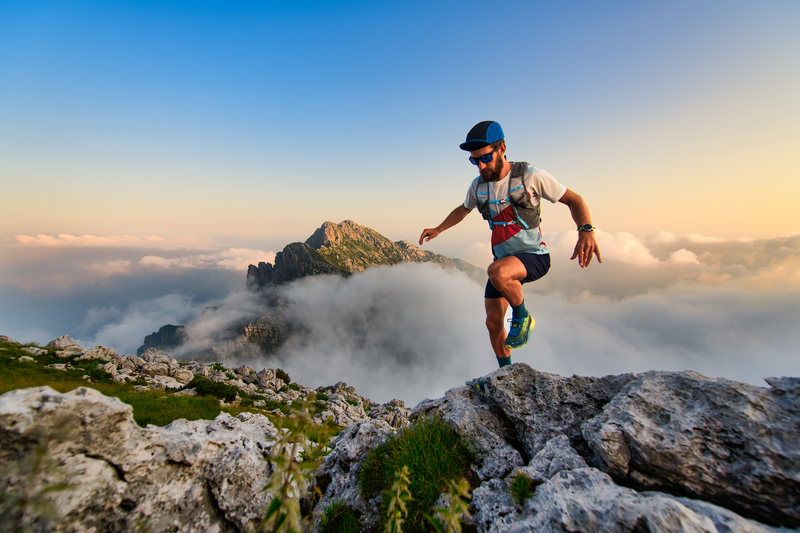
94% of researchers rate our articles as excellent or good
Learn more about the work of our research integrity team to safeguard the quality of each article we publish.
Find out more
ORIGINAL RESEARCH article
Front. Bioinform.
Sec. Integrative Bioinformatics
Volume 5 - 2025 | doi: 10.3389/fbinf.2025.1536783
The final, formatted version of the article will be published soon.
You have multiple emails registered with Frontiers:
Please enter your email address:
If you already have an account, please login
You don't have a Frontiers account ? You can register here
Pancreatic Ductal Adenocarcinoma (PDAC) poses a significant health threat characterized by poor clinical outcomes, largely attributable to late detection, chemotherapy resistance, and the absence of tailored therapies. Despite progress in surgical, radiation, and chemotherapy treatments, 80% of PDAC patients do not benefit optimally from systemic therapy, often due to asymptomatic presentation or disease regression upon diagnosis. The disease's progression is influenced by complex interactions involving immunological, genetic, and environmental factors, among others. However, the precise molecular mechanisms underlying PDAC remain incompletely understood. A major challenge in elucidating PDAC's origins lies in deciphering the genetic variations governing its network. PDAC exhibits heterogeneity, manifesting diverse genetic compositions, cellular attributes, and behaviors across patients and within tumors. This diversity complicates diagnosis, treatment strategies, and prognostication. Identification of "Differentially Expressed Genes" (DEGs) between PDAC and healthy controls is vital for addressing these challenges. These DEGs serve as the foundation for constructing the PDAC protein interaction network, with their network properties being assessed for further insights. Our analysis revealed five key hub genes (KHGs): EGF, SRC, SDC1, ICAM1 and CEACAM5. The KHGs were predominantly enriched in pathways such as: ErbB signaling pathway, Rap1 signaling pathway etc. Acknowledging the therapeutic promise and biomarker importance of PDAC KHGs, we have also pinpointed approved medications for the identified key genes. Nevertheless, it is crucial to conduct experimental validation on KHGs to confirm their effectiveness within the PDAC context. Overall, this study identified potential key hub genes implicated in the progression of PDAC, offering significant guidance for personalized clinical decision-making and molecular-targeted therapy for PDAC patients.
Keywords: PDAC, Differentially Expressed Genes (DEGs), survival analysis, key hub-genes, Gene-interaction network
Received: 29 Nov 2024; Accepted: 03 Mar 2025.
Copyright: © 2025 Bhattacharjee, Sengupta, Kumar and Ghosh. This is an open-access article distributed under the terms of the Creative Commons Attribution License (CC BY). The use, distribution or reproduction in other forums is permitted, provided the original author(s) or licensor are credited and that the original publication in this journal is cited, in accordance with accepted academic practice. No use, distribution or reproduction is permitted which does not comply with these terms.
* Correspondence:
Aryya Ghosh, Ashoka University, Sonepat, India
Disclaimer: All claims expressed in this article are solely those of the authors and do not necessarily represent those of their affiliated organizations, or those of the publisher, the editors and the reviewers. Any product that may be evaluated in this article or claim that may be made by its manufacturer is not guaranteed or endorsed by the publisher.
Research integrity at Frontiers
Learn more about the work of our research integrity team to safeguard the quality of each article we publish.