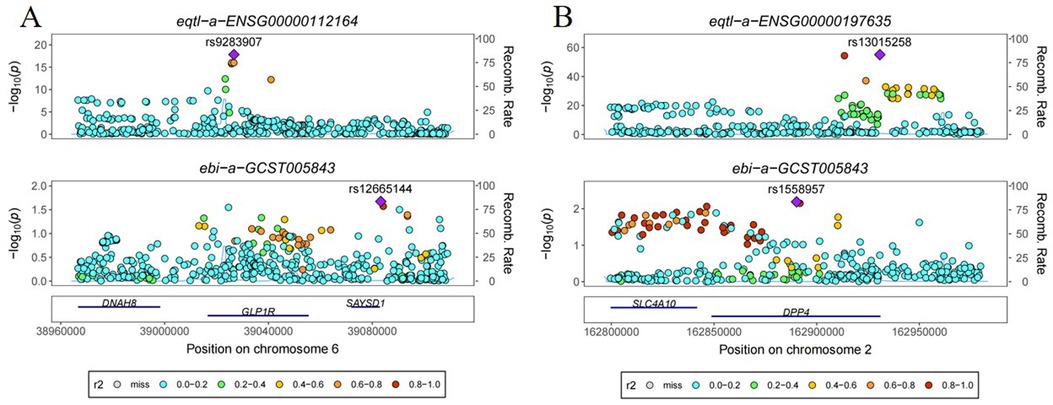
Figure 2. Co-localization analysis of the effect of genetic variants in novel antidiabetic drug targets on ischemic stroke. (A) GLP1R (B) DPP4.
4 Discussion
In recent years, novel antidiabetic agents, including GLP-1 RA, SGLT-2i, and DPP-4i, have exhibited broad therapeutic efficacy, potentially introducing new avenues for ischemic stroke prevention and treatment (35). However, the relationship between novel antidiabetic drugs and ischemic stroke risk lacks consistent conclusions. This study marks the inaugural exploration of the causal association between genetic variants of novel antidiabetic drug targets and ischemic stroke risk through MR analysis. Leveraging large-scale genetic association data on ischemic stroke, our analyses unveil a positive association between genetic variants of GLP-1 RA targets and ischemic stroke risk, contrasting with a negative association for genetic variants of DPP-4i targets. Notably, since GLP-1 RA promotes gene expression, and DPP-4i is an inhibitor of gene expression. Therefore, the final result of the analysis is that both GLP-1 RA and DPP-4i are risk factors for ischemic stroke. Although this result is somewhat contradictory to common sense, rigorous sensitivity analyses, heterogeneity assessments, and horizontal pleiotropy analyses support the robustness of these findings.
GLP-1 RA effectively lowers blood glucose in type 2 diabetes patients by stimulating β- and α-cell GLP-1 receptors, enhancing insulin secretion, inhibiting glucagon secretion, and improving insulin sensitivity (36). A meta-analysis of seven large randomized controlled studies with cardiovascular outcomes as endpoints showed that GLP-1 RA was able to reduce the risk of total stroke by 16% (37). This seems to provide compelling clinical evidence for the protective effect of GLP-1 RA against ischemic stroke. However, in all published studies, the subjects were clearly type 2 diabetic and the incidence of stroke was only one of the secondary indicators. For other patients with other metabolic diseases who may be taking GLP-1 RA for various reasons or for the normal population, there are no clear conclusions about the role of the drug in ischemic stroke. Furthermore, even in studies of patients with type 2 diabetes, the role of GLP-1 RA on stroke remains contradictory (38). For example, in the ELIXA study, it was found that GLP-1 RA appeared to increase the incidence of multiple strokes (39). Similarly, some early findings seem to suggest a protective effect of DPP4i against stroke (40, 41). However its possible risks are yet to be followed up and discussed in real-world study results.
Another conflicting finding came from a previously published Mendelian randomization study (42). This study found that genetic variants in GLP-1 were not associated with ischemic stroke, which seems to be inconsistent with both the previous study and our findings. The reason for this contradiction is mainly related to the limitation of GWAS sequencing depth and the number of SNPs (43). When the number of available SNPs is less than three, limiting the scope of a full Mendelian randomization (MR) analysis, these results should be excluded from the analysis to ensure the robustness and reliability of the results (44, 45). Therefore, the conclusions of the present study can be considered as a complement and extension of the results of that study.
Overall, our study identified GLP-1 RA and DPP-4i as risk factors for ischemic stroke.The MR analysis method was able to avoid confounding factors such as blood glucose levels and population, thus obtaining an exact causal association in a larger population. In addition, co-localization analysis of MR results was performed for the first time in this study, although this result does not seem to be very favorable. This apparent inconsistency may stem from the methodological differences between the two approaches. MR selectively identifies exposure-related variants, whereas co-localization is more conservative, requiring significant associations of causal variants with both traits. The co-localization analysis was performed with a Bayesian test, which assumes that there is only one shared causal variance locus. If more than one shared causal variance locus exists within an association interval, the results of the analysis may be affected (46, 47). In addition, some statistical experts believe that a higher PH4 suggests the presence of horizontal pleiotropy between the two, and therefore a lower PH4 can instead more favorably demonstrate a causal relationship between the two phenotypes (48).
SGLT-2i operates by selectively inhibiting glucose reabsorption in proximal renal tubules, leading to increased urinary glucose excretion (49, 50). Studies have demonstrated its efficacy in reducing the risk of hospitalization for ischemic stroke and improving cardiovascular outcomes, including ischemic stroke (16, 51). A retrospective cohort study indicated a lower risk of new stroke in SGLT-2i users compared to non-users, with an even greater reduction in patients concurrently using other hypoglycemic and lipid-lowering medications (52). Experimental studies further supported the neuroprotective effects of cagliflozin in a mouse model of moderate ischemic injury, reducing infarct volume, brain swelling, and improving neurological function (53). However, conflicting evidence suggests that SGLT-2i may not significantly reduce the incidence of ischemic stroke (14, 15). A meta-analysis involving five large randomized controlled trials with 46,969 subjects revealed no significant efficacy of SGLT-2i against fatal stroke, nonfatal stroke, ischemic stroke, or transient ischemic attack, with potential protective effects only against hemorrhagic stroke (54). Consequently, no consistent conclusions have been drawn regarding the relationship between SGLT-2i and the risk of ischemic stroke. This study, employing drug target-related genetic variants as instrumental variables, aims to infer causality by simulating a randomized controlled trial setting, offering a fresh perspective on addressing this issue. Our findings, however, fail to establish a causal association between gene variants in the target of action of SGLT-2i and the risk of ischemic stroke. It is noteworthy that due to the limitation of sequencing depth, we obtained an insufficient number of SNPs in the SGLT gene, and the results need to be interpreted with caution.
To the best of our knowledge, this represents the inaugural MR analysis on the target of action of novel antidiabetic drugs and the risk of ischemic stroke, providing a novel lens for drug development in this context. The strength of the MR design is its ability to infer causality while reducing confounding bias and reverse causality. Focusing on European ancestry minimizes spurious associations due to population stratification, and the selection of genetic variants as instruments within a narrow window of encoding genes mitigates biases arising from off-target effects and downstream proteins as instrumental variables.
However, there are some limitations to this study. Firstly, the current analysis was limited to European ancestry, which limits the generalizability of the results to other races. In addition, while drug target screening methods are effective in localizing effectiveness at the gene level, this somewhat limits our capacity to screen for SNPs. Although the screening criteria for SNPs in this study were sufficiently rigorous to ensure the reliability of the findings, the results need to be interpreted with caution. Furthermore, although we screened the cis-eQTL with the most significant relationship with gene expression of drug targets as a tool. In some cases, changes in eQTL may not fully reflect changes in blood glucose levels. However, due to the limitation of GWAS sequencing depth, we were unable to employ downstream indicators of blood glucose such as fasting blood glucose level and glycated hemoglobin as genetic tools for detailed discussion and analysis. This to some extent limits the accuracy of our drug target screening using MR analysis, so we look forward to more updated GWAS studies to provide more in-depth analysis and discussion of the findings.
5 Conclusion
This study found that GLP-1 RA and DPP-4i were associated with an increased risk of ischemic stroke by MR analysis. Although sensitivity analyses provide support for this result, it contradicts previous knowledge. Therefore, the results of this study still need to treated with caution. Updated and more in-depth GWAS data and high-quality real-world data are expected to validate the results.
Data availability statement
The original contributions presented in the study are included in the article/Supplementary Material, further inquiries can be directed to the corresponding author.
Author contributions
ZY: Conceptualization, Data curation, Formal Analysis, Methodology, Software, Writing – original draft. XL: Conceptualization, Data curation, Software, Writing – original draft. XF: Data curation, Formal Analysis, Writing – original draft. XZ: Conceptualization, Data curation, Formal Analysis, Writing – original draft. RG: Funding acquisition, Project administration, Resources, Supervision, Writing – review & editing.
Funding
The author(s) declare financial support was received for the research, authorship, and/or publication of this article. The study was financially supported by the National Key R&D Program of China (2021YFF0901404) and Science and Technology Innovation Project of China Academy of Chinese Medical Sciences (CI2021A04701).
Conflict of interest
The authors declare that the research was conducted in the absence of any commercial or financial relationships that could be construed as a potential conflict of interest.
Publisher's note
All claims expressed in this article are solely those of the authors and do not necessarily represent those of their affiliated organizations, or those of the publisher, the editors and the reviewers. Any product that may be evaluated in this article, or claim that may be made by its manufacturer, is not guaranteed or endorsed by the publisher.
Supplementary material
The Supplementary Material for this article can be found online at: https://www.frontiersin.org/articles/10.3389/fcvm.2024.1449185/full#supplementary-material
References
1. Phipps MS, Cronin CA. Management of acute ischemic stroke. Br Med J. (2020) 368:l6983. doi: 10.1136/bmj.l6983
2. Pu L, Wang L, Zhang R, Zhao T, Jiang Y, Han L. Projected global trends in ischemic stroke incidence, deaths and disability-adjusted life years from 2020 to 2030. Stroke. (2023) 54(5):1330–9. doi: 10.1161/strokeaha.122.040073
3. Zhang R, Liu H, Pu L, Zhao T, Zhang S, Han K, et al. Global burden of ischemic stroke in young adults in 204 countries and territories. Neurology. (2023) 100(4):e422–34. doi: 10.1212/wnl.0000000000201467
4. GBD 2016 Stroke Collaborators. Global, regional, and national burden of stroke, 1990–2016: a systematic analysis for the global burden of disease study 2016. Lancet Neurol. (2019) 18(5):439–58. doi: 10.1016/s1474-4422(19)30034-1
5. Xiong Y, Wakhloo AK, Fisher M. Advances in acute ischemic stroke therapy. Circ Res. (2022) 130(8):1230–51. doi: 10.1161/circresaha.121.319948
6. Tuo QZ, Zhang ST, Lei P. Mechanisms of neuronal cell death in ischemic stroke and their therapeutic implications. Med Res Rev. (2022) 42(1):259–305. doi: 10.1002/med.21817
7. Owolabi MO, Thrift AG, Mahal A, Ishida M, Martins S, Johnson WD, et al. Primary stroke prevention worldwide: translating evidence into action. Lancet Public Health. (2022) 7(1):e74–85. doi: 10.1016/s2468-2667(21)00230-9
8. Broocks G, Kemmling A, Aberle J, Kniep H, Bechstein M, Flottmann F, et al. Elevated blood glucose is associated with aggravated brain edema in acute stroke. J Neurol. (2020) 267(2):440–8. doi: 10.1007/s00415-019-09601-9
9. Wang X, Feng B, Huang Z, Cai Z, Yu X, Chen Z, et al. Relationship of cumulative exposure to the triglyceride-glucose index with ischemic stroke: a 9-year prospective study in the kailuan cohort. Cardiovasc Diabetol. (2022) 21(1):66. doi: 10.1186/s12933-022-01510-y
10. Johnston KC, Bruno A, Pauls Q, Hall CE, Barrett KM, Barsan W, et al. Intensive vs standard treatment of hyperglycemia and functional outcome in patients with acute ischemic stroke: the shine randomized clinical trial. Jama. (2019) 322(4):326–35. doi: 10.1001/jama.2019.9346
11. DeFronzo RA, Norton L, Abdul-Ghani M. Renal, metabolic and cardiovascular considerations of sglt2 inhibition. Nat Rev Nephrol. (2017) 13(1):11–26. doi: 10.1038/nrneph.2016.170
12. Brown E, Heerspink HJL, Cuthbertson DJ, Wilding JPH. Sglt2 inhibitors and glp-1 receptor agonists: established and emerging indications. Lancet. (2021) 398(10296):262–76. doi: 10.1016/s0140-6736(21)00536-5
13. Kelsey MD, Newby LK. In cv disease, glp-1 ras and sglt2 inhibitors reduce cv mortality. Ann Intern Med. (2022) 175(3):Jc26. doi: 10.7326/j22-0003
14. Tsai PC, Chuang WJ, Ko AM, Chen JS, Chiu CH, Chen CH, et al. Neutral effects of sglt2 inhibitors in acute coronary syndromes, peripheral arterial occlusive disease, or ischemic stroke: a meta-analysis of randomized controlled trials. Cardiovasc Diabetol. (2023) 22(1):57. doi: 10.1186/s12933-023-01789-5
15. Zhou Z, Jardine MJ, Li Q, Neuen BL, Cannon CP, de Zeeuw D, et al. Effect of sglt2 inhibitors on stroke and atrial fibrillation in diabetic kidney disease: results from the credence trial and meta-analysis. Stroke. (2021) 52(5):1545–56. doi: 10.1161/strokeaha.120.031623
16. Yang YS, Chen HH, Huang CN, Hsu CY, Hu KC, Kao CH. Glp-1ras for ischemic stroke prevention in patients with type 2 diabetes without established atherosclerotic cardiovascular disease. Diabetes Care. (2022) 45(5):1184–92. doi: 10.2337/dc21-1993
17. Wishart DS, Feunang YD, Guo AC, Lo EJ, Marcu A, Grant JR, et al. Drugbank 5.0: a major update to the drugbank database for 2018. Nucleic Acids Res. (2018) 46(D1):D1074–d1082. doi: 10.1093/nar/gkx1037
18. Zdrazil B, Felix E, Hunter F, Manners EJ, Blackshaw J, Corbett S, et al. The chembl database in 2023: a drug discovery platform spanning multiple bioactivity data types and time periods. Nucleic Acids Res. (2024) 52(D1):D1180–d1192. doi: 10.1093/nar/gkad1004
19. Li T, Xu H, Teng S, Suo M, Bahitwa R, Xu M, et al. Modeling 0.6 million genes for the rational design of functional cis-regulatory variants and de novo design of cis-regulatory sequences. Proc Natl Acad Sci U S A. (2024) 121(26):e2319811121. doi: 10.1073/pnas.2319811121
20. Mostafavi H, Spence JP, Naqvi S, Pritchard JK. Systematic differences in discovery of genetic effects on gene expression and complex traits. Nat Genet. (2023) 55(11):1866–75. doi: 10.1038/s41588-023-01529-1
21. Võsa U, Claringbould A, Westra HJ, Bonder MJ, Deelen P, Zeng B, et al. Large-scale cis- and trans-eqtl analyses identify thousands of genetic loci and polygenic scores that regulate blood gene expression. Nat Genet. (2021) 53(9):1300–10. doi: 10.1038/s41588-021-00913-z
22. Malik R, Chauhan G, Traylor M, Sargurupremraj M, Okada Y, Mishra A, et al. Multiancestry genome-wide association study of 520,000 subjects identifies 32 loci associated with stroke and stroke subtypes. Nat Genet. (2018) 50(4):524–37. doi: 10.1038/s41588-018-0058-3
23. Xu L, Zhang R, Zhang X, Liu B, Huang D, Liu Y, et al. Plasma proteomes and genome-wide association data for causal protein identification in stroke. Mol Neurobiol. (2024). doi: 10.1007/s12035-024-04411-1. [Epub ahead of print].
24. Larsson SC, Butterworth AS, Burgess S. Mendelian randomization for cardiovascular diseases: principles and applications. Eur Heart J. (2023) 44(47):4913–24. doi: 10.1093/eurheartj/ehad736
25. Sanna S, van Zuydam NR, Mahajan A, Kurilshikov A, Vich Vila A, Võsa U, et al. Causal relationships among the gut microbiome, short-chain fatty acids and metabolic diseases. Nat Genet. (2019) 51(4):600–5. doi: 10.1038/s41588-019-0350-x
26. Li L, Zhang Y, Liu X, Li J, Yang Q, Jiang J, et al. Potential causal association between aspirin use and the reduced risk of hayfever or allergic rhinitis: a Mendelian randomization study. Front Immunol. (2023) 14:1232981. doi: 10.3389/fimmu.2023.1232981
27. Nazarzadeh M, Pinho-Gomes AC, Smith Byrne K, Canoy D, Raimondi F, Ayala Solares JR, et al. Systolic blood pressure and risk of valvular heart disease: a Mendelian randomization study. JAMA Cardiol. (2019) 4(8):788–95. doi: 10.1001/jamacardio.2019.2202
28. Hartwig FP, Davey Smith G, Bowden J. Robust inference in summary data Mendelian randomization via the zero modal pleiotropy assumption. Int J Epidemiol. (2017) 46(6):1985–98. doi: 10.1093/ije/dyx102
29. Burgess S, Scott RA, Timpson NJ, Davey Smith G, Thompson SG. Using published data in Mendelian randomization: a blueprint for efficient identification of causal risk factors. Eur J Epidemiol. (2015) 30(7):543–52. doi: 10.1007/s10654-015-0011-z
30. Bowden J, Davey Smith G, Burgess S. Mendelian Randomization with invalid instruments: effect estimation and bias detection through egger regression. Int J Epidemiol. (2015) 44(2):512–25. doi: 10.1093/ije/dyv080
31. Bowden J, Davey Smith G, Haycock PC, Burgess S. Consistent estimation in Mendelian randomization with some invalid instruments using a weighted median estimator. Genet Epidemiol. (2016) 40(4):304–14. doi: 10.1002/gepi.21965
32. Verbanck M, Chen CY, Neale B, Do R. Detection of widespread horizontal pleiotropy in causal relationships inferred from Mendelian randomization between complex traits and diseases. Nat Genet. (2018) 50(5):693–8. doi: 10.1038/s41588-018-0099-7
33. Zhou L, Gao H, Zhang J, Xu Q, Wang Q, Wang L, et al. Metabolic syndrome and cancer risk: a two-sample Mendelian randomization study of European ancestry. Int J Surg. (2024). doi: 10.1097/js9.0000000000001926. [Epub ahead of print].
34. Bowden J, Del Greco MF, Minelli C, Zhao Q, Lawlor DA, Sheehan NA, et al. Improving the accuracy of two-sample summary-data Mendelian randomization: moving beyond the nome assumption. Int J Epidemiol. (2019) 48(3):728–42. doi: 10.1093/ije/dyy258
35. Denorme F, Portier I, Kosaka Y, Campbell RA. Hyperglycemia exacerbates ischemic stroke outcome independent of platelet glucose uptake. J Thromb Haemost. (2021) 19(2):536–46. doi: 10.1111/jth.15154
36. Nauck MA, Quast DR, Wefers J, Meier JJ. Glp-1 receptor agonists in the treatment of type 2 diabetes - state-of-the-art. Mol Metab. (2021) 46:101102. doi: 10.1016/j.molmet.2020.101102
37. Bellastella G, Maiorino MI, Longo M, Scappaticcio L, Chiodini P, Esposito K, et al. Glucagon-like peptide-1 receptor agonists and prevention of stroke systematic review of cardiovascular outcome trials with meta-analysis. Stroke. (2020) 51(2):666–9. doi: 10.1161/strokeaha.119.027557
38. Hernandez AF, Green JB, Janmohamed S, D'Agostino RB Sr., Granger CB, Jones NP, et al. Albiglutide and cardiovascular outcomes in patients with type 2 diabetes and cardiovascular disease (harmony outcomes): a double-blind, randomised placebo-controlled trial. Lancet. (2018) 392(10157):1519–29. doi: 10.1016/s0140-6736(18)32261-x
39. Pfeffer MA, Claggett B, Diaz R, Dickstein K, Gerstein HC, Køber LV, et al. Lixisenatide in patients with type 2 diabetes and acute coronary syndrome. N Engl J Med. (2015) 373(23):2247–57. doi: 10.1056/NEJMoa1509225
40. Ou SM, Shih CJ, Chao PW, Chu H, Kuo SC, Lee YJ, et al. Effects on clinical outcomes of adding dipeptidyl peptidase-4 inhibitors versus sulfonylureas to metformin therapy in patients with type 2 diabetes mellitus. Ann Intern Med. (2015) 163(9):663–72. doi: 10.7326/m15-0308
41. Scirica BM, Bhatt DL, Braunwald E, Steg PG, Davidson J, Hirshberg B, et al. Saxagliptin and cardiovascular outcomes in patients with type 2 diabetes mellitus. N Engl J Med. (2013) 369(14):1317–26. doi: 10.1056/NEJMoa1307684
42. Zhu Y, Li M, Wang H, Yang F, Pang X, Du R, et al. Genetically proxied antidiabetic drugs targets and stroke risk. J Transl Med. (2023) 21(1):681. doi: 10.1186/s12967-023-04565-x
43. Schmidt AF, Finan C, Gordillo-Marañón M, Asselbergs FW, Freitag DF, Patel RS, et al. Genetic drug target validation using Mendelian randomisation. Nat Commun. (2020) 11(1):3255. doi: 10.1038/s41467-020-16969-0
44. Davey Smith G, Hemani G. Mendelian Randomization: genetic anchors for causal inference in epidemiological studies. Hum Mol Genet. (2014) 23(R1):R89–98. doi: 10.1093/hmg/ddu328
45. Zheng J, Baird D, Borges MC, Bowden J, Hemani G, Haycock P, et al. Recent developments in Mendelian randomization studies. Curr Epidemiol Rep. (2017) 4(4):330–45. doi: 10.1007/s40471-017-0128-6
46. Zuber V, Grinberg NF, Gill D, Manipur I, Slob EAW, Patel A, et al. Combining evidence from Mendelian randomization and colocalization: review and comparison of approaches. Am J Hum Genet. (2022) 109(5):767–82. doi: 10.1016/j.ajhg.2022.04.001
47. Giambartolomei C, Vukcevic D, Schadt EE, Franke L, Hingorani AD, Wallace C, et al. Bayesian Test for colocalisation between pairs of genetic association studies using summary statistics. PLoS Genet. (2014) 10(5):e1004383. doi: 10.1371/journal.pgen.1004383
48. Hukku A, Pividori M, Luca F, Pique-Regi R, Im HK, Wen X. Probabilistic colocalization of genetic variants from complex and molecular traits: promise and limitations. Am J Hum Genet. (2021) 108(1):25–35. doi: 10.1016/j.ajhg.2020.11.012
49. Packer M, Wilcox CS, Testani JM. Critical analysis of the effects of sglt2 inhibitors on renal tubular sodium, water and chloride homeostasis and their role in influencing heart failure outcomes. Circulation. (2023) 148(4):354–72. doi: 10.1161/circulationaha.123.064346
50. Ghezzi C, Yu AS, Hirayama BA, Kepe V, Liu J, Scafoglio C, et al. Dapagliflozin binds specifically to sodium-glucose cotransporter 2 in the proximal renal tubule. J Am Soc Nephrol. (2017) 28(3):802–10. doi: 10.1681/asn.2016050510
51. Kutz A, Kim DH, Wexler DJ, Liu J, Schneeweiss S, Glynn RJ, et al. Comparative cardiovascular effectiveness and safety of sglt-2 inhibitors, glp-1 receptor agonists, and dpp-4 inhibitors according to frailty in type 2 diabetes. Diabetes Care. (2023) 46(11):2004–14. doi: 10.2337/dc23-0671
52. Lin TK, Chen YH, Huang JY, Liao PL, Chen MC, Pan LF, et al. Sodium-glucose co-transporter-2 inhibitors reduce the risk of new-onset stroke in patients with type 2 diabetes: a population-based cohort study. Front Cardiovasc Med. (2022) 9:966708. doi: 10.3389/fcvm.2022.966708
53. Shim B, Stokum JA, Moyer M, Tsymbalyuk N, Tsymbalyuk O, Keledjian K, et al. (2023). Canagliflozin, an inhibitor of the na(+)-coupled d-glucose cotransporter, sglt2, inhibits astrocyte swelling and brain swelling in cerebral ischemia. Cells, 12(18). doi: 10.3390/cells12182221
Keywords: ischemic stroke, novel antidiabetic agents, GLP-1 receptor agonists, SGLT-2 inhibitors, DPP-4 inhibitors, Mendelian randomization analysis
Citation: Yu Z, Liu X, Feng X, Zhang X and Gao R (2024) Causal relationship between novel antidiabetic drugs and ischemic stroke: a drug-targeted Mendelian randomization study. Front. Cardiovasc. Med. 11:1449185. doi: 10.3389/fcvm.2024.1449185
Received: 14 June 2024; Accepted: 11 September 2024;
Published: 24 September 2024.
Edited by:
Qianman Peng, Harvard Medical School and Boston, United StatesCopyright: © 2024 Yu, Liu, Feng, Zhang and Gao. This is an open-access article distributed under the terms of the Creative Commons Attribution License (CC BY). The use, distribution or reproduction in other forums is permitted, provided the original author(s) and the copyright owner(s) are credited and that the original publication in this journal is cited, in accordance with accepted academic practice. No use, distribution or reproduction is permitted which does not comply with these terms.
*Correspondence: Rui Gao, cnVpZ2FvQDEyNi5jb20=