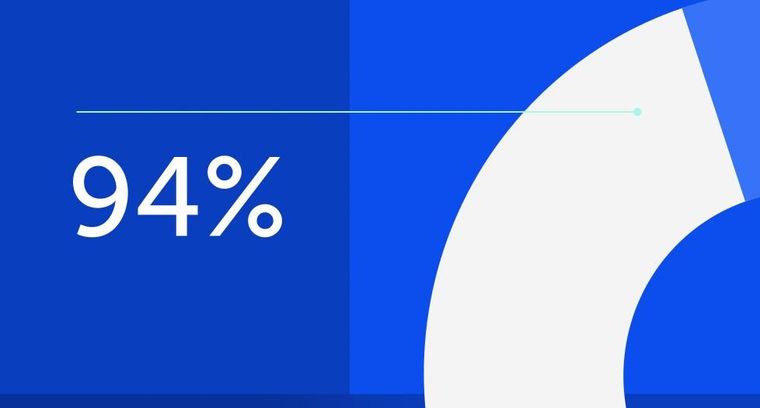
94% of researchers rate our articles as excellent or good
Learn more about the work of our research integrity team to safeguard the quality of each article we publish.
Find out more
PERSPECTIVE article
Front. Built Environ., 04 January 2023
Sec. Structural Sensing, Control and Asset Management
Volume 8 - 2022 | https://doi.org/10.3389/fbuil.2022.1045134
This article is part of the Research TopicStructural Sensing for Asset ManagementView all 5 articles
Managing existing civil infrastructure is challenging due to evolving functional requirements, material aging, and climate change. With increasingly limited economic, environmental, and material resources, more sustainable solutions for practical asset management are required. Significant efforts have been made to monitor civil infrastructure, such as bridges. In-situ measurements are collected with the aim of improving the accuracy of structural capacity evaluations. Monitoring data collected through bridge load testing, continuous condition monitoring, and non-destructive tests provides structural-behavior information that could significantly influence structural-safety examinations. Nonetheless, monitoring techniques are often costly, and the monitoring costs may not always justify the benefits of the information gained. This paper proposes a short perspective of the potential impact of monitoring activities to assess the structural safety of existing bridges. A full-scale bridge in Switzerland is used as an example. Future research needs are also proposed.
The examination of existing structures typically relies on construction drawings, recorded information on the materials, and visual inspection (Bertola and Brühwiler, 2021). Missing information, such as material properties or rebar layouts, is typically compensated by conservative assumptions that follow new-design principles (Brühwiler et al., 2012). In Switzerland, structural examinations are then made using the degree of compliance (DoC),
Collecting monitoring data has the potential to unlock the untapped reserve capacity of existing infrastructure, thus improving decision-making without putting users at risk (Smith, 2016). For example, better knowledge of structural performance through monitoring may be leveraged to extend service durations, optimize structural rehabilitation, focus inspection, and prioritize maintenance activities (Frangopol et al., 2008).
Two main types of structural monitoring exist and cover the evaluation of the structural capacity for a given period of time, called structural performance monitoring (SPM) (Feng et al., 2004), and the continuous diagnosis of the structural condition under operating conditions, called structural health monitoring (SHM) (Farrar and Worden, 2010). These two monitoring methods have radically different implications for infrastructure management. SPM aims at using monitoring information to update analytical or numerical models for the reevaluation of structural capacity (Proverbio et al., 2018). Such model updating relies on a well-selected monitoring campaign, both in terms of implemented monitoring techniques and duration of the monitoring (from a few days to a few months). SPM is also been referred to as structural identification in the literature (Catbas et al., 2013). SHM aims to automatically detect, localize, and track the evolution of damage in structures (Farrar and Worden, 2010), improving their maintenance and safety operation (Orcesi and Frangopol, 2011). This monitoring campaign is usually implemented on a permanent basis and may be performed using purely data-driven models (Brownjohn, 2007). Despite the undeniable potential of structural monitoring, these activities are still rarely put into practice by asset managers. Several reasons, such as the lack of time, resources, and awareness of technology (i.e., courses in curricula) (Große et al., 2019), explain this reluctance.
While the conservative assumptions that are typically made in the absence of monitoring information often call for a structural strengthening—if not even a bridge replacement—as they lead to a DoC below 1.0. SPM can relax conservative assumptions by accurate assessment of structural properties. Hence, by leveraging monitoring information, structural interventions may be avoided, thus significantly reducing the cost and environmental impact of infrastructure management (Pai and Smith, 2022). As sustainability and network resilience are becoming major drivers of decision-making, bridge owners are projected to increasingly require the use of data-informed frameworks rather than conservative models for assessments regarding bridge safety. A crucial enabler of this shift relies in the development of frameworks that accurately quantify economic and environmental benefits prior to monitoring (Ye et al., 2022).
SPM provides a measurement-based reevaluation of the DoC, making use of monitoring information to update either the structural behavior or the load levels. To this goal, multiple measurement technologies are available and involve non-destructive testing (NDT), such as rebound hammer and impact echo (Lee et al., 2014), bridge load testing (Lantsoght et al., 2017), continuous bridge-behavior monitoring under normal service conditions (Sawicki and Brühwiler, 2022), and bridge weigh in motion (BWIM) that infers actual traffic load effects (Lydon et al., 2016). Each monitoring technique provides specific information, whose effects on reevaluated DoCs range from little to significant, depending on the types of information collected, the fidelity of the model, and the quality of the data.
This paper presents a short perspective on the information gain for SPM and, thus, is by no means intended as a literature review and rather discusses the usefulness and remaining challenges of monitoring techniques for the code-based assessment of structural performance. Using the example of a composite steel-concrete bridge in Switzerland, this paper discusses the potential of several SPM techniques. SHM applications as well as the influence of environmental effects on structural behavior are not included in the scope of this study. Future needs in terms of research are also highlighted.
The data collected by monitoring techniques for SPM contain information on structural behavior and action effects, thus enabling a data-driven reevaluation of the DoC. The value of measurement data depends on the influence it has on bridge performance indicators: information gain takes place if the updated DoCs of structural verifications differ from the initial evaluations that have been made prior to monitoring. A possible quantification of monitoring value is thus available through the relative variation of the DoC prior to and after monitoring.
Each monitoring provides unique information improving the knowledge of either structural behavior, structural capacity, or action effects by reducing uncertainties on a subset of bridge properties. For a given case study, the optimal monitoring technique (or combination of techniques) depends on the sensitivity of structural verifications to uncertainty reduction, a sensitivity that depends on three main factors: First, it depends on the level of uncertainty pertaining to the monitored bridge, as, for instance, steel structures have much lower magnitudes of material uncertainties than concrete structures. Then, the impact of a given bridge property on the DoC may change depending on the critical limit states; for instance, while material strengths mostly affect ULS verifications, they have no impact for SLS verifications. Finally, the precision of the property identification depends on the monitoring technique, the sensor precision, and the quality of the monitoring systems (i.e., number of sensors and locations). As SPM involves an analytical or numerical model, the fidelity of the model, and the methodology to fuse model predictions with monitoring data further influence the information-gain outcome and need to be accounted for (Pai et al., 2019). Predicting the usefulness of a monitoring technique needs to account for all of these factors.
Existing methodologies to predict the information gain from monitoring systems using information entropy (Papadimitriou, 2004; Bertola et al., 2017) include the above-mentioned factors. Nonetheless, these approaches are based on uncertainty reductions (i.e., bridge-parameter identification) for a given sensor layout rather than the impacts of these uncertainty reductions on the DoC. Moreover, some DoC may be more crucial to decision-making, particularly if they are estimated to be smaller than 1.0 prior to any monitoring, meaning that the structure is deemed unsafe. The information collected on some particular bridge parameters is thus more valuable for decision-making purposes. Recently, efforts have been made to quantify the value of (monitoring) information (VoI) by comparing maintenance intervention with and without including expected information gain (Straub et al., 2017). Although insightful frameworks have been provided, they have been tested on simulated case studies that involve simplified assumptions with respect to maintenance actions, and hypothetical information gain (Kamariotis et al., 2022). In addition, while the above-mentioned works were developed for SHM, frameworks to quantify the VoI for SPM are currently missing. Such frameworks should include costs of potential actions (i.e., structural strengthening), monetarized risk of bridge failure, monitoring costs, and the probability that the monitoring data enables avoiding structural strengthening. The main framework-development challenge lies in estimating this probability.
Predicting the information gain, when multiple monitoring techniques are used, is another challenge. While each technique provides unique information, redundancy exists in the information gain from multiple sources. VoI frameworks for SPM should be able to account for multiple data sources to define the optimal monitoring scheme. Although frameworks predicting the information gain prior to monitoring are scarce, the information gain can be assessed by comparing the DoC before and after monitoring to demonstrate the use case of SPM.
A full-scale bridge in Switzerland is used to illustrate the potential of monitoring information for improving the accuracy of the structural examination. Built in 1959, this bridge is among the first steel-concrete composite bridges (Figure 1). The superstructure comprises two steel box girders and a concrete deck with a width of 12.7 m. The bridge has eight spans between 15.8 and 25.6 m. The structural-behavior and action-effect predictions made using a three-dimensional numerical model are compared with the requirements of the Swiss standards to evaluate the DoCs.
Four monitoring techniques have been employed between 2016 and 2019 to improve the accuracy of structural verifications: 1) a static load test to improve model predictions of the structural behavior (i.e., deck stiffness and Gerber-joint rotation); 2) rebound hammer and sound-velocity measurements to evaluate the concrete-deck properties (concrete strength); 3) a WIM station near the bridge to update the ULS load models and 4) several long-term rebar strain measurements to measure actual stress cycles and update the fatigue predictions. Additional details of the monitoring campaigns and data interpretation are found in Bertola et al. (2022).
The monitoring data is leveraged to update the DoCs with respect to 25 structural verifications (Figure 2). ULS verifications involve the maximum structural capacities of bridge elements, while FLS verifications include evaluating the maximum traffic-induced tensile-stress difference in steel elements and rebars, and SLS verifications evaluate bridge deflections. Each monitoring information leads to a significant improvement in the estimated DoCs, highlighting the reserve capacity of the bridge. However, only a subset of verifications is updated with the information from each monitoring technique: WIM data significantly improve the DoC for ULS verifications (Verifications 1 to 13 in Figure 2); the 3-year strain monitoring updates the FLS critical structural verifications (Verifications 22 and 23); and the SLS verifications (Verifications 24 and 25) are improved with the bridge load testing (Bertola et al., 2022). When combing all monitoring data, the average increase in the DoCs is 36%.
FIGURE 2. Evaluation of the degrees of compliance for structural verifications of ultimate (ULS), fatigue (FLS), and service (SLS) limit states prior to and after monitoring.
All monitoring techniques provide information on a subset of the bridge parameters and thus, influence the DoC of a subset of structural verifications. In this case, the NDTs provide little information as the concrete strength is rarely critical for the ULS verification of RC-steel composite structures. Also, each technique mostly affects verifications for a given limit state. Therefore, the combination of WIM, load testing, and strain measurements provide information with little redundancy. Based on a broad definition of information gain (i.e., influence on DoCs), these three monitoring techniques are recommended as it could influence future maintenance schemes, or if the bridge must be transformed in the future (e.g., change of code, deck widening). Based on the VoI, only the monitoring techniques that could affect deficient structural verifications with a DoC smaller than 1.0 are useful. In the present bridge, only the fatigue limit state is critical (Verifications 22 and 23 in Figure 2), leading to a VoI-based recommendation of continuous strain monitoring to improve decision-making at this point in time.
Initial assessments of structural verifications 22 and 23 indicate that the bridge fails to meet code requirements. This initial structural-safety assessment is corrected as both DoCs exceed 1.0 after monitoring-based updating. Potential asset-management interventions, such as structural strengthening, are thus avoided, leading to a more cost-effective and more sustainable infrastructure management. The use case of SPM to improve infrastructure management is thus demonstrated by the present case study as the (monetary and environmental) cost of monitoring is way below the cost to retrofit a bridge.
The present case study shows the benefits of monitoring activities for infrastructure management. As highlighted in the introduction, neither SPM nor SHM are widely used in practice. A major frontier towards more practical applications of both, SPM and SHM, lies in convincing bridge owners of the benefits of implementing monitoring systems on their infrastructure. Additional frameworks to quantify the VoI from monitoring activities are required to convince decision-makers to implement data-informed approaches for infrastructure management.
In this context, the following topics present cornerstones for future research:
- Evaluating the economic benefits of monitoring techniques before sensing is crucial in convincing decision-makers to perform SPM. Evaluating the VoI of monitoring techniques is challenging, especially when non-linear structural behavior is involved (Bertola et al., 2020), due to the large number of required forward simulations.
- Optimized monitoring systems that are cheap and rapidly deployable must be developed. Selecting the appropriate sensor devices and the optimal sensor configurations will help reduce the monitoring cost without compromising the information gain (Bertola et al., 2019).
- Although each monitoring technique provides unique information, the optimal combination of multiple techniques, such as SPM, SHM, and NDT, into a holistic framework for data-informed asset management has not been investigated. Methodologies to define the monitoring-technique sets that maximize the VoI are needed and must be further improved.
- Full-scale benchmark datasets of short-and-long term monitoring of civil infrastructure are needed to test and validate scientific methods, which—at an initial stage—are typically tested on either simulated data or reduced-scale experimental data under well-controlled environmental conditions.
- Bridge-safety models should be redefined to incorporate data into the decision loop and reduce the reliance on conservative design assumptions. Relying on probabilistic models rather than on deterministic models unfolds the full potential of sensor data.
- Most frameworks to build digital twins only partially use field measurements and are often time-invariant. Digital twins should estimate the influence of the latest sensor data on the evaluations of bridge DoC and include time-dependent evolutions of the compliance factors.
- More studies are needed to evaluate the economic and environmental gains of monitoring for civil-infrastructure management at the network scale.
Moreover, additional education and training for practitioners are required to apply the theoretical frameworks (Bertola et al., 2022). Researchers should therefore advocate for additional courses on assessing existing structures and structural monitoring in current academic curricula. Moreover, researchers have to go to the field and demonstrate themselves the benefits of their theoretical framework to convince bridge owners to implement them on a larger scale.
This perspective shows the potential and challenges of structural performance monitoring for infrastructure management. As the bridge case study showcased, monitoring leads to more accurate evaluations of structural verifications that can change the outcome regarding bridge safety and, eventually, reduce the cost and environmental footprint of our infrastructure networks. Nonetheless, data-informed infrastructure-management decisions require selecting the appropriate set of monitoring techniques, as each non-destructive evaluation provides unique information. Additional research is needed to evaluate the value of information from structural performance monitoring. Significant effort by the research community is required to bring structural performance monitoring to the practice of infrastructure management.
The original contributions presented in the study are included in the article/supplementary material, further inquiries can be directed to the corresponding author.
All authors have conceptualized the manuscript. NB has written the first version. All authors have proofread the manuscript and agree on the final version.
The authors declare that the research was conducted in the absence of any commercial or financial relationships that could be construed as a potential conflict of interest.
All claims expressed in this article are solely those of the authors and do not necessarily represent those of their affiliated organizations, or those of the publisher, the editors and the reviewers. Any product that may be evaluated in this article, or claim that may be made by its manufacturer, is not guaranteed or endorsed by the publisher.
Bertola, N. J., and Brühwiler, E. (2021). Risk-based methodology to assess bridge condition based on visual inspection. Struct. Infrastructure Eng. 0, 1–14. doi:10.1080/15732479.2021.1959621
Bertola, N. J., Cinelli, M., Casset, S., Corrente, S., and Smith, I. F. C. (2019). A multi-criteria decision framework to support measurement-system design for bridge load testing. Adv. Eng. Inf. 39, 186–202. doi:10.1016/j.aei.2019.01.004
Bertola, N. J., Henriques, G., Schumacher, T., and Brühwiler, E. (2022). “Engineering of existing structures: The need and place for non-destructive evaluation,” in Proceeding ofThe International Symposium on Nondestructive Testing in Civil Engineering, Berlin, Germany, 15-17 Sep 2015 (EPFL).
Bertola, N. J., Papadopoulou, M., Vernay, D., and Smith, I. F. C. (2017). Optimal multi-type sensor placement for structural identification by static-load testing. Sensors 17, 2904. doi:10.3390/s17122904
Bertola, N. J., Proverbio, M., and Smith, I. F. C. (2020). Framework to approximate the value of information of bridge load testing for reserve capacity assessment. Front. Built Environ. 6, 65. doi:10.3389/fbuil.2020.00065
Brownjohn, J. M. (2007). Structural health monitoring of civil infrastructure. Philosophical Trans. R. Soc. Lond. A Math. Phys. Eng. Sci. 365, 589–622. doi:10.1098/rsta.2006.1925
Brühwiler, E., Vogel, T., Lang, T., and Lüchinger, P. (2012). Swiss standards for existing structures. Struct. Eng. Int. 22, 275–280. doi:10.2749/101686612x13291382991209
Catbas, F., Kijewski-Correa, T., Lynn, T., and Aktan, A. (2013). Structural identification of constructed systems. Virginia, United States: American Society of Civil Engineers.
Farrar, C. R., and Worden, K. (2010). “An introduction to structural health monitoring,” in New trends in vibration based structural health monitoring (Berlin, Germany: Springer), 1–17.
Feng, M. Q., Kim, D. K., Yi, J.-H., and Chen, Y. (2004). Baseline models for bridge performance monitoring. J. Eng. Mech. 130, 562–569. doi:10.1061/(asce)0733-9399(2004)130:5(562)
Frangopol, D. M., Strauss, A., and Kim, S. (2008). Use of monitoring extreme data for the performance prediction of structures: General approach. Eng. Struct. 30, 3644–3653. doi:10.1016/j.engstruct.2008.06.010
Große, C. U., Arndt, R. W., Mähner, D., Niederleithinger, E., and Taffe, A. (2019). Zerstörungsfreie Prüfung im Bauwesen: Memorandum zur Lehre an deutschsprachigen Hochschulen. Bautechnik 96, 360–368. doi:10.1002/bate.201900008
Kamariotis, A., Chatzi, E., and Straub, D. (2022). Value of information from vibration-based structural health monitoring extracted via Bayesian model updating. Mech. Syst. Signal Process. 166, 108465. doi:10.1016/j.ymssp.2021.108465
Lantsoght, E. O. L., van der Veen, C., de Boer, A., and Hordijk, D. A. (2017). State-of-the-art on load testing of concrete bridges. Eng. Struct. 150, 231–241. doi:10.1016/j.engstruct.2017.07.050
Lee, S., Kalos, N., and Shin, D. H. (2014). Non-destructive testing methods in the U.S. for bridge inspection and maintenance. KSCE J. Civ. Eng. 18, 1322–1331. doi:10.1007/s12205-014-0633-9
Lydon, M., Taylor, S. E., Robinson, D., Mufti, A., and Brien, E. J. O. (2016). Recent developments in bridge weigh in motion (B-WIM). J. Civ. Struct. Health Monit. 6, 69–81. doi:10.1007/s13349-015-0119-6
Orcesi, A. D., and Frangopol, D. M. (2011). Optimization of bridge maintenance strategies based on structural health monitoring information. Struct. Saf. 33, 26–41. doi:10.1016/j.strusafe.2010.05.002
Pai, S. G. S., Reuland, Y., and Smith, I. F. C. (2019). Data-interpretation methodologies for practical asset-management. J. Sens. Actuator Netw. 8, 36. doi:10.3390/jsan8020036
Pai, S. G. S., and Smith, I. F. C. (2022). Methodology maps for model-based sensor-data interpretation to support civil-infrastructure management. Front. Built Environ. 8, 801583. doi:10.3389/fbuil.2022.801583
Papadimitriou, C. (2004). Optimal sensor placement methodology for parametric identification of structural systems. J. sound Vib. 278, 923–947. doi:10.1016/j.jsv.2003.10.063
Proverbio, M., Vernay, D. G., and Smith, I. F. C. (2018). Population-based structural identification for reserve-capacity assessment of existing bridges. J. Civ. Struct. Health Monit. 8, 363–382. doi:10.1007/s13349-018-0283-6
Sawicki, B., and Brühwiler, E. (2022). Quantification of influence of monitoring duration on measured traffic action effects on fatigue of RC deck slabs of road bridges. Struct. Infrastructure Eng. 0, 1442–1456. doi:10.1080/15732479.2022.2059527
Smith, I. F. C. (2016). Studies of sensor data interpretation for asset management of the Built environment. Front. Built Environ. 2, 2–8. doi:10.3389/fbuil.2016.00008
Straub, D., Chatzi, E., Bismut, E., Courage, W., Döhler, M., Faber, M. H., et al. (2017). Value of information: A roadmap to quantifying the benefit of structural health monitoring. Aalborg, Denmark: Aalborg University's.
Keywords: existing bridge, bridge assessment, structural health monitoring, non-destructive evaluation, bridge load testing, structural performance monitoring
Citation: Bertola N, Reuland Y and Brühwiler E (2023) Sensing the structural behavior: A perspective on the usefulness of monitoring information for bridge examination. Front. Built Environ. 8:1045134. doi: 10.3389/fbuil.2022.1045134
Received: 15 September 2022; Accepted: 21 December 2022;
Published: 04 January 2023.
Edited by:
Branko Glisic, Princeton University, United StatesReviewed by:
Vivek Kumar, KLA-Tencor, United StatesCopyright © 2023 Bertola, Reuland and Brühwiler. This is an open-access article distributed under the terms of the Creative Commons Attribution License (CC BY). The use, distribution or reproduction in other forums is permitted, provided the original author(s) and the copyright owner(s) are credited and that the original publication in this journal is cited, in accordance with accepted academic practice. No use, distribution or reproduction is permitted which does not comply with these terms.
*Correspondence: Numa Bertola, bnVtYS5iZXJ0b2xhQGVwZmwuY2g=
Disclaimer: All claims expressed in this article are solely those of the authors and do not necessarily represent those of their affiliated organizations, or those of the publisher, the editors and the reviewers. Any product that may be evaluated in this article or claim that may be made by its manufacturer is not guaranteed or endorsed by the publisher.
Research integrity at Frontiers
Learn more about the work of our research integrity team to safeguard the quality of each article we publish.