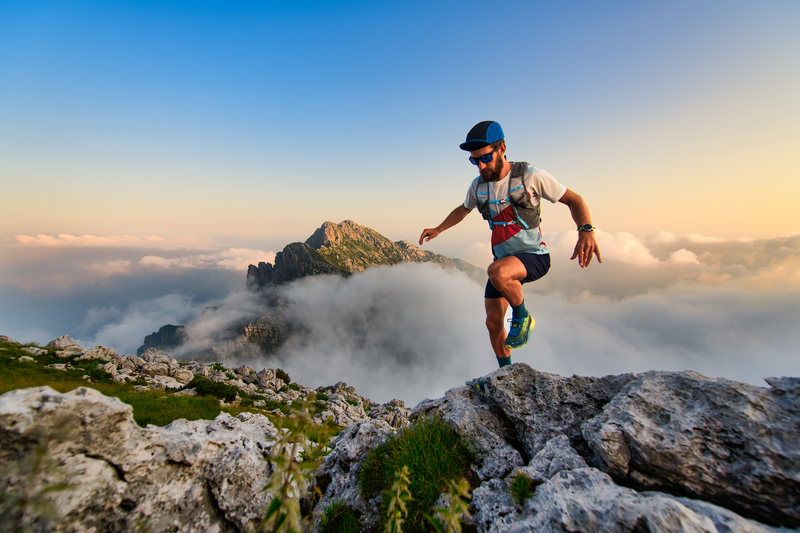
95% of researchers rate our articles as excellent or good
Learn more about the work of our research integrity team to safeguard the quality of each article we publish.
Find out more
ORIGINAL RESEARCH article
Front. Built Environ. , 27 November 2024
Sec. Construction Management
Volume 10 - 2024 | https://doi.org/10.3389/fbuil.2024.1478239
This article is part of the Research Topic Doctoral Research in Construction Management View all 10 articles
In all country construction projects include an enormous number of financial transactions and it’s necessary that the contracts between stakeholders should be more advanced in technology. The advance of Blockchain technology has been incontrovertible in recent years and this allowed the contracts to be programmed on Blockchain as smart contracts. The main goal of this paper is to identify and prioritize critical success factors of Smart Contracts in the construction industry. For identifying factors, we used library study and for prioritizing them, field study. To collect data, we designed a DANP questionnaire, from a panel senior managers and engineering experts. The results show that technology maturity is the most important factor in Smart Contract success; security of contracts, support from the engineering community, stakeholders’ consideration, and competition in the building industry were other important factors which took their next levels.
The significance of having a contract with project execution in the dynamic construction sector is obvious (Drew and Skitmore, 1997). The foundation of agreements between investors and employers are financial contracts, which specify the distribution of risks and rewards, the flow of funds, and the allocation of resources. However, there is a great deal of risk and uncertainty associated with construction projects due to their extended durations, technical complexity, and reliance on several parties. Market fluctuations, changes in regulations, unanticipated site circumstances, and project schedule slips are all potential causes of such risks. Because of this, these risks greatly affect the stability and profitability of the projects. The challenges and unknowns in the construction business, as pointed out by Nanayakkara et al. (2021), increase the costs for construction while also raising the risks associated with projects. Because of this, effective contracting is essential in the industry. Also, in comparing with digital payment method, smart contracts increased data transparency (Perera et al., 2020) and quality of cost and also improvement in information accuracy by 200% (Hamledari and Fischer, 2021). Moreover, Blockchain smart contracts raised trust in data used in construction management applications (Shojaei et al., 2020).
Due to rapid technological advancements, Sharma et al. (2023) explain that an increasing number of contracts will be substituted by technology-dependent solutions. With the promise of performing contract enforcement, these smarter contracts are expected to solve some of the construction industry’s long-standing management problems. They provide an easier, more open, and more effective way to manage the intricate web of funds and contracts that construction projects involve. Thus, using an operations research approach, this research aims to explore contracts in the construction industry and analyze the essential success aspects. This study tries to identify the most important challenges of smart contracts in construction industry and find the success aspect of these implements in the construction industry and rank them in order of importance. This will help reduce uncertainties, mitigate risks, and produce better project outcomes. Researchers, policymakers, and practitioners in the construction sector can use the study’s findings to inform smart contract adoption and optimization efforts, while also adding to the existing body of knowledge in construction project management.
The advance of Block chain technology in recent years has enabled uncentralized programming and revived the concept of Smart contracts presented by Nick Szabo in 1997. The ideas of Smart Contracts were not noticed in 1997, but with the advancement of Blockchain’s technology, it is now followed by organizations and governments because of their high security, low expenses, such as third person’s commission, and the risk of late payments or non-payments (Wang et al., 2018). The original ideas behind this technology appeared in the late 1980s and early 1990s. In 1977 a paper was published by Diffie, which spoke of the concept of a coded ledger (Diffie, 1976) in 1989, Leslie Lamport developed a protocol; and in 1990, he presented a paper to ACM transactions in computer systems. The article, published in 1998 (Lamport, 1998), describes a popular model of getting a conclusion in a network of computers, where the computers or the network itself may be unreliable. In 1991, a chain of signed data was used as a digital ledger of signed documents, which would easily show that none of the signed documents in this set had changed. In 1997, Adam Back introduced another concept called “Hash-Cash”, which offered a way to control spam emails (Back, 1997), and in 2001 he published a paper entitled “Freedom 2.1 security issues and analysis” to consider the issue more thoroughly (Back et al., 2001). These concepts were synthesized and implemented in 2008 with the digital cash, published in the article “Bitcoin: A peer-to-peer electronic cash system” by Satoshi Nakamoto, and then in 2009 with the establishment of the Blockchain network, Bitcoin digital currency took place (Nakamoto, 2008). In 2014 a discussion started about how the “Blockchain Model” would make negotiations possible; it was also expected that by 2025 the annual income of companies from the Blockchain would amount to 19.9 billion dollars (Tabatabaei et al., 2023). (Figure 1) presents the historical background of Blockchain chronologically.
The original purpose of using Blockchain was to make direct transactions between users without the need for a trustworthy third party. With the use of the Blockchain, a mechanism was created to ensure that only authentic transactions and blocks were added to the Blockchain. Bitcoin was the first use of blockchain. Many e-currency projects existed before Bitcoin, but none of them reached wide use. The use of Blockchain made Bitcoin integrated in a distribution way, so that no user controls the e-change cash and there is no breaking point, which increased its usefulness. At Bitcoin, the Blockchain allows users to be unknown, but their account numbers are not. Moreover, all transactions are publicly observed. This trust was usually broken off by trusty intermediaries on both sides before the use of the Blockchain technology. Without trusted agents, the trust needed in a network of Blockchain is activated by the four key processes of Blockchain technologies. 1) Ledgers -This technology uses only supplementary accounting for a full transaction history. All transactions made in the Blockchain are recorded in Ledgers. 2) Security - Blockchains are cryptographically secure, ensuring that the data is not manipulated, and they are verifiable. 3) Sharing - the whole ledger is shared among several participants, which provides transparency among the participants in the network. 4)Distribution–Blockchain can be distributed. This provides for the possibility of adjusting the number of nodes in a network of cables to make it more flexible to the attacks. As the number of nodes increases, the ability of a bad factor to influence the common protocol used in the Blockchain will be reduced. For the Blockchain systems, where anyone is allowed to commence a subscription and participate (which are called Blockchain without an amount) These abilities present a superficial level of trust between the two sides without being aware of each other. This trust could enable individuals and organizations to deal directly, which might result in faster and cheaper transactions. For a highly controlled Blockchain (called a legitimate Blockchain), where there may be some confidence among the users, these abilities help to strengthen that confidence (Ølnes et al., 2017). The Blockchain is a regular list of blocks, where each block is identified by its own cryptograph. Hash is a way of using a data coder, calculating a relatively unique output for almost any size of input (for example, a file, a text, or a screen). This system allows individuals to receive the input data, to assemble them, and to get the same result (which proves that there is no change in the data). Even the slightest change in the entry (for example, a space in a line) will result in a completely different swallowing. Each block refers to the last one which makes a chain of blocks. Each block contains a series of transactions. When a block is created and added to the Blockchain, the transactions in that block cannot be changed or reversed (Alharby and Van Moorsel, 2017). This is to ensure the unity of transactions, and to avoid the problem of double expenditure. A Blockchain user sends information to the network. Data may include the address of the sender (or another related identity), the general key of the sender, digital signature, entry, and existence of the transaction (Hartel and van Staalduinen, 2019). Entries are usually a list of digital assets to be transferred, As the entry of a transaction is a reference to past events, digital assets do not change. With cryptographic values, that means that value cannot be added or deleted to existing digital assets. Instead, a single digital asset can be divided into several new digital assets (each with less value) or combined into a few less (with more valued) new digital assets. Outputs are usually accounts which receive digital assets. Every output specifies the number of digital assets to be transferred to the new owners, the identity of the new owners, and a series of conditions to be met with for the use of that value.
The Blockchain technology uses asymmetrical key (also known as a general cryptography key) to enable the encryption. The symmetrical key encryption uses a pair of keys: a general key and a private key, mathematically related. The public key becomes public without lowering the security of this process, but the private key must remain hidden, if data are to preserve their cryptic protection. Users can secure a password with a public key, and then a private key, establishing a trust between users who do not know each other or trust each other, by providing a mechanism to ensure the unbrokenness and credit of transactions, yet allowing public transactions to remain. To do this, transactions are digitally signed. This means that a private key is used to unlock a transaction so that anyone with a public key can lock it. As the public key is freely available, the cryptography of a transaction with a private key proves that the signature holder has access to a private key (Wang et al., 2018). Users must manage and store their private keys, often using the software for their security retrieval instead of manual recording. This software is often known as a wallet, which can store private keys, public keys, and relevant addresses. It may also do other things, such as computing the total number of digital assets the user may have. If a user loses a private key, then any digital assets connected to that key will be lost, because it is mathematically impossible to rebuild the same private key. If a private key is stolen, the attacker will have full access to all digital assets under the control of that private key. The security of private keys is so important that many users use special security software to keep them locked away.
Smart contracts are a significant development in Blockchain. Smart contracts were first proposed for the purpose of a digital transaction protocol to fulfil the conditions of the agreement in 1990. Smart contracts are simply a set of rules which repeat and preserve the conditions of real-world contracts in the digital field. Agreements are essentially a legally binding agreement between two or more parties, and each party is under an obligation to fulfil its obligations. Moreover, this agreement must be enforced by law, often by a centralized legal institution (Taherdoost, 2023). Smart contracts are written in code, distributed through a network of blocks, and un centralized. Smart contracts allow transactions between unknown or unreliable parties without the need for a central reference. The most famous Blockchains tracts are Ethereum, Tron, and Binance (Wang et al., 2018). Ethereum is now the most popular platform for developing Smart contracts. Among the advantages of Smart contracts, there are a reduction of risk, a reduction of administrative and service costs, an improvement of the efficiency of business processes, a speeding up of business, a greater accuracy, a lower risk of carrying out, a few intermediaries and less costs (Jani, 2020). The construction industry is one of the most important in the world. It produces some of the most complex and massive things, such as buildings, bridges, dams, and tunnels. As an industry, building accounts for more than 10 percent of the world’s GDP, and uses about 7 percent of it (Abdallah Zaky and Nassar, 2021). Construction projects are based on contract arrangements, and contracts are the basis of their success. The contract makes it possible to carry out the work, to transfer payments, to track the key performance indicators, and to facilitate cooperation among the donors. Construction projects have been struggling because of the poor harmony between the expectations of customers, the terms of the contract, and the performance of the contractor. The emergence of advanced digital technology has the potential to benefit construction projects by using active Blockchain contracts, yet acceptance of Smart contracts in Construction projects is in its early age, and the factors that will influence it remain uncertain (Ameyaw et al., 2023).
In building projects there is a lack of control over data and data accessibility and also a major risk to cybersecurity of data (Shojaei and Naderi, 2024). Blockchain Technology for a Circular Built Environment. In A Circular Built Environment in the Digital Age (pp. 213-228). Cham: Springer International Publishing. Smart contracts have the potential to help solve a series of growing building issues, especially contract disputes and payments. There are contract differences in the construction industry; inconsistencies, errors, or misapplications of terms in contracts could cause difficulties in execution. The current spread of paper contracts makes it difficult to track changes and record their execution. With Smart contracts, irrevocability is guaranteed, while all changes are safely recorded and easy to track. Another growing problem is in paying claims. Punctual payments and a steady cash flow are vital to the success of a building project. Troubles such as failure to pay, delay, and an unfair payment, cause delay in building, extra expenses, lower performance, and disagreements. The recent advances in Smart contracts enabled the possibility of secure and precise verification (Ye et al., 2022). In the construction industry, the parties to the contract, the owner, the counsel, the principal contractors, the subsidiary contractors, and the suppliers and documents are very complex in their various ways. Moreover, the process of building is fluctuating and challenging throughout the various stages of construction, so that a Blockchain is a very good technique to overcome these challenges (Ibrahim et al., 2022). In this industry, shareholders in a project must examine their identity to access the Blockchain, or else they cannot access the data of the project. All transactions made in the Blockchain system are recorded in a chain on the network, the analysis of the project’s track and performance can be easily done with this advantage. Moreover, data such as working hours or interrupts could be computed, and payments could be automatically made upon them. Work and rest hours and salary of the workers may be controlled by law and used as a witness against disputes between employers and workers. Also, the transactions in the project require approval from the majority shareholders in the system. This would allow for greater cooperation among the beneficiaries, and at the same time would help to minimize possible disagreements. One of the most important features of the Blockchain is its cryptography. This is a one-way cryptography, which adds to the security and privacy of the system. The Blockchain locks all transactions in the system. So, a change or manipulation of the data in the chain is almost impossible. Each transaction is added in a line to this network in real time, and the network is continually expanding according to the progress of the project. Cost, time, and quality are the essential elements in the construction industry. The aim is to achieve the maximum quality at the least cost and time. The fact that the Blockchain makes the project trackable and transparent helps to increase the quality. The Blockchain technology provides an opportunity to wipe out intermediaries by Smart contracts, yet eliminate such matters as papers, bills, and documents, which therefore makes a positive effect on project costs and time (Altay and Motawa, 2020).
The differences in payment in the construction projects can be considerably reduced by using a smart contract. It is a desirable system by which time and expense are saved in automating specific aspects of the construction process. Preparation is one of the critical phases of a construction project in which several contracts are completed. As the buyers and the sellers may have never made any transactions together before, trust must be maintained between the two parties, or else confidence must be established between them by other parties such as banks, libraries, intermediaries, etc. Smart contracts are un centralized technology, therefore, third persons such as banks may be wiped out in a transaction process. Making application of a Smart contract in the preparation of a project allows the parties of a contract to convert the content of such clauses as amount and date of payment into codes. The amount to be paid, such as equipment, materials, and so on, is fixed in the Smart contract, Furthermore, the date of payment could be entered into the system. The transparency of a Smart contract allows the parties to the contract to be sure that the amount of the goods being paid is blocked in the system so that neither party has access to the money until the payment is made. The exporter can warn the importer, for the goods are ordered through A Smart contract. Moreover, the importer can let the exporter know that the orders are being delivered. using the smart execution facility of the agreement, as both parties approve the coded conditions, the amount of payment will automatically be deposited into the exporting entity’s digital account. Using Smart Contracts Technology in the preparation phase may have the following advantages. In consequence, the higher expenses of intermediaries and executive expenses arising from this process will be considerably reduced. Also, the high speed of approval and transaction, the reduction of secret payment, and the creation of a secure equity environment are advantages to the introduction of Smart contracts in the preparation of construction (Abdallah Zaky and Nassar, 2021). Smart contracts are not yet fully mainstream. There are few trained people who can fully apply for a smart contract in a building project. The fluctuations of crypto currencies could also be a danger to hinder the parties from using Smart contracts in comparison to traditional ways. To apply the system in industry, all parties involved must be aware of the advantages of Smart contracts to trust and accept it. Though Smart contracts minimize differences by their double logic in carrying out their articles, there may still be the potential for unforeseen differences. So, by applying to construction lawyers, possible differences must be identified, and new laws may be introduced to resolve them based on that (Ahmadisheykhsarmast and Sonmez, 2018).
In a study (Ahmadisheykhsarmast and Sonmez, 2018), found that a failure to pay and a refusal to pay are two major problems in the construction industry, which have already driven several parties to the brink of bankruptcy. Smart contracts provide solutions to the conflicts about payments which often occur in the preparation of a manufacturing advance. Reduced costs and time, greater trust between the parties, assurance of payment, a higher speed of performance, and the removal of intermediaries in the preparation of a business, are other advantages of using them in the construction industry Zou et al. (2019) interviewed 20 specialized developers to understand the main differences between the development of a smart contract and the development of a conventional software, and their effects, as well as the challenges in the implementation of various kinds of Smart contracts. Studies showed that developing a Smart contract was still in its early stages, and that there was no acceptable way of ensuring the code of a Smart contract; nor were there enough instruments to guarantee the code, and that the development platforms and time of performance, such as software languages and virtual machines, were still limited, and that online learning and social support were also limited Huang et al. (2019) conducted a study on the safety of Smart contracts, in which the challenges of security research for Smart contracts are summarized as a software engineering problem, and several research paths are proposed for improving the security of Smart contracts. The environment in which Smart contracts are enforced is unfocused and very difficult to repair and maintain, and therefore Smart contracts have many security issues of uncommon security assaults Ibrahim et al. (2022) created a model based on the results of their demonstration and observations, which provided conditions for a reduction of the authority of the contracting executive by replacing the centralized system and human manipulating the contract with an uncentralized automated system. First, a prototype was created using the Python programming language; then, a three-case study was used to show the capacity and creditability of the prototype, using various scenarios to show the capacity and creditability of the prototype; then, performance was evaluated by two main criteria: capacity for storage and time of delay Kirli et al. (2022) examined Smart contracts in energy systems. They stated that using a Smart contract in an energy project or platform would not make the project “Smart” by itself; that these contracts, properly combined with other techniques, were a powerful potential technology that could play an important role in enabling future multi-integrated and highly active energy systems (Ullah et al., 2020) presented a concept framework for accepting a Blockchain agreement for management of estate transactions in a smart city, discussing the layers involved in Blockchains and their un centralized structure of programmed, and offering a concept design for a Blockchain agreement (Kushwaha et al., 2022) conducted a systematic study of aerial analyses of Smart contracts based on Blockchains, in which 86 analysis tools were covered and 145 research articles from literature and other online sources from 2016 to 2021. The results of the study showed that aerial analyses of transactions are a central concern, and that various analysis tools have been developed for creating safe aerial transactions. The most vulnerable one identified by many static and dynamic analyses is “reentering”. (Balcerzak et al., 2022), examined the application of Blockchain and Smart contract in the non-central government system, and how using analytical decision models and scanners could rearrange structures of corporate governance, they concluded that smart governance systems based on Blockchains, using data accounting capabilities, distribution processes, sensitivity analysis and related intelligence, would require public participation (Sigalov et al., 2021), entitled Auto Pay and Contract Management in the Construction Industry, described the BIM contracts, the exchange of pivot data and the digital contract management process. The result is that taking the Blockchain as a social-technical system emphasizes the importance of social, organizational, technical factors, as well as legal policy, and that the integration of Smart contracts into legal construction contracts, given the absence of precedents and legal rules, has posed a challenge.
After conducting a thorough literature analysis and field study, being shown as (Table 1), we were able to identify 17 factors that were later classified into 4 groups according to their relationship and influence on the effectiveness of Smart Contracts. Using a literature review as a starting point, Table 1 compiled the literature-discussed success factors for smart contracts in the construction sector. The industry’s adoption and performance of Smart Contracts are affected by the interrelated elements that make up each group. Figure 2 provides a clear and organized overview of the findings by visually representing the classification and interplay of these elements.
The results are informed, relevant, and valuable for understanding the barriers to the adoption of Smart Contracts in the construction and contracting sectors. The next step is to establish a methodology for evaluating the success factors for smart contract implementation in the construction sector.
Multi-criteria decision method known as MCDM was considered an area for decision analyzing and decision making since 1960s following the development of operational research in World War II (Alias et al., 2008). This approach is used for colossal selections and the best selection will be the one which satisfies decision makers, also it has been used to be a solution for issues that have a limited number of alternatives (Ghaleb et al., 2020). In operations research, the multi-criteria decision method (MCDM) can consider several criteria at once, and help decision makers to assess the best responses, considering the specific features of limited situations available (Tzeng et al., 2002a).
The DANP approach was introduced as a combination of Analytical Network Process known as ANP and Decision-Making Trial Evaluation Laboratory (DEMATEL) which is appropriate for problems with dependent criteria to be solved. In ANP, adjustment is made by dividing each standard in a column over the total of the columns, so that each column has a precise unit. The effect of one cluster on another cluster may be different in rating; hence, the ANP theory that each cluster has the same weight in producing a weighted super matrix, is unreasonable. Consequently, DANP effective weights can correct this weakness and get results based on the basic concept of ANP of the Tc and Td matrix, using the DEMATEL technique. So, the DEMATEL technique of creating INRM is used to improve the traditional ANP normalization process (Chiu et al., 2013). DANP is a suitable method for the interaction and interdependence of the dimensions and norms which appear in the cases of real-world problems. Regarding the physiological characteristics of physical facts, this method could study the interdependence of variables and properties and create a relationship which would show them to a necessary system and evolutionary process.
There is a lack of experts in DANP district, and we must be able to turn the knowledge of experts from oblique knowledge into a visible knowledge and make a model of experts’ minds, therefore MCDM and DANP approaches are suitable for this purpose. In this study, we used the DANP method, a mixture of DEMATEL and ANP communications matrix.
We approached experts to collect data for this study. In MADM methods between 4 and 15 experts are used (Zhang and Zou, 2007; Hyun et al., 2008; Lam et al., 2008; Dalal et al., 2010; Zou and Li, 2010; Pan et al., 2012; Akadiri et al., 2013; Chou et al., 2013). The fewer the number of individuals, the stricter the criteria. Given that our estimate was that the number of individuals is low, we set strict conditions for the entry of experts which included having at least a master’s degree or a minimum of 10 years of work experience in civil engineering or construction industries and having worked with or been involved in smart contracts. We identified this through searching individuals’ resumes. Due to the stringent criteria, the number of participants in the study was low; however, we were confident that they were familiar with the concepts of smart contracts and blockchain technology and had experience working with them, making them suitable experts for this study. We used purposive sampling and were able to find 7 experts, whose characteristics you can see in Table 2. Moreover, it may be unhelpful to use DANP in a study with a large sample size because called experts are likely to provide random answers, which could significantly affect the consistency of the judgments (Cheng and Li, 2002). if the number of experts in a group is relatively small, expert competence should always be taken into consideration (Tsyganok et al., 2012). Similar studies using DANP method have also been published in various journals with a limited number of experts (Tsyganok et al., 2012).
The DANP method have the following steps to do.
Step 1: Compute the direct relation matrix (A)
In this step we analyzed the criteria and success factors of smart contracts in construction industry by reviewing literature and studying in blockchain technology and smart contracts field and sent the DANP questionnaire to the survey experts, and they were asked to answer the influence in criteria by adding numbers 0, 1, 2, 3, 4.
The initial data obtained from the experts in a form of direct relation matrix known as matrix “A” (Equation 1) in which each element aij is denoted as the relationship degree as effect of element i over j.
In this formula (Equation 2), Z is the number of experts and X is defined as matrix elements of each participant.
Step 2: Computing incongruity rate (IR)
We examine the credibility of the respondents by means of this formula.
In (Equation 3),
Step 3: Normalizing Matrix (A) & computing direct relation Matrix (D)
Equation 4 shows how to compute matrix (D)
In this equation,
Step 4: Calculating Total Matrix (T) from Equation 6.
Matrix (I) is identity matrix which is shown here.
Step 5: Computing the cause and effect value.
In this step we calculate and analyze columns and rows of matrix T. D is the sum of the rows and R is the sum of the columns. (D + R) shows the total effect given and received by inhibitors and the degree that inhibitors have in the analyses. (D-R) shows the overall effect of the inhibitors related to the whole system that we call “Relation” (Kumar and Anbanandam, 2020). if D-R is positive, the inhibitor is a variable of a cause, and if it is negative, it is an effect. In the end we draw an Influential network relations map (INRM) which its vertical axis displays (D-R), and horizontal axis displays (D + R). The position of each inhibitor is determined by a point at the coordinates (D + R, D-R).
Step 6: Normalizing total matrix (T)
In each of the matrixes, by dividing the element of each row by the sum of the elements of that row, which are shown in Equation 7, the matrix is normalized. With normalizing all matrixes, which is computed in Equation 8, total matrix (T) is composed.
Step 7: Computing un-weighted super matrix (W)
By shifting the rows and columns of the Total Matrix (T), un-weighted super matrix is computed.
Step 8: Computing weighted super matrix (
Step 9: Limiting weighted super matrix, Determining weights & Prioritizing.
Limited super matrix is computed by powering of all the super matrix elements to be alike until all the super matrix elements are alike. Finally, the relative weight of the criteria of decision is being computed.
(Figure 3) displays the DANP Method that was used for this article as a schematic diagram.
We encountered certain challenges in recruiting qualified individuals with experience in building and contracting, as well as Blockchain and Smart Contracts, to participate in our study within the framework of Iran, a developing nation. Part of the problem with the dearth of specialists in this field is that Blockchain technology is still in its infancy in the country’s industrial sectors, and professors and professionals have paid little attention to it thus far. Notwithstanding these obstacles, we made every effort to contact numerous professionals. We were very picky about the professionals we hired, and we only considered those with extensive experience in the contracting and construction industries as well as a solid grasp of Blockchain technology and smart contracts. Journals, professional networks, and expert groups were among the initial resources we combed through to find and get in touch with possible specialists, then snowball sampling was utilized to expand the invited experts. This method was critical for getting well-informed comments and insights that were applicable to the study’s aims.
Seven experts confirmed their participation in the poll after we conducted thorough outreach and screening. Based on the information that is available and the validity of their expertise in relation to Iran, these individuals constitute the greatest number of specialists that might be considered. Specifically, we wanted to know what was holding Smart Contracts back in the contracting and building sector, so we created this poll to find out. A number of inhibitors were graded by participants as they filled out a questionnaire. The experts participating in the survey reflects the challenges of conducting such a study in a developing country like Iran. Efforts were made to include the maximum number of qualified individuals. Providing a thorough overview of the difficulties and issues to be considered when applying Smart Contracts technology to different sectors, the study’s thorough categorization and analysis of limiting factors adds to its breadth and depth.
The questionnaire we created for this study is designed based on the DANP method and included 17 factors, which were presented to experts in a matrix format. The experts rated each criterion from 0 to 4 based on the impact of the rows and columns (Shao et al., 2018). As mentioned in Section 3.1, 0 means “No Influence”, 1 for “Low Influence”, 2 for “Medium Influence”, 3 for “High Influence” and 4 for “Very High Influence”. The results were presented in a 17*17 matrix across 9 stages, which we will explain in detail in the following section.
As mentioned, to analyze data by DANP method, we have 9 steps. In this section we will conduct them step by step to analyze the results of the various questionnaires.
Step 1: Compute the direct relation matrix (A)
This phase requires seven experts to score from 0 to 4 on the effects of the criteria of the questionnaire, and at the end we have formed a fair average of the responses in the form of the direct relation matrix A, which is shown in Table 3.
Step 2: Computing incongruity rate (IR)
In this step, first, we must remove one expert’s opinions and calculate the estimated average of 6 (N-1) experts; then we shall calculate the total difference in the decimal degrees between the averages of 7 and 6 experts, shown in Tables 4, 5, and finally, based on the adjustment rate, we get a rate of 0.0497, which is below 0.05, and which shows that the experts’ opinions in this questionnaire are reliable.
Step 3: Normalizing Matrix (A) & computing direct relation Matrix (D)
We must divide each criterion of the A matrix into the largest total of rows and columns, and then take the least of them as “b”, which is “b” by applying Formula 9.
Finally, by multiplying the A matrix upon “b”, the D matrix is formed by Table 6.
Step 4: Calculating total Matrix (
The total matrix is a relative quantity of direct and indirect relations, which by powering the D matrix, we reduce the indirect effects of the issue, forming a very healthy total of direct and indirect effects. The total matrix (
Step 5: Calculating total relation Matrix (
In the DANP method, we pass from the total matrix (
Step 6: Normalizing total matrix (
To normalize both matrixes, we divide the criteria into their total entire row; for the
Step 7: Computing un-weighted super matrix (W)
By reversing the normal matrix of criteria, the super matrix is formed.
Step 8: Computing weighted super matrix (
At this step, we form a matrix of 17*17 (in dimensions of the criteria) and by multiplying the criteria in their own group, we form a weighted super matrix, for example, multiplying
Step 9: Computing convergent weighted super matrix.
After the formation of the super matrix, to make a convergency in the matrix, we must multiply it, as you see in Table 8, to the equalization of the criteria.
Step 10: Determining weights and prioritizing criteria.
Step 11: Building the Influential Relation Map (IRM)
To Build the Influential Relation Map, we need the sum of the rows and columns of the
The critical success factors of Smart contracts in the construction industry are weighted and ranked in Table 10.
As shown in Table 10, rank 1 was identified as external factors (D), weighted at 0.28, and the most important group of factors. In this group, the support of the engineering community (
As it is evident in Figure 8, the support of the engineering community (
Rank 2 goes to technical factors with a weight of 0/25. Technology maturity (
As it shows in Figure 7, both technology maturity (
The third category of criteria goes to the legal factors, consists of four criteria on the Transparency of Smart contracts (
Figure 4 displays that Law Legislation (
The fourth and last factors group of the total classification of criteria, with 0.219 weight, is related to programming. Advanced programming languages (
According to Figure 5, Programmers’ skills (
And finally, as shown in Figure 6, you can observe the network relationships between the factor groups. Legal factors (A) impact all three factors and is influenced by only Programming related factors (B). Programming related factors (B) exhibits a two-way relationship with Legal factors (A) and Technical factors (C). Although it has an impact on External factors (D). External factors (D) are the only group that influences other factors and is also influenced by them. However, External factors (D) are influenced by 3 other criteria and only has a direct impact on Technical factors (C), and through this factor, it has an indirect impact on the other 2 factors.
As mentioned in Section 4 “Results” external factors with a weight at 0.28 were identified as rank 1 in group factors. Smart contracts based on Blockchains are a new technology that includes an enormous amount of income and output, especially in the construction industry and it can be a heavy weight in the advancement of financial affairs. Ongoing connection and interaction of engineers community plays a major role (Natarajarathinam et al., 2021). Hence the support and recognition of engineers (
Technical factors are second leading factors. As it shows, the maturity of Smart contracts and Blockchain technology
The third leading group of factors goes to Legals (A). The transparency of Smart contracts (
The last group of factors is related to programming(B). The advancement of programming languages
As with all research, this study has several limitations to be noted. First, our research is based on survey data from a single source at a single point in time. Furthermore, this study was conducted at a specific point in time and in the country of Iran, and it cannot be generalized to all times and all conditions. Also, there are some limitations on the application of DANP method. One of them is that this study is reliance on expert opinion (Kumar and Anbanandam, 2020). The experts’ opinions differ somewhat from each other owing to their different backgrounds (Shao et al., 2018). DANP method is heavily dependent on expert opinions and may introduce bias and partiality into the decision-making process (Naderi et al., 2023). Moreover, the geographical limitations we faced in this study affected the research process and the completion of the questionnaire.
Therefore, this research has been conducted at a specific point in time; further investigations in this area need to be carried out in the future. Also, it is necessary to examine this issue using other methods.
For Smart Contracts to be widely used and effectively implemented, it is essential that technology is advanced enough and that strong security mechanisms are in place. This study’s findings from Iranian specialists highlight the early but promising use of Smart Contracts in the developing world’s building industry. We compiled information from a panel of experts; according to the analysis and findings, technological maturity is the most critical aspect of a successful Smart Contract. Other critical aspects that advanced to the next level are also identified. These factors, along with an awareness of the present state of technology and regulations, are critical for reducing risks and making the most of Smart Contracts on building sites.
Despite the useful preliminary insights provided by this study, it is clear that much more research is required to fully grasp the implications of Smart Contracts and their potential uses beyond the construction industry. Expanding the geographic reach, studying industry-specific and longitudinal difficulties, keeping up with technical improvements, and understanding the changing regulatory environment should all be goals of future investigations. Fostering an atmosphere that is conducive to innovation also requires collecting case studies, concentrating on skill development, and addressing organizational and cultural change. This study lays the groundwork for future research that can lead to the construction industry and other industries in Iran and similar environments adopting Smart Contracts more effectively and efficiently.
The datasets presented in this study can be found in online repositories. The names of the repository/repositories and accession number(s) can be found below: https://data.4tu.nl/private_datasets/DPR6KV1SEtlJxPiVwexXLQColmlj7mu3VB9U8DzMmVE.
The studies involving humans were approved by the Apadana Institute of Higher Education. The studies were conducted in accordance with the local legislation and institutional requirements. The participants provided their written informed consent to participate in this study.
HR: Conceptualization, Data curation, Formal Analysis, Funding acquisition, Investigation, Methodology, Project administration, Resources, Software, Supervision, Validation, Visualization, Writing–original draft, Writing–review and editing. AF: Conceptualization, Data curation, Formal Analysis, Funding acquisition, Investigation, Methodology, Project administration, Resources, Software, Supervision, Validation, Visualization, Writing–original draft, Writing–review and editing. SS: Writing–original draft, Writing–review and editing.
The author(s) declare that no financial support was received for the research, authorship, and/or publication of this article.
The authors declare that the research was conducted in the absence of any commercial or financial relationships that could be construed as a potential conflict of interest.
All claims expressed in this article are solely those of the authors and do not necessarily represent those of their affiliated organizations, or those of the publisher, the editors and the reviewers. Any product that may be evaluated in this article, or claim that may be made by its manufacturer, is not guaranteed or endorsed by the publisher.
Abdallah Zaky, A., and Nassar, H. (2021). The potentials of using smart contracts in the construction industry. Int. J. Of Sci. & Technol. Res. 10 (Issue 09), 2021.
Ahmadisheykhsarmast, S., and Sonmez, R. (2018). “Smart contracts in construction industry,” in 5th international project & construction management conference, 767–774.
Akadiri, P. O., Olomolaiye, P. O., and Chinyio, E. A. (2013). Multi-criteria evaluation model for the selection of sustainable materials for building projects. Automation Constr. 30, 113–125. doi:10.1016/j.autcon.2012.10.004
Alharby, M., and Van Moorsel, A. (2017). Blockchain-based smart contracts: a systematic mapping study. arXiv Prepr. arXiv:1710.06372. doi:10.48550/arXiv.1710.06372
Alias, M. A., Hashim, S. Z. M., and Samsudin, S. (2008). Multi criteria decision making and its applications: a literature review. J. Teknol. Mklm. 20 (2), 129–152.
Altay, H., and Motawa, I. (2020). “An investigation on the applicability of smart contracts in the construction industry,” in Workshop proceedings, 12.
Ameyaw, E. E., Edwards, D. J., Kumar, B., Thurairajah, N., Owusu-Manu, D. G., and Oppong, G. D. (2023). Critical factors influencing adoption of blockchain-enabled smart contracts in construction projects. J. Constr. Eng. Manag. 149 (3), 04023003. doi:10.1061/jcemd4.coeng-12081
Atzei, N., Bartoletti, M., and Cimoli, T. (2017). “A survey of attacks on ethereum smart contracts (sok),” in Principles of Security and Trust: 6th International Conference, POST 2017, Held as Part of the European Joint Conferences on Theory and Practice of Software, ETAPS 2017, April 22-29, 2017 (Uppsala, Sweden: Springer Berlin Heidelberg), 6 164–186. doi:10.1007/978-3-662-54455-6_8
Back, A. (1997). Hashcash. 1997. Available at: http://www.hashcash.org (cit. on p. 3).
Back, A., Goldberg, I., and Shostack, A. (2001). Freedom 2.1 security issues and analysis. Zero Knowledge Systems, Inc. White Paper. Available at: http://www.freedom.net/info/whitepapers/Freedom_Security2-l.pdf.
Balcerzak, A. P., Nica, E., Rogalska, E., Poliak, M., Klieštik, T., and Sabie, O. M. (2022). Blockchain technology and smart contracts in decentralized governance systems. Adm. Sci. 12 (3), 96. doi:10.3390/admsci12030096
Bekrar, A., Ait El Cadi, A., Todosijevic, R., and Sarkis, J. (2021). Digitalizing the closing-of-the-loop for supply chains: a transportation and blockchain perspective. Sustainability 13 (5), 2895. doi:10.3390/su13052895
Capocasale, V., and Perboli, G. (2022). Standardizing smart contracts. IEEE Access 10, 91203–91212. doi:10.1109/access.2022.3202550
Cheng, E. W., and Li, H. (2002). Construction partnering process and associated critical success factors: quantitative investigation. J. Manag. Eng. 18 (4), 194–202. doi:10.1061/(asce)0742-597x(2002)18:4(194)
Chiu, W. Y., Tzeng, G. H., and Li, H. L. (2013). A new hybrid MCDM model combining DANP with VIKOR to improve e-store business. Knowledge-based Syst. 37, 48–61. doi:10.1016/j.knosys.2012.06.017
Chou, J. S., Pham, A. D., and Wang, H. (2013). Bidding strategy to support decision-making by integrating fuzzy AHP and regression-based simulation. Automation Constr. 35, 517–527. doi:10.1016/j.autcon.2013.06.007
Clack, C. D., Bakshi, V. A., and Braine, L. (2016). Smart contract templates: foundations, design landscape and research directions. arXiv Prepr. arXiv:1608.00771. doi:10.48550/arXiv.1612.04496
Contreras-Castillo, J., Zeadally, S., and Guerrero-Ibañez, J. A. (2017). Internet of vehicles: architecture, protocols, and security. IEEE internet things J. 5 (5), 3701–3709. doi:10.1109/jiot.2017.2690902
Corbet, S., Meegan, A., Larkin, C., Lucey, B., and Yarovaya, L. (2018). Exploring the dynamic relationships between cryptocurrencies and other financial assets. Econ. Lett. 165, 28–34. doi:10.1016/j.econlet.2018.01.004
Dalal, J., Mohapatra, P. K., and Chandra Mitra, G. (2010). Prioritization of rural roads: AHP in group decision. Eng. Constr. Archit. Manag. 17 (2), 135–158. doi:10.1108/09699981011024669
Dhawan, D., and Gupta, J. (2017). Comparison of different solvents for phytochemical extraction potential from datura metel plant leaves. Int. J. Biol. Chem. 11 (1), 17–22. doi:10.3923/ijbc.2017.17.22
Drew, D., and Skitmore, M. (1997). The effect of contract type and size on competitiveness in bidding. Constr. Manag. Econ. 15 (5), 469–489. doi:10.1080/014461997372836
Gatteschi, V., Lamberti, F., Demartini, C., Pranteda, C., and Santamaría, V. (2018). Blockchain and smart contracts for insurance: is the technology mature enough? Future internet 10 (2), 20. doi:10.3390/fi10020020
Ghaleb, A. M., Kaid, H., Alsamhan, A., Mian, S. H., and Hidri, L. (2020). Assessment and comparison of various MCDM approaches in the selection of manufacturing process. Adv. Mater. Sci. Eng. 2020, 1–16. doi:10.1155/2020/4039253
Grant, R. M. (1996). Prospering in dynamically-competitive environments: organizational capability as knowledge integration. Organ. Sci. 7 (4), 375–387. doi:10.1287/orsc.7.4.375
Grech, N., Kong, M., Jurisevic, A., Brent, L., Scholz, B., and Smaragdakis, Y. (2018). Madmax: surviving out-of-gas conditions in ethereum smart contracts. Proc. ACM Program. Lang. 2, 1–27. doi:10.1145/3276486
Hamledari, H., and Fischer, M. (2021). Construction payment automation using blockchain-enabled smart contracts and robotic reality capture technologies. Automation Constr. 132, 103926. doi:10.1016/j.autcon.2021.103926
Hartel, P., and van Staalduinen, M. (2019). Truffle tests for free--Replaying Ethereum smart contracts for transparency. arXiv Prepr. arXiv:1907.09208. doi:10.48550/arXiv.1907.09208
Hegedűs, P. (2018). “Towards analyzing the complexity landscape of solidity based ethereum smart contracts,” in Proceedings of the 1st international workshop on emerging trends in software engineering for blockchain, 35–39.
Hewa, T., Ylianttila, M., and Liyanage, M. (2021). Survey on blockchain based smart contracts: applications, opportunities and challenges. J. Netw. Comput. Appl. 177, 102857. doi:10.1016/j.jnca.2020.102857
Hiatt, J. (2006). “ADKAR: a model for change in business, government, and our community.” in Prosci.
Hildenbrandt, E., Saxena, M., Rodrigues, N., Zhu, X., Daian, P., Guth, D., et al. (2018). “Kevm: a complete formal semantics of the ethereum virtual machine,” in 2018 IEEE 31st computer security foundations symposium (CSF) (IEEE), 204–217.
Holotiuk, F., and Moormann, J. (2019). “Dimensions, success factors and obstacles of the adoption of blockchain technology,” in Australasian conference on information systems, 162–172. Available at: https://acis2019.io/pdfs/ACIS2019_PaperFIN_040.pdf.
Huang, Y., Bian, Y., Li, R., Zhao, J. L., and Shi, P. (2019). Smart contract security: a software lifecycle perspective. IEEE Access 7, 150184–150202. doi:10.1109/access.2019.2946988
Hyun, C., Cho, K., Koo, K., Hong, T., and Moon, H. (2008). Effect of delivery methods on design performance in multifamily housing projects. J. Constr. Eng. Manag. 134 (7), 468–482. doi:10.1061/(asce)0733-9364(2008)134:7(468)
Ibrahim, R., Harby, A. A., Nashwan, M. S., and Elhakeem, A. (2022). Financial contract administration in construction via cryptocurrency blockchain and smart contract: a proof of concept. Buildings 12, 1072. doi:10.3390/buildings12081072
Innovalue, L. L. (2015). Blockchain and financial services: industry snapshot and possible future developments. 20 Available at: www.the-blockchain.com/docs/Locke%20Lord.
Jani, S. (2020). Smart contracts: building blocks for digital transformation. Indira Gandhi National Open University. doi:10.13140/RG.2.2.33316.83847
Kale, S., and Arditi, D. (2002). Competitive positioning in United States construction industry. J. Constr. Eng. Manag. 128 (3), 238–247. doi:10.1061/(asce)0733-9364(2002)128:3(238)
Khan, S. N., Loukil, F., Ghedira-Guegan, C., Benkhelifa, E., and Bani-Hani, A. (2021). Blockchain smart contracts: applications, challenges, and future trends. Peer-to-peer Netw. Appl. 14 (5), 2901–2925. doi:10.1007/s12083-021-01127-0
Kirli, D., Couraud, B., Robu, V., Salgado-Bravo, M., Norbu, S., Andoni, M., et al. (2022). Smart contracts in energy systems: a systematic review of fundamental approaches and implementations. Renew. Sustain. Energy Rev. 158, 112013. doi:10.1016/j.rser.2021.112013
Kumar, A., and Anbanandam, R. (2020). Analyzing interrelationships and prioritising the factors influencing sustainable intermodal freight transport system: a grey-DANP approach. J. Clean. Prod. 252, 119769. doi:10.1016/j.jclepro.2019.119769
Kushwaha, S. S., Joshi, S., Singh, D., Kaur, M., and Lee, H. N. (2022). Ethereum smart contract analysis tools: a systematic review. IEEE Access 10, 57037–57062. doi:10.1109/access.2022.3169902
Lam, K. C., Lam, M. C. K., and Wang, D. (2008). MBNQA-oriented self-assessment quality management system for contractors: fuzzy AHP approach. Constr. Manag. Econ. 26 (5), 447–461. doi:10.1080/01446190801965350
Lamport, L. (1998). The part-time parliament. ACM Trans. Comput. Syst. 16 (2), 133–169. ACM Transactions on Computer Systems. doi:10.1145/279227.279229
Lone, A. H., and Naaz, R. (2021). Applicability of Blockchain smart contracts in securing Internet and IoT: a systematic literature review. Comput. Sci. Rev. 39, 100360. doi:10.1016/j.cosrev.2020.100360
Naderi, H., Heydari, M. H., and Parchami Jalal, M. (2023). Risk analysis in implementing building energy performance projects: hybrid DANP-VIKOR model analysis—a case study in Iran. Buildings 13 (8), 2066. doi:10.3390/buildings13082066
Nakamoto, S. (2008). “Bitcoin: a peer-to-peer electronic cash system. — in Decentralized Business Review 21260.
Nanayakkara, S., Perera, S., Senaratne, S., Weerasuriya, G. T., and Bandara, H. M. N. D. (2021). Blockchain and smart contracts: a solution for payment issues in construction supply chains. Informatics 8, 36. doi:10.3390/informatics8020036
Natarajarathinam, M., Qiu, S., and Lu, W. (2021). Community engagement in engineering education: a systematic literature review. J. Eng. Educ. 110 (4), 1049–1077. doi:10.1002/jee.20424
Nejad, T. A., Behbodi, M. R., and Ravanfar, M. M. (2015). Analyzing organizational structure based on 7s model of McKinsey. Int. J. Acad. Res. Bus. Soc. Sci. 5 (5), 43–55. doi:10.6007/ijarbss/v5-i5/1591
Nugent, T., Upton, D., and Cimpoesu, M. (2016). Improving data transparency in clinical trials using blockchain smart contracts. F1000Research 5, 2541. doi:10.12688/f1000research.9756.1
Oliveira, T., and Martins, M. F. (2011). Literature review of information technology adoption models at firm level. Electron. J. Inf. Syst. Eval. 14 (12011), pp110–121.
Ølnes, S., Ubacht, J., and Janssen, M. (2017). Blockchain in government: benefits and implications of distributed ledger technology for information sharing. Gov. Inf. Q. 34 (3), 355–364. doi:10.1016/j.giq.2017.09.007
Pan, W., Dainty, A. R., and Gibb, A. G. (2012). Establishing and weighting decision criteria for building system selection in housing construction. J. Constr. Eng. Manag. 138 (11), 1239–1250. doi:10.1061/(asce)co.1943-7862.0000543
Parizi, R. M., Amritraj, , and Dehghantanha, A. (2018). “Smart contract programming languages on blockchains: an empirical evaluation of usability and security,” in Blockchain–ICBC 2018: first international conference, held as part of the services conference federation, SCF 2018, Seattle, WA, USA, June 25-30, 2018, proceedings 1 (Springer International Publishing), 75–91.
Perera, S., Nanayakkara, S., Rodrigo, M. N. N., Senaratne, S., and Weinand, R. (2020). Blockchain technology: is it hype or real in the construction industry? J. industrial Inf. integration 17, 100125. doi:10.1016/j.jii.2020.100125
Porru, S., Pinna, A., Marchesi, M., and Tonelli, R. (2017). “Blockchain-oriented software engineering: challenges and new directions,” in 2017 IEEE/ACM 39th international conference on software engineering companion (ICSE-C) (IEEE), 169–171.
Rathnayake, D. B., Razzaghi, R., and Bahrani, B. (2022). Generalized virtual synchronous generator control design for renewable power systems. IEEE Trans. Sustain. Energy 13 (2), 1021–1036. doi:10.1109/tste.2022.3143664
Regulation, P. (2016). Regulation (EU) 2016/679 of the European parliament and of the council, 679, 2016. Regulation (eu).
Reyna, A., Martín, C., Chen, J., Soler, E., and Díaz, M. (2018). On blockchain and its integration with IoT. Challenges and opportunities. Future gener. Comput. Syst. 88, 173–190. doi:10.1016/j.future.2018.05.046
Rogers, E. M., Singhal, A., and Quinlan, M. M. (2014). “Diffusion of innovations,” in An integrated approach to communication theory and research (Routledge), 432–448.
Sayeed, S., Marco-Gisbert, H., and Caira, T. (2020). Smart contract: attacks and protections. IEEE Access 8, 24416–24427. doi:10.1109/access.2020.2970495
Schatsky, D., and Muraskin, C. (2015). Beyond bitcoin: blockchain is coming to disrupt your industry. Deloitte Insight. 7.
Schmitt, G., Mladenow, A., Strauss, C., and Schaffhauser-Linzatti, M. (2019). Smart contracts and Internet of things: a qualitative content analysis using the technology-organization-environment framework to identify key-determinants. Procedia Comput. Sci. 160, 189–196. doi:10.1016/j.procs.2019.09.460
Sergey, I., and Hobor, A. (2017). “A concurrent perspective on smart contracts,” in Financial cryptography and data security: FC 2017 international workshops, WAHC, BITCOIN, VOTING, WTSC, and TA, sliema, Malta, april 7, 2017, revised selected papers 21 (Springer International Publishing), 478–493.
Shao, Q. G., Liou, J. J., Weng, S. S., and Chuang, Y. C. (2018). Improving the green building evaluation system in China based on the DANP method. Sustainability 10 (4), 1173. doi:10.3390/su10041173
Sharma, P., Jindal, R., and Borah, M. D. (2023). A review of smart contract-based platforms, applications, and challenges. Clust. Comput. 26 (1), 395–421. doi:10.1007/s10586-021-03491-1
Shojaei, A., and Naderi, H. (2024). “Blockchain technology for a circular built environment,” in A circular Built environment in the digital age (Cham: Springer International Publishing), 213–228.
Shojaei, A., Wang, J., and Fenner, A. (2020). Exploring the feasibility of blockchain technology as an infrastructure for improving built asset sustainability. Built Environ. Proj. Asset Manag. 10 (2), 184–199. doi:10.1108/bepam-11-2018-0142
Sigalov, K., Ye, X., König, M., Hagedorn, P., Blum, F., Severin, B., et al. (2021). Automated payment and contract management in the construction industry by integrating building information modeling and blockchain-based smart contracts. Appl. Sci. 11 (16), 7653. doi:10.3390/app11167653
Singh, N., Tang, Y., Zhang, Z., and Zheng, C. (2020). COVID-19 waste management: effective and successful measures in Wuhan, China. Resour. conservation, Recycl. 163, 105071. doi:10.1016/j.resconrec.2020.105071
Sun, G., Zhu, C., Kramer, M. H., Yang, S. S., Song, W., Piepho, H. P., et al. (2010). Variation explained in mixed-model association mapping. Heredity 105 (4), 333–340. doi:10.1038/hdy.2010.11
Tabatabaei, M. H., Vitenberg, R., and Veeraragavan, N. R. (2023). Understanding blockchain: definitions, architecture, design, and system comparison. Comput. Sci. Rev. 50, 100575. doi:10.1016/j.cosrev.2023.100575
Taherdoost, H. (2023). Smart contracts in blockchain technology: a critical review. Information 14 (2), 117. doi:10.3390/info14020117
Tann, W. J. W., Han, X. J., Gupta, S. S., and Ong, Y. S. (2018). Towards safer smart contracts: a sequence learning approach to detecting security threats. arXiv Prepr. arXiv:1811.06632. doi:10.48550/arXiv.1811.06632
Tsyganok, V. V., Kadenko, S. V., and Andriichuk, O. V. (2012). Significance of expert competence consideration in group decision making using AHP. Int. J. Prod. Res. 50 (17), 4785–4792. doi:10.1080/00207543.2012.657967
Tzeng, G. H., Tsaur, S. H., Laiw, Y. D., and Opricovic, S. (2002a). Multicriteria analysis of environmental quality in Taipei: public preferences and improvement strategies. J. Environ. Manag. 65 (2), 109–120. doi:10.1006/jema.2001.0527
Ullah, Z., Al-Turjman, F., Mostarda, L., and Gagliardi, R. (2020). Applications of artificial intelligence and machine learning in smart cities. Comput. Commun. 154, 313–323. doi:10.1016/j.comcom.2020.02.069
Wang, S., Yuan, Y., Wang, X., Li, J., Qin, R., and Wang, F. Y. (2018). “An overview of smart contract: architecture, applications, and future trends,” in 2018 IEEE intelligent vehicles symposium (IV) (IEEE), 108–113.
Weng, H. H., Chen, J. S., and Chen, P. C. (2015). Effects of green innovation on environmental and corporate performance: a stakeholder perspective. Sustainability 7 (5), 4997–5026. doi:10.3390/su7054997
Ye, X., Zeng, N., and König, M. (2022). Systematic literature review on smart contracts in the construction industry: potentials, benefits, and challenges. Front. Eng. Manag. 9, 196–213. doi:10.1007/s42524-022-0188-2
Zhang, G., and Zou, P. X. (2007). Fuzzy analytical hierarchy process risk assessment approach for joint venture construction projects in China. J. Constr. Eng. Manag. 133 (10), 771–779. doi:10.1061/(asce)0733-9364(2007)133:10(771)
Zou, P. X., and Li, J. (2010). Risk identification and assessment in subway projects: case study of Nanjing Subway Line 2. Constr. Manag. Econ. 28 (12), 1219–1238. doi:10.1080/01446193.2010.519781
Keywords: smart contracts, blockchain, success factors, construction, industry
Citation: Rasti H, Feili A and Sorooshian S (2024) Analysing critical success factor of smart contract in construction industry with DANP approach. Front. Built Environ. 10:1478239. doi: 10.3389/fbuil.2024.1478239
Received: 24 August 2024; Accepted: 11 November 2024;
Published: 27 November 2024.
Edited by:
Zhen Chen, University of Strathclyde, United KingdomReviewed by:
Grit Ngowtanasuwan, Mahasarakham University, ThailandCopyright © 2024 Rasti, Feili and Sorooshian. This is an open-access article distributed under the terms of the Creative Commons Attribution License (CC BY). The use, distribution or reproduction in other forums is permitted, provided the original author(s) and the copyright owner(s) are credited and that the original publication in this journal is cited, in accordance with accepted academic practice. No use, distribution or reproduction is permitted which does not comply with these terms.
*Correspondence: Hooman Rasti, aG9vbWFucmFzdGkxOTk3QGdtYWlsLmNvbQ==; Ardalan Feili, ZmVpbGlAYXBhZGFuYS5hYy5pcg==; Shahryar Sorooshian, c2hhaHJ5YXIuc29yb29zaGlhbkBndS5zZQ==
Disclaimer: All claims expressed in this article are solely those of the authors and do not necessarily represent those of their affiliated organizations, or those of the publisher, the editors and the reviewers. Any product that may be evaluated in this article or claim that may be made by its manufacturer is not guaranteed or endorsed by the publisher.
Research integrity at Frontiers
Learn more about the work of our research integrity team to safeguard the quality of each article we publish.