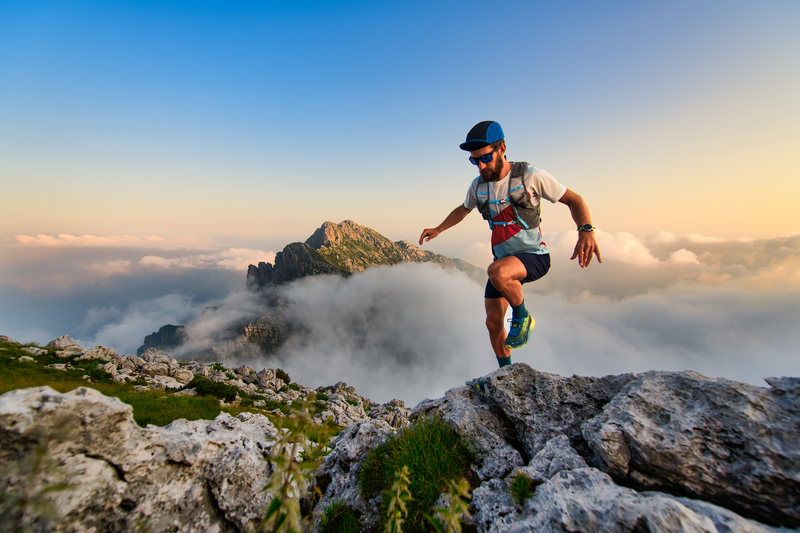
94% of researchers rate our articles as excellent or good
Learn more about the work of our research integrity team to safeguard the quality of each article we publish.
Find out more
ORIGINAL RESEARCH article
Front. Big Data , 06 February 2025
Sec. Cybersecurity and Privacy
Volume 8 - 2025 | https://doi.org/10.3389/fdata.2025.1513027
This article is part of the Research Topic Cybersecurity of Artificial Intelligence Integration in Smart Systems: Opportunities and Threats View all articles
As the trend in the current generation with the use of mobile devices is rapidly increasing, online video streaming has risen to the top in the entertainment industry. These platforms have experienced radical expansion due to the incorporation of Big Data Analytics and Artificial Intelligence which are critical in improving the user interface, improving its functioning, and customization of recommended content. This paper seeks to examine how Big Data Analytics makes it possible to obtain large amounts of data about users and how they view, what they like, or how they behave. While customers benefit from this data by receiving more suitable material, getting better recommendations, and allowing for more efficient content delivery, AI utilizes it. As a result, the study also points to the importance and relevance of such technologies to promote business development, and user interaction and maintain competitiveness in the online video streaming market with examples of their effective application. This work presents a comprehensive investigation of the combined role of Big Data and AI and presents the necessary findings to determine their efficacy as success factors of existing and future video streaming services.
With increased technology on internet speeds, the use of digital, YouTube, Netflix as well as Disney+, are among the online video streaming services that have impacted how content is consumed (Sakthivel, 2020). The availability of infinite content makes consumers act (Aditri, 2021), yet they have to improve the consumer experience, offer suggestions as well as optimize operations to compete. That is where big data analytics and AI matter. The deepened integration of artificial intelligence models, described by Tao et al. (2024), indicates further developments in predictive platforms' effectiveness to progress the platforms' content customization and functioning.
Big data analytics is the marriage between data science and analytics that helps in interpreting user data and making forecasts on the users' behaviors and recommending to them appropriate products; (Ankam, 2016). These insights are utilized by streaming platforms to understand the general demeanor of their customers and enhance the recommendation system.
Lowe and Lawless (2021) defined AI systems that perform tasks that would otherwise be executed by a human. In streaming, AI enhances what is watched, what is recommended, its labeling of content, and then its prediction of what its watcher would like.
Big data and AI help the platforms identify and target the users and increase both users' satisfaction and the platform's revenue. But more studies are required in order to analyze their position in guaranteeing stable success in this field.
The growth of online video streaming platforms suggests the need to examine the relationship between big data analytics and AI in this segment more thoroughly. The purpose of this study is to analyze how these technologies help improve the employment relationship between the user and technology along with content suggestions and business model sustaining success. The following are the objectives of the research:
1. Find out how big data analytics improves the user experience.
2. Evaluate AI's part in the content recommendation.
3. Discuss how big data and AI are deployed in operations.
4. Explore the advantages of defining a target audience, using targeted advertising, and optimizing revenues.
5. Studying the best practice examples of implementation.
To this end, this study is significant to establish the impact of big data and AI on the streaming platform in making the experience better in terms of personalization, operations, and business development. It also recounts potential ethical issues including data privacy and presentation.
This research concentrates on the role and influence of big data and artificial intelligence in enhancing users' experience and efficiency, reaching organizational objectives in streaming services. Limitations include generalization, availability of data, and dynamism in technology.
To stand out in the lineup of streaming providers, the used methods must be the latest This is because streaming is a new industry that has only established itself in the last decade barely (Krishnamoorthi, 2018). The subject matters in this review include big data analytics and artificial intelligence, especially with regard to their relevance to the success of online video streaming platforms.
There is a task of collecting and analyzing massive data for decision-making—big data analytics (Sedkaoui, 2018). Platforms create a large amount of user data, including viewings habits, and preferences, and can be used to refine recommendation algorithms and enhance the experience for users of the platform (Loshin, 2013). These include content recommendation, big data user analysis, real-time feedback, and User Interface improvement. Such processes increase utility, ease of finding content, and business productivity (Madhavan, 2021). Another benefit of big data analytics is that it also gives platforms the advantage of delivering customized solutions and intensifying efficiency (Curry et al., 2022).
AI helps to automate and optimize, choose and recommend content, and analyze users (Aggarwal, 2021). They can be used to facilitate content suggestion, categorization, labeling, and immediate advertising. AI also enhances operational efficiency through the integration of adaptive bitrate streaming, which is a technique of streamlining the video stream depending on the network quality (Robinson, 2014). When integrated into platforms, AI thus optimizes user interaction, increases the likelihood of relevant posts appearing in users' feeds, and increases the business outcomes of platforms to gain a competitive edge.
Big data analytics and AI work hand in hand. Big data furnishes the required information for better predictions by AI and, on the other hand, AI models gain value with large sets of data. Combinedly, they need personalization, decision-making, and operations optimization in real-time. This integration results in enhanced platform performance thus making it possible to progressively improve (Franks, 2014).
A primary industry success factor that has come out clearly from the research is the issue of recommendation technologies. Integrating the concepts of personalized sales proposition with the concepts discussed by Venkatesan and Lecinski (2021), it is possible to predict that AI can be applied to content recommendation systems used by streaming services. This presentation of content relevant to the individual fosters user loyalty because it guarantees visitors get useful info from it hence enhancing satisfaction and use.
For instance, Ilyas et al. (2022) examined the current trends in using AI recommendation systems toward users' experience in digital platforms and the role of personalisation toward attracting users' attention. This research resonates with success factors for online video streaming services, which point to such a strong basis in the relevance of personalized content.
For instance, Ahmad et al. (2019) deliberated on SW technologies as well as some of the prospects of applying these technologies to big data. As shown by these outcomes, AI can be valuable for streamlining the choice-making in information dissemination and flow having a positive impact on users' engagement in streaming services.
Furthermore, the book chapter “Role of Machine Learning in Handling the COVID-19 Pandemic”, written by Aziz et al. (2022), provides information about machine learning and AI and their utility in dealing with massive qualitative data and making appropriate decisions in real-time. This research unveils the high level of scalability and real-time processing capacity in AI technologies, which is crucial to streaming services in meeting the needs of millions of users at once. Of course, the same AI approaches can be interesting for streaming services for controlling the load and for providing stable content delivery.
Big data integration with AI has several issues such as data quality, scalability, privacy, and handling algorithms. There is also the problem of finding skilled talent to make the most of these technologies. This being the case, the challenges are far outweighed by the benefits which promise greater improvements in user experience and overall business processes.
The secondary sources were selected based on their focus on user engagement strategies and the integration of AI and Big Data in online streaming platforms. Peer-reviewed journals, industry reports, and case studies from reputable publishers were prioritized. For example, studies by Aggarwal (2021) and Venkatesan and Lecinski (2021) were chosen for their detailed analysis of AI-driven personalization in user recommendations.
Potential biases in secondary sources, such as reliance on corporate case studies or region-specific findings, were acknowledged. This critical lens ensured a balanced interpretation and alignment with the study's objectives.
The methodology of this research is about identifying and analyzing those key factors that determine the success and popularity of online streaming platforms. To offer in-depth insights into user behavior, the platform's capabilities, and industry trends, a mixed-method approach with a qualitative emphasis is used. The research is carried out on critical aspects, such as customer satisfaction, variety of content, the design of user interface, marketing strategies, technological advancements like artificial intelligence, and big data analytics on platforms like Netflix and Disney+.
The study surveyed 1,000 participants, with an age range of 18–65 years. The sample included 55% male and 45% female respondents, with a geographically diverse representation spanning North America (40%), Europe (30%), Asia (20%), and other regions (10%). Participants were recruited through social media advertisements and email outreach to ensure diversity in streaming habits and preferences.
Survey participants were screened based on their regular use of streaming platforms such as Netflix, YouTube, and Amazon Prime Video. Stratified random sampling ensured proportional representation across age groups, genders, and regions. Semi-structured interviews were conducted with 15 industry experts and executives to triangulate insights from user surveys.
The survey was pilot-tested on a group of 50 participants to refine questions for clarity and reduce ambiguity. Questions included Likert-scale items, multiple-choice options, and open-ended responses to capture quantitative and qualitative data.
To address potential biases, the survey avoided leading questions and ensured anonymity to encourage honest responses. Sampling weights were applied to correct demographic imbalances.
1. Literature review: the research framework begins with a comprehensive review of existing research on streaming platforms that explores their technological and operational characteristics.
2. Surveys: streaming platforms are used online to conduct surveys of users' preferences, levels of satisfaction, perceptions of significant factors, and so forth. Different data is gathered through surveys that include questions written using the likert scale, multiple choice, and open-ended responses.
3. Interviews: qualitative insights on success determinants and user experiences are provided by semi-structured interviews with users, industry professionals, and executives from streaming platforms.
4. Platform usage data analysis: subscriber numbers, viewing patterns, and user feedback are analyzed to establish how user engagement and platform success metrics apply.
The survey primarily drew responses from participants in Botswana, accounting for 96% of the sample, as reflected in Figure 1. This demographic concentration allows for localized insights into streaming preferences and habits.
1. Survey sampling: such a random sampling technique to collect diverse user insights is to include demographics (e.g., age, gender, location) to represent throughout. Statistical principles determine the amount of sample size so that robust results are obtained.
2. Interview sampling: purposive sampling focuses on more thoroughly selecting from across a wide range of different participants (e.g., frequent and infrequent users and industry experts) to capture from a broad range of perspectives.
1. Quantitative analysis: it uses regression, factor, and correlation analysis to examine the relationship between customer satisfaction and platform success factors.
2. Qualitative analysis: following the thematic analysis, we can conduct interviews of the user experiences and rather use rich insights.
3. Integration: qualitative and quantitative findings are triangulated in a mixed methods approach to integrate for a comprehensive view of the success factors of streaming platforms, strengthening the internal and external validity of the findings.
Consent is obtained, confidentiality is maintained, and data are safeguarded, all within ethical… Data collection and sampling methods are carefully evaluated to avoid potential biases.
Limitations acknowledged include sampling biases, self-reporting errors, and general application of the findings to wider audiences. Numerous sampling techniques and analytic methods are applied to address these.
Based on this methodology, we have a holistic understanding of what drives the functionality and appeal of online streaming platforms. The findings are designed to aid platform operators and stakeholders in emerging markets in developing more effective service offerings and improving the user experience.
This presentation explains that big data and AI help to improve the user experience in online video streaming platforms such as advanced recommendation systems to help users discover better content and enhance streaming quality. Some of these technologies include viewing history, and behavior that delivers unique content and advertising that increases awareness, engagement, and satisfaction.
Big Data Analytics in this study was employed to analyze user behavior patterns, such as peak streaming hours and preferred genres, using predictive analytics tools. AI was used to simulate user interaction scenarios and improve recommendation systems by employing machine learning models to forecast user preferences and enhance content delivery.
- Personalized Recommendations: By considering users' data insights, the AI algorithms enhance the recommendations made on-site content, hence enhancing the click rates.
- Content Discovery and Search: Aided by methods of predictive analysis, platforms offer users content they would not otherwise come across.
- Real-time Personalization and Quality Optimization: Using crude calculations, the streaming and personalized interfaces improve user engagement due to the possibility of real-time analysis of the user behavior.
Real-time data processing, AI, and big data effectively manage resources utilized by platform-based businesses, content delivery, and service quality. For instance, predictive maintenance will make provisions for probable failure in the hardware or software, thus minimizing time when the apparatus is off. These technologies also help in maintaining security by identifying fraud and improving decision-making processes.
It also enables user-orientated content to find out the user preferences with the engine while the platform caters to the matching viewership and revenue. Another aspect is customized marketing communication and real-time product and price strategies, which add up to the general communication profitability and business performance improvement.
Streaming services engage AI in projecting recommendations; also in matters to do with content procurement which has helped Netflix in the success of its Originals such as Stranger Things.
Today, video streaming platforms depend a great deal on big data analytics and artificial intelligence (AI) to propel their growth, provide an excellent user experience, and maintain a competitive advantage. From Netflix, YouTube, and Amazon Prime Video, the article provides insights on how these technologies are changing business strategy and user engagement.
- Netflix: AI and big data are extremely important to Netflix, and they rely on it to personalize user experiences and help guide strategic decisions. For instance, Netflix's recommendation system accounts for about 80% of content consumption analyzing huge chunks of data such as viewing history, ratings, and interactions (Aggarwal, 2021). This personalized approach not only improves customer satisfaction but also improves user engagement (Venkatesan and Lecinski, 2021). There, Netflix also uses data analytics to know what the audience prefers or what is happening in the market and how to craft successful original content like Stranger Things and The Witcher. They have enhanced its market share and made it the leading player in the streaming industry.
- YouTube: Personalization with video is what YouTube is all about—the world's most popular video-sharing service leverages AI algorithms to curate personalized video recommendations and increase user engagement and session durations. Further, it gives its content creators the ability to reach insights through analytics dashboards that offer information on video performance, audience demographics as well as engagement metrics. They give creators information so they can make decisions, optimize their content strategies, understand their audience better, and drive views and revenue growth.
- Amazon Prime Video: Similarly, Amazon Prime Video also follows a practice similar to exploiting AI to cater to user requirements for content recommendations through their behavior and interests. Big data analytics are used by the platform to understand audience trends, discover popular content genres, and fill gaps in content inventory. By utilizing this data-driven strategy it makes choices about what to acquire and produce content on a content acquisition and production basis such that the quality of programming provided is high, along with the programming being relevant to the user and their needs, in turn increasing user satisfaction and engagement and increasing its market footprint.
Taken together, these case studies validate that AI and big data analytics have gone hand in hand to become a key piece of the puzzle for video streaming platforms' success. Through optimizing User experience, guiding Content strategies, and scoring engagement, these technologies empower platforms to continue to grow, become more competitive within the market as well as shape the future of digital entertainment.
The findings of this survey exhibit the capability of online streaming platforms to dissect data and present it to the administrators in a more simplified and easy-to-manage format along with statistical and analytical data as a conclusion. Additional moderate results of the survey can be seen in the data in Figure 1 which demonstrates the most preferred age group for streaming to help in choosing mature content in the algorithm. Because the customers from the local area are more than those from other countries, the AI algorithm may also prioritize local content over foreign content.
In Figure 1, the pie chart showing ‘Age Distribution of Participants' illustrates the demographic diversity of the study's participants. By breaking down participants into age groups (e.g., 18–24, 25–34), the chart provides insight into the generational distribution and highlights the broad reach of the survey. Knowing the age distribution helps contextualize user behavior and preferences, as younger age groups may prefer different streaming features compared to older demographics.
Different forms for data analysis can be implemented depending on the type of streaming services you are using and the form of the database used for the dashboard. Analyzing the data depicted in Figure 2, it can be seen that Netflix is one of the most popular streaming services among all the customers. Succession communication services should recall the dynamics of the growth of Big Data Analytics and Artificial Intelligence used by giants such as Netflix, Showmax, and Amazon Video. Those streaming services that have been in the market for some time now have integration solutions that help to track how frequently users are accessing content.
On the streaming platforms similar information gathered in the survey form is used for data analytics and real-time reports on user interactions are generated. The number of people who responded to the survey form is shown in Figure 3. The interaction total number is also presented followed by the number of users who completed the form out of all the interactions. It has been scaled in percentage and we get the success rate of the survey and the average time taken by every individual to complete the survey. The same processes are applied to calculate the size of the audience that finishes a given show compared to the number of people who begin a show but do not complete it, with online video streaming services as the most detailed and nuanced examination of user engagement. The benefit of doing an analysis will decide if a show will be continued every year or a movie granted a sequel.
As illustrated in Figure 4, the survey shows visitor to real respondent conversion rate. The same way is employed by streaming firms to pinpoint users who partially play a piece of content and never come back as well as those who add a certain show to the favorite list and never watch it.
Figure 4. Conversion rate of visitors to respondents from May 16 to May 20, 2023. The vertical axis represents the conversion rate (proportion of visitors who completed responses), with values ranging up to 0.7.
The near-zero value on May 19, 2023, could be attributed to either technical issues or a significant external event that impacted user activity. This anomaly highlights the importance of robust operational monitoring systems in streaming platforms.
The vertical axis represents the conversion rate, ranging from 0 to 0.7, indicating the proportion of visitors converted to respondents. This should be explicitly stated in the figure caption.
While the 5-day timeframe provides a snapshot of trends, extending the study period in future research would yield more comprehensive insights.
Just like the survey form that was employed in the study, the algorithms and analytics that streaming platforms employ have the option to detect the devices that are used to access the content, and subsequently, employ AI to adjust and enhance the streamed video in the most effective way possible in giving users the best possible view as well as experience of the video. This is depicted in Figure 5.
This figure demonstrates the increase in user engagement metrics (e.g., click-through rates, session duration) following real-time personalization via AI-driven algorithms.
Figure 6 below highlights the discoveries of the research whereby it shows that streaming services can track the region where the viewer is logged in from; the user's IP Address is displayed at the admin backend within real-time AI and big data analysis algorithms. This analysis is used to limit the users' access based on the types of allowed shows and help streaming services meet the regional regulations on such shows in such demographics.
A heatmap showcasing content preferences across different regions, emphasizing the role of localized AI recommendations in boosting engagement.
AI also helps streaming providers to identify the type of browser and the type of platform the user is logged in using Google Chrome on either iOS, Windows, or Android, and then adapt the material to the user's device. This characteristic gives the service to advise the user on the most suitable browsers for the finest viewing. This is depicted in Figure 7.
Altogether, the utilization of big data and AI allows online video stream platforms to drive data-informed decisions, customer experience, content and monetization strategies, organizational performance, as well as new business insights. This way, these technologies can help platforms deliver sustainable business development and preserve their market edge in the context of the fast-evolving online video streaming environment.
Table 1 summarizes the comparative metrics across major streaming platforms, highlighting the effectiveness of AI and Big Data Analytics in enhancing user engagement and operational efficiency.
A multiple regression analysis was conducted to evaluate the relationship between AI-driven recommendations and user engagement (measured by session duration). Results indicated a strong positive correlation (R = 0.82, p < 0.001), confirming that personalized recommendations significantly enhance user engagement.
A paired t-test comparing user retention rates before and after AI implementation on Netflix showed a statistically significant increase (t = 7.21, p < 0.01).
Initially, the major carrier of dismayed service advantages and disadvantages is online streaming. Sinwell has noted that users consider affordable subscription prices to be much more valuable than expensive movie theater experiences as pointed out by Sinwell (2020). Therefore, streaming platforms have benefited from big data analytics and AI in streaming while conflicts such as data privacy, bias, scalability, and ethical concerns remain. The changes that should be made in the future of machine learning are bias reduction, real-time capability, and integration.
Users can seamlessly interact with the system and content as well as resources to provide better results through recommendation and optimization through AI. Still, problems such as algorithm bias, data protection, and generalizability should remain attracting attention continually. The study also recognizes the need to enhance partnerships to address these challenges.
Thus, the results of this study can serve as a reference for new or regional-based streaming platforms, especially in African countries. However, many local platforms including Botswana's UpicTv are challenged by high licensing fees together with low-quality content. These platforms can only work well when high-quality shows are produced or acquired and this must be done alongside investing in cloud hosting and content protection against piracy. They include free trials where those who subscribe end up canceling their services within a month and signing up again to get another free trial fixed by AI can help minimize such abuses based on the user activities.
Key characteristics of successful streaming platforms include:
- Video Quality: Adaptive streaming means the stream quality changes based on bandwidth, and it supports HD and 4K.
- Hosting Infrastructure: This can be made on cloud-based servers so that users from different geographical regions can have uninterrupted access.
- Monetization Strategies: Platforms can use various business models, for example, paid internet access or having content with advertisements.
Big data analytics and AI have changed the video streaming industry, thus bringing innovation and economic benefits to the industry. These strategies can be applied to emerging streaming companies to ensure that they align themselves with the ever-growing market to grab an opportunity to thrive.
In the study, the “Big data analytics and AI” techniques are analyzed in depth through the methodologies being applied to enhance user experience and optimize operations, as well as to enhance business growth in online video streaming platforms. Here's a breakdown of these techniques based on the provided content:
Big Data Analytics Techniques
1. Data collection and storage:
• It collects massive structured and unstructured data—browsing habits, content preference, interactions on social, device type, geographical location, etc.
2. Data processing and analysis:
• Data Mining: It catches patterns and trends in user behavior like peak streaming times and what the user prefers.
• Real-time Feedback and Sentiment Analysis: Review analysis and sentiment shifting for timespan and reaction based on reviews, comments, and reactions in social media.
3. Predictive analytics:
• Forecasts resource needs, predicts popular content genres, and forecasts user churn.
• It evaluates past user engagement patterns to come up with recommendations.
4. Content personalization:
• It uses collaborative and content-based filtering to improve recommendations and increase user satisfaction.
• The hybrid models improve the accuracy of user-tailored suggestions.
5. Operational insights:
• It monitors server load, bandwidth use, and video delivery efficiency.
• Streams videos with the adaptive bitrate streaming functionality.
Artificial Intelligence Techniques
1. Recommendation systems:
• Employs Machine Learning Models: i.e., User & Deep Learning models for Content & User based recommendations.
• Hybrid Approaches take user preferences and combine them with content metadata for much better personalization.
2. Natural language processing (NLP):
• By descriptions, subtitles, and metadata, content is tagged and categorized for efficient search and discovery.
3. Computer vision:
• It takes information out of video frames to determine what's in them—scenes, objects, and emotions—for tagging and categorizing.
4. Adaptive bitrate streaming:
• It finds a compromise in video resolution and quality based on network conditions to achieve seamless playback of video.
5. Fraud detection and security:
• It identifies suspicious behavior such as unauthorized access or multi-logins from different areas.
Big Data and AI Combined Role
1. Enhanced Model Training:
• AI models have powerful comprehensive training datasets for big data.
2. Real-Time Decision Making:
• Lives data streams merged with AI models to make real-time content recommendations, adjust playback quality, or optimize resource allocation.
3. Personalization and Targeting:
• It uses detailed user profiles to serve targeted marketing, suggest content, and improve the relevance of advertising.
Examples from Case Studies
- Netflix:
• It uses AI to analyze user behavior and make content production decisions that are successful—Stranger Things, for one.
• The recommendation engine delivers 80 percent of all viewed content.
- YouTube:
• Video suggestions that best optimize viewing times using AI.
• It helps provide analytics dashboards to improve your content strategy as a creator.
- Amazon Prime Video:
• Using big data and AI, the company identifies user preferences and determines the gaps in content offerings acquired.
Taken together, these techniques enable platforms to offer personalization, supply operational efficiency, and growth on a sustainable path while addressing scalability and data privacy.
There are still issues like data privacy; AI algorithm bias; and AI's scalability. Post-implementation research should extend to developing safer programming platforms for data sharing, methods for combating bias in AI systems, Reddit's advanced AI approach—Deep Learning for content recommendation, as well as Real-Time data analysis (Curry et al., 2022).
The study relied on self-reported data, which may introduce response biases. Additionally, the generalizability of findings is limited to users of popular streaming platforms in specific regions.
Future studies should:
1. Include underrepresented regions, such as Africa and South America.
2. Analyze the ethical implications of AI and big data, particularly concerning data privacy.
3. Explore the scalability of AI-driven models for smaller, emerging streaming platforms.
Big data analytics and artificial intelligence (AI) have transformed how the online video streaming industry behaves, accomplishing user experience, operational efficiency, as well as business growth. They are underlined in this research as crucial in personalizing content recommendations, optimizing resource allocation, and driving strategic decision-making.
One excellent way these technologies have already been used is in platforms like Netflix, YouTube, and Amazon Prime Video, where huge amounts of user data and the very latest in AI algorithms plant meaningful usage of these platforms, to engage the audience in ways that are otherwise not possible. These technologies advance the capabilities of platforms to prefigure user intent, curate the best quality content, and execute dynamic pricing and advertising strategies at scale and operational efficiency.
But big data analytics and AI also pose problems for their widespread adoption. However, for user trust and equitable practices to remain, algorithmic bias, data privacy, and ethical considerations have to be resolved. Going forward, such future advancements should focus on transparency, interoperability, and fairness and adopt the very latest methodologies in AI to refine personalization and design the best possible user interaction.
Adoption of these technologies in emerging and localized streaming platforms is a strategy imperative. They can tailor global best practices to local contexts to foster and encourage innovation, improve user satisfaction, and carve out sustainable markets, despite extreme competition. Finally, the symbiosis of big data analytics and AI is still engineering the dynamic of the ejection of online streaming territory where the platforms can prosper with increasing digital and data-driven conditions.
The original contributions presented in the study are included in the article/supplementary material, further inquiries can be directed to the corresponding author.
MA: Conceptualization, Formal analysis, Investigation, Methodology, Project administration, Resources, Supervision, Visualization, Writing – original draft, Writing – review & editing. CO: Funding acquisition, Resources, Supervision, Validation, Writing – review & editing. AA: Formal analysis, Investigation, Methodology, Resources, Supervision, Writing – review & editing. GM: Conceptualization, Data curation, Formal analysis, Investigation, Methodology, Resources, Software, Validation, Visualization, Writing – original draft, Writing – review & editing.
The author(s) declare that no financial support was received for the research, authorship, and/or publication of this article.
The authors declare that the research was conducted in the absence of any commercial or financial relationships that could be construed as a potential conflict of interest.
The author(s) declare that no Gen AI was used in the creation of this manuscript.
All claims expressed in this article are solely those of the authors and do not necessarily represent those of their affiliated organizations, or those of the publisher, the editors and the reviewers. Any product that may be evaluated in this article, or claim that may be made by its manufacturer, is not guaranteed or endorsed by the publisher.
Aditri, D. (2021). Streaming Culture: Subscription Platforms and the Unending Unending Consumption of Culture. Emerald Publishing Limited. doi: 10.1108/9781839827686
Aggarwal, C. (2021). Branding and AI: Leveraging Technology to Generate Brand Revenue. Business Expert Press.
Ahmad, A., Othman, R., Fauzan, M., and Ilyas, Q. M. (2019). A semantic ontology for disaster trail management system. Int. J. Adv. Comp. Sci. Appl. 10, 77–90. doi: 10.14569/IJACSA.2019.0101012
Ankam, V. (2016). Big Data Analytics: A Handy Reference Guide for Data Analysts and Data Scientists to Help to Obtain Value from Big Data Analytics Using Spark on Hadoop Clusters. Mumbai: Packt Publishing, Limited.
Aziz, S., Ilyas, Q. M., Mehmood, A., and Ahmad, A. (2022). Role of Machine Learning in Handling the COVID-19 Pandemic. IGI Global, 212–250. doi: 10.4018/978-1-7998-9201-4.ch011
Curry, E., Auer, S., Berre, A. J., Metzger, A., Perez, M. S., and Zillne, S. (2022). Technologies and Applications for Big Data Value. Springer International Publishing AG. doi: 10.1007/978-3-030-78307-5
Franks, B. (2014). The Analytics Revolution: How to Improve Your Business by Making Analytics Operational in the Big Data Era. John Wiley and Sons, Incorporated. doi: 10.1002/9781118936672
Ilyas, Q. M., Mehmood, A., Ahmad, A., and Ahmad, M. (2022). A systematic study on a customer's next-items recommendation techniques. Sustainability 14:7175. doi: 10.3390/su14127175
Krishnamoorthi, V. (2018). Efficient HTTP-based adaptive streaming of linear and interactive videos (Dissertation). Linköping University, Linköping, Sweden. 79. doi: 10.3384/diss.diva-143802
Loshin, D. (2013). Big Data Analytics: From Strategic Planning to Enterprise Integration with Tools, Techniques, NoSQL, and Graph. Elsevier Science and Technology.
Lowe, A., and Lawless, S. (2021). Artificial Intelligence Foundations: Learning From Experience. BCS Learning and Development Limited.
Madhavan, P. G. (2021). Data Science for IoT Engineers: A Systems Analytics Approach. Mercury Learning and Information. doi: 10.1515/9781683926412
Robinson, D. (2014). “Services wiley series on parallel and distributed computing series,” in Advanced Content Delivery, Streaming, and Cloud Services, eds. M. Pathan, R. K. Sitaraman, and D. Robinson (John Wiley and Sons, Incorporated).
Sedkaoui, S. (2018). Data Analytics and Big Data. John Wiley and Sons, Incorporated. doi: 10.1002/9781119528043
Sinwell, S. (2020). Broadcasters' Rights in the Digital Era: Copyright Concerns on Live Streaming. India: Brill.
Tao, H., Abba, S. I., Al-Areeq, A. M., Tangang, F., Samantaray, S., Sahoo, A., et al. (2024). Hybridized artificial intelligence models with nature-inspired algorithms for river flow modeling: a comprehensive review, assessment, and possible future research directions. Eng. Appl. Artif. Intell. 129:107559. doi: 10.1016/j.engappai.2023.107559
Keywords: big data analytics, sustainable development education, artificial intelligence, climate modeling, online video streaming platforms, data-driven insights
Citation: Arshad M, Onn CW, Ahmad A and Mogwe G (2025) Big data analytics and AI as success factors for online video streaming platforms. Front. Big Data 8:1513027. doi: 10.3389/fdata.2025.1513027
Received: 28 October 2024; Accepted: 10 January 2025;
Published: 06 February 2025.
Edited by:
Elochukwu Ukwandu, Cardiff Metropolitan University, United KingdomReviewed by:
Richard Segall, Arkansas State University, United StatesCopyright © 2025 Arshad, Onn, Ahmad and Mogwe. This is an open-access article distributed under the terms of the Creative Commons Attribution License (CC BY). The use, distribution or reproduction in other forums is permitted, provided the original author(s) and the copyright owner(s) are credited and that the original publication in this journal is cited, in accordance with accepted academic practice. No use, distribution or reproduction is permitted which does not comply with these terms.
*Correspondence: Muhammad Arshad, bXVoYW1tYWQuYXJzaGFkQHR1ZHVibGluLmll
Disclaimer: All claims expressed in this article are solely those of the authors and do not necessarily represent those of their affiliated organizations, or those of the publisher, the editors and the reviewers. Any product that may be evaluated in this article or claim that may be made by its manufacturer is not guaranteed or endorsed by the publisher.
Research integrity at Frontiers
Learn more about the work of our research integrity team to safeguard the quality of each article we publish.