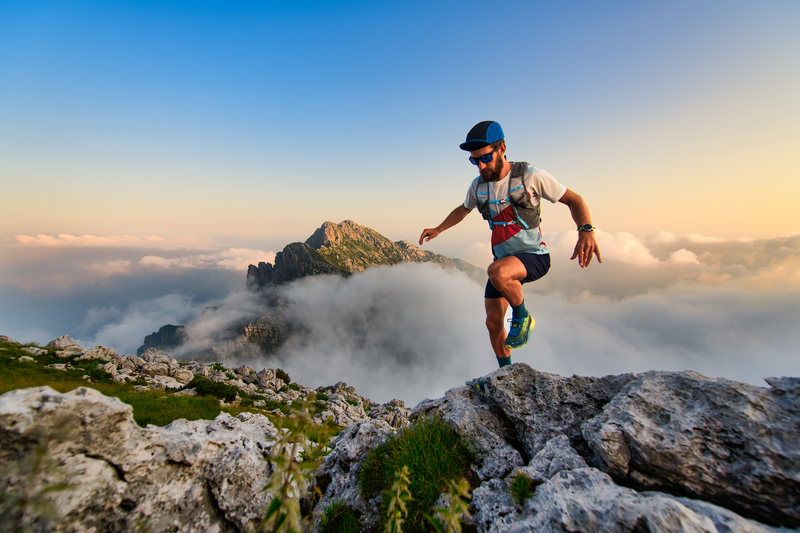
94% of researchers rate our articles as excellent or good
Learn more about the work of our research integrity team to safeguard the quality of each article we publish.
Find out more
ORIGINAL RESEARCH article
Front. Bioeng. Biotechnol.
Sec. Biomechanics
Volume 13 - 2025 | doi: 10.3389/fbioe.2025.1530950
This article is part of the Research Topic Use of Digital Human Modeling for Promoting Health, Care and Well-Being View all 10 articles
The final, formatted version of the article will be published soon.
You have multiple emails registered with Frontiers:
Please enter your email address:
If you already have an account, please login
You don't have a Frontiers account ? You can register here
Accurate prediction of joint torque is critical for preventing injury by providing precise insights into the forces acting on joints during activities. Traditional approaches, including inverse dynamics, EMG-driven neuromusculoskeletal (NMS) models, and standard machine learning methods, typically use surface EMG (sEMG) signals and kinematic data. However, these methods often struggle to reveal the complex, non-linear relationship between muscle activation and joint motion, particularly with complex or unfamiliar movements. The generalization of joint torque estimation models across different individuals faces a significant challenge, as feature transferability tends to decline in higher, task-specific layers, reducing model performance. In this study, we proposed a CNN-GRU-Attention neural network model combining a neuromusculoskeletal (NMS) solver-informed (hybrid-CNN) augmented with transfer learning, designed to predict knee joint torque with higher accuracy. The neural network was trained using EMG signals, joint angles, and muscle forces as inputs to predict knee joint torque in different activities, and the predictive performance of the model was evaluated both within and between subjects.Additionally, we have developed a transfer learning method in the inter-subject model, which improved the accuracy of knee torque prediction by transferring the learning knowledge of previous participants to new participants. Our results showed that the hybrid CNN model can predict knee joint torque within subjects with a significantly lower error (root mean square error ≤ 0.16 Nm/kg). A transfer learning technique was adopted in the inter-subject tests to significantly improve the 2 generalizability with a lower error (root mean square error ≤ 0.14 Nm/kg). The transfer learning-enhanced CNN-GRU-Attention with the NMS model shows great potential in the prediction of knee joint torque.
Keywords: Knee joint torque, Convolutional Neural Network, Transfer Learning, Neuromusculoskeletal model, muscle force
Received: 19 Nov 2024; Accepted: 12 Feb 2025.
Copyright: © 2025 Zhang, Xie, Wang, Liu, Yan and Zeng. This is an open-access article distributed under the terms of the Creative Commons Attribution License (CC BY). The use, distribution or reproduction in other forums is permitted, provided the original author(s) or licensor are credited and that the original publication in this journal is cited, in accordance with accepted academic practice. No use, distribution or reproduction is permitted which does not comply with these terms.
* Correspondence:
Kuan Zhang, School of Biomedical Engineering, Capital Medical University, Beijing, 100069, Beijing Municipality, China
Disclaimer: All claims expressed in this article are solely those of the authors and do not necessarily represent those of their affiliated organizations, or those of the publisher, the editors and the reviewers. Any product that may be evaluated in this article or claim that may be made by its manufacturer is not guaranteed or endorsed by the publisher.
Research integrity at Frontiers
Learn more about the work of our research integrity team to safeguard the quality of each article we publish.