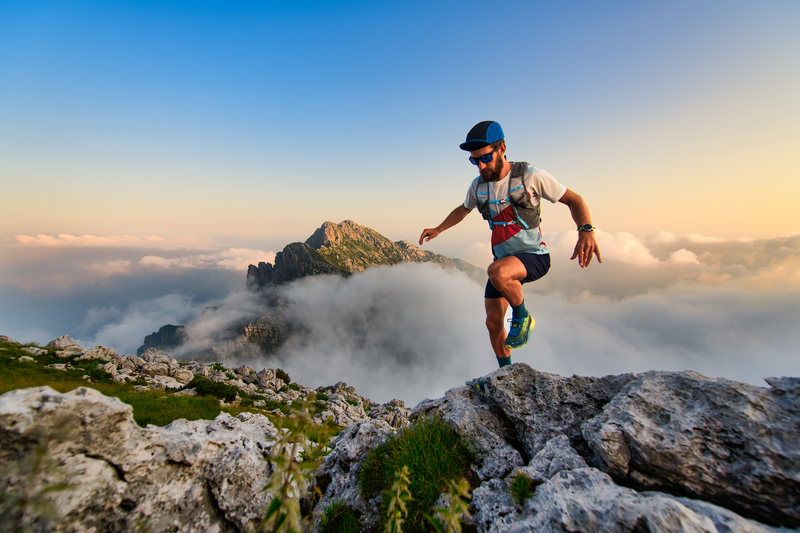
95% of researchers rate our articles as excellent or good
Learn more about the work of our research integrity team to safeguard the quality of each article we publish.
Find out more
ORIGINAL RESEARCH article
Front. Built Environ. , 06 November 2024
Sec. Construction Materials
Volume 10 - 2024 | https://doi.org/10.3389/fbuil.2024.1455915
The compressive strength behavior of high-strength geopolymer concrete (HSGPC) has been studied in this research work with varying alkali concentration using the novel machine learning techniques. The alkali concentration in the activation solution plays a significant role in the geopolymerization process and affects the resulting compressive strength. In this research work, the range between 4 M and 16 M for alkali molarity (M), 18 kg/m3 and 160 kg/m3 for NaOH and 41 kg/m3 and 229 kg/m3 for NaSi was collected from literature and used in the various design mixes of this exercise. This was necessary because higher alkali concentrations promote a more efficient dissolution and activation of the aluminosilicate compounds, leading to increased geopolymerization and the formation of more calcium silicate hydrate (C-S-H) gel. The increased C-S-H gel content contributes to improved strength development. However, there is an optimal alkali concentration range for the sustainable production of geopolymer concrete, and exceeding this range can have a negative impact on compressive strength and ecofriendly handling of concrete. A total of fifty-three records were collected from literature and deployed in modeling the compressive strength (Fc) considering various curing regimes. Three symbolic machine learning techniques such as genetic programming (GP), evolutionary polynomial regression (EPR), and the artificial neural network (ANN) are used in this research model. The relative importance values for each input parameter were also evaluated, which indicated that all factors have significant impacts on (Fc), but Age (i.e., curing regime) has the most influence compared to FA, NaOH, and CAg then the other inputs. From the model relations between the calculated and predicted values, it can be shown that the decisive model, ANN produced line of parametric equation of y = 0.995x, and produced performance indices; MAE of 2.13 MPa, RMSE of 2.86 MPa and R-squared of 0.981, which makes the ANN the most reliable model in agreement with previous applications of the technique. These are against the poor performance of the EPR and GP, which produced R-squared less than 0.8 with higher error rates. The Taylor chart and the variance distribution, which further compares the accuracy and variances of the developed models support the outcomes. Generally, alkali molarity has shown its potential in the production of HSGPC due to its role in the reactivity phases of the concrete formulation; hydration, activation, pozzolanic, and geopolymerization reactions producing the gel needed for the strength gain in HSGPC.
High-strength geopolymer concrete is a type of concrete that is produced using geopolymers as a binder material instead of traditional Portland cement (Verma, 2023; Ahmad et al., 2022; Ahmad et al., 2022b; Ahmad et al., 2021). Geopolymers are inorganic polymers that are typically derived from industrial by-products such as fly ash, slag, or other aluminosilicate materials (Alyaseen et al., 2023a; Alyaseen et al., 2023b). Geopolymer concrete offers several advantages over conventional Portland cement-based concrete (Verma, 2023; Forouzandeh Jounaghani et al., 2023). Geopolymer concrete can achieve higher compressive and flexural strength compared to ordinary Portland cement concrete. It can exhibit compressive strengths in the range of 50–100 MPa or even higher. Geopolymer concrete has excellent resistance to chemical attack, abrasion, and fire. It can withstand harsh environments and is less prone to deterioration and cracking. Geopolymers are typically produced from industrial by-products, which helps in reducing the consumption of traditional cement and the associated carbon dioxide emissions. Geopolymer concrete has lower embodied carbon and contributes to sustainable construction practices. Geopolymer concrete exhibits better thermal insulation properties than ordinary concrete, making it suitable for applications where thermal efficiency is important, such as in cold climates or for energy-efficient buildings. Geopolymer concrete can gain strength rapidly, allowing for faster construction schedules and reduced curing times. However, there are some challenges associated with the use of geopolymer concrete. It requires careful mixture design and optimization to achieve the desired properties. The sourcing and availability of suitable raw materials can also be a limitation in some regions. Additionally, geopolymer concrete may have a different appearance and color compared to traditional concrete, which may require aesthetic considerations in certain applications (Verma, 2023; Forouzandeh Jounaghani et al., 2023). On overall, high-strength geopolymer concrete is a promising alternative to conventional concrete, offering enhanced performance and sustainability benefits. Ongoing research and development in this field aim to further improve its properties and optimize its use in various construction applications.
The mix design of high-strength geopolymer concrete plays a crucial role in determining its compressive strength. Geopolymer concrete is a type of concrete that utilizes fly ash or other pozzolanic materials as a binder instead of traditional Portland cement. The compressive strength of geopolymer concrete depends on several factors related to mix design (Verma, 2023; Forouzandeh Jounaghani et al., 2023). The type and composition of the binder materials used in geopolymer concrete significantly affect its compressive strength. The selection of fly ash or other pozzolanic materials, their particle size distribution, chemical composition, and reactivity play a vital role in the geopolymerization process (Gogineni et al., 2023). Optimal binder composition should be determined through experimental testing and optimization to achieve higher compressive strength. Geopolymer concrete requires an alkaline activator solution to initiate the geopolymerization reaction. The concentration and type of alkaline activator, such as sodium hydroxide (NaOH) or potassium hydroxide (KOH), can influence the compressive strength (Verma, 2023). The ratio of activator solution to binder materials should be carefully determined to achieve the desired strength. The properties of aggregates, including particle size, shape, grading, and surface texture, can affect the compressive strength of geopolymer concrete. Well-graded aggregates with a suitable particle size distribution help in achieving better packing and interlocking, leading to improved strength. Additionally, the use of high-quality aggregates with low impurities is essential for achieving high-strength geopolymer concrete. The water-to-binder ratio (W/B ratio) affects the workability and strength of geopolymer concrete. A lower W/B ratio generally results in higher compressive strength due to a higher concentration of binder materials and a reduced porosity (Verma, 2023; Forouzandeh Jounaghani et al., 2023; Gogineni et al., 2023). However, it is essential to balance the W/B ratio to maintain workability while maximizing strength. Proper curing is critical to developing the desired compressive strength in geopolymer concrete (Jain and Marathe, 2023; Onyelowe and Ebid, 2023; Onyelowe et al., 2023). The curing temperature, duration, and moisture conditions influence the geopolymerization reaction and the formation of a strong, dense matrix (Verma, 2023). Adequate curing conditions, such as elevated temperatures (typically around 60°C–90°C) and moisture retention, should be maintained for an appropriate duration to achieve optimal strength development (Onyelowe et al., 2021a; Onyelowe et al., 2021b; Shen et al., 2022; Khan and Ali, 2018). It is important to note that the influences mentioned above are interdependent and should be considered collectively during the mix design process (Gogineni et al., 2023; Jain and Marathe, 2023). Achieving high compressive strength in geopolymer concrete requires a balance between all the influencing factors to optimize the mix proportions and achieve the desired performance characteristics (Verma, 2023; Forouzandeh Jounaghani et al., 2023). Experimental testing and optimization are typically conducted to develop an optimized mix design for high-strength geopolymer concrete (Kumar and Pratap, 2023).
The alkali molarity, or concentration, has a significant influence on the pozzolanic reaction in high-strength geopolymer concrete (Mortar et al., 2020; Ebid et al., 2023; Hoffman et al., 1983; Kashyap et al., 2024). The pozzolanic reaction refers to the chemical reaction between the alkaline activator and the pozzolanic materials (such as fly ash or slag) to form a geopolymer binder (Verma, 2023; Forouzandeh Jounaghani et al., 2023; Gogineni et al., 2023; Jain and Marathe, 2023; Onyelowe and Ebid, 2023; Onyelowe et al., 2023; Onyelowe et al., 2021a; Onyelowe et al., 2021b; Ahmad and Rafiq, 2023). Alkali molarity affects the kinetics of the pozzolanic reaction. Higher alkali molarity generally accelerates the reaction rate, leading to faster geopolymer formation (Pratap et al., 2023; Iqbal et al., 2020). This can result in shorter setting times and early strength development (Onyelowe et al., 2021b). Conversely, lower alkali molarity may slow down the reaction kinetics, requiring longer curing periods to achieve the desired strength. Alkali molarity influences the extent of activation of the pozzolanic materials (Liu et al., 2021). Optimal alkali molarity ensures sufficient contact and reaction between the alkaline activator and the pozzolanic materials, promoting the dissolution and transformation of reactive silica and alumina into geopolymer gels (Verma, 2023; Gogineni et al., 2023; Jain and Marathe, 2023). Deviating from the optimal molarity range can reduce the degree of activation and compromise the strength development. The alkali molarity affects the structure and morphology of the geopolymer binder formed during the pozzolanic reaction (Verma, 2023; Forouzandeh Jounaghani et al., 2023; Onyelowe et al., 2021b). Higher alkali molarity generally promotes the formation of a denser and more compact geopolymer matrix, resulting in increased strength and improved durability properties. Lower alkali molarity may lead to a less dense structure, which can affect the strength and permeability characteristics of the concrete. Alkali molarity influences the chemical composition of the geopolymer binder. Higher alkali molarity can result in a higher concentration of alkali ions within the geopolymer matrix, affecting its overall composition and properties (Onyelowe et al., 2021a). This can impact the mechanical strength, chemical resistance, and long-term durability of the high-strength geopolymer concrete. Alkali molarity plays a role in the formation of geopolymer gels and subsequent curing (Jain and Marathe, 2023; Onyelowe et al., 2021b). The concentration of alkali ions affects the solubility and availability of reactive species, influencing the gel formation and curing process. Optimal alkali molarity ensures proper gel formation and curing conditions, leading to improved strength development. It is important to note that alkali molarity should be considered in conjunction with other factors such as the type and composition of pozzolanic materials, water-to-binder ratio, curing conditions, and aggregate properties (Verma, 2023; Forouzandeh Jounaghani et al., 2023). An experimental approach, combined with proper mix design and optimization techniques, is typically employed to determine the optimal alkali molarity for achieving the desired strength and performance characteristics of high-strength geopolymer concrete (Verma, 2023; Forouzandeh Jounaghani et al., 2023; Onyelowe et al., 2021b). The alkali molarity, or concentration, has a significant influence on the geopolymerization process and subsequently on the properties of high-strength geopolymer concrete (Ahmed H. U. et al., 2022). Alkali molarity affects the rate of geopolymerization reaction. Higher alkali molarity generally leads to a faster reaction rate, resulting in a shorter setting time and early strength development (Ahmed et al., 2023b). Conversely, lower alkali molarity may slow down the reaction, necessitating longer curing periods to achieve the desired strength. The compressive strength of geopolymer concrete is influenced by the alkali molarity. Increasing the alkali molarity within an optimal range can enhance the chemical reaction and promote the formation of a denser geopolymer matrix, leading to higher compressive strength (Jain and Marathe, 2023). However, excessively high alkali molarity can result in excessive shrinkage, cracking, and reduced strength. Alkali molarity affects the workability of geopolymer concrete. A higher alkali molarity may decrease the workability by accelerating the setting time and reducing the fluidity of the mix (Verma, 2023; Onyelowe et al., 2023). This can make the concrete more difficult to handle and place. Conversely, lower alkali molarity can increase workability but may require longer curing times for strength development. The alkali molarity influences the porosity and density of geopolymer concrete. Higher alkali molarity generally promotes the formation of a denser structure with reduced porosity, resulting in increased strength and durability (Onyelowe et al., 2023). Lower alkali molarity may lead to a more porous matrix, which can affect the strength and permeability characteristics of the concrete. The efficiency of alkali activation in geopolymer concrete is affected by the alkali molarity. Optimal alkali molarity ensures sufficient contact and reaction between the alkali activator and the pozzolanic materials, maximizing the geopolymerization process (Verma, 2023; Gogineni et al., 2023; Onyelowe et al., 2023). Deviating from the optimal molarity range can reduce the activation efficiency and compromise the strength development. It is crucial to note that the influence of alkali molarity on geopolymerization in high-strength geopolymer concrete is interconnected with other factors, such as the type and composition of pozzolanic materials, curing conditions, water-to-binder ratio, and aggregate properties (Verma, 2023; Forouzandeh Jounaghani et al., 2023; Onyelowe et al., 2023; Onyelowe et al., 2021b). An experimental approach, combined with proper mix design and optimization techniques, is typically employed to determine the optimal alkali molarity for achieving the desired strength and performance characteristics of high-strength geopolymer concrete (Ahmed et al., 2023c). The alkali molarity in high-strength geopolymer concrete can have a significant influence on its compressive strength. The alkali solution, typically sodium hydroxide (NaOH) or potassium hydroxide (KOH), is an essential component in the activation of the geopolymer reaction. Here are some key points regarding the influence of alkali molarity on compressive strength: The molarity or concentration of the alkali solution affects the geopolymerization process. Generally, higher alkali molarity leads to faster reaction kinetics and higher early-age strength development (Verma, 2023; Forouzandeh Jounaghani et al., 2023). However, excessively high alkaline concentrations can cause a rapid increase in heat generation and may result in a decrease in long-term strength. Increasing the alkali molarity can enhance the compressive strength of high-strength geopolymer concrete. This is mainly attributed to the increased dissolution and activation of the aluminosilicate materials, resulting in more geopolymer gel formation and a denser microstructure (Verma, 2023; Forouzandeh Jounaghani et al., 2023; Onyelowe et al., 2021b). The geopolymer gel contributes to the binding and strength development of the concrete matrix. (iii) Optimum alkali molarity: There is typically an optimum alkali molarity range for achieving the highest compressive strength. This range depends on factors such as the specific geopolymer formulation, type and fineness of the raw materials, curing conditions, and desired strength requirements (Verma, 2023; Forouzandeh Jounaghani et al., 2023). It is important to conduct laboratory trials and optimize the alkali molarity to obtain the desired strength properties. Higher alkali molarity can reduce the workability of the geopolymer concrete mix (Ahmed et al., 2023a). This is due to increased viscosity and faster setting time. It may be necessary to adjust the mix design, including the water-to-binder ratio and the use of superplasticizers, to maintain the desired workability while achieving the target compressive strength (Verma, 2023; Jain and Marathe, 2023). It's worth noting that the influence of alkali molarity on compressive strength may vary depending on other factors such as the specific geopolymer mix design, curing conditions, and the presence of additional additives or fillers. Therefore, it is recommended to conduct thorough laboratory testing and optimization to determine the most suitable alkali molarity for achieving high-strength geopolymer concrete with the desired compressive strength properties.
Curing time and temperature play crucial roles in the development of compressive strength in high-strength geopolymer concrete. Geopolymer concrete gains strength rapidly during the initial curing period. The first few days of curing are particularly important for achieving early-age strength development. Curing time significantly affects the long-term compressive strength of geopolymer concrete. Extended curing durations allow for further geopolymerization reactions, resulting in continued strength development and improved overall performance. There is an optimum curing time for achieving the highest compressive strength. It depends on factors such as geopolymer mix design, alkali concentration, ambient conditions, and desired strength requirements (Verma, 2023; Forouzandeh Jounaghani et al., 2023). Longer curing times are generally beneficial for attaining higher strengths, but there may be diminishing returns beyond a certain duration. Elevated curing temperatures can accelerate the geopolymerization reaction and promote faster strength gain. Higher temperatures increase the chemical kinetics and diffusion rates, leading to earlier strength development. Geopolymerization is an exothermic reaction, but the rate of reaction is also influenced by temperature. Higher temperatures reduce the activation energy required for the reaction, resulting in faster and more complete geopolymerization. Thermal curing, using elevated temperatures (typically between 40°C and 90°C), can be employed to achieve high early-age strengths and shorten the curing time. However, careful control is necessary to avoid detrimental effects such as excessive shrinkage or thermal cracking. Geopolymer concrete can also be cured at ambient temperatures, but the curing time will be longer compared to thermal curing (Gogineni et al., 2023; Jain and Marathe, 2023). Ambient curing is more suitable for large-scale applications where thermal curing may not be practical or cost-effective. It's important to note that the influence of curing time and temperature on compressive strength is interrelated and depends on the specific geopolymer mix design, alkali concentration, binder composition, and other factors (Onyelowe et al., 2021b). Optimal curing conditions should be determined through laboratory testing and optimization, considering the desired strength requirements and project-specific constraints. Proper curing practices, whether thermal or ambient, are essential for achieving the desired compressive strength and overall performance of high-strength geopolymer concrete.
High-strength geopolymer concrete offers several sustainability benefits compared to conventional concrete (Onyelowe et al., 2021b). Geopolymer concrete can significantly lower the carbon footprint of construction projects. Traditional Portland cement production is a major source of carbon dioxide emissions. Geopolymers, on the other hand, are typically produced using industrial by-products such as fly ash or slag, which are waste materials from other industries (Verma, 2023; Forouzandeh Jounaghani et al., 2023). By utilizing these by-products, geopolymer concrete reduces the demand for traditional cement, thereby reducing carbon dioxide emissions. The production of geopolymer concrete requires lower curing temperatures compared to conventional concrete. This lower curing temperature reduces energy consumption during the curing process, leading to improved energy efficiency. Geopolymer concrete provides a solution for the utilization of industrial by-products that would otherwise be disposed of as waste. By incorporating these materials into the geopolymer binder, geopolymer concrete contributes to the circular economy by reducing waste generation and promoting resource efficiency. Geopolymer concrete has excellent durability properties, including resistance to chemical attack, abrasion, and fire. Its long service life reduces the need for frequent repairs or replacement, resulting in reduced material consumption and waste generation over the lifecycle of the structure. Geopolymer concrete exhibits better thermal insulation properties compared to conventional concrete. This characteristic can contribute to energy savings during the operation of buildings by reducing the need for heating and cooling. Geopolymer concrete can be produced using locally available industrial by-products, reducing the need for long-distance transportation of raw materials (Verma, 2023; Forouzandeh Jounaghani et al., 2023; Jain and Marathe, 2023). This aspect contributes to a lower environmental impact associated with transportation and supports regional or local sourcing of materials. Remarkedly, Alyaseen et al. (2023a) had investigated the compressive strength of geopolymer concrete however with the addition of recycled aggregates and remarkable results were achieved, which changed the perspective of the production of geopolymer concrete in the built environment. Alyaseen et al. (2023b) also went further to conduct a comprehensive review on the performance of self-compacting concrete mixed with recycled aggregate concrete exposing areas of future investigation. It's important to note that while geopolymer concrete offers sustainability advantages, there are still challenges to be addressed. These include optimizing mix designs, ensuring consistent quality, and addressing the availability of suitable raw materials on a larger scale. Ongoing research and development efforts are focused on improving these aspects to enhance the sustainability of high-strength geopolymer concrete.
Predicting the compressive strength of high-strength geopolymer concrete can be achieved using various techniques, including Artificial Neural Networks (ANN), Genetic Programming (GP), and Evolutionary Polynomial Regression (EPR). ANNs are computational models inspired by the human brain’s neural structure (Ahmed H. U. et al., 2022). They are effective in capturing nonlinear relationships and can be trained to predict the compressive strength of geopolymer concrete (Onyelowe et al., 2023; Shen et al., 2022; Khan and Ali, 2018; Kumar and Pratap, 2023; Mortar et al., 2020). To develop an ANN model, you would need a dataset containing input variables (such as mix proportions, curing conditions, and chemical compositions) and corresponding compressive strength values. The dataset is divided into training and testing sets. The ANN model is trained using the training set, and its performance is evaluated using the testing set. The architecture of the ANN, including the number of hidden layers and neurons, is determined through experimentation and optimization. GP is an evolutionary computation technique that evolves computer programs to solve a specific problem (Onyelowe and Ebid, 2023; Onyelowe et al., 2023; Onyelowe et al., 2021a). In this case, you would formulate the problem as a symbolic regression task, where GP searches for an equation that represents the relationship between the input variables and the compressive strength. A population of candidate equations is randomly generated, and through successive generations, the fittest equations are selected, recombined, and mutated to produce new generations. The fitness of each equation is determined by how well it predicts the compressive strength. GP continues until it finds a satisfactory equation that accurately predicts the compressive strength of geopolymer concrete. EPR is another evolutionary computation technique that combines genetic programming with polynomial regression. It evolves both the structure and coefficients of polynomial equations to fit the data. EPR begins by generating a population of polynomial equations with random coefficients. Through generations, the fittest equations are selected, and their structure and coefficients are modified using genetic operators, such as crossover and mutation. The fitness of each equation is determined by how well it predicts the compressive strength. EPR continues until it finds a polynomial equation that adequately represents the relationship between the input variables and compressive strength (Onyelowe and Ebid, 2023). It is important to note that the success of each technique depends on the quality and representativeness of the dataset, as well as proper model training and validation. Each method has its advantages and limitations, and their performance may vary depending on the specific problem and dataset. Therefore, it is recommended to compare and evaluate the results obtained from each technique to determine the most accurate and reliable approach for predicting the compressive strength of high-strength geopolymer concrete. This research paper has focused on the important role alkalis place in the formulation of activators utilized in geopolymers applied in the production of geopolymer concrete. Care is taken in the utilization of different dosages of molarity of alkali due to environmental considerations and pozzolanic reaction triggering potential (Onyelowe and Ebid, 2023; Onyelowe et al., 2021b). Various other research protocols have been undertaken to formulate the geopolymer concrete with most sharing ideas on the impregnation of concrete with nanosilica and with a few discussing the machine learning application of ANN and M5P (Ahmed H. U. et al., 2022; Ahmed et al., 2023b). The roadmap to sustainability requires that construction materials meet the requirement of the COP27 towards carbon neutrality and environmentally friendly guidelines (Onyelowe et al., 2023; Mortar et al., 2020). Having reviewed the above available resources, it is important to emphasize that the present work focused on the impact of the alkali molarity as an important component in the production of geopolymer concretes (GPCs) on its compressive strength.
A total of fifty-three (53) records were collected from experimentally tested samples of high-strength geopolymer concrete with different NaOH and NaSi dosages and this is contained in a previous literature (Mortar et al., 2020).
Each record contains the following data:
• FA Fly Ash content (kg/m3)
• NaOH sodium hydroxide content (kg/m3)
• NaSi Sodium silicate content (kg/m3)
• FAg Fine Aggregates content (kg/m3)
• CAg Coarse Aggregates content (kg/m3)
• M Molarity (mole)
• T Temperature (oC)
• Age Concrete age (day)
• Fc Compressive strength (MPa)
The collected records were divided into training set (40 records) and validation set (13 records) following the requirements of Ebid et al. (2023). The process of developing and validating symbolic machine methods and Multiple Linear Regression (MLR) models usually involves several stages. Initially, data preprocessing is conducted to prepare the data properly, which includes tasks such as handling missing values, outliers, and inconsistencies through data cleaning, selecting relevant features to enhance model performance, and scaling numerical features via normalization or standardization. Next, the data is divided into training and testing sets according to established guidelines (Ebid et al., 2023), and the model is built. Model validation is then carried out using the necessary statistical indices. Regularization technique was applied to overcome overfitting due to database size (Ebid et al., 2023). Tables 1, 2 summarizes their statistical characteristics and the Pearson correlation matrix. Finally, Figure 1 shows the histograms for both inputs and outputs and Figure 2 shows the relations between the inputs and the outputs. Table 1 shows the minimum (Min), maximum (Max), average (Avg), standard deviation (SD) and variance (Var) values of the input (FA, NaOH, NaSi, FAg, CAg, M, T, and Age) variables and the output (Fc) for both the training and validation entries. Table 2 shows the consistency and correlation between the input and output variables. It can be observed that none of the parameters showed a consistency of up to 50%. However, FA and T produced a correlation of a little above 40%, which is still very poor. The necessitated the intelligent modeling of the compressive strength of the HSGPC to optimize the values of the variables at which the optimal strength id produced for concrete production, design and construction purposes. Figure 2 further shows the relations between all the independent variables and the dependent Fc. The correlations with the Fc are as follows; FA = 16%, NaOH = 3.3%, NaSi = 14.8%, FAg = 2.1, CAg = 13%, M = 3%, T = 10%, and Age = 65%. It can be shown that curing regime produced the highest correlation of 65% with the Fc, while NaOH, FAg, and M produced the lowest set of correlations; 3.3%, 2.1%, and 3%, respectively with the Fc. The primary focus of the work, which is on the influence of the alkali molarity (M), reinforces the need to further model the parameters using the machine learning techniques.
Three different artificial intelligent (AI) techniques were used to predict the compressive strengths (Fc) of geo-polymer concrete with NaOH and NaSi using the collected database. These techniques are “genetic programming” (GP), three models of “artificial neural network” (ANN) and “evolutionary polynomial regression” (EPR). Flowcharts for the used techniques are presented in Figure 3. All the three developed models were used to predict (Fc) in (MPa) using mix contents (FA, NaOH, NaSi, FAg and CAg), Molarity (M) and Temperature (T) with the Age of concrete (Age) in days. The following section discusses the results of each model. The accuracies of developed models were evaluated by comparing the sum of squared error (SSE), mean average error (MAE), mean squared error (MSE), root mean squared error (RMSE), and R-squared (R2) between the predicted and calculated shear strength parameters values. A preliminary sensitivity analysis was carried out on the collected database to estimate the impact of each input on the (Fc) values. “Single variable per time” technique is used to determine the “Sensitivity Index” (SI) for each input using Hoffman et al. (1983) formula as follows:
Accordingly, the (SI) values are (0.04, 0.42, 0.22, 0.02, 0.02, 0.53, 0.18 and 0.92) for (FA, NaOH, NaSi, FAg, CAg, M, T and Age), respectively. A sensitivity index of 1.0 indicates complete sensitivity, a sensitivity index less than 0.01 indicates that the model is insensitive to changes in the parameter.
Genetic programming (GP) the framework of which is shown in Figure 4, is a type of evolutionary algorithm inspired by the process of natural selection. It is used for evolving computer programs to solve problems or perform tasks through the iterative application of genetic operators such as mutation, crossover, and selection. GP starts with a population of randomly generated programs, which are represented as trees, and evolves them over generations to improve their performance according to a fitness measure (Ebid et al., 2023). Intelligent computation refers to the broader field of computational techniques and methodologies that draw inspiration from biological, cognitive, or other natural processes to develop intelligent systems. This encompasses a wide range of computational paradigms, including evolutionary algorithms (such as genetic programming), neural networks, fuzzy systems, swarm intelligence, and more. Intelligent computation techniques aim to solve complex problems by mimicking or emulating intelligent behavior observed in nature or human cognition. In summary, genetic programming is a specific technique within the broader domain of intelligent computation, focusing on evolving computer programs using principles derived from biological evolution. Intelligent computation encompasses various computational paradigms that aim to solve problems by emulating intelligent behavior observed in natural systems or human cognition. In genetic programming (GP) computation, the terms “parents,” “offspring,” and “cross-points” refer to key concepts in the evolutionary process.
In GP, parents refer to the individuals or programs selected from the population to undergo reproduction. These individuals are chosen based on their fitness, typically using a selection mechanism such as tournament selection, roulette wheel selection, or rank-based selection. Parents serve as the basis for generating new offspring through genetic operators like crossover and mutation. Offspring are the new individuals or programs created through the reproduction process from the selected parents. Offspring inherit genetic material from their parents through genetic operators such as crossover and mutation. Offspring are essentially the next-generation of programs in the GP population and undergo evaluation to determine their fitness for survival and potential for further evolution. Cross-points, also known as crossover points, are specific locations or nodes within the program trees where crossover occurs during reproduction (Ebid et al., 2023). In GP, crossover is a genetic operator that involves swapping subtrees between two parent programs to create new offspring. Cross-points determine where the exchange of genetic material takes place, and they play a crucial role in generating diverse offspring with different combinations of traits from their parents. In summary, in genetic programming computation, parents are selected from the population based on fitness, offspring are generated from parents through genetic operators like crossover and mutation, and cross-points define the locations where crossover occurs during reproduction to create new offspring. These concepts are fundamental to the evolutionary process in GP, enabling the generation of new individuals with improved characteristics over successive generations. The overall process of GP can be summarized using the following equation:
where Pt is the population at generation t, Select is the selection mechanism of the computation interface, Reproduce encompasses the genetic operators (crossover and mutation) and replacement strategy. This equation captures the iterative nature of GP, where each generation of the population is produced from the previous generation through selection, reproduction, and replacement, ultimately leading to the evolution of solutions to the optimization problem.
Artificial neural networks (ANNs), the framework of which is shown in Figure 5, are computational models inspired by the structure and function of biological neural networks in the human brain (Ahmed H. U. et al., 2022). ANNs consist of interconnected nodes, called neurons or units, organized in layers. These networks can learn complex patterns and relationships from data and are widely used for tasks such as classification, regression, pattern recognition, and optimization. Here’s an overview of the key components and concepts of artificial neural networks: Neurons (Nodes): Neurons are the basic processing units in artificial neural networks. Each neuron receives input signals, processes them using an activation function, and produces an output signal. Neurons are typically organized in layers: an input layer, one or more hidden layers, and an output layer.
Weights and Bias: Connections between neurons are represented by weights, which determine the strength of influence one neuron has on another. Additionally, each neuron has a bias term, which allows the network to learn and model complex relationships in the data. Activation Function: The activation function of a neuron determines its output based on the weighted sum of its inputs. Common activation functions include sigmoid, hyperbolic tangent (tanh), rectified linear unit (ReLU), and softmax. Activation functions introduce non-linearity into the network, enabling it to model complex relationships in the data. Feedforward Propagation: In the feedforward phase, input data is passed through the network layer by layer, with each layer applying a weighted sum and activation function to produce outputs. This process generates predictions or classifications based on the input data. Backpropagation: Backpropagation is an optimization algorithm used to train neural networks by adjusting the weights and biases based on the difference between predicted and actual outputs. This process involves computing the gradient of the loss function with respect to the network parameters and updating the weights and biases using gradient descent or its variants. Loss Function: The loss function measures the difference between the predicted outputs of the network and the true labels in supervised learning tasks. Common loss functions include mean squared error (MSE) for regression tasks and cross-entropy loss for classification tasks. Training Data and Validation: Neural networks are trained using labeled training data, where both input features and target outputs are provided. The training process involves iteratively adjusting the network parameters to minimize the loss function on the training data. Validation data is used to monitor the performance of the network during training and prevent overfitting. Architecture and Hyperparameters: The architecture of a neural network refers to its structure, including the number of layers, the number of neurons in each layer, and the connectivity between neurons. Hyperparameters, such as learning rate, batch size, and regularization strength, are settings that control the training process and influence the performance of the network. Artificial neural networks have demonstrated remarkable success in various fields, including image recognition, natural language processing, speech recognition, and medical diagnosis, among others. They continue to be a fundamental tool in machine learning and artificial intelligence research and applications.
The performance of artificial neural network (ANN) computations is influenced by several key factors, including activation functions, hyperparameters, loss functions, weights, and biases. Activation functions introduce non-linearity into the network, allowing it to model complex relationships in the data. The choice of activation function can significantly impact the network’s ability to learn and generalize from the data. Common activation functions include sigmoid, hyperbolic tangent (tanh), rectified linear unit (ReLU), and softmax. The selection of the appropriate activation function depends on the specific task and the characteristics of the data. Hyperparameters are settings that control the training process of the neural network. They include parameters such as learning rate, batch size, number of epochs, dropout rate, and regularization strength. The choice of hyperparameters can have a significant impact on the convergence speed, generalization performance, and stability of the network. Tuning hyperparameters through techniques such as grid search, random search, or Bayesian optimization is essential for achieving optimal performance. Loss functions measure the difference between the predicted outputs of the network and the true labels in supervised learning tasks. The choice of loss function depends on the nature of the task (e.g., regression or classification) and the distribution of the data. Common loss functions include mean squared error (MSE) for regression tasks and cross-entropy loss for classification tasks. Using an appropriate loss function is crucial for training the network effectively and optimizing its performance. Weights and biases are parameters in the neural network that determine the strength of connections between neurons and the neuron’s activation threshold, respectively. The initial values of weights and biases, as well as their updates during training (e.g., through backpropagation), affect the network’s ability to learn and generalize from the data. Proper initialization of weights and biases, along with appropriate regularization techniques, can prevent issues such as vanishing or exploding gradients and improve the network’s convergence and performance. In summary, activation functions, hyperparameters, loss functions, weights, and biases all play critical roles in determining the performance of artificial neural network computations. Understanding how these factors interact and tuning them appropriately is essential for building effective and reliable neural network models for various tasks and applications. The general mathematical equation governing an artificial neural network (ANN) can be expressed in Equation 3:
where: y is the output of the neural network. f is the activation function applied element-wise to the result of the linear transformation. w is the weight matrix, representing the connections between neurons in adjacent layers. x is the input vector or matrix. b is the bias vector or matrix. The activation function of the ANNs as shown in the architectural framework is illustrated in Equation 4;
Evolutionary polynomial regression (EPR) is a technique used for fitting polynomial functions to data using evolutionary algorithms. Unlike traditional polynomial regression, which typically involves selecting the polynomial degree manually, EPR automatically determines the polynomial degree and coefficients through an evolutionary optimization process. Initialization: EPR starts by initializing a population of candidate polynomial models. Each model represents a potential solution to the regression problem and is encoded as a mathematical expression involving polynomial terms and coefficients. Evaluation: The fitness of each candidate model is evaluated using a fitness function, which measures how well the model fits the training data. This typically involves calculating the root mean squared error (RMSE) or another metric that quantifies the difference between the model’s predictions and the actual target values. Evolutionary Process: EPR employs evolutionary algorithms, such as genetic algorithms or genetic programming, to evolve the population of candidate models over multiple generations. During each generation, the following steps are performed: Selection: A subset of candidate models is selected for reproduction based on their fitness. Variation: New candidate models (offspring) are generated through genetic operators such as crossover and mutation. Crossover involves combining parts of two parent models to create new offspring, while mutation introduces small random changes to the models. Replacement: The offspring replace some of the existing candidate models in the population, typically based on their fitness. Termination: The evolutionary process continues for a fixed number of generations or until a termination condition is met, such as reaching a satisfactory fitness level or running out of computational resources. Best Model Selection: After the evolutionary process is complete, the best-performing model in the final population is selected as the solution to the regression problem. This model represents the polynomial function that best fits the training data according to the chosen fitness metric. EPR offers several advantages over traditional polynomial regression, including the ability to automatically determine the polynomial degree and coefficients without the need for manual intervention. Additionally, EPR can handle non-linear relationships between variables more effectively and is less prone to overfitting, especially in cases where the optimal polynomial degree is unknown. However, EPR may require more computational resources and longer computation times compared to traditional regression methods due to the nature of the evolutionary optimization process. The general mathematical equation of Evolutionary Polynomial Regression (EPR) can be represented in Equation 5:
where: y is the output variable. Xi are the input variables. wi are the coefficients or weights associated with each input variable. pi are the polynomial degrees corresponding to each input variable. n is the number of input variables. In EPR, the goal is to find the optimal values of the coefficients wi and polynomial degrees, pi that minimize the difference between the predicted output y and the actual output for a given set of input variables xi. This optimization process is typically performed using evolutionary algorithms, where candidate solutions (models) are evaluated based on their fitness, which is often measured using a metric such as the root mean square error (RMSE) or another objective function. EPR is capable of automatically determining the polynomial degrees pi and coefficients wi that best fit the training data, without the need for manual selection of the polynomial degree. This makes it a versatile and powerful technique for regression tasks, particularly in cases where the relationship between the input and output variables is non-linear and complex.
When analyzing the performance of models using various metrics such as sum of squared errors (SSE), mean absolute error (MAE), mean squared error (MSE), root mean squared error (RMSE), and R-squared, each metric provides different insights into the accuracy and goodness of fit of the model (Kashyap et al., 2024). SSE measures the total squared difference between the predicted values and the actual values. It gives an overall measure of the model’s error, where lower SSE indicates better model performance. See Equation 6
MSE measures the average of the squared differences between the predicted values and the actual values. It penalizes larger errors more heavily than smaller errors. See Equation 7
RMSE is the square root of the MSE and provides a measure of the average magnitude of the errors in the predicted values. It is in the same unit as the target variable. See Equation 8
R-squared measures the proportion of the variance in the dependent variable (target) that is explained by the independent variables (features) in the model. It ranges from 0 to 1, where higher values indicate better model fit. See Equation 9
In summary, when analyzing models’ performance using SSE, MAE, MSE, RMSE, and R-squared, it's important to consider the specific characteristics of each metric and how they relate to the problem at hand (Kashyap et al., 2024). Lower values of SSE, MAE, MSE, and RMSE indicate better model performance, while higher values of R-squared indicate a better fit of the model to the data. It's often useful to consider multiple metrics together to gain a comprehensive understanding of the model’s performance.
The compressive strength behavior of high-strength geopolymer concrete studied in this research work with varying alkali concentration can be influenced by several factors. Geopolymer concrete is a cementitious material that is produced by activating aluminosilicate materials, such as fly ash or slag, with an alkaline solution as the activator. The alkali concentration in the activating solution plays a significant role in the geopolymerization process and can affect the resulting compressive strength. In the present research work, the range between 18 kg/m3 and 160 kg/m3 for NaOH and 41 kg/m3 and 229 kg/m3 for NaSi was used in the various design mixes of this exercise (Mortar et al., 2020). In general, the compressive strength of geopolymer concrete tends to increase with increasing alkali concentration within a certain range as presented in the table in Appendix. This is because higher alkali concentrations promote a more efficient dissolution and activation of the aluminosilicate materials, leading to increased geopolymerization and the formation of more calcium silicate hydrate (C-S-H) gel (Onyelowe et al., 2021b; Mortar et al., 2020; Ahmad and Rafiq, 2023; Pratap et al., 2023). The increased C-S-H gel content contributes to improved strength development. However, there is an optimal alkali concentration range for geopolymer concrete, and exceeding this range can have a negative impact on compressive strength. At very high alkali concentrations, excessive dissolution and reaction may occur, leading to the formation of a less dense and weaker geopolymer gel. This can result in a decrease in compressive strength and other mechanical properties. It is important to note that the optimal alkali concentration range can vary depending on the type and properties of the aluminosilicate materials used, the specific activators employed, and the curing conditions (Onyelowe et al., 2021b; Mortar et al., 2020). Therefore, experimental investigations and machine learning-based optimization studies have been typically conducted and reported to determine the suitable alkali concentration for achieving high-strength geopolymer concrete. It's also worth mentioning that in addition to alkali concentration, other factors such as the Si/Al ratio of the aluminosilicate material, curing temperature, curing regime, and the inclusion of supplementary cementitious materials or additives can also influence the compressive strength behavior of geopolymer concrete (Onyelowe et al., 2021b; Mortar et al., 2020). Therefore, a comprehensive understanding of the interplay between these factors is important in designing and producing high-strength geopolymer concrete with desired properties.
Five GP models were developed with complexity levels ranged between two and six. The population size, survivor size and number of generations were 1,000, 300 and 2000, respectively. Figure 6 shows the improvement in accuracy with increasing the complexity. Equation 10 presented the output formula for (Fc) from the last trial. The average error % of total dataset is (20%), while the (R2) value is (0.771). This model produced a closed-form equation with which this model can manually be applied in the evaluation of the optimal compressive strength of the HSGPC, with the molarity (M), curing regime in terms of age and temperature and the FAg forming the primary factors of the parametric equation. This has substantiated the importance of these factors to the behavior of the modeled parameter (Fc). However, the model could only perform with 77.1% accuracy.
The developed EPR model was limited to sixth level polynomial, for 8 inputs, there are 1716 possible terms (792 + 330+120 + 36+8 + 1 = 1716) as shown in Equation 11:
GA technique was applied on these 1716 terms to select the most effective terms to predict the values of (UCS). The process began with only 5 terms and increased gradually up to 25 terms, Figure 7 presents the enhancement of fitness with increasing the number of terms and indicates that 5 is the optimum number of terms. The output is illustrated in Equation 12. The average error % and (R2) values were (22% and 0.703) respectively. Once more, the EPR with its GA powered interface produced a closed-form equation, which performed in the modeling of the Fc with an accuracy of 70.3%. In this parametric equation, the FA added its relevance unlike in the GP parametric equation.
Three (ANN) models were developed to predict (Fc) values. All the models used normalization method (−1.0 to 1.0), activation function (Hyper Tan) and “Back propagation” (BP) training algorithm. Only the number of neurons in the hidden layer was increased from 1 to 3 to find out the optimum layout of the network. The SSE and R2 values of the six ANN’s and the optimum network layout are illustrated in Figures 8, 9 while the weight matrix of the model is showed in Table 3. The average error % of total dataset is (6%) and the (R2) value is (0.981). The three models in this ANN model execution are the 8-1-1, 8-2-1, and the 8-3-1 architectures. These three varying neurons of the hidden structure reduced the error produced by the model in that order as the hidden layers increased and this is agreeable to previous work (Liu et al., 2021).
The relative importance values for each input parameter are illustrated in Figure 10, which indicated that all factors have significant impacts on (Fc), but Age (i.e., curing regime) has the most influence compared to FA, NaOH, and CAg then the other inputs. The relations between calculated and predicted values are shown in Figure 9. The outliers are greatest with the EPR line of fit with a parametric equation of y = 0.960x, MAE of 8.12 MPa, RMSE of 10.2 MPa, while the GP with less outliers in its line of fit structure beyond the ±25% envelop produced a parametric fit expression of y = 0.973x, MAE of 7.07 MPa, and RMSE of 9.17 MPa and the best model, ANN produced line of parametric equation of y = 0.995x, MAE of 2.13 MPa and RMSE of 2.86 MPa. The GP, EPR, and the ANN produced R-squared of 0.703, 0.771, and 0.981, respectively, which makes the ANN the decisive model in agreement with previous applications of the technique (Onyelowe and Ebid, 2023; Onyelowe et al., 2023; Onyelowe et al., 2021a; Shen et al., 2022; Pratap et al., 2023). Also, in the previous application of this same database, the present research prediction techniques have outperformed it showing superior techniques in the prediction of geopolymer concrete strength considering the varying alkali molarities in the activator mix (Mortar et al., 2020; Iqbal et al., 2020; Liu et al., 2021). The Taylor chart presented in Figure 11 and the variance distribution in Figure 12, which further compares the accuracy and variances of the developed models support the outcomes recorded in Figure 13. The summary of the model operations’ performance accuracies is presented in Table 4.
Figure 13. Relation between predicted and calculated (Fc) values using the developed models. (A)for GP, (B)for EPR, (C)for ANN.
This research presents three models using (AI) techniques (GP, ANN and EPR) to predict the compressive strengths (Fc) of high-strength geopolymer concrete (HSGPC) with NaOH and NaSi using mix contents (FA, NaOH, NaSi, FAg and CAg), Molarity (M) and Temperature (T) with the Age of concrete (Age) (i.e., curing regime) in days. The influence of the alkali molarity (M) was closely observed. The results of comparing the accuracies of the developed models could be concluded in the following points:
• (ANN) model showed the best accuracy of 94%, while both (GP) and (EPR) models had almost the same accuracy of 80%.
• (EPR) depended on only four inputs (Age, T, FA and CAg). Also, (GP) model depended on only four inputs (Age, T, FAg and M). This indicates the high importance of both (Age and T) and also give a reason for the low accuracy of these models.
• (ANN) results indicated that all inputs had significant impacts on the (Fc) values. (Age) is the most influential input, then (FA, NaOH, CAg) and finally (FAg, NaSi, T and M).
• The developed models are valid within the considered range of parameter values, beyond this range; the prediction accuracy should be verified.
• Generally, alkali molarity has shown its potential in the production of HSGPC due to its role in the reactivity phases of the concrete formulation; hydration, activation, pozzolanic, and geopolymerization reactions producing the gel needed for the strength gain in HSGPC.
The research database can be expanded in future research papers to included various other formation components of the geopolymer cement like those with the addition of nanosilica, microsilica, rice husk ash, and other industrial and agro waste materials. The size of the database collected from (Mortar et al., 2020) was a little limitation, which was overcome by regularization technique, but expanding the data entries to incorporate other materials components and their impacts on the behavior of the studied concrete, there may not be any need for the rigors of machine learning regularization. Also, the metaheuristic machine learning techniques can be deployed to compare their speed and robustness in the computation of the compressive strength of geopolymer concretes.
The original contributions presented in the study are included in the article/Supplementary Material, further inquiries can be directed to the corresponding authors.
NU: Data curation, Formal Analysis, Funding acquisition, Investigation, Methodology, Project administration, Resources, Validation, Visualization, Writing–review and editing. KO: Conceptualization, Data curation, Formal Analysis, Funding acquisition, Investigation, Methodology, Project administration, Resources, Software, Supervision, Validation, Visualization, Writing–original draft, Writing–review and editing. AE: Data curation, Formal Analysis, Funding acquisition, Investigation, Methodology, Project administration, Resources, Software, Supervision, Validation, Visualization, Writing–original draft, Writing–review and editing. CC: Data curation, Formal Analysis, Funding acquisition, Investigation, Validation, Writing–review and editing. MZ: Data curation, Funding acquisition, Investigation, Methodology, Validation, Writing–review and editing. AA: Data curation, Formal Analysis, Funding acquisition, Investigation, Methodology, Visualization, Writing–review and editing. MO: Data curation, Formal Analysis, Funding acquisition, Investigation, Methodology, Visualization, Writing–review and editing.
The author(s) declare that no financial support was received for the research, authorship, and/or publication of this article.
The authors declare that the research was conducted in the absence of any commercial or financial relationships that could be construed as a potential conflict of interest.
The Supplementary Material for this article can be found online at: https://www.frontiersin.org/articles/10.3389/fbuil.2024.1455915/full#supplementary-material
FA, Fly Ash content (kg/m3); NaOH, Sodium hydroxide content (kg/m3); NaSi, Sodium silicate content (kg/m3); FAg, Fine Aggregates content (kg/m3); CAg, Coarse Aggregates content (kg/m3); M, Molarity (mole); T, Temperature (oC); Age, Concrete age (day); Fc, Compressive strength (MPa); SSE, sum of squared error; MAE, mean average error; MSE, mean squared error; RMSE, root mean squared error; R2, R-squared; GP, genetic programming; EPR, evolutionary polynomial regression; ANN, artificial neural network; SI, sensitivity analysis; HSGPC, high-strength geopolymer concrete; MPa, megapascal; Kg/m3, kilogram per cubic meter; MLR, multilinea.
Ahmad, A., Ahmad, W., Aslam, F., and Joyklad, P. (2022). Compressive strength prediction of fly ash-based geopolymer concrete via advanced machine learning techniques. Case Stud. Constr. Mater. 16, e00840. doi:10.1016/j.cscm.2021.e00840
Ahmad, A., Ahmad, W., Chaiyasarn, K., Ostrowski, K. A., Aslam, F., Zajdel, P., et al. (2021). Prediction of geopolymer concrete compressive strength using novel machine learning algorithms. Polymers 13 (19), 3389. doi:10.3390/polym13193389
Ahmad, S. A., and Rafiq, S. K. (2023). Numerical modeling to predict the impact of granular glass replacement on mechanical properties of mortar. Asian J. Civ. Eng. 25, 19–37. doi:10.1007/s42107-023-00753-8
Ahmed, H. U., Mahmood, L. J., Muhammad, M. A., Faraj, R. H., Qaidi, S. M. A., Nadhim, H. S., et al. (2022b). Geopolymer concrete as a cleaner construction material: an overview on materials and structural performances. Clean. Mater. 5, 100111. doi:10.1016/j.clema.2022.100111
Ahmed, H. U., Mohammed, A. A., and Mohammed, A. S. (2023a). Effectiveness of nano-SiO2 on the mechanical, durability, and microstructural behavior of geopolymer concrete at different curing ages. Arch. Civ. Mech. Eng. 23, 129. doi:10.1007/s43452-023-00668-w
Ahmed, H. U., Mohammed, A. S., and Mohammed, A. A. (2022a). Proposing several model techniques including ANN and M5P-tree to predict the compressive strength of geopolymer concretes incorporated with nano-silica. Environ. Sci. Pollut. Res. 29, 71232–71256. doi:10.1007/s11356-022-20863-1
Ahmed, H. U., Mohammed, A. S., and Mohammed, A. A. (2023b). RETRACTED: fresh and mechanical performances of recycled plastic aggregate geopolymer concrete modified with Nano-silica: experimental and computational investigation. Constr. Build. Mater. 394, 132266. doi:10.1016/j.conbuildmat.2023.132266
Ahmed, H. U., Mohammed, A. S., and Mohammed, A. A. (2023c). Engineering properties of geopolymer concrete composites incorporated recycled plastic aggregates modified with nano-silica. J. Build. Eng. 75, 106942. doi:10.1016/j.jobe.2023.106942
Alyaseen, A., Poddar, A., Hunnain Alahmad, N. K., Parveen, S., and Sihag, P. (2023b). High-performance self-compacting concrete with recycled coarse aggregate: comprehensive systematic review on mix design parameters. J. Struct. Integr. Maintenance 8 (3), 161–178. doi:10.1080/24705314.2023.2211850
Alyaseen, A., Poddar, A., Kumar, N., Tajjour, S., Prasad, C. V. S. R., Alahmad, H., et al. (2023a). High-performance self-compacting concrete with recycled coarse aggregate: soft-computing analysis of compressive strength. J. Build. Eng. 77, 107527. doi:10.1016/j.jobe.2023.107527
Ebid, A., Onyelowe, K. C., and Deifalla, A. F. (2023). “Data utilization and partitioning for machine learning applications in civil engineering,” in International Conference on Advanced Technologies for Humanity, ICATH, Morocco, 25-26 December, 2023.
Forouzandeh Jounaghani, M., Jahangiri, A., and Jamekhorshid, A. (2023). Experimental investigation on the durability of metakaolin-based geopolymer concrete in aggressive environments. Asian J. Civ. Eng. 24, 1567–1581. doi:10.1007/s42107-023-00589-2
Gogineni, A., Panday, I. K., Kumar, P., and Paswan, R. K. (2023). Predicting compressive strength of concrete with fly ash and admixture using XGBoost: a comparative study of machine learning algorithms. Asian J. Civ. Eng. 25, 685–698. doi:10.1007/s42107-023-00804-0
Hoffman, F. O., and Gardner, R. H. (1983). “Evaluation of uncertainties in radiological assessment models,” in Chapter 11 of radiological assessment: a textbook on environmental dose analysis. Editors J. E. Till, and H. R. Meyer (Washington, D. C: NRC Office of Nuclear Reactor Regulation).
Iqbal, M. F., Liu, Q. F., Azim, I., Zhu, X., Yang, J., Javed, M. F., et al. (2020). Prediction of mechanical properties of green concrete incorporating waste foundry sand based on gene expression programming. J. Hazard. Mater. 384, 121322. doi:10.1016/j.jhazmat.2019.121322
Jain, A., and Marathe, S. and S. A. (2023). Soft computing modeling on air-cured slag-fly ash-glass powder-based alkali activated masonry elements developed using different industrial waste aggregates. Asian J. Civ. Eng. 24, 1515–1527. doi:10.1007/s42107-023-00584-7
Kashyap, V., Alyaseen, A., and Poddar, A. (2024). Supervised and unsupervised machine learning techniques for predicting mechanical properties of coconut fiber reinforced concrete. Asian J. Civ. Eng. 25, 3879–3899. doi:10.1007/s42107-024-01018-8
Khan, M., and Ali, M. (2018). Effect of superplasticizer on the properties of medium strength concrete prepared with coconut fiber. Constr. Build. Mater. 182, 703–715. doi:10.1016/j.conbuildmat.2018.06.150
Kumar, P., and Pratap, B. (2023). Feature engineering for predicting compressive strength of high-strength concrete with machine learning models. Asian J. Civ. Eng. 25, 723–736. doi:10.1007/s42107-023-00807-x
Liu, Q. F., Iqbal, M. F., Yang, J., Lu, X. Y., Zhang, P., and Rauf, M. (2021). Prediction of chloride diffusivity in concrete using artificial neural network: modelling and performance evaluation. Constr. Build. Mater. 268, 121082. doi:10.1016/j.conbuildmat.2020.121082
Mortar, N. A. M., Kamarudin, H., Rafiza, R. A., Meor, T. A. F., and Rosnita, M. (2020). Compressive strength of fly ash geopolymer concrete by varying sodium hydroxide molarity and aggregate to binder ratio. IOP Conf. Ser. Mater. Sci. Eng. 864, 012037. doi:10.1088/1757-899X/864/1/012037
Onyelowe, K. C., and Ebid, A. M. (2023). The influence of fly ash and blast furnace slag on the compressive strength of high-performance concrete (HPC) for sustainable structures. Asian J. Civ. Eng. 25, 861–882. doi:10.1007/s42107-023-00817-9
Onyelowe, K. C., Ebid, A. M., Hanandeh, S., Moghal, A. A. B., Onuoha, I. C., Obianyo, I. I., et al. (2023). The influence of fines on the hydro-mechanical behavior of sand for sustainable compacted liner and sub-base construction applications. Asian J. Civ. Eng. 25, 623–635. doi:10.1007/s42107-023-00800-4
Onyelowe, K. C., Iqbal, M., Jalal, F. E., Onyia, M. E., and Onuoha, I. C. (2021a). Application of 3-algorithm ANN programming to predict the strength performance of hydrated-lime activated rice husk ash treated soil. Multiscale Multidiscip. Model. Exp. Des. 4 (4), 259–274. doi:10.1007/s41939-021-00093-7
Onyelowe, K. C., Onyia, M. E., Bui Van, D., Baykara, H., and Ugwu, H. U. (2021b). Pozzolanic reaction in clayey soils for stabilization purposes: a classical overview of sustainable transport geotechnics. Adv. Mater. Sci. Eng. 2021. Article ID 6632171, 7. doi:10.1155/2021/6632171
Pratap, B., Mondal, S., and Rao, B. H. (2023). Prediction of compressive strength of bauxite residue-based geopolymer mortar as pavement composite materials: an integrated ANN and RSM approach. Asian J. Civ. Eng. 25, 597–607. doi:10.1007/s42107-023-00797-w
Shen, Z., Deifalla, A. F., Kamiński, P., and Dyczko, A. (2022). Compressive strength evaluation of ultra-high-strength concrete by machine learning. Materials 15 (10), 3523. doi:10.3390/ma15103523
Keywords: high-strength geopolymer concrete, compressive strength, machine learning, curing temperature and time, alkali molarity, geopolymerization
Citation: Ulloa N, Onyelowe KC, Ebid AM, Curay Yaulema CS, Zuiga Rodguez MG, Adrade Vally AI and Onyia ME (2024) Influence of alkali molarity on compressive strength of high-strength geopolymer concrete using machine learning techniques based on curing regimes and temperature. Front. Built Environ. 10:1455915. doi: 10.3389/fbuil.2024.1455915
Received: 27 June 2024; Accepted: 21 October 2024;
Published: 06 November 2024.
Edited by:
Hosam Saleh, Egyptian Atomic Energy Authority, EgyptReviewed by:
Afaq Ahmad, University of Engineering and Technology, Taxila, PakistanCopyright © 2024 Ulloa, Onyelowe, Ebid, Curay Yaulema, Zuiga Rodguez, Adrade Vally and Onyia. This is an open-access article distributed under the terms of the Creative Commons Attribution License (CC BY). The use, distribution or reproduction in other forums is permitted, provided the original author(s) and the copyright owner(s) are credited and that the original publication in this journal is cited, in accordance with accepted academic practice. No use, distribution or reproduction is permitted which does not comply with these terms.
*Correspondence: Néstor Ulloa, bmVzdG9yLnVsbG9hQGVzcG9jaC5lZHUuZWM=; Kennedy C. Onyelowe, a29ueWVsb3dlQG1vdWF1LmVkdS5uZw==, ay5vbnllbG93ZUBnby51b3AuZ3I=, a29ueWVsb3dlQGdtYWlsLmNvbQ==; Ahmed M. Ebid, YWhtZWQuYWJkZWxraGFsZXFAZnVlLmVkdS5lZw==
Disclaimer: All claims expressed in this article are solely those of the authors and do not necessarily represent those of their affiliated organizations, or those of the publisher, the editors and the reviewers. Any product that may be evaluated in this article or claim that may be made by its manufacturer is not guaranteed or endorsed by the publisher.
Research integrity at Frontiers
Learn more about the work of our research integrity team to safeguard the quality of each article we publish.