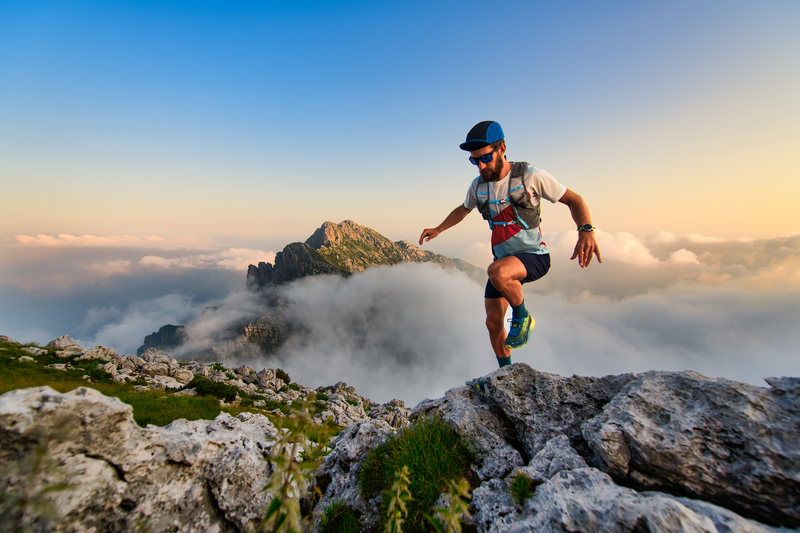
95% of researchers rate our articles as excellent or good
Learn more about the work of our research integrity team to safeguard the quality of each article we publish.
Find out more
ORIGINAL RESEARCH article
Front. Bioeng. Biotechnol. , 04 December 2024
Sec. Bioprocess Engineering
Volume 12 - 2024 | https://doi.org/10.3389/fbioe.2024.1499940
Introduction: H. pylori (Helicobacter pylori) infection represents a significant global health concern, exacerbated by the emergence of drug-resistant strains resulting from conventional antibiotic treatments. Consequently, the development of vaccines with both preventive and therapeutic properties has become crucial in addressing H. pylori infections. The H. pylori adhesin protein HpaA has demonstrated strong immunogenicity across various adjuvants and dosage forms, positioning it as a key candidate antigen for recombinant subunit vaccines against H. pylori. Optimizing fermentation culture conditions is an effective strategy to enhance product yield and lower production costs. However, to date, there has been no systematic investigation into methods for improving the fermentation yield of HpaA. Enhancing the fermentation medium to increase HpaA yield holds significant potential for application and economic benefits in the prevention and detection of H. pylori infection.
Methods: To achieve a stable and high-yielding H. pylori vaccine antigen HpaA, this study constructed recombinant Escherichia coli expressing HpaA. The impact of fermentation medium components on the rHpaA yield was assessed using a one-factor-at-a-time approach alongside Plackett–Burman factorial experiments. Optimal conditions were effectively identified through response surface methodology (RSM) and artificial neural network (ANN) statistical computational models. The antigenicity and immunogenicity of the purified rHpaA were validated through immunization of mice, followed by Western Blot analysis and serum IgG ELISA quantification.
Results: Glucose, yeast extract, yeast peptone, NH4Cl and CaCl2 all contributed to the production of rHpaA, with glucose, yeast extract, and NH4Cl demonstrating particularly significant effects. The artificial neural network linked genetic algorithm (ANN-GA) model exhibited superior predictive accuracy, achieving a rHpaA yield of 0.61 g/L, which represents a 93.2% increase compared to the initial medium. Animal immunization experiments confirmed that rHpaA possesses good antigenicity and immunogenicity.
Discussion: This study pioneers the statistical optimization of culture media to enhance rHpaA production, thereby supporting its large-scale application in H. pylori vaccines. Additionally, it highlights the advantages of the ANN-GA approach in bioprocess optimization.
Helicobacter pylori (H. pylori) is a conditional pathogen that selectively colonizes the human gastric mucosa (Wadström et al., 1996; Amieva and Peek Jr, 2016) and is closely linked to various gastrointestinal diseases (Malfertheiner, 2018; Zhou et al., 2021). H. pylori infection has posed a serious threat to human health for many years (Hooi et al., 2017; Li et al., 2023), with standard treatments involving triple or quadruple therapies comprising two antibiotics alongside proton pump inhibitors (PPIs) or bismuth agents (Malfertheiner et al., 2017). However, these treatments can lead to gastrointestinal microbiota dysbiosis (Karakan et al., 2021) and contribute to the development of antibiotic resistance, resulting in a continuous decline in the effectiveness of antibiotic treatment in infection eradication (Nestegard et al., 2022; Setshedi and Smith, 2023). Thus, there is an urgent need for new strategies to prevent and treat H. pylori infection.
Numerous studies involving the cloning of various genetic clusters or antigen genes for use in genetically engineered vaccines have been performed (Mirzaei et al., 2017; Liu et al., 2020). A pivotal 2015 study published in The Lancet reported a recombinant H. pylori vaccine that significantly reduced the natural infection rate in volunteers, highlighting vaccination as a promising preventive and therapeutic approach against H. pylori infection (Zeng et al., 2015). However, challenges remain, including the low immunogenicity and low production efficiency of antigenic components (Zhang et al., 2022; Yunle et al., 2024). Therefore, the selection and preparation of specific antigens are of great importance.
HpaA, a lipoprotein located on the surface and flagellar sheath of H. pylori, is a primary adhesin that binds to various receptors on gastric epithelial cells, facilitating bacterial colonization and activation and leading to gastric mucosal damage (Valkonen et al., 1997; Martini et al., 2024). HpaA is conserved across different H. pylori strains and is essential for colonization (Carlsohn et al., 2006). Its low homology with other H. pylori proteins and the strong immunogenicity make it a key candidate for vaccine development, as studies have indicated its potential to induce protective immune responses in mice (Lundström et al., 2001). While current studies have focused primarily on the immunological effects of HpaA, there is a need to optimize the production process of recombinant antigens, which is where the development and optimization of fermentation medium become crucial.
The fermentation medium defines the chemical and nutritional environment for host cells during the production of heterologous proteins and directly impacts production efficiency and economic viability (Zhang and Gtrasham, 1999). High-level expression of heterologous proteins may impose a metabolic stress on host cells, affecting their growth efficiency (Goormans et al., 2020). Adjusting the culture conditions can alleviate this stress and optimize the balance between cell growth and protein production. Considering the stringent safety standards required for vaccine components, the use of some molecular tools may increase the complexity of downstream production and purification processes, which is not conducive to industrial-scale production.
Nonetheless, a substantial body of research demonstrated that refining the nutritional parameters within the cultivation process can markedly elevate the efficiency of recombinant protein production (Su et al., 2015; Behravan and Hashemi, 2021). Among them, experimental design methods such as response surface methodology (RSM) and artificial neural network (ANN) have been successfully employed to optimize the expression and yield of recombinant proteins in host cells (Papaneophytou and Kontopidis, 2014). RSM employs polynomial functions to elucidate the interactive effects of input variables, while ANNs, structured to mimic biological neurons, identify nonlinear relationships within data (Pandey et al., 2018). Genetic algorithms (GA), grounded in evolutionary theory, have enhanced the optimization of neural networks, offering greater predictive accuracy than traditional orthogonal methods (Prabhu et al., 2017). Production of the recombinant pneumonia vaccine candidate antigen PsaA was efficiently optimized in Escherichia coli by RSM. The optimized medium and seed culture conditions reduced the processing time by 35% and doubled the productivity to 40 (mg/L)/h, while also decreasing the raw material costs (Larentis et al., 2012). In another study, RSM and ANN were used to predicted the specific growth rate and biomass of recombinant Pichia pastoris during fed-batch methanol fermentation. The feedstock control strategy for intracellular HBsAg production by P. pastoris was optimized, and the yield increased by 26%–31% (Beiroti et al., 2019).
To address the issues of low yield and the lack of systematic optimization of the cultivation conditions, in this study, E. coli BL21(DE3) was engineered to produce rHpaA, and the culture parameters were optimized via various experimental methods and statistical computational models based on RSM and ANN. The optimized production conditions increased the rHpaA yield by 93.2%, providing a reference for large-scale production. Additionally, the immunogenicity of the purified rHpaA was confirmed, and its ability to elicit a robust immune response in mice was demonstrated, underscoring its potential in the prevention and treatment of H. pylori infection.
The gene encoding the HpaA protein was derived from H. pylori 26,695 (GenBank accession number: AE000511.1) and optimized for E. coli codon usage. The hpaA gene, synthesized without a signal peptide by GeneCreate Biotechnology Company (Wuhan, China) was inserted into the NcoI and XhoI sites of the pET28a(+) vector, yielding the recombinant plasmid. The plasmid was transformed into E. coli BL21 (DE3) for protein expression. For long-term preservation, the recombinant bacterial strains were stored at −80°C in a solution containing 8% (v/v) glycerol.
Luria Bertani (LB) medium, composed of 5 g/L yeast extract, 10 g/L tryptone, and 10 g/L NaCl was employed for both strain revival and liquid seed culture preparation. The glycerol stock of the recombinant E. coli BL21 (DE3) harboring the rhpaA/pET28a(+) plasmid was revived on LB agar plates at 37 °C for 16 h. A single colony was selected, inoculated into 10 mL of LB broth containing 100 μg/mL ampicillin, and incubated at 37°C and 220 rpm on a rotary shaker (Mingquan, Shanghai, China) overnight. Subsequently, 1 mL of the seed culture was diluted into 100 mL of induction media at a 1% (v/v) ratio and incubated under the same conditions until the optical density at 600 nm (OD600) reached 0.6–0.8. The expression of the rHpaA protein was induced by the addition of isopropyl-β-D-thiogalactoside (IPTG) to a final concentration of 0.5 mmol/L. After a 4-h induction period, the cells were harvested by centrifugation at 12,000 g for 5 min.
The collected induced cells were suspended in phosphate-buffered saline (PBS) and sonicated. The total protein content of the lysate was quantified using the Lowry method (Lowry et al., 1951). Equal amounts of 10 μg protein were loaded for analysis by 12% sodium dodecyl sulfate‒polyacrylamide gel electrophoresis (SDS‒PAGE). After electrophoresis, the gel was stained with 0.1% Coomassie Brilliant Blue R-250 and visualized with a ChemiDoc™ MP Imaging System (Bio-Rad Laboratories, California, USA). The band areas in the SDS‒PAGE gel were quantified with ImageLab 6.1 software (Bio-Rad Laboratories, California, USA). The rHpaA yield was determined by multiplying the total protein concentration of the sample by the percentage signal intensity of rHpaA.
Traditional Terrific Broth (TB) was selected as the basic culture medium for comparison. To screen effective medium components, the types of carbon source, nitrogen source, metal ions and phosphate in the medium formulation were chosen as variables, and the bacterial biomass yield and recombinant protein expression were considered as the response variables. Glucose, maltose, lactose, galactose, dextrin, sucrose, and soluble starch, each containing an equal amount of carbon content, were used to replace glycerol in the basic medium. Yeast peptone, yeast extract, beef extract, and corn flour, each with equal nitrogen content, were used as individual nitrogen sources to substitute the original mixture of yeast extract and tryptone. Furthermore, the organic nitrogen source in the TB medium was halved and replaced with an equal amount of one of the following inorganic nitrogen sources, ammonium sulfate, ammonium citrate, or ammonium chloride. To determine the effects of different metal ions on the formation of rHpaA, 1 mmol/L of Mg2+, Zn2+, Cu2+, Fe2+, Fe3+, Mn2+, Ni2+, Ca2+, or Co2+ was added to the basic culture medium. Additionally, the phosphate ion concentration was maintained based on the original TB composition, but the type of phosphate was changed from potassium phosphate to sodium phosphate or a mixture of both in equal concentrations. In each single screening experiment, all other factors were held constant. Statistical analysis of the quantitative results was conducted using one-way analysis of variance (ANOVA) with GraphPad Prism 9.5 software (GraphPad Software, San Diego, CA, USA). A p-value of <0.05 was considered to indicate statistical significance.
The Plackett–Burman design was conducted via Design Expert 13 software (Stat-Ease, Inc., Minneapolis, MN, USA) to find key factors significantly affecting rHpaA yield. The design contained six elements selected from one-factor-at-a-time experiments: glucose, NH4Cl, yeast peptone, yeast extract, CaCl2, and mixed phosphate. Each factor was set at two levels, resulting in a matrix of 12 experimental groups (see Supplementary Material) with triplicate replications to ensure the reliability of the results.
The steepest ascent path was utilized to guide the experimental conditions to the near-optimal region. The significant factors identified by the Plackett–Burman experiment, glucose, NH4Cl, and yeast extract, were optimized in steps sizes of 2, 3, and 4 based on the coefficient estimates (Table 1). Meanwhile, the remaining factors were set to their respective extreme levels, specifically with CaCl2 of 1 mmol/L, yeast peptone of 24 g/L, and phosphate buffer of 100 mmol/L. The highest level of rHpaA production reached was considered to be close to the center point of the experimental optimization design.
The BBD-RSM was employed to further optimize and determine the optimal levels of significant variables. Design Expert 13 software was utilized to conduct a three-factor, three-level experimental design. Seventeen sets of experiments, including five center points, were carried out with the highest point of the steepest ascent path as the preset center point, and the yield of rHpaA as the response value (Table 2). The second-order equation was fitted and the regression model was established as shown in Equation 1.
where Y represents the response value; β0, βi and βij are the equation coefficients; and xi and xj are the independent variables. The response optimizer of Design Expert software was used to determine the optimal values of key variables and the maximum yield of rHpaA within the experimental range.
The back propagation method was used to establish the ANN model, with the glucose, yeast extract, and NH4Cl concentrations in the medium as input and the rHpaA yield as the output. The transfer functions tansig and purelin were used for the hidden and output layers, respectively. The trainbr algorithm was employed to train the network. The BBD-RSM experimental data were utilized to train the neural network, with allocation ratios for the training set, test set, and validation setof 70%, 15%, and 15%, respectively. Training was halted when the mean square error (MSE) reached 1 × 10−3. To evaluate the fitting and predictive ability of the model, the root mean square error (RMSE), variance (R2) and standard error of prediction (SEP) were calculated for the established model via Equations 2–4, respectively (Mondal et al., 2023; Patruni and Rao, 2023).
In these formulae, n represents the number of samples, Yi,e represents the experimental value, Yi,p represents the predicted value, and “-” above a variable represents the average value of that variable.
The GA optimization approach was utilized to determine the optimal levels of significant variables in the cultivation process within the ANN model. The rHpaA yield function was employed as the fitness function for the GA, directing the iterative selection process. The program was developed using MATLAB 2023b software (MathWorks, Inc., Natick, MA, USA) along with its integrated GA toolbox.
The scale of the rHpaA/pET28a(+)/E. coli BL21 (DE3) cultures was increased to 1 L under the optimized culture conditions. The cells were harvested and suspended at a ratio of wet cell weight (g) to buffer volume (mL) of 1:20 in ice-cold 50 mmol/L phosphate buffer (PB, pH 7.0) supplemented with 500 mmol/L NaCl, 10 mmol/L imidazole, 100 U of benzonase and 200 mmol/L MgCl2. After high-pressure homogenization, the lysate was centrifuged at 12,000 g for 30 min. The supernatant was collected and applied to a pre-equilibrated Ni-Sepharose affinity column (Cytiva, Marlborough, MA, USA). Proteins were eluted with 50 mmol/L PB, 500 mmol/L NaCl and 100 mmol/L imidazole. The eluate was subsequently diluted 50-fold and subjected to anion-exchange chromatography using a Q-Sepharose column (Cytiva, Marlborough, MA, USA). The bound proteins were eluted with a solution of 20 mmol/L PB containing 150 mmol/L NaCl at pH 7.4 and stored at −80°C.
The purity of the purified rHpaA was assessed via size exclusion chromatography–high-performance liquid chromatography (SEC‒HPLC) on an Agilent Infinity 1260 II system (Santa Clara, CA, USA) with an Xtimate SEC-300A column (300 mm × 7.8 mm; Welch Materials Inc., Shanghai, China). The mobile phase comprised 150 mmol/L PB and 10% acetonitrile, with the flow rate of 1.0 mL/min. The column temperature was maintained at 20°C, and the injection volume was 10 µL. The protein was detected by an UV detector at 280 nm.
Six-week-old, specific-pathogen-free (SPF) female BALB/c mice were randomly divided into a control group and an experimental group, with 20 mice per group. The experimental group received immunization with 50 μg of rHpaA mixed with 1 mg of aluminum adjuvant (Thermo Fisher Scientific, Waltham, MA, USA) in an equal volume via intramuscular injection on days 0, 14, and 21. The control group received an equivalent treatment with PBS. Serum samples were collected from the tail veins of mice 1 week after the final immunization and isolated by centrifugation at 3,000 g for 5 min.
The experimental animals were purchased from Dossy Experimental Animals Co., Ltd. (Chengdu, China) and housed in the experimental animal center of West China Hospital, Sichuan University. All experiments were approved by the institutional ethics committee (number: 20220113002).
The antigenicity of rHpaA was assessed via Western blotting. The purified protein was subjected a 12% SDS-polyacrylamide gel and electrophoretically transferred onto a 0.22 μm pore-sized polyvinylidene fluoride (PVDF) membrane. The membrane was blocked with 5% (w/v) nonfat dry milk in Tris-buffered saline with 0.1% Tween-20 (TBST) for 1 h. After three washes with TBST, the membrane was incubated with either mouse anti-H. pylori lysate serum or anti-rHpaA serum, which was prepared in our laboratory, as the primary antibody for 2 h. Following three additional washes with TBST, the membrane was incubated with horseradish peroxidase (HRP)-conjugated goat anti-mouse IgG (Sangon Biotech, Shanghai, China) as the secondary antibody for 1 h. The SuperFemto ECL Chemiluminescence Kit (Vazyme, Nanjing, China) was used to visualize the immunoreactive bands with a ChemiDoc™ MP Imaging System.
The immunogenicity of rHpaA was evaluated using an enzyme-linked immunosorbent assay (ELISA). The wells of a 96-well ELISA plate were coated with 100 μL purified rHpaA at a concentration of 2 μg/mL and then blocked with 1% (w/v) bovine serum albumin (BSA) for 1 h at 37°C. Serial dilutions of serum samples from immunized mice were added to the wells and incubated for 2 h. Following three washes with PBST, HRP-conjugated goat anti-mouse IgG was added and incubated for 30 min at 37°C. The plate was then washed again, and 100 μL of TMB chromogenic substrate solution was added to each well for 15-min incubation at 37°C. The enzymatic reaction was stopped by adding 50 μL of 2 mol/L sulfuric acid (H2SO4) per well, and the absorbance at 450 nm was measured via a microplate reader (BioTek Instruments, Winooski, VT, USA).
To enhance the yield of rHpaA, one-factor-at-a-time experiments were performed to assess the composition of the basic medium. Understanding the optimal carbon source is crucial, as it serves as the primary energy substrate for microorganisms. The experimental data (Figure 1A) showed that maltose and glucose significantly enhanced rHpaA expression, achieving levels of 44.20% and 44.33%, respectively. These levels were 10.39% and 10.71% higher than the control group. Further evaluation revealed that when glucose was used as the carbon source, resulted in the highest cell density, with an OD600 value of 4.72, which was 1.29-fold higher than that of the control at 3.65. The maximum rHpaA yield reached 0.45 g/L, marking a 1.41-fold increase compared to the control group.
Figure 1. Effects of different medium components on rHpaA production. (A) rHpaA yield, expression rate, and OD600 under different carbon source conditions. (B) rHpaA yield, expression rate, and OD600 under different nitrogen source conditions. (C) rHpaA yield, expression rate, and OD600 under different phosphate and metal ion conditions. *p < 0.05, **p < 0.01, ***p < 0.001.
Upon identifying the optimal carbon source, the focus shifted to the nitrogen source. The experiments (Figure 1B) revealed that the addition of inorganic nitrogen sources effectively increased rHpaA expression, but ammonium citrate inhibited cell growth. The combination of NH4Cl with yeast extract and yeast peptone resulted in the highest OD600 at 3.87 and the protein expression of 47.25%. This mix yielded 0.40 g/L of rHpaA, which was 1.27 times greater than that of the control.
Metal ions are essential for microorganisms, often serving as enzyme cofactors that influence cellular physiological processes. The next step was to investigate these elements for their potential to enhance rHpaA production. Compared to the control, the addition of Mg2+, Zn2+, Cu2+, Ni2+ and Ca2+ boosted rHpaA expression. Notably, only Ca2+ increased biomass simultaneously, resulting in an rHpaA yield of 0.39 g/L, which was 1.31 times greater than that of the control (Figure 1C).
The types of phosphate were also investigated, as they are necessary for electrochemical gradients and cellular signaling. The results indicated that the simultaneous addition of sodium phosphate and potassium phosphate to the culture medium resulted in a higher yield of rHpaA compared to the use of a single phosphate (Figure 1C). In the culture medium containing mixed phosphates, the yield of rHpaA reached 0.38 g/L, which was 1.27 times that of the control group, with an expression level of 46.06% and an OD600 of 3.83.
The Plackett–Burman experimental design is a factorial screening method used to identify key variables from a comprehensive multivariate analysis (Plackett and Burman, 1946; Quinlan and Lin, 2015). On the basis of preliminary one-factor-at-a-time experiments, six medium components were selected as variables, labeled A, B, C, D, E, and F representing glucose, NH4Cl, CaCl2, yeast extract, yeast peptone and mixed phosphate solution, respectively. The response values in the design matrix for the 12 sets of experimental conditions varied from 0.3568 to 0.4575 g/L (see Supplementary Material). Analysis with Design Expert 13 revealed that the model was statistically significant at p < 0.05. Among the factors, glucose (p = 0.0016), NH4Cl (p = 0.0022) and yeast extract (p = 0.0102) had the most significant effects on rHpaA yield, with contributions of 34.3664%, 30.1742% and 14.6369%, respectively (Table 1).
The steepest ascent path is an optimization strategy for multifactorial experiments, designed to incrementally adjust factor levels towards the optimal design center. The coefficients from the Plackett–Burman experiment (Table 1) indicated that NH4Cl positively affecting the production of rHpaA, whereas glucose and yeast extract had negative effects. This implied that to reach the maximum response, the concentration of NH4Cl should be progressively increased, whereas the concentrations of glucose and yeast extract should be decreased. During the second step of the five-step ascent, the yield of rHpaA peaked at 0.585 g/L, with the concentrations of the medium components approaching the optimal solution, at which point the concentrations of glucose, NH4Cl, and yeast paste were 7 g/L, 5 g/L, and 16 g/L, respectively (Table 2).
BBD-RSM was used to simulate the specific effects of the amounts of glucose, NH4Cl and yeast extract added on rHpaA production within the range of values around the peak determined by the steepest ascent path. From the design matrix of the coding variables and rHpaA production under the corresponding conditions (Table 3), the quadratic regression equation as Equation 5 was obtained.
where Y represents the yield of rHpaA (g/L), A represents glucose concentration (g/L), B represents NH4Cl concentration (g/L), and C represents yeast extract concentration (g/L).
The quadratic regression model’s ANOVA (Table 4) reveals a model p-value <0.0001, signifying statistical significance, and a lack of fit p-value >0.05, indicating an adequate fit. Thus, the model is considered both significant and sufficiently robust for the biological data analysis (Duan et al., 2020). The R2 value of 0.9832 suggested a strong correlation between the experimental and predicted response values. The adjusted determination coefficient (adj R2) value was 0.9616, indicating that 96.16% of the variation in rHpaA yield was attributed to the independent variables. From the perspective of these individual variables, yeast extract (C), glucose (A), and NH4Cl (B) all had significant effects on the yield of rHpaA. Additionally, the interaction between AB and AC is significant, and the contour plot is elliptical.
The three-dimensional response surface and contour plots (Figure 2) visually demonstrate the effects of the different variables on the response values in the regression equation. The three sets of variables showed the same response trend: when one variable remained unchanged, the response value initially increased and then decreased as the other two variables increased. This indicated that the yield of rHpaA has a maximum value on the surface. The response optimizer of Design Expert software was utilized to maximize rHpaA yield within a defined range. At glucose, NH4Cl, and yeast extract concentrations of 6.488 g/L, 5.653 g/L, and 14.807 g/L, respectively, the RSM forecasted a peak rHpaA yield of 0.597 g/L. Three parallel experiments were carried out under the optimal conditions to verify the optimized results. The yield of rHpaA was 0.5878 ± 0.0157 g/L (n = 3), which was 88.4% higher than the basic medium.
Figure 2. Response surface and contour plot for rHpaA production. (A) Response surface of NH4Cl and glucose concentrations on rHpaA yield. (B) Contour plot of NH4Cl and glucose concentrations on rHpaA yield. (C) Response surface of yeast extract and glucose concentrations on rHpaA yield. (D) Contour plot of yeast extract and glucose concentrations on rHpaA yield. (E) Response surface of yeast extract and NH4Cl concentrations on rHpaA yield. (F) Contour plot of yeast extract and NH4Cl concentrations on rHpaA yield.
To develop more precise and advanced optimization strategies, the following sections were dedicated to the application of the ANN and GA. The neural network was trained with the data from the BBD, and the number of neurons in the middle layer was determined to be 6 via trial and error process; thus, the optimal topology of the neural network was determined to be 3-6-1. The training performance plot (Figure 3D) and the training state plot (Figure 3B) showed a progressive reduction in model error with each iteration. At the third iteration, the MSE reached its nadir, signifying model stabilization and minimal predictive error. The error histogram (Figure 3C) closely adhered to a normal distribution centered near zero, indicating the absence of systemic prediction biases and high predictive accuracy. The fitting plots for the training, testing, and validation data (Figure 3A) exhibited a high degree of data fitting, with an R2 value of 0.9895, suggesting the robust generalizability of the model. Therefore, the ANN model is capable of accurately predicting the rHpaA yield, demonstrating high precision and low error rates.
Figure 3. ANN model analysis. (A) Data regression plot. (B) Training state plot. (C) Error histogram. (D) Training performance plot.
GA was applied to the aforementioned neural network model for global training, with iterative repetitions to achieve the maximum yield of rHpaA. After 58 rounds of GA optimization, the average fitness value and optimal fitness value of the objective function approached the same value (Figure 4), yielding a theoretical maximum yield of 0.614 g/L, with the optimal medium composition comprising 6.492 g/L glucose, 6.655 g/L NH4Cl, and 14.910 g/L yeast extract. Under these cultivation conditions, the rHpaA yield obtained from three replicate experiments was 0.6123 ± 0.0391 g/L (n = 3), which was 93.2% higher than that of the initial basal medium.
Optimization and prediction through two models have led to the determination of the optimal medium formulation for rHpaA production. To facilitate a direct comparison, parameters for the RSM and ANN models were calculated. Both models exhibited strong fitting capabilities, with R2 values exceeding 0.95. The ANN model demonstrated superior predictive accuracy and data fitting, with RMSE and SEP values of 5.496 × 10−3 and 1.021%, respectively, which are lower than those of the RSM model at 5.66 × 10−2 and 10.49%. More intuitively, the scatter plot of data comparison (Figure 5) clearly showed the predicted results of the ANN model are closer to the experimental values. Under the two optimal culture conditions, the predicted yield from the RSM model differed from the experimental yield by 1.57%, while the predicted yield from the ANN-GA model differed from the actual yield by 0.28%, showing a smaller deviation of the ANN-GA predictions from the experimental values.
The optimal cultivation conditions determined with the ANN-GA model were upscaled, and the cell pellets were harvested and lysed. The rHpaA was expressed in a soluble form in the optimized medium (Figure 6A). Upon lysis, the supernatant was separated, and the target protein was subjected to sequential purification steps involving Ni2+ affinity chromatography and anion-exchange chromatography. The purity and homogeneity of the final rHpaA preparation were assessed by SEC-HPLC, which revealed a distinct peak at a retention time of 9.468 min (Figure 6D), indicating a high degree of purity and uniformity.
Figure 6. Identification and analysis of purified rHpaA. (A) Expression analysis of rHpaA via SDS‒PAGE. Lane M, molecular weight marker. Lane 1; rHpaA in whole bacteria; Lane 2, rHpaA in the supernatant; Lane 3, rHpaA in the precipitate. (B) Western blot analysis of purified rHpaA. Lane M, molecular weight marker; Lane 1, reactivity bands of purified rHpaA with anti-rHpaA serum; Lane 2, blank control for Lane 1; Lane 3, reactivity bands of purified rHpaA with anti-H. pylori lysate; Lane 4, blank control for Lane 3. (C) Serum-specific IgG analysis of the rHpaA-immunized group and the PBS control group. (D) SEC-HPLC analysis of purified rHpaA.
To validate the application value of rHpaA, an immunization study was conducted in mice via intramuscular injection. Western blotting was performed to evaluate the antigenicity of rHpaA, using mouse anti-H. pylori lysate serum or anti-rHpaA serum as the primary antibody. There was a single band at approximately 28 kDa (Figure 6B), revealing both samples specifically reacted with rHpaA. The recombinant antigen can be recognized by the mouse anti-H. pylori lysate serum.
ELISA was conducted to evaluate the immunogenicity of rHpaA. After serial dilution up to 262,144-fold, the levels of specific IgG in the anti-rHpaA serum remained significantly higher than those in the negative control (PBS group), with a statistically significant difference (p < 0.0001) (Figure 6C).
The current study systematically investigated the effects of various culture medium components on rHpaA production by recombinant E. coli for the first time, and the optimal component concentration of the medium was determined by RSM and ANN model optimization. It also explored the purification methods and the fundamental immunological properties of rHpaA.
Different culture medium compositions may exert varying effects on microbial growth and product metabolism. The results of one-factor-at-a-time experiments indicated that utilizing glucose as the carbon source significantly enhanced the expression efficiency of the recombinant E. coli, leading to a marked increase in rHpaA production. The preference for glucose may be attributed to its rapid uptake and metabolism by bacteria, which facilitated more efficient energy conversion (Jeckelmann and Erni, 2020). Furthermore, this study demonstrated that the combined use of inorganic and organic nitrogen sources is more advantageous for rHpaA production compared to the use of a single nitrogen source. Notably, the addition of NH4Cl resulted in a significant increase in rHpaA production. Inorganic nitrogen sources provide more quick-acting nitrogen and reduce the lag phase, thereby promoting rapid strain growth and metabolism (Kuypers et al., 2018). Replacing complex organic nitrogen sources like yeast extract and peptone with inorganic nitrogen sources also helps control production costs for economic feasibility (Terol et al., 2019). The addition of Ca2+ and the use of a mixed phosphate significantly enhanced the production of rHpaA. Ca2+ can affect ribosome function and protein folding (Ohkuri et al., 2002). The phosphate mixture can supply both K+ and Na+, providing greater stability in maintaining ion balance and osmotic pressure across the cell membrane, thereby better supporting the transport of nutrients into cells (Stautz et al., 2021; Szatmári et al., 2020).
Microbial metabolite synthesis critically depends on the nutritional environment, with different culture media causing significant changes in protein expression, primarily due to variations in resource allocation (Goormans et al., 2020). Considering the interaction and complex effects of multiple factors in the engineered E. coli culture medium, multi-factor optimization methods were used to avoid deviation from the results of one-dimensional studies. Plackett–Burman experimental analysis identified glucose, yeast extract, and NH4Cl as the most significant variables, which are essential carbon and nitrogen sources that greatly influence rHpaA as direct energy substrates. The steepest ascent design further determined the value range of the central composite design, promoting the transition from preliminary variable screening to a more detailed optimization process, and prepared for subsequent precise adjustments (Sztendur and Diamond, 2002). The RSM model established a quadratic regression equation relating actual variables to rHpaA yield, with a three-dimensional plot highlighting significant interactions between glucose and yeast extract, as well as glucose and NH4Cl (Jankovic et al., 2021). Utilizing RSM data, the ANN model further dissected the implicit nonlinear relationships of input variables on rHpaA yield. With GA optimization, a definitive formula for the culture medium that significantly enhances rHpaA production was derived. Concurrently, the ANN-GA model exhibited superior regression accuracy in both the prediction and optimization process (Prabhu et al., 2017). The limitation of BBD-RSM is its capacity to construct only polynomial regression models, which restricts its generalization ability when addressing complex data sets (Hamza et al., 2024). In contrast, artificial neural networks possess a nonlinear activation function that effectively captures intricate nonlinear relationships within the data, thereby providing enhanced data processing and generalization capabilities (Liu et al., 2022). This makes neural networks more suitable for guiding the optimization process of biologics manufacturing.
The structure and immunogenicity of rHpaA were critical factors influencing the protective efficacy of the vaccine. SEC-HPLC results indicated that rHpaA, following two-step purification, exhibited high purity and homogeneity. The recombinant antigen was recognized by mouse anti-H.pylori lysate serum, demonstrating that the rHpaA possessed antigenicity comparable to that of the natural HpaA from H. pylori (Lundström et al., 2003). The quantitative analyses of specific IgG in the serum of immunized mice revealed that the rHpaA antigen displayed high immunogenicity and effectively induced specific IgG responses, thereby confirming its potential for vaccine application (Banga Ndzouboukou et al., 2021).
In the development of H. pylori vaccines, HpaA is widely regarded as one of the most promising antigens. While most previous studies have concentrated on the immune effects and mechanisms of HpaA, there has been less focus on its engineering applications. To achieve large-scale application in the vaccine field, it is crucial to systematically enhance the antigen production yield. The optimization of fermentation conditions is a key strategy to significantly improve both product yield and quality. This study successfully increased rHpaA production through a series of experiments and model design methodologies, establishing a foundation for the future commercialization of H. pylori vaccines composed of rHpaA. Despite these positive findings, it is important to acknowledge the limitations of this study, which primarily focused on optimizing the composition of the culture medium. Future research must tackle the challenge of refining a broader range of culture conditions during scale-up fermentation to ensure improved scalability and reproducibility of the production method.
This study applied a systematic optimization strategy for the culture medium, in conjunction with RSM and ANN, leading to a significant enhancement in the production of rHpaA. The obtained rHpaA exhibited robust immunogenicity in animal models, providing a viable solution for its large-scale production in H. pylori vaccines. The ANN-GA model demonstrated superior accuracy in prediction and optimization, underscoring its broad utility in biotechnology.
The original contributions presented in the study are included in the article/Supplementary Material, further inquiries can be directed to the corresponding authors.
The animal study was approved by The Animal Ethical and Experimental Committee of West China Hospital, Sichuan University. The study was conducted in accordance with the local legislation and institutional requirements.
RT: Conceptualization, Data curation, Software, Writing–original draft, Writing–review and editing. SZ: Investigation, Validation, Writing–review and editing. MS: Formal Analysis, Methodology, Writing–review and editing. YL: Resources, Validation, Writing–review and editing. XN: Methodology, Writing–review and editing. JH: Data curation, Visualization, Writing–review and editing. GG: Project administration, Resources, Supervision, Writing–review and editing. KL: Funding acquisition, Project administration, Supervision, Writing–review and editing.
The author(s) declare that financial support was received for the research, authorship, and/or publication of this article. This work was supported by the 1.3.5 project for disciplines of excellence, West China Hospital, Sichuan University (No. ZYXY21003).
The authors declare that the research was conducted in the absence of any commercial or financial relationships that could be construed as a potential conflict of interest.
All claims expressed in this article are solely those of the authors and do not necessarily represent those of their affiliated organizations, or those of the publisher, the editors and the reviewers. Any product that may be evaluated in this article, or claim that may be made by its manufacturer, is not guaranteed or endorsed by the publisher.
The Supplementary Material for this article can be found online at: https://www.frontiersin.org/articles/10.3389/fbioe.2024.1499940/full#supplementary-material
Amieva, M., and Peek Jr, R. M. (2016). Pathobiology of Helicobacter pylori-induced. Gastric Cancer 150, 64–78. doi:10.1053/j.gastro.2015.09.004
Banga Ndzouboukou, J., Lei, Q., Ullah, N., Zhang, Y., Hao, L., and Fan, X. (2021). Helicobacter pylori adhesins: HpaA a potential antigen in experimental vaccines for H. pylori. Helicobacter 26, e12758. doi:10.1111/hel.12758
Behravan, A., and Hashemi, A. (2021). RSM-Based model to predict optimum fermentation conditions for soluble expression of the antibody fragment derived from 4D5MOC-B humanized mab in SHuffleTM T7 E. coli. Iran. J. Pharm. Res. 20, 254–266. doi:10.22037/ijpr.2020.114377.14822
Beiroti, A., Hosseini, S. N., Aghasadeghi, M. R., and Norouzian, D. (2019). Comparative study of μ-stat methanol feeding control in fed-batch fermentation of Pichia pastoris producing HBsAg: an open-loop control versus recurrent artificial neural network-based feedback control. J. Chem. Technol. Biotechnol. 94, 3924–3931. doi:10.1002/jctb.6192
Carlsohn, E., Nyström, J., Bölin, I., Nilsson, C. L., and Svennerholm, A.-M. (2006). HpaA is essential for Helicobacter pylori colonization in mice. Infect. Immun. 74, 920–926. doi:10.1128/IAI.74.2.920-926.2006
Duan, M., Wang, Y., Yang, G., Li, J., Wan, Y., Deng, Y., et al. (2020). High-level production of γ-cyclodextrin glycosyltransferase in recombinant Escherichia coli BL21 (DE3): culture medium optimization, enzymatic properties characterization, and product specificity analysis. Ann. Microbiol. 70, 70. doi:10.1186/s13213-020-01610-8
Goormans, A. R., Snoeck, N., Decadt, H., Vermeulen, K., Peters, G., Coussement, P., et al. (2020). Comprehensive study on Escherichia coli genomic expression: does position really matter? Metab. Eng. 62, 10–19. doi:10.1016/j.ymben.2020.07.007
Hamza, A., Khalad, A., and Kumar, D. S. (2024). Enhanced production of mycelium biomass and exopolysaccharides of Pleurotus ostreatus by integrating response surface methodology and artificial neural network Bioresour. Technol. 399, 130577. doi:10.1016/j.biortech.2024.130577
Hooi, J. K. Y., Lai, W. Y., Ng, W. K., Suen, M. M. Y., Underwood, F. E., Tanyingoh, D., et al. (2017). Global prevalence of Helicobacter pylori infection: systematic review and meta-analysis. Gastroenterology 153, 420–429. doi:10.1053/j.gastro.2017.04.022
Jankovic, A., Chaudhary, G., and Goia, F. (2021). Designing the design of experiments (DOE) – an investigation on the influence of different factorial designs on the characterization of complex systems. Energy Build. 250, 111298. doi:10.1016/j.enbuild.2021.111298
Jeckelmann, J.-M., and Erni, B. (2020). Transporters of glucose and other carbohydrates in bacteria. Pflüg. Arch. - Eur. J. Physiol. 472, 11291153. doi:10.1007/s00424-020-02379-0
Karakan, T., Ozkul, C., Akkol, E. K., Bilici, S., Sobarzo-Sánchez, E., and Capasso, R. (2021). Gut-brain-microbiota Axis: antibiotics and functional gastrointestinal disorders. Nutrients 13, 389. doi:10.3390/nu13020389
Kuypers, M. M. M., Marchant, H. K., and Kartal, B. (2018). The microbial nitrogen-cycling network. Nat. Rev. Microbiol. 16, 263276. doi:10.1038/nrmicro.2018.9
Larentis, A. L., Argondizzo, A. P. C., Galler, R., Rodrigues, M. I., and Medeiros, M. A. (2012). Optimization of medium formulation and seed conditions for expression of mature PsaA (pneumococcal surface adhesin A) in Escherichia coli using a sequential experimental design strategy and response surface methodology. J. Ind. Microbiol. Biotechnol. 39, 897–908. doi:10.1007/s10295-012-1099-8
Li, Y., Choi, H., Leung, K., Jiang, F., Graham, D. Y., and Leung, W. K. (2023). Global prevalence of Helicobacter pylori infection between 1980 and 2022: a systematic review and meta-analysis. Lancet Gastroenterol. Hepatol. 8, 553–564. doi:10.1016/S2468-1253(23)00070-5
Liu, M., Zhong, Y., Chen, J., Liu, Y., Tang, C., Wang, X., et al. (2020). Oral immunization of mice with a multivalent therapeutic subunit vaccine protects against Helicobacter pylori infection. Vaccine 38, 3031–3041. doi:10.1016/j.vaccine.2020.02.036
Liu, Y., Gong, H., Shi, C., Yuan, H., Zuo, X., Chang, Y., et al. (2022). Modeling and optimization of the hydrolysis and acidification via liquid fraction of digestate from corn straw by response surface methodology and artificial neural network. J. Clean. Prod. 361, 132241. doi:10.1016/j.jclepro.2022.132241
Lowry, O. H., Rosebrough, N. J., Farr, A. L., and Randall, R. J. (1951). Protein measurement with the Folin phenol reagent. J. Biol. Chem. 193, 265–275. doi:10.1016/S0021-9258(19)52451-6
Lundström, A. M., Blom, K., Sundaeus, V., and Bölin, I. (2001). HpaA shows variable surface localization but the gene expression is similar in different Helicobacter pylori strains. Microb. Pathog. 31, 243–253. doi:10.1006/mpat.2001.0466
Lundström, A. M., Bölin, I., Byström, M., and Nyström, S. (2003). Recombinant HpaA purified from Escherichia coli has biological properties similar to those of native Helicobacter pylori HpaA. APMIS 111, 38997. doi:10.1034/j.1600-0463.2003.t01-1-1110203.x
Malfertheiner, P. (2018). Helicobacter pylori treatment for gastric cancer prevention. N. Engl. J. Med. 378, 1154–1156. doi:10.1056/NEJMe1800147
Malfertheiner, P., Megraud, F., O’Morain, C. A., Gisbert, J. P., Kuipers, E. J., Axon, A. T., et al. (2017). Management of Helicobacter pylori infection—the maastricht V/florence consensus report. Gut 66, 6–30. doi:10.1136/gutjnl-2016-312288
Martini, C., Araba, V., Beniani, M., Armoa Ortiz, P., Simmons, M., Chalbi, M., et al. (2024). Unraveling the crystal structure of the HpaA adhesin: insights into cell adhesion function and epitope localization of a Helicobacter pylori vaccine candidate. mBio 15, e0295223. doi:10.1128/mbio.02952-23
Mirzaei, N., Poursina, F., Moghim, S., Rashidi, N., and Ghasemian Safaei, H. (2017). The study of H. pylori putative candidate factors for single- and multi-component vaccine development. Crit. Rev. Microbiol. 43, 631–650. doi:10.1080/1040841X.2017.1291578
Mondal, P. P., Galodha, A., Verma, V. K., Singh, V., Show, P. L., Awasthi, M. K., et al. (2023). Review on machine learning-based bioprocess optimization, monitoring, and control systems. Bioresour. Technol. 370, 128523. doi:10.1016/j.biortech.2022.128523
Nestegard, O., Moayeri, B., Halvorsen, F.-A., Tønnesen, T., Sørbye, S. W., Paulssen, E., et al. (2022). Helicobacter pylori resistance to antibiotics before and after treatment: incidence of eradication failure. PLOS ONE 17, e0265322. doi:10.1371/journal.pone.0265322
Ohkuri, T., Ueda, T., Yoshida, Y., Abe, Y., Hamasaki, N., and Imoto, T. (2002). A metal binding in the polypeptide chain improves the folding efficiency of a denatured and reduced protein. Biopolymers 64, 106114. doi:10.1002/bip.10153
Pandey, R., Kumar, N., Prabhu, A. A., and Veeranki, V. D. (2018). Application of medium optimization tools for improving recombinant human Interferon gamma production from Kluyveromyces lactis. Prep. Biochem. Biotechnol. 48, 279–287. doi:10.1080/10826068.2018.1425714
Papaneophytou, C. P., and Kontopidis, G. (2014). Statistical approaches to maximize recombinant protein expression in Escherichia coli: a general review. Protein Expr. Purif. 94, 22–32. doi:10.1016/j.pep.2013.10.016
Patruni, K., and Rao, P. S. (2023). Viscoelastic behaviour, sensitivity analysis and process optimization of aloe Vera/HM pectin mix gels: an investigation using RSM and ANN and its application to food gel formulation. LWT 176, 114564. doi:10.1016/j.lwt.2023.114564
Plackett, R. L., and Burman, J. P. (1946). The design of optimum multifactorial experiments. Biom. Trust 33, 305325. doi:10.2307/2332195
Prabhu, A. A., Mandal, B., and Veeranki, V. D. (2017). Medium optimization for high yield production of extracellular human interferon-γ from Pichia pastoris: a statistical optimization and neural network-based approach. Korean J. Chem. Eng. 34, 1109–1121. doi:10.1007/s11814-016-0358-1
Setshedi, M., and Smith, S. I. (2023). Helicobacter pylori infection: antibiotic resistance and solutions for effective management in africa. Antibiotics 12, 969. doi:10.3390/antibiotics12060969
Stautz, J., Hellmich, Y., Fuss, M. F., Silberberg, J. M., Devlin, J. R., Stockbridge, R. B., et al. (2021). Molecular mechanisms for bacterial potassium homeostasis. J. Mol. Biol. 433, 166968. doi:10.1016/j.jmb.2021.166968
Su, L., Huang, Y., and Wu, J. (2015). Enhanced production of recombinant Escherichia coli glutamate decarboxylase through optimization of induction strategy and addition of pyridoxine. Bioresour. Technol. 198, 63–69. doi:10.1016/j.biortech.2015.08.153
Szatmári, D., Sárkány, P., Kocsis, B., Nagy, T., Miseta, A., Barkó, S., et al. (2020). Intracellular ion concentrations and cation-dependent remodelling of bacterial MreB assemblies. Sci. Rep. 10, 12002. doi:10.1038/s41598-020-68960-w
Sztendur, E. M., and Diamond, N. T. (2002). Extensions to confidence region calculations for the path of steepest ascent. J. Qual. Technol. 34, 289296. doi:10.1080/00224065.2002.11980159
Terol, G. L., Gallego-Jara, J., Martínez, R. A. S., Díaz, M. C., and Puente, T. de D. (2019). Engineering protein production by rationally choosing a carbon and nitrogen source using E. coli BL21 acetate metabolism knockout strains. Microb. Cell Factories 18, 151. doi:10.1186/s12934-019-1202-1
Valkonen, K. H., Wadström, T., and Moran, A. P. (1997). Identification of the N-acetylneuraminyllactose-specific laminin-binding protein of Helicobacter pylori. Infect. Immun. 65, 916–923. doi:10.1128/iai.65.3.916-923.1997
Wadström, T., Hirmo, S., and Borén, T. (1996). Biochemical aspects of Helicobacter pylori colonization of the human gastric mucosa. Aliment. Pharmacol. Ther. 10, 17–27. doi:10.1046/j.1365-2036.1996.22164002.x
Yunle, K., Tong, W., Jiyang, L., and Guojun, W. (2024). Advances in Helicobacter pylori vaccine research: from candidate antigens to adjuvants—a review. Helicobacter 29, e13034. doi:10.1111/hel.13034
Zeng, M., Mao, X.-H., Li, J.-X., Tong, W.-D., Wang, B., Zhang, Y.-J., et al. (2015). Efficacy, safety, and immunogenicity of an oral recombinant Helicobacter pylori vaccine in children in China: a randomised, double-blind, placebo-controlled, phase 3 trial. Lancet 386, 1457–1464. doi:10.1016/S0140-6736(15)60310-5
Zhang, J., and Gtrasham, R. (1999). Chemically defined media for commercial fermentations. Appl. Microbiol. Biotechnol. 51, 407–421. doi:10.1007/s002530051411
Zhang, Y., Li, X., Shan, B., Zhang, H., and Zhao, L. (2022). Perspectives from recent advances of Helicobacter pylori vaccines research. Helicobacter 27, e12926. doi:10.1111/hel.12926
Keywords: Helicobacter pylori, artificial neural network, response surface methodology, recombinant antigen, rHpaA
Citation: Tan R, Zhou S, Sun M, Liu Y, Ni X, He J, Guo G and Liu K (2024) Modeling and optimization of culture media for recombinant Helicobacter pylori vaccine antigen HpaA. Front. Bioeng. Biotechnol. 12:1499940. doi: 10.3389/fbioe.2024.1499940
Received: 22 September 2024; Accepted: 20 November 2024;
Published: 04 December 2024.
Edited by:
Xupeng Cao, Chinese Academy of Sciences (CAS), ChinaReviewed by:
Ashish A Prabhu, National Institute of Technology Warangal, IndiaCopyright © 2024 Tan, Zhou, Sun, Liu, Ni, He, Guo and Liu. This is an open-access article distributed under the terms of the Creative Commons Attribution License (CC BY). The use, distribution or reproduction in other forums is permitted, provided the original author(s) and the copyright owner(s) are credited and that the original publication in this journal is cited, in accordance with accepted academic practice. No use, distribution or reproduction is permitted which does not comply with these terms.
*Correspondence: Gang Guo, Z3VvZ2FuZzcwMDFAMTYzLmNvbQ==; Kaiyun Liu, bGl1a2FpeXVuQHdjaHNjdS5lZHUuY24=
Disclaimer: All claims expressed in this article are solely those of the authors and do not necessarily represent those of their affiliated organizations, or those of the publisher, the editors and the reviewers. Any product that may be evaluated in this article or claim that may be made by its manufacturer is not guaranteed or endorsed by the publisher.
Research integrity at Frontiers
Learn more about the work of our research integrity team to safeguard the quality of each article we publish.