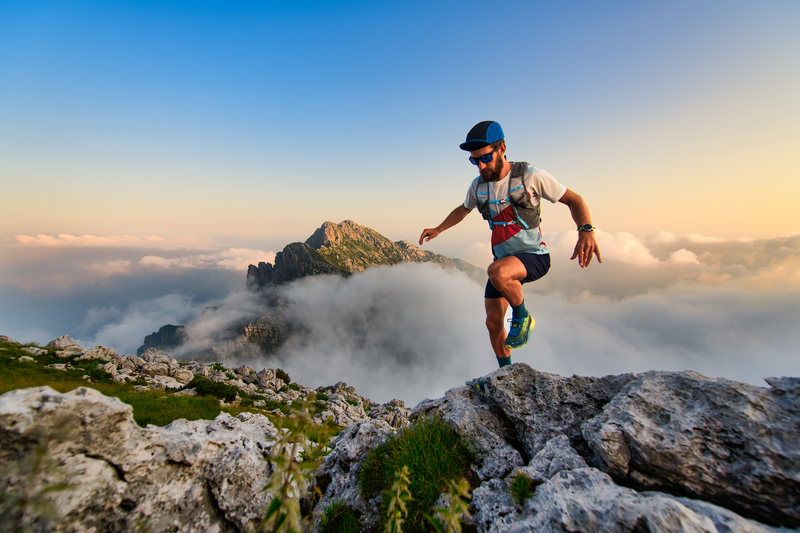
94% of researchers rate our articles as excellent or good
Learn more about the work of our research integrity team to safeguard the quality of each article we publish.
Find out more
ORIGINAL RESEARCH article
Front. Bioeng. Biotechnol.
Sec. Biomechanics
Volume 13 - 2025 | doi: 10.3389/fbioe.2025.1548265
This article is part of the Research Topic Use of Digital Human Modeling for Promoting Health, Care and Well-Being View all 12 articles
The final, formatted version of the article will be published soon.
You have multiple emails registered with Frontiers:
Please enter your email address:
If you already have an account, please login
You don't have a Frontiers account ? You can register here
Existing evaluation criteria for pedestrian head impact injury only focus on single impact, and less attention has been paid to repetitive traumatic brain injury (rTBI), which is common in motor vehicle collisions, falls, and sports.Improving pedestrian collision protection safety requires a complete understanding of the tolerance of the repeated collisions of the human brain to injury. Therefore,this study was to collect data from 72 pedestrian collisions that were reconstructed using MADYMO and THUMS finite element head models(version 4.0.2). The evaluation metrics for rTBI were developed by integrating brain injury criteria based on time-domain features, including the Head Injury Criterion (HIC), Brain Injury Criterion (BrIC), Diffuse Axonal Multi-Axis General Evaluation (DAMAGE), and Maximum Principal Strain (MPS), with frequency-domain features obtained from wavelet packet transform energy analysis of head motion responses.The proposed brain tolerance for mild and severe rTBI was estimated through parametric survival analysis and presented as injury risk curves based on the selected injury metrics. Results showed a significant difference in brain injury tolerance between repetitive and single collisions. For the 50% probability of mild and severe brain injury in real accidents, the thresholds for rTBI metrics based on BrIC and DAMAGE were 1.085 and 1.513, and 0.494 and 0.678, respectively, all higher than the thresholds of single-impact reported in previous studies. However, the thresholds for repetitive head injury criteria based on MPS were 0.604 and 0.838, lower than the thresholds of single-impact reported in previous studies, which implies that the prediction of tolerance to repetitive brain injury is more consistent with tissue kinematics than head kinematics. This study developed injury risk functions (IRFs) for rTBI by integrating the amplitude-frequency characteristics of head responses and brain injury criteria. This knowledge further provides crucial support for understanding the tolerance to rTBI and enhancing pedestrian safety.
Keywords: accident reconstruction, Wavelet packet transform, survival analysis, injury risk function, repetitive traumatic brain injury tolerance
Received: 19 Dec 2024; Accepted: 03 Mar 2025.
Copyright: © 2025 Xiong, Luo, Chen, Shi, Duan, Liu and Li. This is an open-access article distributed under the terms of the Creative Commons Attribution License (CC BY). The use, distribution or reproduction in other forums is permitted, provided the original author(s) or licensor are credited and that the original publication in this journal is cited, in accordance with accepted academic practice. No use, distribution or reproduction is permitted which does not comply with these terms.
* Correspondence:
Shengxiong Liu, School of Pharmacy and Bioengineering, Chongqing University of Technology, Chongqing, China
Kui Li, College of Medical Information, Chongqing Medical University, Chongqing, 400016, China
Disclaimer: All claims expressed in this article are solely those of the authors and do not necessarily represent those of their affiliated organizations, or those of the publisher, the editors and the reviewers. Any product that may be evaluated in this article or claim that may be made by its manufacturer is not guaranteed or endorsed by the publisher.
Research integrity at Frontiers
Learn more about the work of our research integrity team to safeguard the quality of each article we publish.