- 1Department of Civil and Environmental Engineering, Texas A&M University, College Station, TX, United States
- 2Department of Computer Science and Engineering, Texas A&M University, College Station, TX, United States
- 3Department of Electrical and Computer Engineering, Texas A&M University, College Station, TX, United States
- 4Community Team, Mapbox, Washington, DC, United States
The spread of pandemics such as COVID-19 is strongly linked to human activities. The objective of this article is to specify and examine early indicators of disease spread risk in cities during the initial stages of outbreak based on patterns of human activities obtained from digital trace data. In this study, the Venables distance (
Introduction
The objective of this study is to reveal and evaluate early indicators of human activity during COVID-19 period in cities at the initial stages of the outbreak using measures of human activities derived from digital trace data. As an arguably unprecedented global pandemic, the coronavirus disease 2019 (COVID-19) has infected millions of people worldwide with a mortality rate of 6.6% and a high infection rate (Keni et al., 2020; World Health Organization, 2020). Since the spread of COVID-19 is highly dependent on human activities, incidence of infection could be contained by restricting human activities and mobility (Gollwitzer et al., 2020). Many countries and authorities have implemented various nonpharmaceutical interventions (e.g., shelter-in-place orders, regional lockdowns, and travel restrictions), which were undertaken to slow the spread of disease by disrupting transmission chains through restricting human mobility and activities. Such social distancing and activity reduction interventions have proven to be critical in slowing down the spread of pandemics both in previous epidemics (Caley et al., 2008) and during COVID-19 (Anderson et al., 2020; Tian et al., 2020; Li, et al., 2020b; Ramchandani, et al., 2020).
While reduction in human activities is considered an effective measure for containing epidemics and pandemics, there are limited reliable, proven, real-time leading indicators related to human activities that could provide early insights about the risk of disease spread in a region to inform proactive policy making. One reason for this limitation has been the absence of quantitative measures and data that could be examined to proactively evaluate human activities. With advancements in location intelligence data technologies, however, information derived from cellular devices offers a large depository of digital trace data related to human activities increasingly adapted and analyzed to promote understanding of and to quantify human activity and mobility in pandemic analysis, as well as in other applications (Balcan et al., 2009; Asgari et al., 2013; Barbosa et al., 2018). For example, in the context of COVID-19, the radius of gyration, which captures the mobility of individuals using human movement trajectories, was adopted to analyze the COVID-19 spread in Japan (Yabe et al., 2020). Daily step-counts (gathered from smartphones) were used to estimate and predict decreased movement of individuals within the United States during COVID-19 (Gollwitzer et al., 2020). Two of the most important aspects of human activities during an epidemic are agglomeration of activities and intensity of activities.
Although previous research reveals insights regarding human activities in the context of COVID-19, the relationship between human activities and disease-spreading risk has not been fully explored, and leading indicators of human activities to proactively assess the risk of disease spread during the early stages of pandemics are lacking. The majority of research studies (Chang et al., 2020; Cintia et al., 2020; Gao et al., 2020; Li et al., 2020a) focus on quantifying and analyzing the changes in human activities as a consequence of the outbreak of the virus and in response to protective policies (such as shelter-in-place policies). The time-lag relationship between these human activity metrics and the spread of virus, which can be generally described by the basic reproduction number (
In this study, the empirical data related to human activities during COVID-19 was used to reveal the leading relationship between the human activity and the basic reproduction number. We adopted the Venables distance (
The rest of this article is organized into three sections. The first section discusses the description of the two datasets (Mapbox data and total confirmed cases number data), as well as the analysis methods. The second section describes the results of time-lag correlation analysis between the two metrics and the basic reproduction number. The last section presents the results and the implications of the findings for future work.
Methods
In this section, we describe the two datasets—Mapbox data and total confirmed cases number data—and the procedures for human activity categorization. Also covered in this section are definitions and equations related to the Venables distance (
Data Source and Preprocessing
We utilized digital trace telemetry data obtained from Mapbox from January 1 to March 30, 2020. The dataset contains a metric of telemetry-based human activity,
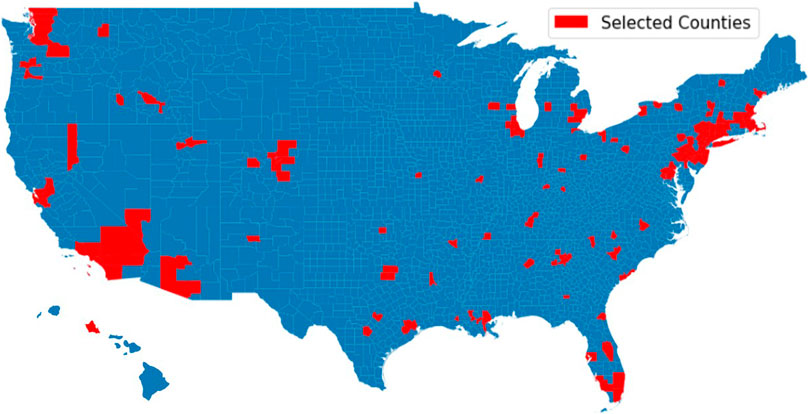
FIGURE 1. 193 selected counties whose cumulative confirmed cases were greater than 100 as of March 31, 2020.
To reveal the time-lag relationship between metrics and spread of the virus, the total number of confirmed cases was used. We obtained the data from the COVID-19 Data Repository by the Center for Systems Science and Engineering (CSSE) at Johns Hopkins University (2020). The data in this repository were gathered and aggregated from various sources, such as the World Health Organization (WHO) and the Centers for Disease Control and Prevention (CDC). We extracted the total number of confirmed cases
Tile Categorization
The nature of an activity might put its participants at a higher risk level for contracting the virus. For example, activities in public common areas, such as grocery stores or gyms, would lead to greater risk of disease spread compared to the activities in residential areas, such as working from home or walking a dog in the community. The fine granularity of the spatial resolution enables classification of each tile into one of the four categories: (1) social tiles, (2) traffic tiles, (3) work tiles, and (4) other tiles. Categorization is based on the following characteristics: (1) social tiles are the location of at least one point of interest location; the location information of point of interest is extracted from SafeGraph data (including restaurant, gas station, and commercial complex) (SafeGraph, 2020); (2) traffic tiles are extracted by mapping the traffic network with all tiles including roads; (3) work tiles are identified based on lack of activity during the late evening hours; and (4) other tiles are located in residential areas. We assigned these four tags to each tile from social, traffic, work to other. Once a tile is assigned with a tag, it is excluded for further categorization. We categorized tiles in this way to examine the importance of human activity in each category and its relationship with the reproduction number. The analysis in this research examines human activities for social, work, and traffic tiles separately. All the residential tiles are excluded from the analysis because the human activities in these tiles have less influence on the contact level among people. Example tile maps related to each category are shown in Figure 2 for Harris County, Texas.
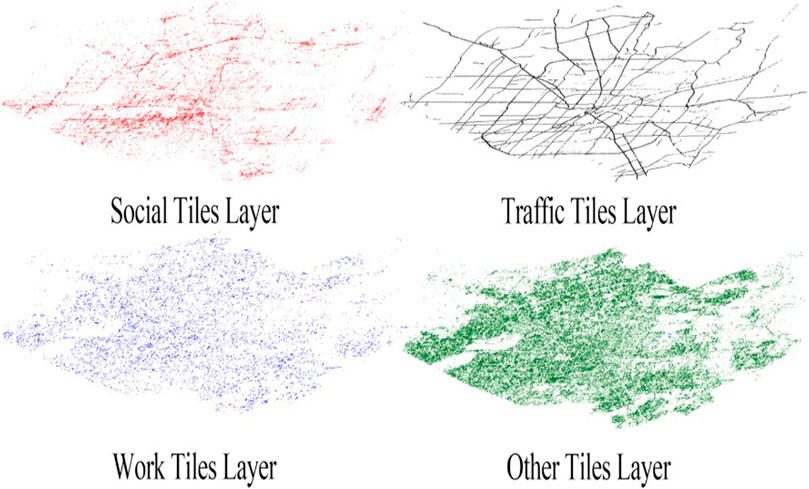
FIGURE 2. Maps of four different tile categories (social, traffic, work, and other) in Harris County, Texas.
Venables Distance
To quantify the agglomeration of human activities, we used the Venables distance (
where
where
where
Activity Density ( )
Although
Basic Reproduction Number Estimation
The basic reproduction number (
where
where
Time Lagged Cross-Correlation Analysis
In the next step, we examined the correlation between the two human activity indicators and the basic reproduction number across all counties. Since these variables are a time series, we used time-lagged cross-correlation analysis to assess the synchrony of time series data sets. The cross-correlation coefficient was calculated using Eq. 7:
where
Results
This section presents the results related to the calculation of the two human activity metrics and their time-lagged correlation with the basic reproduction number across 193 counties during the initial stage of the COVID-19 outbreak in the United States.
Evaluation of Human Activity for Each Category Among Counties
In this study, the Venables distance (
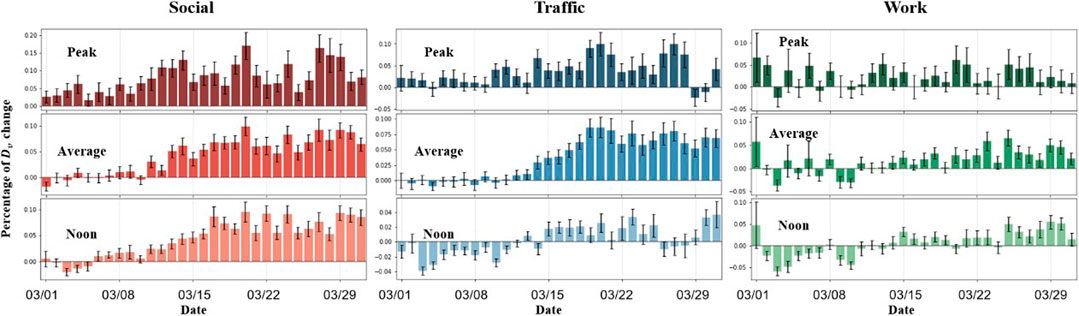
FIGURE 3. The percentage change of
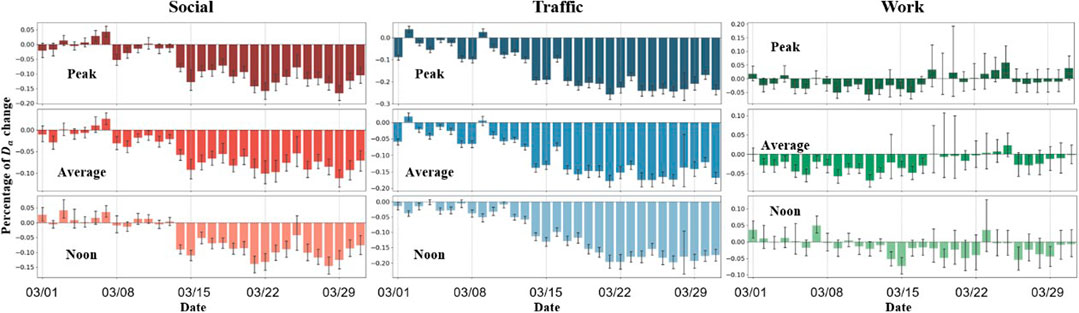
FIGURE 4. The percentage change of
The increasing trend of Venables distance (
Histograms of average percentage change of
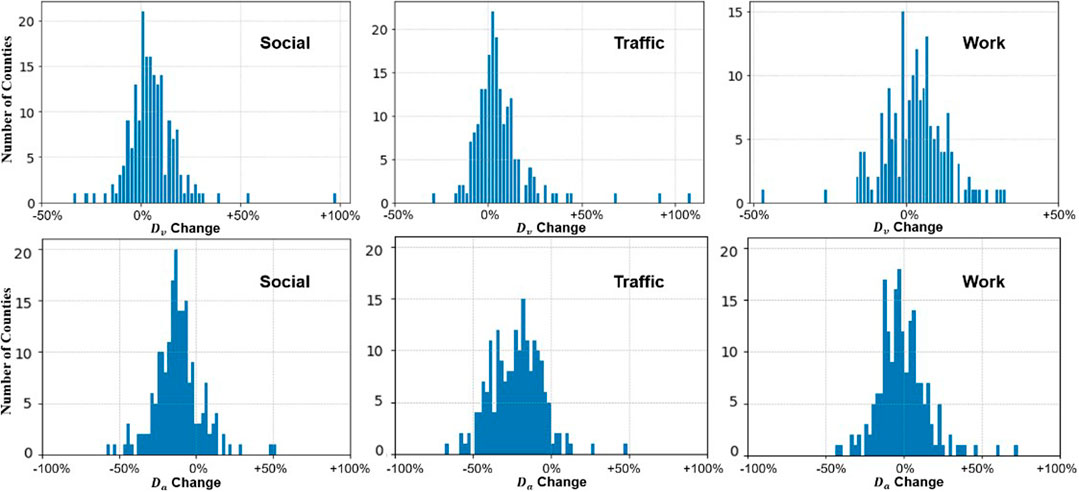
FIGURE 5. Histogram plots of average percentage change of
While
Time-Lagged Correlation Analysis
The spread of the coronavirus is closely related to the human activity patterns. In the previous section, we showed that the average distance between human activities (
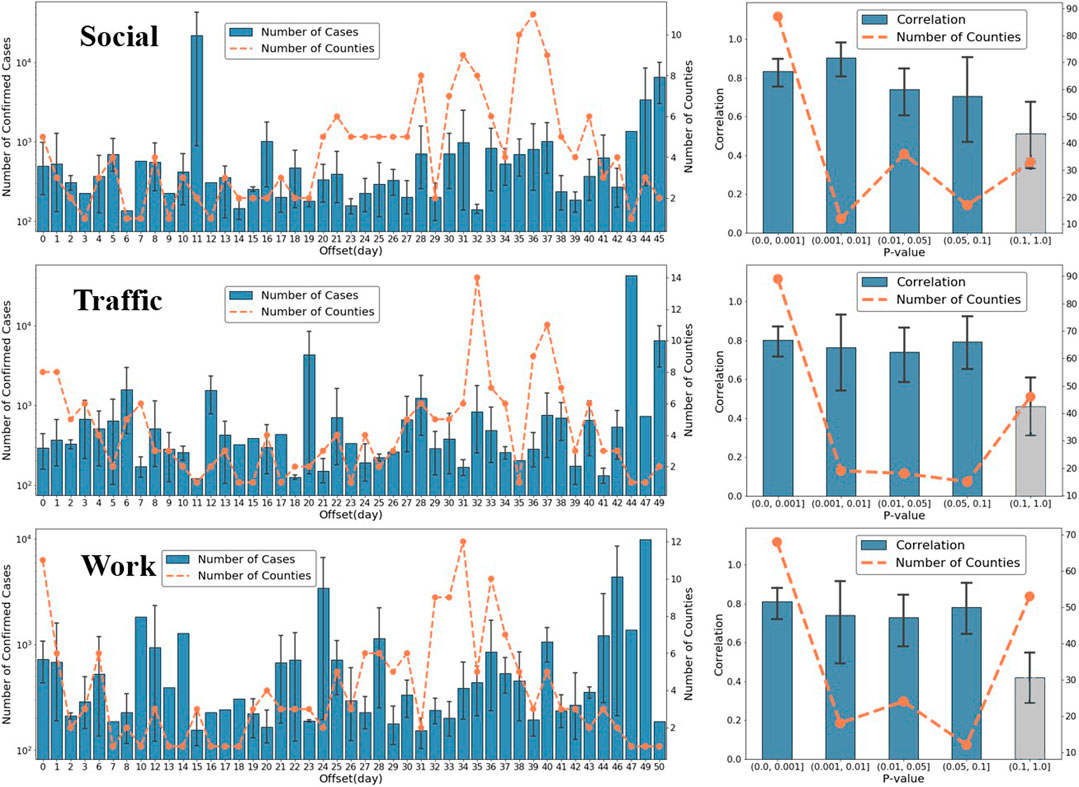
FIGURE 6. The time-lagged correlation analysis between Venables distance (
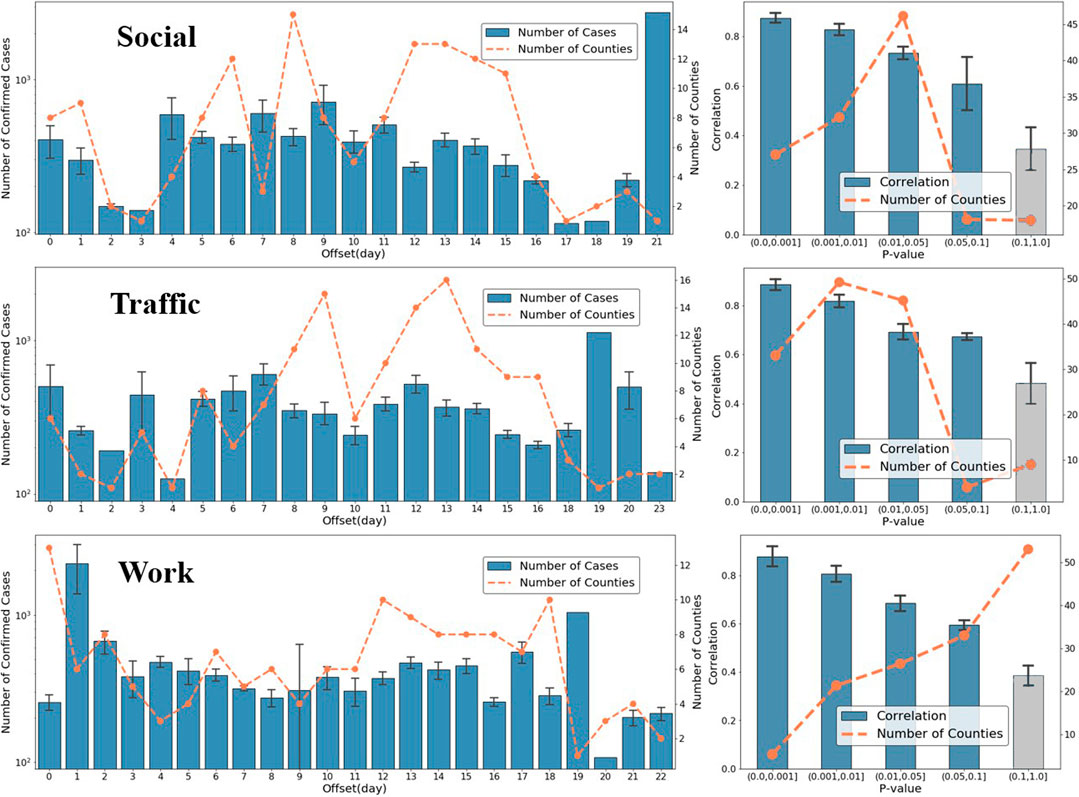
FIGURE 7. The time-lagged correlation analysis result between activity density (
Figure 7 shows the time offset result between activity density (
Heterogeneity for Different County Features
In the next step, we examined the variation of findings across counties with different population sizes, number of confirmed cases, and date of first confirmed cases. The goal is to examine the extent to which the correlation between the two metrics of human activities and the reproduction number is sensitive to these county features. The 193 counties were divided into three uniform categories according to population size and confirmed cases (on March 18, 2020) labeled high, medium, and low. Similarly, the first case dates were labeled as early, mid-range, and late for each one-third of counties. Then, the changes in
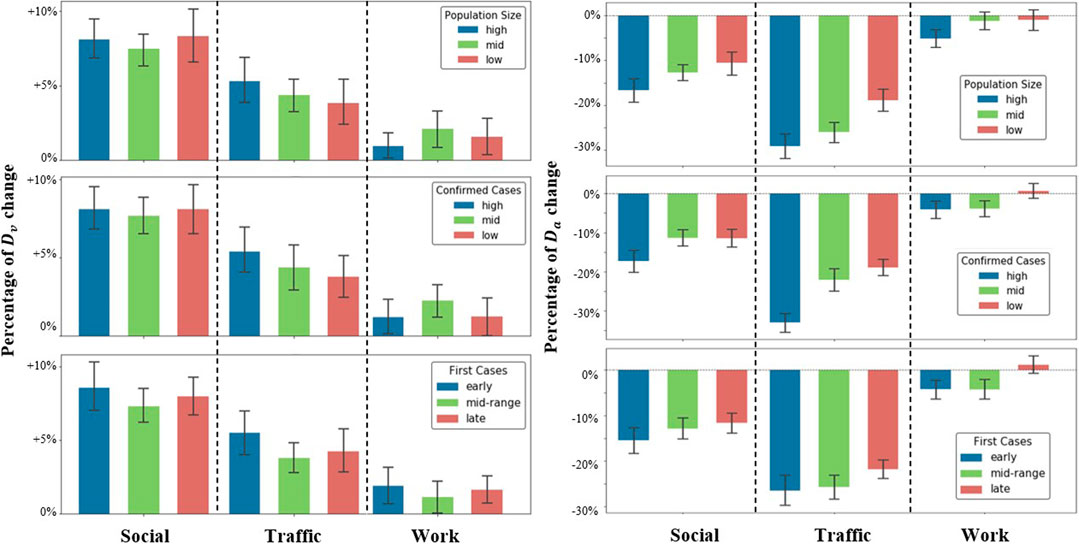
FIGURE 8. Change in Venables distances (
Discussion
This study shows the utility of two human activity metrics [the Venables distance (
There exist other studies that examined human mobility in the context of COVID-19. Wang et al. (2020) examined the similar time-lag effect of human mobility on the COVID-19 infections in the 80 cities most affected in China from Jan 17 to Feb 29. The results showed that the time lag is about 10 days. The index of intracity traffic volume (provided by Baidu) was used to represent the human mobility. Such highly aggregated data, however, may lose some critical spatial information about human activities. Xiong et al. (2020) analyzed mobile device data at each United States county for the COVID-19 period. The origin-destination travel demand and aggregate mobility inflow were used to represent the human activities, and the results showed the dynamics in a positive relationship between human mobility and COVID-19 transmission. Compared with the mentioned similar metrics describing human activities, the two indicators in this article (
This study has some limitations which need to be improved in future studies. First, the tile activity categorization—social, work, and traffic—is not precise. One tile could be labeled as both social and work. In this study and due to data availability limitations, however, we classified tiles into only one of the three categories. Second, the CDC confirmed-cases data had limitations due to testing availability. In this study, we did not adjust the confirmed case data based on the extent of testing in different counties. A lack of testing in some areas resulted in the underestimation of the total cases.
Data Availability Statement
The data analyzed in this study is subject to the following licenses/restrictions: the data that support the findings of this study are available from Mapbox, but restrictions apply to the availability of these data, which were used under license for the current study and so are not publicly available. Requests to access these datasets should be directed to Mikel Maron, bWlrZWxAbWFwYm94LmNvbQ==.
Author Contributions
Research design and conceptualization were carried out by XG, CF, and AM; data collection, processing, analysis, and visualization were performed by XG, CF, YY, SL, and QL; writing was done by XG and AM; reviewing and revising were made by all authors.
Funding
This work was supported by several grants including the United States National Science Foundation RAPID project #2026814, Urban Resilience to Health Emergencies: “Revealing Latent Epidemic Spread Risks from Population Activity Fluctuations and Collective Sense-making,” and Microsoft AI for Health COVID-19 Grant for cloud computing resources.
Conflict of Interest
The authors declare that the research was conducted in the absence of any commercial or financial relationships that could be construed as a potential conflict of interest.
Acknowledgments
The authors would also like to acknowledge that Mapbox provided digital trace telemetry data of human activity and that SafeGraph provided POI data. The authors would like to thank Kieran Gupta, Sofia Heisler, and Ruggero Tacchi from Mapbox for providing technical support.
References
Aleta, A., Martin-Corral, D., y Piontti, A. P., Ajelli, M., Litvinova, M., Chinazzi, M., et al. (2020). Modeling the impact of social distancing, testing, contact tracing and household quarantine on second-wave scenarios of the COVID-19 epidemic. medRxiv [Preprint]. Available at: https://doi.org/10.1101/2020.05.06.20092841 (Accessed May 18, 2020).
Althaus, C. L. (2014). Estimating the reproduction number of Ebola virus (EBOV) during the 2014 outbreak in West Africa. PLoS Curr 6. doi:10.1371/currents.outbreaks.91afb5e0f279e7f29e7056095255b288
Anderson, R. M., Heesterbeek, H., Klinkenberg, D., and Hollingsworth, T. D. (2020). How will country-based mitigation measures influence the course of the COVID-19 epidemic? Lancet 395 (10228), 931–934. doi:10.1016/S0140-6736(20)30567-5
Asgari, F., Gauthier, V., and Becker, M. (2013). A survey on human mobility and its applications. arXiv [Preprint]. Available at: https://arxiv.org/abs/1307.0814 (Accessed Jul 2, 2013).
Balcan, D., Colizza, V., Gonçalves, B., Hu, H., Ramasco, J. J., and Vespignani, A. (2009). Multiscale mobility networks and the spatial spreading of infectious diseases. Proc. Natl. Acad. Sci. United States 106 (51), 21484–21489. doi:10.1073/pnas.0906910106
Barbosa, H., Barthelemy, M., Ghoshal, G., James, C. R., Lenormand, M., Louail, T., et al. (2018). Human mobility: models and applications. Phys. Rep. 734, 1–74. doi:10.1016/j.physrep.2018.01.001
Caley, P., Philp, D. J., and McCracken, K. (2008). Quantifying social distancing arising from pandemic influenza. J. R. Soc. Interface 5 (23), 631–639. doi:10.1098/rsif.2007.1197
Chang, S. Y., Pierson, E., Koh, P. W., Gerardin, J., Redbird, B., Grusky, D., et al. (2020). Mobility network modeling explains higher SARS-CoV-2 infection rates among disadvantaged groups and informs reopening strategies. medRxiv [Preprint]. Available at: https://doi.org/10.1101/2020.06.15.20131979 (Accessed Aug 14, 2020).
Chen, Y. C., Lu, P. E., Chang, C. S., and Liu, T. H. (2020). A Time-dependent SIR model for COVID-19 with undetectable infected persons. IEEE Transactions on Network Science and Engineering 7 4. arXiv [Preprint]. Available at: https://arxiv.org/abs/2003.00122 (Accessed Apr 28, 2020).
Cintia, P., Fadda, D., Giannotti, F., Pappalardo, L., Rossetti, G., Pedreschi, D., et al. (2020). The relationship between human mobility and viral transmissibility during the COVID-19 epidemics in Italy. arXiv [Preprint]. Available at: https://arxiv.org/abs/2006.03141 (Accessed June 4, 2020).
Delamater, P. L., Street, E. J., Leslie, T. F., Yang, Y. T., and Jacobsen, K. H. (2019). Complexity of the basic reproduction number (R0). Emerg. Infect. Dis. 25 (1), 1. doi:10.3201/eid2501.171901
Dietz, K. (1993). The estimation of the basic reproduction number for infectious diseases. Stat. Methods Med. Res. 2 (1), 23–41. doi:10.1177/096228029300200103
Ellison, G. (2020). Implications of heterogeneous SIR models for analyses of COVID-19. Massachusetts, MA: National Bureau of Economic Research, Working paper No. w27373. doi:10.3386/w27373
Fan, C., Lee, S., Yang, Y., Oztekin, B., Li, Q., and Mostafavi, A. (2020). Effects of population co-location reduction on cross-county transmission risk of COVID-19 in the United States. arXiv [Preprint]. Available at: https://arxiv.org/abs/2006.01054. (Accessed Jun 1, 2020).
Gao, S., Rao, J., Kang, Y., Liang, Y., and Kruse, J. (2020). Mapping county-level mobility pattern changes in the United States in response to COVID-19. Sigspatial Special 12 (1), 16–26. doi:10.1145/3404111.3404115
Gatto, M., Bertuzzo, E., Mari, L., Miccoli, S., Carraro, L., Casagrandi, R., et al. (2020). Spread and dynamics of the COVID-19 epidemic in Italy: effects of emergency containment measures. Proc. Natl. Acad. Sci. U.S.A. 117 (19), 10484–10491. doi:10.1073/pnas.2004978117
Giordano, G., Blanchini, F., Bruno, R., Colaneri, P., Di Filippo, A., Di Matteo, A., et al. (2020). Modelling the COVID-19 epidemic and implementation of population-wide interventions in Italy. Nat. Med. 26, 855–860. doi:10.1038/s41591-020-0883-7
Gollwitzer, A., Martel, C., Marshall, J., Höhs, J. M., and Bargh, J. A. (2020). Connecting self-reported social distancing to real-world behavior at the individual and us state level. PsyArXiv [Preprint]. Available at: https://doi.org/10.31234/osf.io/kvnwp.
John Hopkins University (2020). COVID-19 map - Johns Hopkins coronavirus resource center. Available at: https://coronavirus.jhu.edu/map.html (Accessed July 19, 2020).
Keni, R., Alexander, A., Nayak, P. G., Mudgal, J., and Nandakumar, K. (2020). COVID-19: emergence, spread, possible treatments, and global burden. Front Public Health 8, 216. doi:10.3389/fpubh.2020.00216
Lampos, V., Moura, S., Yom-Tov, E., Cox, I. J., McKendry, R., and Edelstein, M. (2020). Tracking COVID-19 using online search. arXiv [Preprint]. Available at: https://arxiv.org/abs/2003.08086. (Accessed Jul 19, 2020).
Li, Q., Bessell, L., Xiao, X., Fan, C., Gao, X., and Mostafavi, A. (2020a). Disparate patterns of movements and visits to points of interests located in Urban hotspots across US metropolitan cities during COVID-19. arXiv preprint [Preprint]. Available at: https://arxiv.org/abs/2006.14157 (Accessed Jun 26, 2020).
Li, Q., Tang, Z., Coleman, N., and Mostafavi, A. (2020b). Detecting early-warning signals in time series of visits to points of interests to examine population response to COVID -19 pandemic. arXiv [Preprint]. Available at: https://arxiv.org/abs/2008.02905 (Accessed Aug 10, 2020).
Liu, Q.-H., Ajelli, M., Aleta, A., Merler, S., Moreno, Y., and Vespignani, A. (2018). Measurability of the epidemic reproduction number in data-driven contact networks. Proc. Natl. Acad. Sci. U.S.A. 115 (50), 12680–12685. doi:10.1073/pnas.1811115115
Louail, T., Lenormand, M., Cantu Ros, O. G., Picornell, M., Herranz, R., Frias-Martinez, E., et al. (2014). From mobile phone data to the spatial structure of cities. Sci. Rep. 4, 5276. doi:10.1038/srep05276
Lu, T., and Reis, B. Y. (2020). Internet search patterns reveal clinical course of disease progression for COVID-19 and predict pandemic spread in 32 countries. medRxiv [Preprint]. Available at: https://doi.org/10.1101/2020.05.01.20087858 (Accessed Sep 16, 2020).
Newman, M. E. (2002). Spread of epidemic disease on networks. Phys. Rev. E—Stat. Nonlinear Soft Matter Phys. 66 (1), 016128. doi:10.1103/PhysRevE.66.016128
Nishiura, H., and Chowell, G. (2009). The effective reproduction number as a prelude to statistical estimation of time-dependent epidemic trends—Mathematical and statistical estimation approaches in epidemiology. Dordrecht, Netherlands: Springer, 103–121.
Ramchandani, A., Fan, C., and Mostafavi, A. (2020). DeepCOVIDNet: an interpretable deep learning model for predictive surveillance of COVID-19 using heterogeneous features and their interactions. IEEE Access 8, 159915–159930. doi:10.1109/ACCESS.2020.3019989
SafeGraph (2020). Safe Graph weekly pattern Version 2. Available at: https://docs.safegraph.com/docs/weekly-patterns (Accessed Nov 4, 2020).
Tian, H., Liu, Y., Li, Y., Wu, C. H., Chen, B., Kraemer, M. U. G., et al. (2020). An investigation of transmission control measures during the first 50 days of the COVID-19 epidemic in China. Science 368 (6491), 638–642. doi:10.1126/science.abb6105
Wang, X., Pei, T., Liu, Q., Song, C., Liu, Y., Chen, X., et al. (2020). Quantifying the time-lag effects of human mobility on the COVID-19 transmission: a multi-city study in China. IEEE Access 8, 216752–216761. doi:10.1109/ACCESS.2020.3038995
World Health Organization (2020). WHO coronavirus disease (COVID-19) dashboard. Available at: https://covid19.who.int (Accessed Jul 19, 2020).
Wu, N., Ben, X., Green, B., Rough, K., Venkatramanan, S., Marathe, M., et al. (2020). Predicting onset of COVID-19 with mobility-augmented SEIR model. medRxiv [Preprint]. Available at: https://www.medrxiv.org/content/10.1101/2020.07.27.20159996v2 (Accessed July 29, 2020).
Xiong, C., Hu, S., Yang, M., Luo, W., and Zhang, L. (2020). Mobile device data reveal the dynamics in a positive relationship between human mobility and COVID-19 infections. Proc. Natl. Acad. Sci. U.S.A. 117 (44), 27087–27089. doi:10.1073/pnas.2010836117
Yabe, T., Tsubouchi, K., Fujiwara, N., Wada, T., Sekimoto, Y., and Ukkusuri, S. V. (2020). Non-compulsory measures sufficiently reduced human mobility in Japan during the COVID-19. arXiv [Preprint]. Available at: https://arxiv.org/abs/2005.09423 (Accessed May 18, 2020).
Zhang, J., Litvinova, M., Liang, Y., Wang, Y., Wang, W., Zhao, S., et al. (2020a). Changes in contact patterns shape the dynamics of the COVID-19 outbreak in China. Science 368 (6498), 1481–1486. doi:10.1126/science.abb8001
Keywords: COVID-19, early indicators, population activities, time lag relationship, Venables distance, activity density
Citation: Gao X, Fan C, Yang Y, Lee S, Li Q, Maron M and Mostafavi A (2021) Early Indicators of Human Activity During COVID-19 Period Using Digital Trace Data of Population Activities. Front. Built Environ. 6:607961. doi: 10.3389/fbuil.2020.607961
Received: 18 September 2020; Accepted: 29 December 2020;
Published: 04 February 2021.
Edited by:
Samiul Hasan, University of Central Florida, United StatesReviewed by:
Yuan Liao, Chalmers University of Technology, SwedenTakahiro Yabe, Purdue University, United States
Copyright © 2021 Gao, Fan, Yang, Lee, Li, Maron and Mostafavi. This is an open-access article distributed under the terms of the Creative Commons Attribution License (CC BY). The use, distribution or reproduction in other forums is permitted, provided the original author(s) and the copyright owner(s) are credited and that the original publication in this journal is cited, in accordance with accepted academic practice. No use, distribution or reproduction is permitted which does not comply with these terms.
*Correspondence: Xinyu Gao, eHkuZ2FvQHRhbXUuZWR1
†These authors have contributed equally to this work