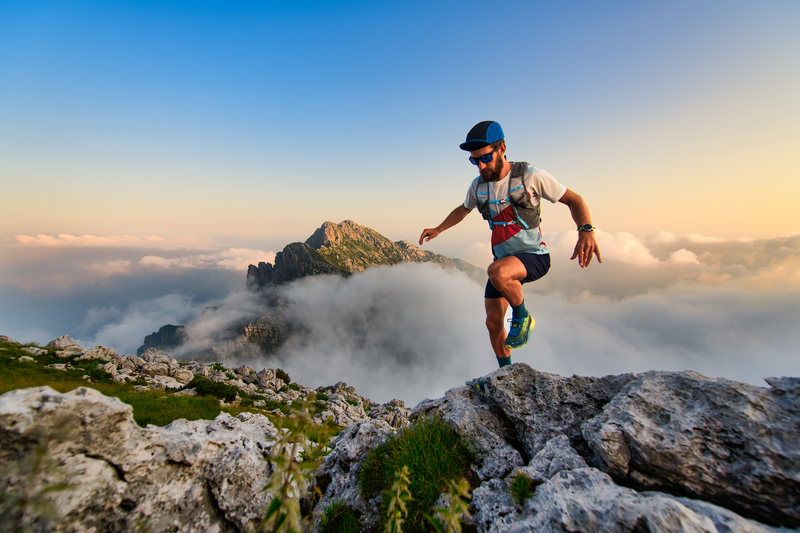
94% of researchers rate our articles as excellent or good
Learn more about the work of our research integrity team to safeguard the quality of each article we publish.
Find out more
ORIGINAL RESEARCH article
Front. Built Environ.
Sec. Coastal and Offshore Engineering
Volume 11 - 2025 | doi: 10.3389/fbuil.2025.1418492
The final, formatted version of the article will be published soon.
You have multiple emails registered with Frontiers:
Please enter your email address:
If you already have an account, please login
You don't have a Frontiers account ? You can register here
Pollutant emissions due to hurricane-induced petrochemical infrastructure disruptions pose a significant threat to the public health of fenceline communities and the surrounding environment. The objective of this study is to develop a framework for cascading consequence modeling of petrochemical processing infrastructure subjected to hurricane hazards. Overall the proposed framework leverages Bayesian networks for predictive modeling and potential updating of facility shutdown and excess emissions quantification due to hurricane-induced facility failures. The NHERI DesignSafe Cyberinfrastructure is leveraged to reuse prior hindcast storm datasets, develop and share a petrochemical infrastructure performance database, conduct statistical analyses for model development, and perform case study regional risk analyses. As input to the framework, predictive models for likelihood and expected duration of petrochemical facility idle and restart times and expected resulting excess emissions quantities are proposed. Such models are presently lacking in the literature yet vital for risk and resilience modeling of the cascading consequences of petrochemical complex shutdowns ranging from resilience analyses of regional petrochemical processing infrastructure to potential health effects on fenceline communities tied to shutdown and restart activities. A database of empirical petrochemical facility characteristics, downtime, and hurricane hazards data is developed, and statistical analyses are conducted to investigate the relationship between facility and storm features and shutdown duration.The proposed method for expected shutdown modeling with the highest predictive accuracy is determined to be one comprised of a logistic regression binary classification component related to facility shutdown potential and a gamma distribution generalized linear model component related to idle time duration determination. To illustrate the utility of the proposed framework, a case study is performed investigating the potential mitigative impact of the proposed Galveston Bay Park Plan on Houston Ship Channel regional petrochemical processing resilience and cascading air pollutant emissions risk. Such analyses expose community and regional impacts of facility failures and can support resilience improvement decisions.
Keywords: Bayesian (Belief) networks, Petrochemical processing, Hurricane, resilience, risk
Received: 16 Apr 2024; Accepted: 14 Feb 2025.
Copyright: © 2025 Capshaw and Padgett. This is an open-access article distributed under the terms of the Creative Commons Attribution License (CC BY). The use, distribution or reproduction in other forums is permitted, provided the original author(s) or licensor are credited and that the original publication in this journal is cited, in accordance with accepted academic practice. No use, distribution or reproduction is permitted which does not comply with these terms.
* Correspondence:
Jamie Ellen Padgett, Rice University, Houston, United States
Disclaimer: All claims expressed in this article are solely those of the authors and do not necessarily represent those of their affiliated organizations, or those of the publisher, the editors and the reviewers. Any product that may be evaluated in this article or claim that may be made by its manufacturer is not guaranteed or endorsed by the publisher.
Research integrity at Frontiers
Learn more about the work of our research integrity team to safeguard the quality of each article we publish.