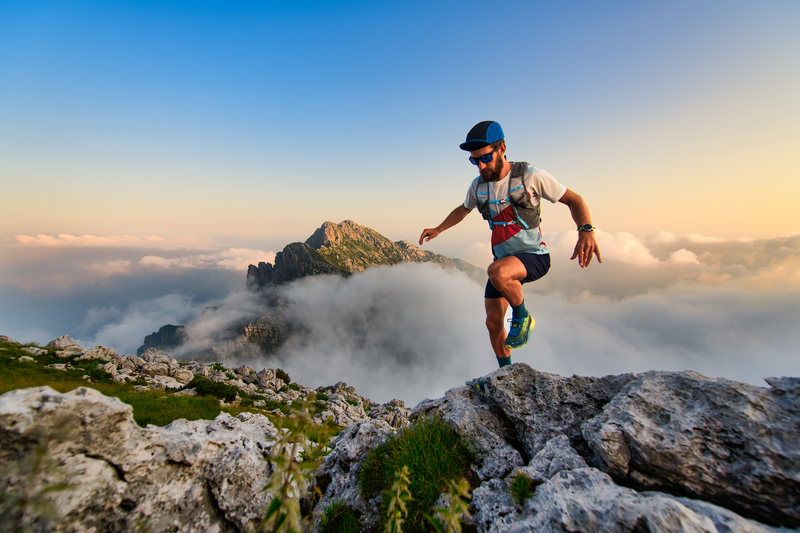
94% of researchers rate our articles as excellent or good
Learn more about the work of our research integrity team to safeguard the quality of each article we publish.
Find out more
REVIEW article
Front. Cardiovasc. Med. , 07 September 2023
Sec. Heart Failure and Transplantation
Volume 10 - 2023 | https://doi.org/10.3389/fcvm.2023.1231000
This article is part of the Research Topic Virtual Reality in Acute Cardiovascular Care View all 5 articles
The widespread adoption of mobile technologies offers an opportunity for a new approach to post-discharge care for patients with heart failure (HF). By enabling non-invasive remote monitoring and two-way, real-time communication between the clinic and home-based patients, as well as a host of other capabilities, mobile technologies have a potential to significantly improve remote patient care. This literature review summarizes clinical evidence related to virtual healthcare (VHC), defined as a care team + connected devices + a digital solution in post-release care of patients with HF. Searches were conducted on Embase (06/12/2020). A total of 171 studies were included for data extraction and evidence synthesis: 96 studies related to VHC efficacy, and 75 studies related to AI in HF. In addition, 15 publications were included from the search on studies scaling up VHC solutions in HF within the real-world setting. The most successful VHC interventions, as measured by the number of reported significant results, were those targeting reduction in rehospitalization rates. In terms of relative success rate, the two most effective interventions targeted patient self-care and all-cause hospital visits in their primary endpoint. Among the three categories of VHC identified in this review (telemonitoring, remote patient management, and patient self-empowerment) the integrated approach in remote patient management solutions performs the best in decreasing HF patients' re-admission rates and overall hospital visits. Given the increased amount of data generated by VHC technologies, artificial intelligence (AI) is being investigated as a tool to aid decision making in the context of primary diagnostics, identifying disease phenotypes, and predicting treatment outcomes. Currently, most AI algorithms are developed using data gathered in clinic and only a few studies deploy AI in the context of VHC. Most successes have been reported in predicting HF outcomes. Since the field of VHC in HF is relatively new and still in flux, this is not a typical systematic review capturing all published studies within this domain. Although the standard methodology for this type of reviews was followed, the nature of this review is qualitative. The main objective was to summarize the most promising results and identify potential research directions.
Heart failure (HF) represents a growing social burden that significantly impacts the quality of life of individual patients and imposes escalating costs on healthcare systems (1). Recent data in the US show that an estimated 6.2 million people have HF, but the projections are worrisome since it is expected that by 2030 more than 8 million people will have this condition (2). The rise in HF incidence is not a problem unique to the US; globally, the absolute number of HF cases increased by 91% between 1990 and 2017 and reached 64.3 million (3).
Although the introduction of new drugs and devices led to significant progress in the treatment of HF in recent years, the mortality in HF patients remains high, reaching up to 65% at 5 years after diagnosis (4, 5). One reason for this is that only a small proportion of patients with HF achieve optimal doses of recommended HF therapy (6), and treatment adherence remains a persistent challenge in this population (7). In addition, HF typically evolves by bouts of hemodynamic deterioration, triggered by a vast number of factors leading to frequent and high-mortality hospitalizations (8). Therefore, the traditional in-clinic monitoring of HF patients is often inadequate to capture early signs of deterioration in time. Given that the earliest detectable changes in physiological measurements might occur prior to the onset of symptoms (6), timely detection of impending crisis is crucial to avoid hospitalization and premature death.
The widespread adoption of mobile technologies offers an opportunity for implementing solutions that can capture the early signs of cardiac decompression and provide timely intervention. Non-invasive remote monitoring and two-way, real-time communication between the clinic and home-based patients (9, 10), are already being tested in pilot trials in the HF population.
In the literature, these solutions are alternately referred to as telehealth, telemedicine, eHealth, mHealth, and several other creative names, reflecting lack of consensus on a proper terminology in the field. This review will adopt the term “virtual healthcare” (VHC), broadly defined as the remote delivery of healthcare via connected devices, mobile phones or tablets, and related internet technologies. The efficacy of VHC interventions is still in the early stages of investigations, with several studies reporting encouraging results in HF populations (11, 12), although questions regarding the comparative effectiveness of specific devices remain open (13).
While defining the objectives of this review, the authors noted that one neglected research topic is the challenge related to upscaling of the results of small-size clinical trials into real-world settings. In contrast to established procedures of real-world drug and medical device development, approval, and distribution, widespread utilization of VHC solutions poses additional challenges. The logistics of VHC deployment among the patients and caregivers require a much more active role of patients as well as an increased level of cooperation and shared decision making among healthcare professionals. In addition, the large amount of data gathered by mobile devices necessitate new approaches to data acquisition, storage, and analysis. Here, the adaptation of artificial intelligence algorithms (AI) will be necessary not only to process the real-time data inflow but also to aid in advanced diagnostics, personalization, and decision-making. These issues are largely neglected in existing clinical trials, where the focus is mainly on the comparative efficacy and utility of VHC interventions.
This review surveys the current state of VHC in HF with a focus on the practical aspect of VHC implementations and its challenges in real-world situations, summarizing existing evidence on methodology, efficacy, and integration into routine clinical settings.
Standard methodology for conducting and reporting systematic reviews recommended by the Cochrane Collaboration's Handbook for Systematic Reviews of Interventions was adapted to conduct a literature review (14). The review surveyed VHC solutions in patients with HF with the research objective to describe and characterize the landscape of evidence of the past 5 years (2015–2020) of the approved and in-development virtual or mobile healthcare solutions, defined as a care team + connected devices + a digital solution (e.g., a smartphone app and/or wearable devices). The results were then summarized in a narrative form.
Using predefined search strategies, Embase was searched via the Ovid platform from inception until December 6th, 2020 (Supplementary Table S1). All abstracts identified by the search were reviewed by a single reviewer according to predefined, PICOS-framed eligibility criteria (Supplementary Table S2). All studies identified as eligible during title/abstract screening were then screened at the full-text stage by two reviewers. A Preferred Reporting Items for Systematic Reviews and Meta-Analyses (PRISMA) diagram was generated for complete transparency and reproducibility of the search and screening process (15).
A standardized data extraction table was generated to define the study characteristics, patient characteristics, intervention characteristics, and outcomes that were extracted from eligible studies. Quality control procedures were undertaken during data extraction to verify the accuracy and completeness of each collected data point.
An additional search targeting studies with keywords related to scaling up VHC solutions in HF within the real-world setting was performed on September 27th, 2021 to supplement the evidence base (Supplementary Table S3). The need for additional search arose post hoc after summarizing the main body of evidence. Evidence from this search was incorporated narratively into the results.
One of the results of reviewing the included studies and observing shared methodological patterns was identification of three distinct paradigms of VHC interventions. These three paradigms were distinguished by the clinical objectives targeted by the VHC interventions:
○ Telemonitoring: remote monitoring of cardiac and extra-cardiac variables with regular uploading and evaluation of the data at the centre in order to detect early signs of cardiac decompensation.
○ Remote patient management (RPM): an integrated healthcare solution that includes telemonitoring as its part, but it also provides a service platform for real-time interactive patient-clinician communications.
○ Self-care: solutions that empower patients in their independent decision-making process of sustaining health through disease education, symptom monitoring, treatment-seeking, and evaluating the effects of treatment.
The post hoc rationale for this classification and examples of each category are below:
• The telemonitoring studies used as a part of the intervention a device or a mobile app measuring clinical variable(s) with regularly scheduled automatic data uploads. Data uploads did not require the patient's involvement, and data evaluation was performed by medical staff at the health centre. A typical example is a study evaluating the efficacy of a device for remote monitoring of lung fluid by measuring dielectric properties of tissues (16). Measurements were transmitted via a cellular data link to a secured server for review by a health care professional using a dedicated web-based electronic data capture and viewing system.
• The RPM studies included, in addition to telemonitoring, direct regular interactive communication between the patient and the healthcare centre. The main purpose was to establish regular human-to-human contact and to offer medical advice, answering patients' questions, and provide encouragement. The communications channels usually included videoconferencing, phone calls, or dedicated websites.
• The self-care interventions were usually stand-alone apps (17) or self-contained devices such as an accelerometer (18) providing patients feedback and information about their condition and advice for behavioural changes to improve their health and well-being.
The AI in the context of this review is defined as machine-based data processing to achieve objectives that typically require human cognitive function (19). The studies reporting on the use of AI in HF can be broadly classified into three groups based on the purpose of the algorithms:
• Primary diagnostics: the algorithms are used to identify patients with HF among a wider population of patients either in primary care (20) or among hospitalized patients (21).
• Phenotype identification: the algorithms are used to identify different phenotypes within the primary diagnosis of HF by evaluating the associations of a variety of clinical parameters with pre-specified subgroups of patients (22–25). The word “phenotype” in the studies was used in broad sense and the identified phenotypes did not always correspond to traditional clinical phenotypes as understood by cardiologists. This was especially true for unsupervised learning algorithms (26–28) where the data set was comprised of large number of extracted features and the algorithm was trying to find clusters in hyperdimensional feature space. The resulting clusters were then interpreted post hoc by the researchers.
• Outcome prediction: this was the largest set of studies, where the algorithms were used to predict outcomes such as mortality and hospitalization risks (29–31), re-admission (32–34), tissue remodelling (35, 36), and a variety of other clinical outcomes (22, 37–39).
A PRISMA flow diagram of the study selection procedure is presented in Supplementary Figure S1. A total of 171 studies were included for data extraction and evidence synthesis: 96 studies related to VHC efficacy, and 75 studies related to AI in HF. In addition, 15 publications were included from the search on studies scaling up VHC solutions in HF within the real-world setting (PRISMA flow diagram in Supplementary Figure S2).
Below is the summary of the results for the efficacy data set of VHC in HF. Table 1 shows the distribution of the study types for the 96 included studies.
Table 1. The number of included studies of each respective study type in the efficacy evidence base.
The population sizes ranged from 10 patients (40, 41) to 3,449 patients in one study retrospectively analysing adherence to a telehealth program in US Veteran Administration centres (42). The mean population size in the included studies was 311 patients, and the median was 110 patients. This discrepancy is caused by the one outlier study, including 3,449 patients. The summary of study characteristics and population characteristics can be seen in supplementary materials (Supplementary Figures S3, S4).
The inclusion criteria for patient enrolment in most of the studies were based on the New York Heart Association (NYHA) functional classification (43). Seventy out of the 96 included studies used this classification for patient inclusion. Twenty-one of the 96 included studies enrolled only patients with reduced ejection fraction defined as less or equal to 40%. Forty studies enrolled a mixture of patients with both reduced and preserved ejection fractions. Thirty-five studies did not include information about ejection fraction (EF) status among the patients. Nineteen studies reported average EF of the enrolled population ranging from the minimum average EF of 21.5% (44) to maximum of 58% (45) with overall mean of 34.1%. Four studies enrolled patients with wearable cardioverter defibrillators which were used for remote data acquisition (44, 46–48).
The three VHC categories (telemonitoring, RPM, self-care) were used to compare clinical efficacy of the interventions. Table 2 shows the total number of studies and the number of RCTs within each of these categories. Both the telemonitoring and remote care studies used some form of remote data capture and upload. The types of patient data captured varied across the studies. The most commonly monitored data were self-reported patient symptoms (40, 49, 74, 75, 124), physical activity measured by accelerometers (18, 46, 125–127), body weight, blood pressure, and heart rate (50, 76–79), data transmitted by cardioverter defibrillators (44, 46–48, 76), and others.
There were 27 distinct primary outcomes reported across all included studies. The top ten most reported primary outcomes, together with the number of studies reporting significant results in the respective primary outcome, are summarized in Table 3. The data for all included studies, as well as the subset of data pertaining only to RCTs, are shown.
Medication adherence was generally reported as patients following the prescription schedule for drug taking for a significant fraction (usually >80%) of the study duration. Quality of life was measured using HF-specific questionnaires such as The Minnesota Living with Heart Failure Questionnaire or Kansans City Cardiomyopathy Questionnaire. Depression was evaluated using 9-item Patient Health Questionnaire. Self-care is a growing area of interest in all chronic diseases, and it was defined (with a few variations) as a process of maintaining health through health-promoting practices and by managing illness (e.g., by exercising, weight monitoring, taking medication, and seeking a health care provider when symptoms are deteriorating).
In the “Other” category, the most reported primary outcome was based on the series of publications related to the TIM-HF and TIM-HF2 trials (80–83). The primary outcome in this series was “the percentage of days lost due to unplanned cardiovascular hospital admissions and all-cause mortality”. In addition, the category “Other” also includes outcomes such as “cardiac acoustic biomarkers” (47), composite outcomes (51–54, 125), non-fatal HF episode (45), general health status (55, 74), functional capacity (128), and other unique endpoints.
The most frequently investigated primary outcome across all studies was the patient experience, loosely defined as “usability” (56, 84), “satisfaction/acceptability” (85, 124), “goal attainment or life satisfaction” (17), and variations thereof. However, most of these studies were non-comparative by design and therefore they described the outcome in a single arm. The two RCTs investigating patient experience (56, 86) reported high levels of patient satisfaction in both arms (with and without VHC), with no significant differences observed. The majority of the studies used the RPM paradigm (9/17, 53%), followed by telemonitoring (4/17, 23.5%), and self-care (4/17, 23.5%).
The most successful VHC interventions, as measured by the number of reported significant results, were those targeting reduction in rehospitalization rates. Eight (16, 57, 58, 87–90, 127) out of 15 (53%) studies reported significant reduction in rates of rehospitalizations, including one RCT (88). The majority of the interventions targeting rehospitalization rates used RPM approach (8/15, 53%), followed by telemonitoring (6/15, 40%), and self-care (1/15, 7%).
In terms of relative success rate, the two most effective interventions targeted patient self-care and all-cause hospital visits in their primary endpoint. Six (59, 91–93, 129, 130) out of seven (85.7%) studies targeting improved self-care reported significant results. Interestingly, even for self-care primary endpoints, the most common intervention paradigm was RPM (4/7, 57.2%), followed by self-care paradigm (2/7, 28.5%) and telemonitoring (1/7, 14.3%). Four (60, 94–96) out of five (80%) studies reported significant improvements in reducing all-cause hospital visits in the HF population. Three out of the five studies (60%) in this category used the RPM paradigm, and two (40%) used telemonitoring.
Patient adherence as the primary endpoint was investigated in 12 studies, four of which were RCTs (61, 84, 133, 134). Two of the four RCTs reported significant improvement due to the VHC intervention (133, 134). In both cases the remote care model of intervention was used, with healthcare professionals aiding patients in adopting the technologies into their everyday routines. Both studies noted increased self-awareness of patients when managing their condition.
The total of 75 studies reporting on the AI algorithms in HF were included in the evidence base. Table 4 shows the distribution of the studies across the three categories described in the Methods section, together with the validation status. Most studies used internal validation of the algorithms either by splitting data into a training set and validation set and re-sampling the sets 5–10 times for cross-validation (30, 135–137) or leave-one-out (76, 138, 139) method. The data used for training and validation of the algorithms came from a variety of sources such as clinical trial database (30, 138), electronic health records (34, 140–142), or internal institutional databases (143, 144).
Currently, the majority of AI studies are using data gathered by routine in-clinic laboratory procedures such as patient characteristics (37), cardiovascular MRI (145), ECG (146) and others. The outputs of the algorithms can be classified into three general domains (see the Methods section):
• Primary diagnostics algorithms are being developed with the purpose to use readily available clinical data to identify patients with heart failure while in the hospital. Input data are gathered from electronic health records (20, 21, 141) or internal hospital databases (147, 148). Outputs of the algorithms are aimed at helping clinicians in correct diagnosis of HF in patients admitted for a variety of cardiac conditions.
• Phenotype identification algorithms are used to identify sub-groups of HF patients either using a pre-defined classification or using clustering methods to discover hidden groupings within the data sets. In the first case, algorithms are identifying phenotypes such as HF patients with cardiac amyloidosis relying on routinely determined laboratory parameters (22), diagnosing PLN p.Arg14del cardiomyopathy using ECG (23), or identifying responders to cardiac resynchronization therapy using 2D echocardiography data (24). Novel sub-groups discovered in studies using clustering algorithms included three distinct phenotypes in patients with HFpEF that may respond differently to treatments or interventions (26), six HFpEF phenotypes, for which significant differences in the prevalence of concomitant atrial fibrillation, anaemia and kidney disease were observed (27), and four subgroups in patients with hypertrophic cardiomyopathy with distinct ECG features leading to a novel risk stratification (28).
• Outcome prediction was the largest group of studies. The outcomes of interest varied across the studies but most of the algorithms focused on predicting treatment responses in terms of mortality (30, 31), re-hospitalization (149, 150), adverse events (39) and other related outcomes.
Out of the 75 papers on AI in HF, only eight were set in the context of VHC where the data was acquired remotely:
• Primary diagnostics was the goal of one study (151) that investigated whether wristband data can be used to predict a diagnosis of HF in a cohort of 97 monitored cardiac inpatients. The AI algorithm performed best when the wearable data was combined with demographics, medical history, and vital signs. The achieved discrimination defined by the test area under the curve (AUC) was 0.87 with the specificity of 72% and sensitivity of 90% (151).
• Phenotype identification was investigated in one study (152). The study explored the use of unsupervised machine learning to identify subgroups of patients with HF who used telehealth services in the home health setting. The study identified patterns of association between (1) mental health status, pulmonary disorders, and obesity and (2) healthcare utilization for patients with heart failure who used telehealth in the home health setting (152).
• Outcome prediction was investigated in the remaining six studies (76, 149, 150, 153–155). Two studies used retrospective data sets to predict clinical outcomes: one study analysed data from the Telemonitoring to Improve Heart Failure Outcomes trial to predict readmissions in patients with HF (149), and one study developed a model for cardiomyopathy prediction (154). Four clinical trials in this group incorporated AI and VHC prospectively into the study design. One study (150) examined the performance of a personalized analytical platform using continuous data streams to predict rehospitalization after HF admission (LINK-HF study). Participants were monitored for up to 3 months using a disposable multisensor patch placed on the chest that recorded physiological data. Data were uploaded continuously via smartphone to a cloud analytics platform. Machine learning was used to design a prognostic algorithm to detect HF exacerbation. The cloud-based analytics platform used a general machine learning method of similarity-based modelling to analyse collected data. The algorithm used a 1-minute trim-mean (10%) heart rate, respiratory rate, a cumulative gross activity, and posture as inputs. The platform was able to detect precursors of hospitalization for HF exacerbation with 76%–88% sensitivity and 85% specificity. The median time between initial alert and readmission was 6.5 (4.2–13.7) days (150). One study developed and validated an algorithm to predict the occurrence of ventricular tachyarrhythmia in HF patients with implantable-cardioverter defibrillator (153). The algorithm used heart rate variability data and machine learning to automatically predict ventricular arrhythmia. The algorithm achieved performance quantified by AUC of 0.81 for 5-minute prediction and mean AUC of 0.87–0.88 for 10 s prediction (153). One study (76) investigated whether certain user characteristics (i.e., personal and clinical variables) predict the use of remote monitoring systems (RMS) using advanced machine learning software algorithms in patients with HF. The data support that RMS use was higher in patients who did not receive care from a healthcare provider with HF specialty. The study findings also showed that participants who had an internal cardioverter defibrillator were more likely to use the RMS (76). One study (155) tested a telephone intervention algorithm for monitoring ventricular assist devices (VAD) in outpatients with HF. A structured inquiry was used to gather information on pump parameters, vitals, and symptoms which was then electronically categorized by an algorithm into five levels of severity. Propensity-adjusted 2-year survival (89% vs. 57%, P = 0.027) was significantly higher for the telephone intervention group compared to standard of care group (155).
The challenges of scaling up VHC solutions to real-world implementation have been mostly neglected in the pilot clinical trials and only addressed in a handful of studies. Hovland-Tanneryd et al. (94) published their experience of validation of a home-based tool for HF patients, previously tested in an RCT, in a cohort in a primary care setting in a clinical controlled trial. The aim was to compare the RCT findings to the more pragmatic design of a validation project in primary care. Data from both trials were analysed with respect to HF-related in-hospital days, self-care behaviour, and system adherence during a 6-month intervention. The results in both settings were similar in terms of the risk ratio of in-hospital days (RCT RR = 0.72, Clinic RR = 0.67), and the pooled data set showed improvement in self-care by 27%, with median adherence of 94% (94).
Several studies focused on patient experience and barriers to acceptance in a wider population of HF patients (156–160). The most common themes explored were regimen complexity, forgetfulness, difficulty coping with side effects (159), convenience (157), trust, perceived risk (160), and added responsibility for nurses and caregivers (158). Elderly patients with HF face additional barriers to adopting VHC. A study by Cajita et al. found that older adults do not base their intention to use mHealth solely on perceived ease of use and perceived usefulness. Instead, the following themes emerged from the content analysis: facilitators included previous experience with mobile technology, willingness to learn mHealth, ease of use, presence of useful features, adequate training, free equipment, and doctor's recommendation (156).
The emergence of COVID-19 accelerated the adoption of VHC in the real-world management of HF patients (161, 162). It also revealed the challenges of transitioning the care delivery and administrative organization to conform to a new healthcare environment while still providing high-quality care. Sayer et al. (163) described the experience of a large tertiary HF program in widespread adoption of telehealth, restructuring outpatient care, initiating a shared clinic model, and introducing a comprehensive remote monitoring program to manage patients with HF and heart transplants. The transition employed a high-intensity telehealth approach, centralized monitoring and intervention program, and the development of video conference-based support groups. It also required a collaborative approach, with contributions from dedicated teams of inpatient clinicians, outpatient clinicians, and administrative staff (163).
The technologies used in VHC solutions are relatively new, and consequently, their fit into the existing models of HF patient care is still being explored in a variety of settings. When considering the results reported in the reviewed studies, the challenge is in translating them into the real-world clinical practice. Unfortunately, few studies addressed the issue of scalability of the VHC solutions and potential issues that may impede their acceptance. In order to carefully assess the available pathways towards the wide deployment of VHC in HF populations one need to start by looking at what works in the context of clinical trials and then try to identify potential roadblocks in the scaling-up those solutions.
From the standpoint of treatment efficacy, the RPM approach seems to be most effective among the three intervention categories (Figure 1). When looking at the endpoints where VHC showed the most success (rehospitalization, self-care, all-cause hospital visits), the majority of interventions used RPM paradigm. The multi-component intervention included telemonitoring devices with the daily upload of clinical measurements as well as communications with patients' general physician and cardiologist (80, 81). A similar approach integrating automated data monitoring with personalized communications was found in a majority of the studies (see Table 2). The RPM intervention could be as simple as a tool to encourage HF patients to work collaboratively with their clinicians to “make one positive change” in medication regimen (97), or a fully integrated system including a multidisciplinary care team consisting of a nurse coordinator, cardiologist, psychiatrist, and primary care physician, home telemonitoring, patient self-management support, and screening and treatment for comorbid depression (74). RPM was also used to interactively guide physical exercise in HF patients (94, 98) or to organize post-discharge remote visits and consultations (45).
The telemonitoring paradigm relies mostly on automatic data upload with limited patient involvement. Direct medical intervention is only triggered when the uploaded data cross predefined threshold signalling and impending crisis. The data upload can contain clinical variables such as lung fluid measures (16), medication taking (60, 62), vital signs (including, in some cases ECG) (50–52, 63, 64, 99), and self-reporting (55). A special case of remote monitoring concerns wearable cardioverter defibrillators, where the intervention is in part automated and implemented in the software of the wearable device (44, 46–48, 76). Historically, this paradigm pioneered the use of wearable devices and the concept of remote monitoring, however it is currently being integrated as part of the RPM model.
The concept of self-care in VHC includes a variety of approaches to patient empowerment, such as improving self-reported symptoms via mHealth apps (131), engaging the elderly patient population in technology-guided self-care (17, 124, 126, 132), and increasing self-awareness when managing patients' condition (130, 133).
There are several caveats to be considered when trying to generalize the results of the reviewed clinical trials into general practice. Most published studies are conducted in a controlled environment of research hospitals with relatively small groups of HF patients. The efficacy claims of the VHC interventions are valid within the pre-selected HF populations and limited follow-up of the studies. How will these results translate into a standard of care that can be applied to a variety of clinical settings is an open question. Even within the context of controlled studies, some results cast doubt on the efficacy of VHC interventions. For example, VHC interventions seem to have no effect on reducing all-cause mortality. Out of the five RCTs investigating this outcome none achieved significant improvement. Reasons are currently unclear and this issue merits further investigation. An interesting hypothesis to consider is the importance of direct human-to-human interactions aided by technology as reflected in the RPM paradigm. The number of significant results achieved by integrated solutions as compared to purely technology-based interventions may be, in part, explained by the presence of the human component. The technology is best utilized in support of shared decision-making by providing timely information and opening new channels for communications between patients and their care team. The main challenge going forward is to maintain this advantage in large-scale settings of existing healthcare systems.
With a few exceptions, the study durations did not extend beyond a 1-year follow-up. Given that the average lifespan of patients discharged from hospital with HF is 5.5 years (164), extending up to 19.5 years in a younger, low-risk population, the lack of long-term studies in this field represents an unmet need. In addition, HF patient populations span generations of patients starting from middle-aged “Gen-X”, through baby-boomers, to geriatric patients (see Supplementary Figure S4). Technology acceptance varies greatly among generations, hence a personalized approach is needed to achieve desired utilization. Here the studies analysing user experiences and barriers to acceptance are particularly valuable. For example, Woo et al. 2018 analysed factors facilitating/hindering the acceptance of VHC services in HF patients (165). Using a modified Unified Theory of Acceptance Use of Technology, the authors identified several factors associated with HF patients' initiation or use of VHC services in a home setting, some of which are relatively straightforward to implement (e.g., high-risk drugs education by visiting nurses).
One technological challenge to the general adaptation of VHC solutions is the large amount of clinical data the devices can collect and store. Particularly in the context of general use, this can be overwhelming, and clinicians will need assistance with the analysis and interpretation of the patient data. A promising development is the introduction of AI algorithms targeting the diagnosis, phenotyping, and prognosis in HF. The utility of using AI in diagnosing HF may be in aiding the clinician in deciding ambiguous cases (i.e., being a supporting factor to the clinical judgement of the physician). For example, an existing algorithm using a simple wristband achieved 74% accuracy (151) in diagnosing chronic HF (present/absent) from data of cardiology patients undergoing bedside monitoring. More sophisticated algorithms using better data sources (e.g., ECG) may be able to achieve greater diagnostic accuracy. Similarly, algorithms detecting sub-groups of HF patients using a collection of remote data (152) can identify clusters of patients that display different patterns of comorbidities and healthcare outcomes. This information can be then used to tailor the VHC intervention to address specific challenges facing the identified sub-groups. The limitations of AI are mostly in the academic nature of the algorithms with limited availability of these technologies where they are mostly needed—in real-world practice. To achieve a larger utilization, several barriers need to be overcome, such as gaining the trust in the algorithms by clinicians (by illustrating the physiology behind the algorithms), integrating the algorithms with the current workflow, resolving problems with privacy and data sharing, and providing adequate technical support in the field.
The topic of VHC in HF has been subject to several recent systematic literature reviews (6, 8, 162, 166). In this review, the intent was to highlight the comparative efficacy of the three identified categories of interventions: telemonitoring, RPM, and self-care. While summarizing the evidence, the limitations of the included clinical studies prompted additional research, including the questions of AI utilization and the real-world scaling of the results reported by the published studies. One of the findings in this review was that studies leveraging the synergy between AI and VHC in the HF population are limited in numbers. This seems like a potential area for future research. In particular, the AI algorithms can bring expert-level decision support for diagnostics and risk prediction to non-specialist general practitioners and facilitate the scaling up of the VHC solutions into real-world settings. A large-scale study of a distributed AI algorithms aiding clinicians in HF diagnostics and risk evaluations across multitude of real-world clinics could expose the barriers and challenges to AI acceptance.
Additional promising areas of future research include the apparent lack of efficacy of VHC intervention in reducing all-cause mortality in the HF population, long-term adherence and efficacy of VHC with follow-up spanning several years, and age-specific approach to technology adaptation with focus on the elderly HF patients. Additionally, given the relative novelty of these interventions and lack of experience in utilizing them in wider clinical settings, focus on education and transfer of knowledge may help in improving their acceptance and consequently their efficacy. This is particularly true in developing countries where the distances are large, and the number of healthcare professionals are limited.
This is not a typical systematic review capturing all published studies within this domain. Although the standard methodology for this type of reviews was followed, the nature of this review is qualitative. Our intention was to provide a narrative summary of the most relevant findings related to the stated objectives of the study. The quantitative information provided here is selected based on the representativeness of the data without providing additional statistical analyses. The choice of narrative summary instead of the standard meta-analysis was made for several reasons. First, digital interventions in HF are heterogeneous both in the ways intervention is delivered and, in the variety of targeted outcomes. Unlike drug clinical trials where the methods of delivery and the measured outcomes are relatively well understood, for VHC interventions neither is true. How to deliver the intervention (e.g., text messages, phone-calls, video conferencing), frequency of the communications (e.g., daily, weekly, on-demand), and specific content of the communication are still being investigated. Methods of collecting clinical data (what to collect and how) and their upload are far from standardized. Targeted outcomes vary from easily quantifiable (mortality, re-hospitalization) to more ambiguous (patient experiences). Therefore, comparing the results using meta-analysis would require adopting many assumptions about the homogeneity of the included clinical trials. A narrative summary allows for a more qualitative description of the respective interventions although it lacks the rigor of quantitative meta-analysis and definite conclusions are more difficult to draw. However, given the exploratory nature of the clinical trials in VHC, a narrative summary may provide a useful way to survey the current state of the field.
The main finding of this review is that the promise of VHC in HF has been established in some studies but not all interventions reached their desired outcomes. The solutions with the most consistent positive impact on clinical outcomes are those that address already existing unmet needs such as early detection of warning signs of cardiac decompensation, easy patient access to the healthcare team, convenient access to relevant educational materials, and encouragement of healthy lifestyle. On the other hand, reducing mortality seems to be quite challenging and little progress has been achieved in boosting patient adherence. Artificial intelligence has a potential in interpretation of large data sets, but it is largely confined to academic institutions. Overall, while research studies administered by high-end academic institutions show some success, scaling the VHC intervention to general clinical practice remains a challenge.
KCSL and AU contributed to the study design and conceptualization. BB carried out data configuration. FK and SSM provided clinical input. All authors contributed to the article and approved the submitted version.
This work was supported by Sanofi.
We appreciate the coordination of the development of this manuscript and assistance with revisions as provided by Cecile Dessapt-Baradez, and Sai Krishnaveni Chevooru, at Sanofi. This literature review was performed by Evidinno Outcomes Research Inc., funded by Sanofi. We also thank Christopher Crotty, (Evidinno Outcomes Research Inc.) for medical writing support and Mir-Masoud Pourrahmat (Evidinno Outcomes Research Inc.) for project management, funded by Sanofi.
KCSL and AU report employment by Sanofi and may hold shares and/or stock options in the company. BB reports contract employment by Evidinno Outcomes Research Inc. FK is currently employed by Charité – Universitätsmedizin and has an ownership interest in Zentrum für kardiovaskuläre Telemedizin GmbH. FK has received research funding grants from the German Federal Ministry of Education and Research and grants from the Federal Ministry of Economic Affairs and Energy for conducting research and clinical trials in remote patient management. FK has received personal fees (honoraria) for lectures from Boston Scientific, Sanofi-Aventis Deutschland GmbH, Biotronik SE & Co. KG, Novartis, Linde/Saúde, Roche Pharma AG, Amgen GmbH and Astra Zeneca outside the submitted work. FK is co-holder of the patents PCT/EP2020/058700, European Patent Application No. 19166382.2, No. 19166425.9, No. 19175720.2. FK has received personal fees for advisory boards from Abbott, Sanofi-Aventis Deutschland GmbH and Biotronik Se & Co. KG. FK is a member of the European Society of Cardiology (ESC), German Cardiac Society (DGK), German Society for Internal Medicine (DGIM) and German Centre for Cardiovascular Research (DHZK). SSM is supported by the American Heart Association (20SFRN35380046 and COVID19-811000), PCORI (ME-2019C1-15328), NIH (P01 HL108800), the Aetna Foundation, the David and June Trone Family Foundation, the Pollin Digital Innovation Fund, the PJ Schafer Cardiovascular Research Fund, Sandra and Larry Small, CASCADE FH, Apple, Google, and Amgen. Under a license agreement between Corrie Health and the Johns Hopkins University, the University owns equity in Corrie Health, and the University and SSM are entitled to royalty distributions related to the technology. In addition, SSM is a co-founder of, and holds equity in Corrie Health. This arrangement has been reviewed and approved by Johns Hopkins University in accordance with its conflict of interest policies. SSM reports consulting in the last 36 months with Amgen, AstraZeneca, DalCor, Esperion, iHealth, Kaneka, Novartis, Novo Nordisk, Sanofi, Regeneron, REGENXBIO, and 89bio.
All claims expressed in this article are solely those of the authors and do not necessarily represent those of their affiliated organizations, or those of the publisher, the editors and the reviewers. Any product that may be evaluated in this article, or claim that may be made by its manufacturer, is not guaranteed or endorsed by the publisher.
The Supplementary Material for this article can be found online at: https://www.frontiersin.org/articles/10.3389/fcvm.2023.1231000/full#supplementary-material
1. Urbich M, Globe G, Pantiri K, Heisen M, Bennison C, Wirtz HS, et al. A systematic review of medical costs associated with heart failure in the USA (2014-2020). Pharmacoeconomics. (2020) 38(11):1219–36. doi: 10.1007/s40273-020-00952-0
2. Lund LH, Savarese G. Global public health burden of heart failure. Card Fail Rev. (2017) 03(01):7–11. doi: 10.15420/cfr.2016:25:2
3. Bragazzi NL, Zhong W, Shu J, Abu Much A, Lotan D, Grupper A, et al. Burden of heart failure and underlying causes in 195 countries and territories from 1990 to 2017. Eur J Prev Cardiol. (2021) 28:zwaa147. doi: 10.1093/eurjpc/zwaa147
4. McDonagh TA, Metra M, Adamo M, Gardner RS, Baumbach A, Bohm M, et al. 2021 Esc guidelines for the diagnosis and treatment of acute and chronic heart failure. Eur Heart J. (2021) 42(36):3599–726. doi: 10.1093/eurheartj/ehab368
5. Spitaleri G, Lupon J, Domingo M, Santiago-Vacas E, Codina P, Zamora E, et al. Mortality trends in an ambulatory multidisciplinary heart failure unit from 2001 to 2018. Sci Rep. (2021) 11(1):732. doi: 10.1038/s41598-020-79926-3
6. Bekfani T, Fudim M, Cleland JGF, Jorbenadze A, von Haehling S, Lorber A, et al. A current and future outlook on upcoming technologies in remote monitoring of patients with heart failure. Eur J Heart Fail. (2020) 23:175–85. doi: 10.1002/ejhf.2033
7. Ruppar TM, Cooper PS, Mehr DR, Delgado JM, Dunbar-Jacob JM. Medication adherence interventions improve heart failure mortality and readmission rates: systematic review and meta-analysis of controlled trials. J Am Heart Assoc. (2016) 5(6):e002606. doi: 10.1161/JAHA.115.002606
8. Silva-Cardoso J, Juanatey JRG, Comin-Colet J, Sousa JM, Cavalheiro A, Moreira E. The future of telemedicine in the management of heart failure patients. Card Fail Rev. (2021) 7:e11. doi: 10.15420/cfr.2020.32
9. Ding H, Chen SH, Edwards I, Jayasena R, Doecke J, Layland J, et al. Effects of different telemonitoring strategies on chronic heart failure care: systematic review and subgroup meta-analysis. J Med Internet Res. (2020) 22(11):e20032. doi: 10.2196/20032
10. Faragli A, Abawi D, Quinn C, Cvetkovic M, Schlabs T, Tahirovic E, et al. The role of non-invasive devices for the telemonitoring of heart failure patients. Heart Fail Rev. (2020) 26:1063–80. doi: 10.1007/s10741-020-09963-7
11. Son YJ, Lee Y, Lee HJ. Effectiveness of mobile phone-based interventions for improving health outcomes in patients with chronic heart failure: a systematic review and meta-analysis. Int J Environ Res Public Health. (2020) 17(5):1749. doi: 10.3390/ijerph17051749
12. Zhu Y, Gu X, Xu C. Effectiveness of telemedicine systems for adults with heart failure: a meta-analysis of randomized controlled trials. Heart Fail Rev. (2020) 25(2):231–43. doi: 10.1007/s10741-019-09801-5
13. Aronow WS, Shamliyan TA. Comparative effectiveness of disease management with information communication technology for preventing hospitalization and readmission in adults with chronic congestive heart failure. J Am Med Dir Assoc. (2018) 19(6):472–9. doi: 10.1016/j.jamda.2018.03.012
14. Jamal SM, Kichloo A, Albosta M, Bailey B, Singh J, Wani F, et al. In-hospital outcomes and prevalence of comorbidities in patients with infective endocarditis with and without heart blocks: insight from the national inpatient sample. J Investig Med. (2020) 69:358–63. doi: 10.1136/jim-2020-001501
15. Page MJ, McKenzie JE, Bossuyt PM, Boutron I, Hoffmann TC, Mulrow CD, et al. The prisma 2020 statement: an updated guideline for reporting systematic reviews. PLoS Med. (2021) 18(3):e1003583. doi: 10.1371/journal.pmed.1003583
16. Amir O, Ben-Gal T, Weinstein JM, Schliamser J, Burkhoff D, Abbo A, et al. Evaluation of remote dielectric sensing (reds) technology-guided therapy for decreasing heart failure re-hospitalizations. Int J Cardiol. (2017) 240:279–84. doi: 10.1016/j.ijcard.2017.02.120
17. Buck H, Pinter A, Poole E, Boehmer J, Foy A, Black S, et al. Evaluating the older adult experience of a web-based, tablet-delivered heart failure self-care program using gerontechnology principles. Geriatr Nurs. (2017) 38(6):537–41. doi: 10.1016/j.gerinurse.2017.04.001
18. McCarthy MM, Dickson VV, Katz SD, Chyun DA. An exercise counseling intervention in minority adults with heart failure. Rehabil Nurs. (2017) 42(3):146–56. doi: 10.1002/rnj.265
19. Feeny AK, Chung MK, Madabhushi A, Attia ZI, Cikes M, Firouznia M, et al. Artificial intelligence and machine learning in arrhythmias and cardiac electrophysiology. Circ Arrhythm Electrophysiol. (2020) 13(8):e007952. doi: 10.1161/CIRCEP.119.007952
20. Chen R, Stewart WF, Sun J, Ng K, Yan X. Recurrent neural networks for early detection of heart failure from longitudinal electronic health record data: implications for temporal modeling with respect to time before diagnosis, data density, data quantity, and data type. Circ Cardiovasc Qual Outcomes. (2019) 12(10):e005114. doi: 10.1161/CIRCOUTCOMES.118.005114
21. Blecker S, Sontag D, Horwitz LI, Kuperman G, Park H, Reyentovich A, et al. Early identification of patients with acute decompensated heart failure. J Card Fail. (2018) 24(6):357–62. doi: 10.1016/j.cardfail.2017.08.458
22. Agibetov A, Seirer B, Dachs TM, Koschutnik M, Dalos D, Rettl R, et al. Machine learning enables prediction of cardiac amyloidosis by routine laboratory parameters: a proof-of-concept study. J Clin Med. (2020) 9(5):1334. doi: 10.3390/jcm9051334
23. Bleijendaal H, Ramos LA, Lopes RR, Verstraelen TE, Baalman SWE, Oudkerk Pool MD, et al. Computer versus cardiologist: is a machine learning algorithm able to outperform an expert in diagnosing phospholamban (pln) P.Arg14del mutation on ecg? Heart Rhythm. (2020) 18(1):79–87. doi: 10.1016/j.hrthm.2020.08.021
24. Cikes M, Sanchez-Martinez S, Claggett B, Duchateau N, Piella G, Butakoff C, et al. Machine learning-based phenogrouping in heart failure to identify responders to cardiac resynchronization therapy. Eur J Heart Fail. (2019) 21(1):74–85. doi: 10.1002/ejhf.1333
25. Kay FU, Abbara S, Joshi PH, Garg S, Khera A, Peshock RM. Identification of high-risk left ventricular hypertrophy on calcium scoring cardiac computed tomography scans: validation in the dhs. Circ Cardiovasc Imaging. (2020) 13:e009678. doi: 10.1161/CIRCIMAGING.119.009678
26. Casebeer A, Horter L, Hayden J, Simmons J, Evers T. Phenotypic clustering of heart failure with preserved ejection fraction reveals different rates of hospitalization. J Cardiovasc Med. (2020) 15. doi: 10.2459/JCM.0000000000001116
27. Hedman AK, Hage C, Sharma A, Brosnan MJ, Buckbinder L, Gan LM, et al. Identification of novel pheno-groups in heart failure with preserved ejection fraction using machine learning. Heart. (2019):315481. doi: 10.1136/heartjnl-2019-315481
28. Lyon A, Ariga R, Minchole A, Mahmod M, Ormondroyd E, Laguna P, et al. Distinct ECG phenotypes identified in hypertrophic cardiomyopathy using machine learning associate with arrhythmic risk markers. Front Physiol. (2018) 9:213. doi: 10.3389/fphys.2018.00213
29. Al-Mallah MH, Elshawi R, Ahmed AM, Qureshi WT, Brawner CA, Blaha MJ, et al. Using machine learning to define the association between cardiorespiratory fitness and all-cause mortality (from the henry ford exercise testing project). Am J Cardiol. (2017) 120(11):2078–84. doi: 10.1016/j.amjcard.2017.08.029
30. Angraal S, Mortazavi BJ, Gupta A, Khera R, Ahmad T, Desai NR, et al. Machine learning prediction of mortality and hospitalization in heart failure with preserved ejection fraction. JACC Heart Fail. (2020) 8(1):12–21. doi: 10.1016/j.jchf.2019.06.013
31. Mpanya D, Celik T, Klug E, Ntsinjana H. Machine learning and statistical methods for predicting mortality in heart failure. Heart Fail Rev. (2020) 26:545–52. doi: 10.1007/s10741-020-10052-y
32. Awan SE, Bennamoun M, Sohel F, Sanfilippo FM, Chow BJ, Dwivedi G. Feature selection and transformation by machine learning reduce Variable numbers and improve prediction for heart failure readmission or death. PLoS One. (2018) 14(6):e0218760. doi: 10.1371/journal.pone.0218760
33. Awan SE, Bennamoun M, Sohel F, Sanfilippo FM, Dwivedi G. Machine learning-based prediction of heart failure readmission or death: implications of choosing the right model and the right metrics. ESC Heart Fail. (2019) 6(2):428–35. doi: 10.1002/ehf2.12419
34. Golas SB, Shibahara T, Agboola S, Otaki H, Sato J, Nakae T, et al. A machine learning model to predict the risk of 30-day readmissions in patients with heart failure: a retrospective analysis of electronic medical records data. BMC Med Inform Decis Mak. (2018) 18(1):44. doi: 10.1186/s12911-018-0620-z
35. Kagiyama N, Shrestha S, Cho JS, Khalil M, Singh Y, Challa A, et al. A low-cost texture-based pipeline for predicting myocardial tissue remodeling and fibrosis using cardiac ultrasound: texture-based myocardial tissue characterization using cardiac ultrasound. EBioMedicine. (2020) 54:102726. doi: 10.1016/j.ebiom.2020.102726
36. Sabovcik F, Cauwenberghs N, Kouznetsov D, Haddad F, Alonso-Betanzos A, Vens C, et al. Applying machine learning to detect early stages of cardiac remodelling and dysfunction. Eur Heart J Cardiovasc Imaging. (2020) 26:1208–17. doi: 10.1093/ehjci/jeaa135
37. Adler ED, Voors AA, Klein L, Macheret F, Braun OO, Urey MA, et al. Improving risk prediction in heart failure using machine learning. Eur J Heart Fail. (2020) 22(1):139–47. doi: 10.1002/ejhf.1628
38. Guo CY, Chan CH, Chou YC, Sung SH, Cheng HM. A statistical predictive model consistent within a 5-year follow-up period for patients with acute heart failure. J Chin Med Assoc. (2020) 83(11):1008–13. doi: 10.1097/JCMA.0000000000000403
39. Kilic A, Macickova J, Duan L, Movahedi F, Seese L, Zhang Y, et al. Machine learning approaches to analyzing adverse events following durable lvad implantation. Ann Thorac Surg. (2020) 19:770–7. doi: 10.1016/j.athoracsur.2020.09.040
40. Foster M. HF app to support self-care among community dwelling adults with HF: a feasibility study. Appl Nurs Res. (2018) 44:93–6. doi: 10.1016/j.apnr.2018.10.007
41. Werhahn SM, Dathe H, Rottmann T, Franke T, Vahdat D, Hasenfuss G, et al. Designing meaningful outcome parameters using mobile technology: a new Mobile application for telemonitoring of patients with heart failure. ESC Heart Fail. (2019) 6(3):516–25. doi: 10.1002/ehf2.12425
42. Guzman-Clark J, Yefimova M, Farmer MM, Wakefield BJ, Viernes B, Lee ML, et al. Home telehealth technologies for heart failure: an examination of adherence among veterans. J Gerontol Nurs. (2020) 46(7):26–34. doi: 10.3928/00989134-20200605-05
43. Ponikowski P, Voors AA, Anker SD, Bueno H, Cleland JGF, Coats AJS, et al. 2016 Esc guidelines for the diagnosis and treatment of acute and chronic heart failure: the task force for the diagnosis and treatment of acute and chronic heart failure of the European society of cardiology (ESC)developed with the special contribution of the heart failure association (HFA) of the esc. Eur Heart J. (2016) 37(27):2129–200. doi: 10.1093/eurheartj/ehw128
44. Barsheshet A, Kutyifa V, Vamvouris T, Moss AJ, Biton Y, Chen L, et al. Study of the wearable cardioverter defibrillator in advanced heart-failure patients (swift). J Cardiovasc Electrophysiol. (2017) 28(7):778–84. doi: 10.1111/jce.13229
45. Jimenez-Marrero S, Yun S, Cainzos-Achirica M, Enjuanes C, Garay A, Farre N, et al. Impact of telemedicine on the clinical outcomes and healthcare costs of patients with chronic heart failure and mid-range or preserved ejection fraction managed in a multidisciplinary chronic heart failure programme: a sub-analysis of the icor randomized trial. J Telemed Telecare. (2020) 26(1-2):64–72. doi: 10.1177/1357633X18796439
46. Burch AE, Scherr D, Rieth A, Griffin J, Bianco NR, Odeneg T, et al. Wearable cardioverter defibrillator-guided 6-min walk test performed at home is accurate and reliable: results of the trends study. J Cardiopulm Rehabil Prev. (2019) 40:E14–E17. doi: 10.1097/HCR.0000000000000441
47. Burkhoff D, Bailey G, Gimbel JR. Characterization of cardiac acoustic biomarkers in patients with heart failure. Ann Noninvasive Electrocardiol. (2020) 25(2):e12717. doi: 10.1111/anec.12717
48. Erath JW, Wanczura P, Wranicz J, Linke A, Rohrer U, Scherr D. Influence of decompensated heart failure on cardiac acoustic biomarkers: impact on early readmissions. ESC Heart Fail. (2020) 7:4198–205. doi: 10.1002/ehf2.13045
49. Galinier M, Roubille F, Berdague P, Brierre G, Cantie P, Dary P, et al. Telemonitoring versus standard care in heart failure: a randomised multicentre trial. Eur J Heart Fail. (2020) 22(6):985–94. doi: 10.1002/ejhf.1906
50. Kotooka N, Kitakaze M, Nagashima K, Asaka M, Kinugasa Y, Nochioka K, et al. The first multicenter, randomized, controlled trial of home telemonitoring for Japanese patients with heart failure: home telemonitoring study for patients with heart failure (homes-HF). Heart Vessels. (2018) 33(8):866–76. doi: 10.1007/s00380-018-1133-5
51. Joshi R, Gyllensten IC. Changes in daily measures of blood pressure and heart rate improve weight-based detection of heart failure deterioration in patients on telemonitoring. IEEE J Biomed Health Inform. (2019) 23(3):1041–8. doi: 10.1109/JBHI.2018.2856916
52. Kraai I, de Vries A, Vermeulen K, van Deursen V, van der Wal M, de Jong R, et al. The value of telemonitoring and ICT-guided disease management in heart failure: results from the in touch study. Int J Med Inform. (2016) 85(1):53–60. doi: 10.1016/j.ijmedinf.2015.10.001
53. Nunes-Ferreira A, Agostinho JR, Rigueira J, Aguiar-Ricardo I, Guimaraes T, Santos R, et al. Non-invasive telemonitoring improves outcomes in heart failure with reduced ejection fraction: a study in high-risk patients. ESC Heart Fail. (2020) 7:3996–4004. doi: 10.1002/ehf2.12999
54. Pedone C, Rossi FF, Cecere A, Costanzo L, Antonelli Incalzi R. Efficacy of a physician-led multiparametric telemonitoring system in very old adults with heart failure. J Am Geriatr Soc. (2015) 63(6):1175–80. doi: 10.1111/jgs.13432
55. Jayaram NM, Khariton Y, Krumholz HM, Chaudhry SI, Mattera J, Tang F, et al. Impact of telemonitoring on health status. Circ Cardiovasc Qual Outcomes. (2017) 10(12):e004148. doi: 10.1161/CIRCOUTCOMES.117.004148
56. Dang S, Karanam C, Gomez-Orozco C, Gomez-Marin O. Mobile phone intervention for heart failure in a minority urban county hospital population: usability and patient perspectives. Telemed J E Health. (2017) 23(7):544–54. doi: 10.1089/tmj.2016.0224
57. Eilat-Tsanani S, Golovner M, Marcus O, Dayan M, Sade Z, Iktelat A, et al. Evaluation of telehealth service for patients with congestive heart failure in the north of Israel. Eur J Cardiovasc Nurs. (2016) 15(3):e78–84. doi: 10.1177/1474515115602677
58. Ware P, Ross HJ, Cafazzo JA, Boodoo C, Munnery M, Seto E. Outcomes of a heart failure telemonitoring program implemented as the standard of care in an outpatient heart function clinic: pretest-posttest pragmatic study. J Med Internet Res. (2020) 22(2):e16538. doi: 10.2196/16538
59. Yanicelli LM, Goy CB, Gonzalez VDC, Palacios GN, Martinez EC, Herrera MC. Non-invasive home telemonitoring system for heart failure patients: a randomized clinical trial. J Telemed Telecare. (2020) 27(9):553–61. doi: 10.1177/1357633X19899261
60. Hale TM, Jethwani K, Kandola MS, Saldana F, Kvedar JC. A remote medication monitoring system for chronic heart failure patients to reduce readmissions: a two-arm randomized pilot study. J Med Internet Res. (2016) 18(5):e91. doi: 10.2196/jmir.5256
61. Gallagher BD, Moise N, Haerizadeh M, Ye S, Medina V, Kronish IM. Telemonitoring adherence to medications in heart failure patients (team-HF): a pilot randomized clinical trial. J Card Fail. (2017) 23(4):345–9. doi: 10.1016/j.cardfail.2016.11.001
62. Andrews AM, Russell CL. Impact of a systemchangetm intervention on medication adherence in older adults with heart failure: a feasibility study. J Gerontol Nurs. (2019) 45(4):15–9. doi: 10.3928/00989134-20190212-01
63. Kao DP, Lindenfeld J, Macaulay D, Birnbaum HG, Jarvis JL, Desai US, et al. Impact of a telehealth and care management program on all-cause mortality and healthcare utilization in patients with heart failure. Telemed J E Health. (2016) 22(1):2–11. doi: 10.1089/tmj.2015.0007
64. Koulaouzidis G, Barrett D, Mohee K, Clark AL. Telemonitoring in subjects with newly diagnosed heart failure with reduced ejection fraction: from clinical research to everyday practice. J Telemed Telecare. (2019) 25(3):167–71. doi: 10.1177/1357633X17751004
65. Breathett K, Maffett S, Foraker RE, Sturdivant R, Moon K, Hasan A, et al. Pilot randomized controlled trial to reduce readmission for heart failure using novel tablet and nurse practitioner education. Am J Med. (2018) 131(8):974–8. doi: 10.1016/j.amjmed.2018.02.017
66. Gastelurrutia P, Cuba-Gyllensten I, Lupon J, Zamora E, Llibre C, Caballero A, et al. Wearable vest for pulmonary congestion tracking and prognosis in heart failure: a pilot study. Int J Cardiol. (2016) 215:77–9. doi: 10.1016/j.ijcard.2016.04.024
67. Gingele AJ, Brunner-la Rocca H, Ramaekers B, Gorgels A, De Weerd G, Kragten J, et al. Telemonitoring in patients with heart failure: is there a long-term effect? J Telemed Telecare. (2019) 25(3):158–66. doi: 10.1177/1357633X17747641
68. Jaana M, Sherrard H, Pare G. A prospective evaluation of telemonitoring use by seniors with chronic heart failure: adoption, self-care, and empowerment. Health Informatics J. (2019) 25(4):1800–14. doi: 10.1177/1460458218799458
69. Lind L, Carlgren G, Karlsson D. Old-and with severe heart failure: telemonitoring by using digital pen technology in specialized homecare: system description, implementation, and early results. Comput Inform Nurs. (2016) 34(8):360–8. doi: 10.1097/CIN.0000000000000252
70. Mizukawa M, Moriyama M, Yamamoto H, Rahman M, Naka M, Kitagawa T, et al. Nurse-led collaborative management using telemonitoring improves quality of life and prevention of rehospitalization in patients with heart failure a pilot study. Int Heart J. (2019) 60(6):1293–302. doi: 10.1536/ihj.19-313
71. Olivari Z, Giacomelli S, Gubian L, Mancin S, Visentin E, Di Francesco V, et al. The effectiveness of remote monitoring of elderly patients after hospitalisation for heart failure: the renewing health European project. Int J Cardiol. (2018) 257:137–42. doi: 10.1016/j.ijcard.2017.10.099
72. Ong MK, Romano PS, Edgington S, Aronow HU, Auerbach AD, Black JT, et al. Effectiveness of remote patient monitoring after discharge of hospitalized patients with heart failure the better effectiveness after transition-heart failure (beat-HF) randomized clinical trial. JAMA Intern Med. (2016) 176(3):310–8. doi: 10.1001/jamainternmed.2015.7712
73. Yehle KS, Plake KS, Nguyen P, Smith D. Health-Related quality of life in heart failure patients with varying levels of health literacy receiving telemedicine and standardized education. Home Healthc now. (2016) 34(5):267–72. doi: 10.1097/NHH.0000000000000384
74. Bekelman DB, Plomondon ME, Carey EP, Sullivan MD, Nelson KM, Hattler B, et al. Primary results of the patient-centered disease management (PCDM) for heart failure study a randomized clinical trial. JAMA Intern Med. (2015) 175(5):725–32. doi: 10.1001/jamainternmed.2015.0315
75. Comin-Colet J, Enjuanes C, Verdu-Rotellar JM, Linas A, Ruiz-Rodriguez P, Gonzalez-Robledo G, et al. Impact on clinical events and healthcare costs of adding telemedicine to multidisciplinary disease management programmes for heart failure: results of a randomized controlled trial. J Telemed Telecare. (2016) 22(5):282–95. doi: 10.1177/1357633X15600583
76. Evangelista LS, Ghasemzadeh H, Lee JA, Fallahzadeh R, Sarrafzadeh M, Moser DK. Predicting adherence to use of remote health monitoring systems in a cohort of patients with chronic heart failure. Technol Health Care. (2017) 25(3):425–33. doi: 10.3233/THC-161279
77. Evans J, Papadopoulos A, Silvers CT, Charness N, Boot WR, Schlachta-Fairchild L, et al. Remote health monitoring for older adults and those with heart failure: adherence and system usability. Telemed J E Health. (2016) 22(6):480–8. doi: 10.1089/tmj.2015.0140
78. Gardner CL, Flanagan MC, Franklin C, John-Swayers C, Walsh-Pouch S, Bryant FJ, et al. Electronic physiologic and subjective data acquisition in home-dwelling heart failure patients: an assessment of patient use and perception of usability. Int J Med Inform. (2016) 93:42–8. doi: 10.1016/j.ijmedinf.2016.06.001
79. Haynes SC, Tancredi DJ, Tong K, Hoch JS, Ong MK, Ganiats TG, et al. Association of adherence to weight telemonitoring with health care use and death: a secondary analysis of a randomized clinical trial. JAMA Netw Open. (2020) 3(7):e2010174. doi: 10.1001/jamanetworkopen.2020.10174
80. Koehler F, Koehler K, Deckwart O, Prescher S, Wegscheider K, Kirwan BA, et al. Efficacy of telemedical interventional management in patients with heart failure (tim-HF2): a randomised, controlled, parallel-group, unmasked trial. Lancet. (2018) 392(10152):1047–57. doi: 10.1016/S0140-6736-2818-2931880-4
81. Koehler F, Koehler K, Prescher S, Kirwan BA, Wegscheider K, Vettorazzi E, et al. Mortality and morbidity 1 year after stopping a remote patient management intervention: extended follow-up results from the telemedical interventional management in patients with heart failure ii (tim-HF2) randomised trial. Lancet Digit Health. (2020) 2(1):e16–24. doi: 10.1016/S2589-7500-2819-2930195-5
82. Koehler J, Stengel A, Hofmann T, Wegscheider K, Koehler K, Sehner S, et al. Telemonitoring in patients with chronic heart failure and moderate depressed symptoms: results of the telemedical interventional monitoring in heart failure (tim-HF) study. Eur J Heart Fail. (2020) 23:186–94. doi: 10.1002/ejhf.2025
83. Stegmann T, Koehler K, Wachter R, Moeller V, Zeynalova S, Koehler F, et al. Heart failure patients with atrial fibrillation benefit from remote patient management: insights from the tim-Hf2 trial. ESC Heart Fail. (2020) 7(5):2516–26. doi: 10.1002/ehf2.12819
84. Rahimi K, Velardo C, Triantafyllidis A, Conrad N, Shah SA, Chantler T, et al. A user-centred home monitoring and self-management system for patients with heart failure: a multicentre cohort study. Eur Heart J Qual Care Clin Outcomes. (2015) 1(2):66–71. doi: 10.1093/ehjqcco/qcv013
85. Akyar I, Dionne-Odom JN, Bakitas MA. Using patients and their caregivers feedback to develop enable CHF-PC: an early palliative care intervention for advanced heart failure. J Palliat Care. (2019) 34(2):103–10. doi: 10.1177/0825859718785231
86. Hwang R, Mandrusiak A, Morris NR, Peters R, Korczyk D, Bruning J, et al. Exploring patient experiences and perspectives of a heart failure telerehabilitation program: a mixed methods approach. Heart Lung. (2017) 46(4):320–7. doi: 10.1016/j.hrtlng.2017.03.004
87. Dadosky A, Overbeck H, Barbetta L, Bertke K, Corl M, Daly K, et al. Telemanagement of heart failure patients across the post-acute care continuum. Telemed J E Health. (2018) 24(5):360–6. doi: 10.1089/tmj.2017.0058
88. Idris S, Degheim G, Ghalayini W, Larsen TR, Nejad D, David S. Failure: a pilot study of integrated telemonitoring and virtual provider appointments. Rev Cardiovasc Med. (2015) 16(2):156–62. doi: 10.3909/ricm0760
89. Jenneve A, Lorenzo-Villalba N, Courdier G, Talha S, Severac F, Zulfiqar AA, et al. Benefit of ambulatory management of patients with chronic heart failure by protocolized follow-up therapeutic education and remote monitoring solution: an original study in 159 patients. J Clin Med. (2020) 9(10):1–14. doi: 10.3390/jcm9103106
90. Veenstra W, op den Buijs J, Pauws S, Westerterp M, Nagelsmit M. Clinical effects of an optimised care program with telehealth in heart failure patients in a community hospital in the Netherlands. Neth Heart J. (2015) 23(6):334–40. doi: 10.1007/s12471-015-0692-7
91. Hagglund E, Lynga P, Frie F, Ullman B, Persson H, Melin M, et al. Patient-centred home-based management of heart failure: findings from a randomised clinical trial evaluating a tablet computer for self-care, quality of life and effects on knowledge. Scand Cardiovasc J. (2015) 49(4):193–9. doi: 10.3109/14017431.2015.1035319
92. Lycholip E, Aamodt IT, Lie I, Simbelyte T, Puronaite R, Hillege H, et al. The dynamics of self-care in the course of heart failure management: data from the in touch study. Patient Prefer Adherence. (2018) 12:1113–22. doi: 10.2147/PPA.S162219
93. Melin M, Hagglund E, Ullman B, Persson H, Hagerman I. Effects of a tablet computer on self-care, quality of life, and knowledge: a randomized clinical trial. J Cardiovasc Nurs. (2018) 33(4):336–43. doi: 10.1097/JCN.0000000000000462
94. Hovland-Tanneryd A, Melin M, Hagglund E, Hagerman I, Persson HE. From randomised controlled trial to real world implementation of a novel home-based heart failure tool: pooled and comparative analyses of two clinical controlled trials. Open Heart. (2019) 6(1):e000954. doi: 10.1136/openhrt-2018-000954
95. Riley WT, Keberlein P, Sorenson G, Mohler S, Tye B, Ramirez AS, et al. Program evaluation of remote heart failure monitoring: healthcare utilization analysis in a rural regional medical center. Telemed J E Health. (2015) 21(3):157–62. doi: 10.1089/tmj.2014.0093
96. Srivastava A, Do JM, Sales VL, Ly S, Joseph J. Impact of patient-centred home telehealth programme on outcomes in heart failure. J Telemed Telecare. (2019) 25(7):425–30. doi: 10.1177/1357633X18775852
97. Bavishi A, Bruce M, Ning H, Freaney PM, Glynn P, Ahmad FS, et al. Predictive accuracy of heart failure-specific risk equations in an electronic health record-based cohort. Circ Heart Fail. (2020) 13(11):e007462. doi: 10.1161/CIRCHEARTFAILURE.120.007462
98. Hwang R, Bruning J, Morris NR, Mandrusiak A, Russell T. Home-based telerehabilitation is not Inferior to a centre-based program in patients with chronic heart failure: a randomised trial. J Physiother. (2017) 63(2):101–7. doi: 10.1016/j.jphys.2017.02.017
99. Jaana M, Sherrard H. Rural-urban comparison of telehome monitoring for patients with chronic heart failure. Telemed J E Health. (2019) 25(2):101–8. doi: 10.1089/tmj.2017.0303
100. Cajita MI, Hodgson NA, Budhathoki C, Han HR. Intention to use mhealth in older adults with heart failure. J Cardiovasc Nurs. (2017) 32(6):E1–7. doi: 10.1097/JCN.0000000000000401
101. Evangelista LS, Lee JA, Moore AA, Motie M, Ghasemzadeh H, Sarrafzadeh M, et al. Examining the effects of remote monitoring systems on activation, self-care, and quality of life in older patients with chronic heart failure. J Cardiovasc Nurs. (2015) 30(1):51–7. doi: 10.1097/JCN.0000000000000110
102. Frederix I, Vanderlinden L, Verboven AS, Welten M, Wouters D, De Keulenaer G, et al. Long-term impact of a six-month telemedical care programme on mortality, heart failure readmissions and healthcare costs in patients with chronic heart failure. J Telemed Telecare. (2019) 25(5):286–93. doi: 10.1177/1357633X18774632
103. Guo X, Gu X, Jiang J, Li H, Duan R, Zhang Y, et al. A hospital-community-family-based telehealth program for patients with chronic heart failure: single-arm, prospective feasibility study. JMIR Mhealth Uhealth. (2019) 7(12):e13229. doi: 10.2196/13229
104. Haynes SC, Tancredi DJ, Tong K, Hoch JS, Ong MK, Ganiats TG, et al. The effect of rehospitalization and emergency department visits on subsequent adherence to weight telemonitoring. J Cardiovasc Nurs. (2020) 36(5):482–8. doi: 10.1097/JCN.0000000000000689
105. Kang Y, McHugh MD, Chittams J, Bowles KH. Risk factors for all-cause rehospitalization among medicare recipients with heart failure receiving telehomecare. Telemed J E Health. (2017) 23(4):305–12. doi: 10.1089/tmj.2016.0048
106. Lloyd T, Buck H, Foy A, Black S, Pinter A, Pogash R, et al. The penn state heart assistant: a pilot study of a web-based intervention to improve self-care of heart failure patients. Health Informatics J. (2019) 25(2):292–303. doi: 10.1177/1460458217704247
107. Lundgren JG, Dahlstrom O, Andersson G, Jaarsma T, Karner Kohler A, Johansson P. The effect of guided web-based cognitive behavioral therapy on patients with depressive symptoms and heart failure: a pilot randomized controlled trial. J Med Internet Res. (2016) 18(8):e194. doi: 10.2196/jmir.5556
108. Martin S, Anderson B, Vincenzo JL, Zai SY. A retrospective comparison of home telehealth and nursing care with or without rehabilitation therapy on rehospitalization rates of individuals with heart failure. J Cardiopulm Rehabil Prev. (2017) 37(3):207–13. doi: 10.1097/HCR.0000000000000221
109. Messina W. Decreasing congestive heart failure readmission rates within 30 days at the Tampa va. Nurs Adm Q. (2016) 40(2):146–52. doi: 10.1097/NAQ.0000000000000154
110. Mo Y, Wang H, Huang G, Chu M. Effectiveness of nurse-led program on mental health status and quality of life in patients with chronic heart failure. Medicine. (2020) 99(33):e21746. doi: 10.1097/MD.0000000000021746
111. Nguyen L, Keshavjee K, Archer N, Patterson C, Gwadry-Sridhar F, Demers C. Barriers to technology use among older heart failure individuals in managing their symptoms after hospital discharge. Int J Med Inform. (2017) 105:136–42. doi: 10.1016/j.ijmedinf.2017.06.001
112. Pekmezaris R, Nouryan CN, Schwartz R, Castillo S, Makaryus AN, Ahern D, et al. A randomized controlled trial comparing telehealth self-management to standard outpatient management in underserved black and hispanic patients living with heart failure. Telemed J E Health. (2019) 25(10):917–25. doi: 10.1089/tmj.2018.0219
113. Peng X, Su Y, Hu Z, Sun X, Li X, Dolansky MA, et al. Home-based telehealth exercise training program in Chinese patients with heart failure a randomized controlled trial. Medicine. (2018) 97(35):e12069. doi: 10.1097/MD.0000000000012069
114. Pennucci F, De Rosis S, Passino C. Piloting a web-based systematic collection and reporting of patient-reported outcome measures and patient-reported experience measures in chronic heart failure. BMJ Open. (2020) 10(10):e037754. doi: 10.1136/bmjopen-2020-037754
115. Piette JD, Striplin D, Marinec N, Chen J, Aikens JE. A randomized trial of mobile health support for heart failure patients and their informal caregivers. Med Care. (2015) 53(8):692–9. doi: 10.1097/MLR.0000000000000378
116. Piotrowicz E, Pencina MJ, Opolski G, Zareba W, Banach M, Kowalik I, et al. Effects of a 9-week hybrid comprehensive telerehabilitation program on long-term outcomes in patients with heart failure: the telerehabilitation in heart failure patients (telereh-HF) randomized clinical trial. JAMA Cardiol. (2020) 5(3):300–8. doi: 10.1001/jamacardio.2019.5006
117. Rahimi K, Nazarzadeh M, Pinho-Gomes AC, Woodward M, Salimi-Khorshidi G, Ohkuma T, et al. Home monitoring with technology-supported management in chronic heart failure: a randomised trial. Heart. (2020) 106(20):1573–8. doi: 10.1136/heartjnl-2020-316773
118. Rosen D, McCall JD, Primack BA. Telehealth protocol to prevent readmission among high-risk patients with congestive heart failure. Am J Med. (2017) 130(11):1326–30. doi: 10.1016/j.amjmed.2017.07.007
119. Sohn A, Speier W, Lan E, Aoki K, Fonarow GC, Ong MK, et al. Integrating remote monitoring into heart failure patients’ care regimen: a pilot study. PLoS One. (2020) 15(11):e0242210. doi: 10.1371/journal.pone.0242210
120. Tarte VP, Amirehsani KA. Perceptions of mobile technology for heart failure education and self-management among middle-aged and older adults. J Gerontol Nurs. (2019) 45(11):30–8. doi: 10.3928/00989134-20191011-05
121. Tersalvi G, Vicenzi M, Kirsch K, Gunold H, Thiele H, Lombardi F, et al. Structured telephone support programs in chronic heart failure may be affected by a learning curve. J Cardiovasc Med. (2020) 21(3):231–7. doi: 10.2459/JCM.0000000000000934
122. Woo K, Dowding DW. Decision-making factors associated with telehealth adoption by patients with heart failure at home: a qualitative study. Comput Inform Nurs. (2020) 38(4):204–14. doi: 10.1097/CIN.0000000000000589
123. Nouryan CN, Morahan S, Pecinka K, Akerman M, Lesser M, Chaikin D, et al. Home telemonitoring of community-dwelling heart failure patients after home care discharge. Telemed J E Health. (2019) 25(6):447–54. doi: 10.1089/tmj.2018.0099
124. Portz JD, Vehovec A, Dolansky MA, Levin JB, Bull S, Boxer R. The development and acceptability of a Mobile application for tracking symptoms of heart failure among older adults. Telemed J E Health. (2018) 24(2):161–5. doi: 10.1089/tmj.2017.0036
125. Alosco ML, Spitznagel MB, Cohen R, Sweet LH, Hayes SM, Josephson R, et al. Decreases in daily physical activity predict acute decline in attention and executive function in heart failure. J Card Fail. (2015) 21(4):339–46. doi: 10.1016/j.cardfail.2014.12.010
126. Floegel TA, Allen KD, Buman MP. A pilot study examining activity monitor use in older adults with heart failure during and after hospitalization. Geriatr Nurs. (2019) 40(2):185–9. doi: 10.1016/j.gerinurse.2018.10.001
127. Snipelisky D, Kelly J, Levine JA, Koepp GA, Anstrom KJ, McNulty SE, et al. Accelerometer-measured daily activity in heart failure with preserved ejection fraction: clinical correlates and association with standard heart failure severity indices. Circ Heart Fail. (2017) 10(6):e003878. doi: 10.1161/CIRCHEARTFAILURE.117.003878
128. de Canniere H, Smeets CJP, Schoutteten M, Varon C, Hoof CV, Huffel SV, et al. Using biosensors and digital biomarkers to assess response to cardiac rehabilitation: observational study. J Med Internet Res. (2020) 22(5):e17326. doi: 10.2196/17326
129. Rosen D, Berrios-Thomas S, Engel RJ. Increasing self-knowledge: utilizing tele-coaching for patients with congestive heart failure. Soc Work Health Care. (2016) 55(9):711–9. doi: 10.1080/00981389.2016.1191581
130. Wagenaar KP, Broekhuizen BDL, Jaarsma T, Kok I, Mosterd A, Willems FF, et al. Effectiveness of the European society of cardiology/heart failure association website ‘heartfailurematters.org’ and an E-health adjusted care pathway in patients with stable heart failure: results of the ‘e-Vita HF’ randomized controlled trial. Eur J Heart Fail. (2019) 21(2):238–46. doi: 10.1002/ejhf.1354
131. Baik D, Reading M, Jia H, Grossman LV, Masterson Creber R. Measuring health status and symptom burden using a web-based mhealth application in patients with heart failure. Eur J Cardiovasc Nurs. (2019) 18(4):325–31. doi: 10.1177/1474515119825704
132. Poli A, Kelfve S, Klompstra L, Stromberg A, Jaarsma T, Motel-Klingebiel A. Prediction of (non)participation of older people in digital health research: exergame intervention study. J Med Internet Res. (2020) 22(6):e17884. doi: 10.2196/17884
133. Varon C, Alao M, Minter J, Stapleton M, Thomson S, Jaecques S, et al. Telehealth on heart failure: results of the recap project. J Telemed Telecare. (2015) 21(6):340–7. doi: 10.1177/1357633X15577310
134. Allen LA, Venechuk G, McIlvennan CK, Page Ii RL, Knoepke CE, Helmkamp LJ, et al. An electronically delivered, patient-activation tool for intensification of medications for chronic heart failure with reduced ejection fraction: the epic-HF trial. Circulation. (2020) 143(5):427–37. doi: 10.1161/circulationaha.120.051863
135. Ahmad T, Lund LH, Rao P, Ghosh R, Warier P, Vaccaro B, et al. Machine learning methods improve prognostication, identify clinically distinct phenotypes, and detect heterogeneity in response to therapy in a large cohort of heart failure patients. J Am Heart Assoc. (2018) 7(8):e008081. doi: 10.1161/JAHA.117.008081
136. Desai RJ, Wang SV, Vaduganathan M, Evers T, Schneeweiss S. Comparison of machine learning methods with traditional models for use of administrative claims with electronic medical records to predict heart failure outcomes. JAMA Netw Open. (2020) 3(1):e1918962. doi: 10.1001/jamanetworkopen.2019.18962
137. Feeny AK, Rickard J, Patel D, Toro S, Trulock KM, Park CJ, et al. Machine learning prediction of response to cardiac resynchronization therapy: improvement versus current guidelines. Circ Arrhythm Electrophysiol. (2019) 12(7):e007316. doi: 10.1161/CIRCEP.119.007316
138. Bellavia D, Iacovoni A, Agnese V, Falletta C, Coronnello C, Pasta S, et al. Usefulness of regional right ventricular and right atrial strain for prediction of early and late right ventricular failure following a left ventricular assist device implant: a machine learning approach. Int J Artif Organs. (2020) 43(5):297–314. doi: 10.1177/0391398819884941
139. Pugin D, Hofmeister J, Gasche Y, Vulliemoz S, Lovblad KO, Ville DV, et al. Resting-state brain activity for early prediction outcome in postanoxic patients in a coma with indeterminate clinical prognosis. Am J Neuroradiol. (2020) 41(6):1022–30. doi: 10.3174/ajnr.A6572
140. Bhattacharya M, Lu DY, Kudchadkar SM, Greenland GV, Lingamaneni P, Corona-Villalobos CP, et al. Identifying ventricular arrhythmias and their predictors by applying machine learning methods to electronic health records in patients with hypertrophic cardiomyopathy (HCM-Var-risk model). Am J Cardiol. (2019) 123(10):1681–9. doi: 10.1016/j.amjcard.2019.02.022
141. Blecker S, Katz SD, Horwitz LI, Kuperman G, Park H, Gold A, et al. Comparison of approaches for heart failure case identification from electronic health record data. JAMA Cardiol. (2016) 1(9):1014–20. doi: 10.1001/jamacardio.2016.3236
142. Ng K, Steinhubl SR, Defilippi C, Dey S, Stewart WF. Early detection of heart failure using electronic health records: practical implications for time before diagnosis, data diversity, data quantity, and data density. Circ Cardiovasc Qual Outcomes. (2016) 9(6):649–58. doi: 10.1161/CIRCOUTCOMES.116.002797
143. Nakajima K, Nakata T, Doi T, Tada H, Maruyama K. Machine learning-based risk model using 123I-metaiodobenzylguanidine to differentially predict modes of cardiac death in heart failure. J Nucl Cardiol. (2020) 29:190–201. doi: 10.1007/s12350-020-02173-6
144. Nanayakkara S, Fogarty S, Tremeer M, Ross K, Richards B, Bergmeir C, et al. Characterising risk of in-hospital mortality following cardiac arrest using machine learning: a retrospective international registry study. PLoS Med. (2018) 15(11):e1002709. doi: 10.1371/journal.pmed.1002709
145. Bratt A, Kim J, Pollie M, Beecy AN, Tehrani NH, Codella N, et al. Machine learning derived segmentation of phase velocity encoded cardiovascular magnetic resonance for fully automated aortic flow quantification. J Cardiovasc Magn Reson. (2019) 21(1):1. doi: 10.1186/s12968-018-0509-0
146. Inan OT, Baran Pouyan M, Javaid AQ, Dowling S, Etemadi M, Dorier A, et al. Novel wearable seismocardiography and machine learning algorithms can assess clinical Status of heart failure patients. Circulation Heart Failure. (2018) 11(1):e004313. doi: 10.1161/CIRCHEARTFAILURE.117.004313
147. Choi DJ, Park JJ, Ali T, Lee S. Artificial intelligence for the diagnosis of heart failure. NPJ Digit Med. (2020) 3(1):54. doi: 10.1038/s41746-020-0261-3
148. Jovic A, Brkic K, Krstacic G. Detection of congestive heart failure from short-term heart rate variability segments using hybrid feature selection approach. Biomed Signal Process Control. (2019) 53:101583. doi: 10.1016/j.bspc.2019.101583
149. Mortazavi BJ, Downing NS, Bucholz EM, Dharmarajan K, Manhapra A, Li SX, et al. Analysis of machine learning techniques for heart failure readmissions. Circ Cardiovasc Qual Outcomes. (2016) 9(6):629–40. doi: 10.1161/CIRCOUTCOMES.116.003039
150. Stehlik J, Schmalfuss C, Bozkurt B, Nativi-Nicolau J, Wohlfahrt P, Wegerich S, et al. Continuous wearable monitoring analytics predict heart failure hospitalization: the link-HF multicenter study. Circ Heart Fail. (2020) 13:e006513. doi: 10.1161/CIRCHEARTFAILURE.119.006513
151. Shah AJ, Isakadze N, Levantsevych O, Vest A, Clifford G, Nemati S. Detecting heart failure using wearables: a pilot study. Physiol Meas. (2020) 41(4):044001. doi: 10.1088/1361-6579/ab7f93
152. Bose E, Radhakrishnan K. Using unsupervised machine learning to identify subgroups among home health patients with heart failure using telehealth. Comput Inform Nurs. (2018) 36(5):242–8. doi: 10.1097/CIN.0000000000000423
153. Au-Yeung WTM, Reinhall PG, Bardy GH, Brunton SL. Development and validation of warning system of ventricular tachyarrhythmia in patients with heart failure with heart rate variability data. PLoS One. (2018) 13(11):e0207215. doi: 10.1371/journal.pone.0207215
154. Ding W, Abdel-Basset M, Eldrandaly KA, Abdel-Fatah L, de Albuquerque VHC. Smart supervision of cardiomyopathy based on fuzzy Harris Hawks optimizer and wearable sensing data optimization: a new model. IEEE Trans Cybern. (2020) 24:4944–58. doi: 10.1109/TCYB.2020.3000440
155. Schloglhofer T, Horvat J, Moscato F, Hartner Z, Necid G, Schwingenschlogl H, et al. A standardized telephone intervention algorithm improves the survival of ventricular assist device outpatients. Artif Organs. (2018) 42(10):961–9. doi: 10.1111/aor.13155
156. Cajita MI, Hodgson NA, Lam KW, Yoo S, Han HR. Facilitators of and barriers to mhealth adoption in older adults with heart failure. Comput Inform Nurs. (2018) 36(8):376–82. doi: 10.1097/CIN.0000000000000442
157. Kerr B, Pharithi RB, Barrett M, Halley C, Gallagher J, Ledwidge M, et al. Changing to remote management of a community heart failure population during COVID-19 - clinician and patient perspectives’. Int J Cardiol Heart Vasc. (2020) 31:100665. doi: 10.1016/j.ijcha.2020.100665
158. Liljeroos M, Thylen I, Stromberg A. Patients’ and nurses’ experiences and perceptions of remote monitoring of implantable cardiac defibrillators in heart failure: cross-sectional, descriptive, mixed methods study. J Med Internet Res. (2020) 22(9):e19550. doi: 10.2196/19550
159. Meeker D, Goldberg J, Kim KK, Peneva D, Campos HO, Maclean R, et al. Patient commitment to health (pact-health) in the heart failure population: a focus group study of an active communication framework for patient-centered health behavior change. J Med Internet Res. (2019) 21(8):e12483. doi: 10.2196/12483
160. Zippel-Schultz B, Palant A, Eurlings C, Ski CF, Hill L, Thompson DR, et al. Determinants of acceptance of patients with heart failure and their informal caregivers regarding an interactive decision-making system: a qualitative study. BMJ Open. (2021) 11(6):e046160. doi: 10.1136/bmjopen-2020-046160
161. Gorodeski EZ, Goyal P, Cox ZL, Thibodeau JT, Reay RE, Rasmusson K, et al. Virtual visits for care of patients with heart failure in the era of COVID-19: a statement from the heart failure society of America. J Card Fail. (2020) 26(6):448–56. doi: 10.1016/j.cardfail.2020.04.008
162. Afonso Nogueira M, Ferreira F, Raposo AF, Monica L, Simoes Dias S, Vasconcellos R, et al. Impact of telemedicine on the management of heart failure patients during coronavirus disease 2019 pandemic. ESC Heart Fail. (2021) 8(2):1150–5. doi: 10.1002/ehf2.13157
163. Sayer G, Horn EM, Farr MA, Axsom K, Kleet A, Gjerde C, et al. Transition of a large tertiary heart failure program in response to the COVID-19 pandemic: changes that will endure. Circ Heart Fail. (2020) 13(9):e007516. doi: 10.1161/CIRCHEARTFAILURE.120.007516
164. Alter DA, Ko DT, Tu JV, Stukel TA, Lee DS, Laupacis A, et al. The average lifespan of patients discharged from hospital with heart failure. J Gen Intern Med. (2012) 27(9):1171–9. doi: 10.1007/s11606-012-2072-y
165. Woo K, Shang J, Dowding DW. Patient factors associated with the initiation of telehealth services among heart failure patients at home. Home Health Care Serv Q. (2018) 37(4):277–93. doi: 10.1080/01621424.2018.1523767
Keywords: heart failure, virtual healthcare, telemedicine, eHealth, patient empowerment, self-care
Citation: Lee KCS, Breznen B, Ukhova A, Martin SS and Koehler F (2023) Virtual healthcare solutions in heart failure: a literature review. Front. Cardiovasc. Med. 10:1231000. doi: 10.3389/fcvm.2023.1231000
Received: 29 May 2023; Accepted: 29 August 2023;
Published: 7 September 2023.
Edited by:
Tomasz Zieliński, National Institute of Cardiology, PolandReviewed by:
Roderick Willem Treskes, Leiden University Medical Center (LUMC), Netherlands© 2023 Lee, Breznen, Ukhova, Martin and Koehler. This is an open-access article distributed under the terms of the Creative Commons Attribution License (CC BY). The use, distribution or reproduction in other forums is permitted, provided the original author(s) and the copyright owner(s) are credited and that the original publication in this journal is cited, in accordance with accepted academic practice. No use, distribution or reproduction is permitted which does not comply with these terms.
*Correspondence: Keni Cheng-Siang Lee a2VuaS5sZWVAc2Fub2ZpLmNvbQ==
Disclaimer: All claims expressed in this article are solely those of the authors and do not necessarily represent those of their affiliated organizations, or those of the publisher, the editors and the reviewers. Any product that may be evaluated in this article or claim that may be made by its manufacturer is not guaranteed or endorsed by the publisher.
Research integrity at Frontiers
Learn more about the work of our research integrity team to safeguard the quality of each article we publish.