- 1AI Institute, University of South Carolina, Columbia, SC, United States
- 2Hewlett Packard Enterprise Labs, Houston, TX, United States
The emergence of advanced artificial intelligence (AI) models has driven the development of frameworks and approaches that focus on automating model training and hyperparameter tuning of end-to-end AI pipelines. However, other crucial stages of these pipelines such as dataset selection, feature engineering, and model optimization for deployment have received less attention. Improving efficiency of end-to-end AI pipelines requires metadata of past executions of AI pipelines and all their stages. Regenerating metadata history by re-executing existing AI pipelines is computationally challenging and impractical. To address this issue, we propose to source AI pipeline metadata from open-source platforms such as Papers-with-Code, OpenML, and Hugging Face. However, integrating and unifying the varying terminologies and data formats from these diverse sources is a challenge. In this study, we present a solution by introducing Common Metadata Ontology (CMO) which is used to construct an extensive AI Pipeline Metadata Knowledge Graph (AIMKG) consisting of 1.6 million pipelines. Through semantic enhancements, the pipeline metadata in AIMKG is also enriched for downstream tasks such as search and recommendation of AI pipelines. We perform quantitative and qualitative evaluations on AIMKG to search and recommend relevant pipelines to user query. For quantitative evaluation, we propose a custom aggregation model that outperforms other baselines by achieving a retrieval accuracy (R@1) of 76.3%. Our qualitative analysis shows that AIMKG-based recommender retrieved relevant pipelines in 78% of test cases compared to the state-of-the-art MLSchema-based recommender which retrieved relevant responses in 51% of the cases. AIMKG serves as an atlas for navigating the evolving AI landscape, providing practitioners with a comprehensive factsheet for their applications. It guides AI pipeline optimization, offers insights and recommendations for improving AI pipelines, and serves as a foundation for data mining and analysis of evolving AI workflows.
1 Introduction
The rapid evolution of artificial intelligence (AI) has led to significant advancements in techniques, necessitating continuous knowledge updates. The submission rates at conferences such as NeurIPS, which constitutes several thousand papers, demonstrate the rapid growth and competitiveness in AI research. Furthermore, there are several versions of generative models being released for various tasks which are difficult to keep track of. The rate of invention of new AI methods also introduces a challenge of suitable model selection for a given task and dataset. The success of AI methods has also led to the development of larger and more complex models to tackle various challenges, due to which training of AI models has become increasing challenging with its increasing complexity. To improve training efficiency, AutoML methods (He et al., 2021; Karmaker et al., 2021) have been introduced for optimizing models and hyperparameter tuning. MLFlow (Zaharia et al., 2018) and MLSchema (Publio et al., 2018) use a model-centric approach for metadata logging, requiring separate instances for each trained model for a given pipeline, say, entity extraction from health records. Openlineage (Hariharan et al., 2024) tracks data lineage through its lifecycle. However, AI pipeline development also includes stages such as dataset selection, preprocessing, feature engineering, and deployment. For reproducibility, metadata logging must encompass all stages, not just model selection and tuning. A comprehensive framework is needed to track all stages, executions, models, and datasets to solve a given AI task.
The Common Metadata Framework (CMF; Koomthanam et al., 2024) addresses this by serving as a pipeline-centric metadata logging system that captures metadata for all stages, executions, models, datasets, and metrics in an integrated manner, enabling the search for the optimal execution path. While CMF provides a holistic approach to metadata logging, a robust framework is required to facilitate AI pipeline optimization. Such optimization can be achieved by leveraging logged metadata to recommend past successful pipeline executions as a seed, reducing the overall experimentation runs. This recommendation requires detailed metadata of numerous pipelines executed, capturing the interactions and dependencies (e.g., input/output datasets, parameters, and configurations) of each stage. Generating such metadata by executing pipelines is not feasible as it demands time and computational resources. On the other hand, open-source platforms such as Papers-with-code (2018), OpenML (Vanschoren et al., 2014), Hugging Face (2016), and Kaggle (2010) expose metadata of already executed pipelines which can be leveraged. To enable metadata interoperability from diverse sources, they must be integrated. However, it poses challenges such as differing nomenclature, data structure variations, and lack of component semantics to understand context and perform reasoning on the entities.
To address these challenges, we introduce the Common Metadata Ontology (CMO), built on the foundations of CMF's pipeline-centric approach. CMO is an unifying schema to integrate metadata from these diverse sources to construct AI pipeline Metadata Knowledge Graph (AIMKG) that enables search and recommendation of relevant AI pipelines for optimization. Knowledge graphs (KG) provide a deeper understanding of relationships and enable context-aware recommendations by capturing both explicit and implicit connections. CMO supports such semantic and multimodal properties which are computed while constructing AIMKG. For example, AIMKG can identify the shared semantics between pipelines for object detection and 3d instance segmentation, recognizing them as vision-based tasks even without explicit naming and facilitate reasoning. As a downstream application, we develop a search and recommender system that demonstrates the potential of AIMKG to recommend relevant pipelines for optimization. The recommender system provides explainable recommendations and ensures reproducibility by providing source information of AI pipelines. The specific contributions of the study are as follows:
1. Proposing Common Metadata Ontology with a pipeline-centric view to integrate and aggregate the metadata mined from diverse sources. CMO supports semantic properties and multimodal properties such as text and embedding vectors.
2. Construction of the first of its kind AIMKG using CMO that serves as an atlas to navigate the ever-growing AI field.
3. Enriching the AIMKG with additional knowledge and by computing semantic properties.
4. Introducing custom heuristic ranking function to recommend relevant pipelines using task, dataset, or model.
5. Introducing a custom aggregation model to generate graph embeddings that enable AI pipeline recommendation for natural language queries.
Conventional knowledge graphs capture relationships among concepts or entities and their semantic properties. For example, Linked Open Data Cloud (Musto et al., 2016) and DSKG (Färber and Lamprecht, 2021) capture semantic relationships among datasets. In contrast, AIMKG consists of process graphs that capture procedural interactions of entities in the context of training and execution, such as how datasets and models combine to produce performance metrics (e.g., dataset + model metrics, model weights). AIMKG follows the traditional semantics of entities and extends it further to process graphs. This procedural representation is a notable contribution not typically found in traditional knowledge graphs.
2 Related work
With the growth of AI models (Menghani, 2023; Mathew et al., 2021; Shrestha and Mahmood, 2019; Mohammadi et al., 2024; Berahmand et al., 2024), several frameworks have been proposed to enable the search and discoverability of these models and architectures. DeepSciKG (Kannan et al., 2020) project proposes a mechanism to create and query knowledge graphs to represent multimodal information from AI publication metadata, i.e., code, pseudocode, tables, images, and diagrams in addition to text/equations in publications. STM-KG (Brack et al., 2021) proceeds along similar lines to demonstrate how science, technology, and medicine papers can be automatically mined to automatically populate a scientific concepts knowledge graph and drive a “citation recommender.” ML Schema (Publio et al., 2018) proposed a model-centric ontology to formalize only OpenML data. Humm and Zender (2021) developed an ontology to represent ML metadata to organize and store limited number of tasks (15 as compared to 5 k in our study). AI-KG (Dessì et al., 2020) generated AI knowledge graph from published papers consisting of 330k research publications with 14 M triples that describes five types of entities (tasks, methods, metrics, materials, and others). AIMKG consists of combination of published papers (1 million) and also user-recorded metadata from OpenML and HuggingFace.
The extraction of knowledge from publicly available resources remains an active and dynamic area of research. Notably, the GraphGen4Code (Abdelaziz et al., 2021) approach has emerged as a comprehensive toolkit for constructing knowledge graphs from program code, effectively facilitating subsequent endeavors to address the creation of AutoML pipelines utilizing such knowledge graphs (Helali et al., 2022). These endeavors complement our own study, which leverages existing public repositories and published research to infer relations between AI pipeline entities. By constructing a knowledge graph, our approach aims to solve downstream tasks within the field.
In literature, there has been a consistent effort to recommend datasets for scientific problems, e.g., DataHunter (Färber and Leisinger, 2021) and DataFinder (Viswanathan et al., 2023). Croissant is a high-level format for machine learning datasets that combines metadata, resource file descriptions, data structure, and default ML semantics into a single file for downstream tasks (Akhtar et al., 2024). SIGMOD (Kumar et al., 2023) recommends datasets, models, processing steps etc. along with pipeline lineage. Similarly, other studies such as Müllner et al. (2022) use the history of AI pipelines to recommend datasets and models to solve new tasks allowing sharing of these artifacts among multiple pipelines. In our study, we integrate and aggregate multiple data sources instead of focusing on a particular data source. Specifically, our study focuses on pipeline optimization, knowledge discovery, search, and recommendation through mining metadata from diverse open sources. HuggingGPT (Shen et al., 2023) is a collaborative system that consists of an LLM as the controller and numerous expert models as collaborative executors from HuggingFace. It uses LLM-based chat interface to recommend models for tasks from different domains. We plan to incorporate an LLM interface similar to HuggingGPT in the future while focusing recommending pipeline that includes task, dataset, dataset preprocessing steps, model, metrics, and hyperparameters.
Several techniques such as CASH (Thornton et al., 2013; Guo et al., 2019) and NAS (Elsken et al., 2019) have been proposed for model optimization and hyperparameter tuning. However, our study distinguishes itself by extending beyond the confines of solely addressing problem-dataset or model-hyperparameter relationships. Instead, it delves into capturing intricate associations among models, datasets, and tasks, encompassing their hierarchical connections.
3 AI pipeline Metadata Knowledge Graph construction
3.1 Data sources
In this study, we collect AI pipeline metadata from Papers-with-Code, OpenML, and HuggingFace to construct AIMKG. The data availability of each source can be found in Table 1. In the future, we also plan to incorporate the metadata from Kaggle.
3.1.1 Papers-with-Code
Papers-with-Code provides extensive metadata for research papers and associated code repositories, encompassing over 1 million entries at the time of this paper submission. The metadata covers various components and stages of AI pipelines described in the papers. Through their API, Papers-with-Code offers metadata including PDF URLs, GitHub repository links, task details, dataset information, methods employed, and evaluation metrics and results. While not all stages of metadata are available for every paper through the API, the information can still be obtained by referring to the research papers and their code repositories.
3.1.2 OpenML
OpenML provides metadata on ML pipelines logged by users, offering detailed information on tasks, datasets, flows, runs with parameter settings, and evaluations. OpenML encompasses eight major task types executed on various datasets, resulting in 1,600 unique tasks. For each task, the most recent 500 runs have been collected which amounts to a total of 330,000 runs.
3.1.3 HuggingFace
Huggingface is a model hub that offers users access to numerous pretrained models. It covers a wide range of tasks, including domains such as computer vision, natural language processing, tabular data, reinforcement learning, and multimodal learning. Huggingface provides model-centric information, along with datasets and evaluations, enabling the construction of complete pipelines. At the time of paper submission, ~270,000+ pipelines have been collected from HuggingFace.
3.2 Common metadata ontology
The metadata from these sources follows different data structures and nomenclatures. For example, the concept “Model” is referred to as “Methods” in Papers-with-Code, “Flow” in OpenML, and “Model” in Hugging Face. We propose the Common Metadata Ontology (CMO), a unifying schema to integrate diverse data structures from Papers-with-Code, OpenML, and Hugging Face. Built on the Common Metadata Framework (CMF; Koomthanam et al., 2024), CMO ensures interoperability of metadata, enabling knowledge discovery, search, and reasoning capabilities. The overview of CMO is shown in Figure 1. The novel features of CMO are as follows: (i) following a pipeline-centric approach, similar to CMF, to capture multiple experimentation runs for each stage (train, test, validation) with parameter settings, facilitating the identification of the best execution path; (ii) modularity that allows distributed experiments and parallel logging of pipeline metadata, enabling seamless metadata capture across different teams and machines; (iii) support for additional semantic and statistical properties that can be extracted, computed, or generated from entity names (e.g., identifying tasks as image-based, text-based, or audio-based); and (iv) support for multimodal properties, including text and vector embeddings of entity names, to enable keyword and approximate search. A detailed overview of CMO and its properties can be found in Venkataramanan (2024). MLSchema (Publio et al., 2018) and MLFlow (Zaharia et al., 2018) adopt a model-centric approach. When building a pipeline, say, entity extraction from semi-structured electronic health records and testing it with multiple models, MLSchema and MLFlow require creating several instances “one for each model” to record metadata. In contrast, CMO allows all models, variations, hyperparameters, metrics, and datasets to be documented as a single instance, facilitating a scaleable and flexible metadata recording process by taking a holistic view of the entire pipeline. Hence, CMO builds upon the principles of CMF.
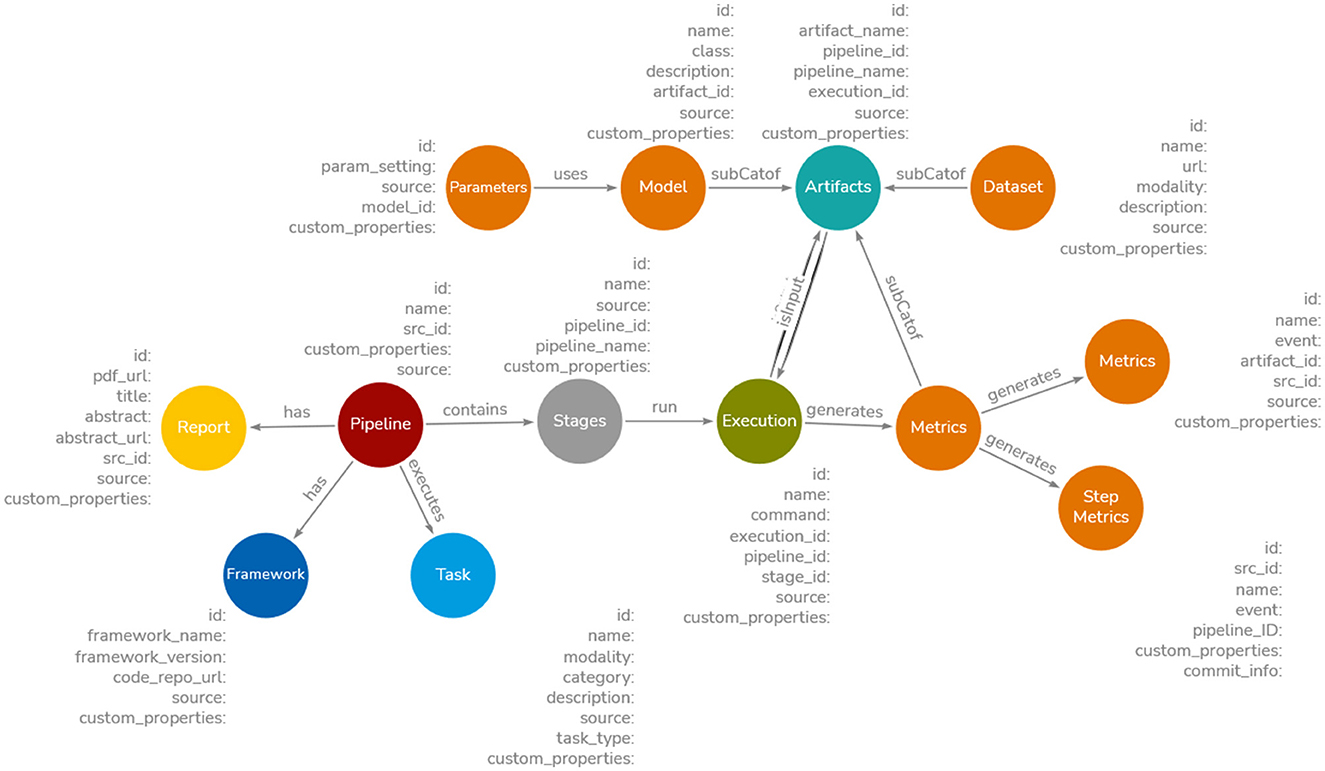
Figure 1. Overview of proposed Common Metadata Ontology. The detailed version of CMO with properties can be found at Venkataramanan (2024). The ontology consists of a pipeline node executing several stages such as data preprocessing, train, test, and validation. Each stage can have several Executions. Each Execution can use a Model and Dataset to produce Metrics and trained model weights. The Task captures a broader view of pipelines such as anomaly detection or demand forecasting. The code base is captured by Framework node and any published report or papers are present in Report node.
3.3 Problem statement
The goal is to design a correspondence mapping function F that maps the Entity-Relationship-Attributes present in the relational database of three data sources to the Nodes-Relationships-Properties of CMO. Each data source D = {E, R, A} where E = {e1, e2, …, ei} represent the set of entities, R = {r1, r2, …, rj} represent the relationship between ei and ej, A = {a1, a2, …, an} represents the set of attributes for any entity ei. First, each data source D is mapped to a graph G using a mapping function f : D → G.G = {V, M, K} where V = {v1, v2, …, vi} represents the set of vertices, M = {m1, m2, …, mj} represents the set of edges between vertices, and K = {k1, k2, …, kn} represents the set of properties of the vertices. The graph G of each data source consists of inherent entities, their associations, and properties present in relational database. Then, we compute a correspondence function F : G → KG that maps the elements from graph G by computing, extracting, or generating necessary information. KG = {N, R, P} where N = {n1, n2, …, ni} are the set of nodes, R = {r1, r2, …, rj} are the set of relationships between nodes, and P = {p1, p2, …, pn} are the set of properties of the nodes. For each G, F : V → N, F : M → R and F : K → P. Finally, the AIMKG is constructed as (KG1 ∪ KG2 ∪ KG3).
3.4 AIMKG construction and enrichment
3.4.1 Construction
The algorithm for construction of AIMKG is described in Algorithm 1, and the system architecture is shown in Figure 2. We collect metadata from Papers-with-Code, OpenML, and HuggingFace and represent the data using relational database. The metadata, in the relational database format D, is then converted into graph data models G through a mapping function f : D → G to analyze the inherent graph structure of each data source. To align the concepts of graph G to the concepts in the CMO, we implement a correspondence mapping function F : G → KG. The mapping function F consists of a predefined set of mappings of concepts presented in Supplementary Table 1. While specific nodes, relationships, and properties in G directly correspond to CMO, additional elements are computed, extracted, or generated by analyzing indirect associations among the entities in each data source. For example, while mapping OpenML data to CMO, the concept node Hyperparameters and Metrics needs to be computed from attributes of Runs given by OpenML. Since these are computed nodes, the relationships need to be computed by studying the associations between tables in relational database provided by OpenML. The mapping of entities from the data sources to CMO can be found in Supplementary material. Currently, AIMKG exists as both Resource Description Format (RDF) and Labeled Property Graph (LPG), and the results are presented in Section 6.
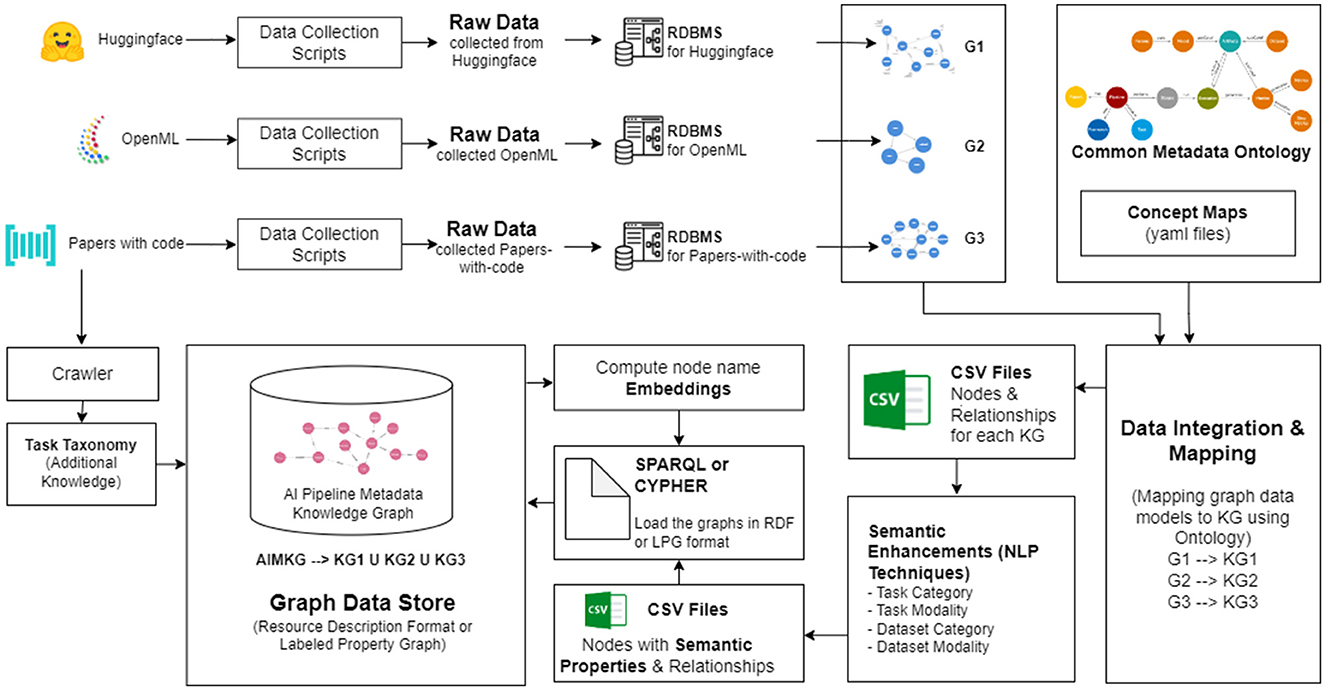
Figure 2. Overview of AIMKG construction. The data collected from Papers-with-code, OpenML, and Huggingface are translated into their relational database formats and then to their graph data models G1, G2, and G3. Then, they are mapped onto CMO. The pipeline metadata undergoes semantic enhancements before loaded as AIMKG.
3.4.2 AIMKG enrichment
To enable advanced search and recommendation capabilities, we compute semantic properties for pipeline entities, specifically tasks, and datasets. We aim to identify required semantic properties for other nodes in the future work. These semantic properties capture implicit knowledge about entities, providing valuable insights. For instance, the semantic property modality identifies the visual nature of tasks such as object detection and video instance segmentation, even if not explicitly stated. Similarly, capturing task categories such as segmentation, classification, or regression clarifies the nature of tasks and aids in organizing and categorizing pipelines by problem type.
3.4.2.1 Rule-based entity extraction
To identify the modality and category of tasks, we utilize a rule-based entity extraction approach. An extensive vocabulary is curated that includes synonyms for each modality and category, such as dialogue, translation, and text for the text modality, and terms such as classification and summarization for categories. Task names and descriptions are analyzed to assign modality and category. The main modalities we consider are Image, Text, Audio, Video, and Multimodal. While this information is available in Papers-with-Code and Huggingface, OpenML presents a challenge as task names are generated by combining task type and dataset name, which obscures modality. To address this, we analyze dataset entities in OpenML, marked as nominal or numeric, to infer task modality. Although the method is straightforward and reliable, using a manually curated vocabulary may introduce biases and limit scalability. Future research will focus on developing more scalable, automated approaches that mitigate biases and enhance robustness.
3.4.2.2 Graph-based labeling of dataset modalities
For the datasets, we determine only modality because category varies for every pipeline as per the task. For example, MS-COCO dataset can be used for detection, segmentation, or localization. The dataset from all these data sources does not contain sufficient information such as description to identify the modality. Therefore, we study the association between the task and dataset nodes to label modality for each dataset. The calculation of dataset modality is as follows:
Where D1 is the dataset, T1 to Tn are the associated tasks, and Mod(T1) represents the modality computed for a given task.
3.4.2.3 External knowledge
AIMKG also incorporates additional knowledge from various sources to enhance its semantic properties. We crawl task hierarchy information from Papers-with-Code, comprising of three levels: The first level represents task areas such as computer vision, natural language processing, or speech. The second level groups tasks into categories such as segmentation, classification, or detection. Finally, at the leaf nodes, we find the specific tasks provided by Papers-with-Code through their API. This hierarchical structure adds valuable knowledge to AIMKG, enabling a more comprehensive understanding of different task domains.
3.4.3 Node embeddings
To facilitate recommendations or approximate searches, we also compute and store embeddings for the names of tasks, datasets, models, and pipelines. A sentence transformer, all-mpnet-base-v2 (SBERT Documentation, 2023), with default embedding size 768 was used to create embeddings. The computation of embeddings can be extended to other components of the pipeline as needed. These embeddings, along with the semantic properties, are used in similarity metric calculation to rank relevant recommendation described in the following section. The embeddings are computed and added after standing up AIMKG, allowing flexibility with different models.
4 AI pipeline search and recommendation
In certain cases, the exact pipeline the user is searching for can be found in AIMKG. However, it is not always the case. We propose two different recommender systems to search and recommend relevant pipelines to user input query that can seed the experimentation.
4.1 Relevant pipeline recommendation using custom heuristics
In this section, we propose a recommender system that enables user to query a relevant pipeline based on its entities such as tasks, datasets, models, or combinations of them. Currently, we develop a custom heuristic ranking metric for tasks, datasets, and models to identify similar pipeline as these three entities are most indicative of a pipeline.
4.1.1 Problem formulation
For a given task ti, dataset di, or model mi, rank the tasks T = {t1, t2, ...tn}, datasets D = {d1, d2, ...dn}, or models M = {m1, m2, ...mn} present in AIMKG, respectively, using custom heuristics defined below. Once the most similar entities are identified, identify the pipelines associated with top-ranked items by traversing through the graph. Presently, the pipelines recommended consist of coarse-level entities such as tasks, datasets, models, metrics, frameworks, reports, and code repositories. These custom heuristic functions can be used alone or in combination as required. These recommendations act as a seed, reducing the search space for ML practitioners and minimizing the number of experiments needed to achieve optimal solutions.
4.1.2 Heuristic functions
Task similarity: Using the modality and category properties, we compute similarity for the task nodes in AIMKG as follows:
where ti, tj are any two task nodes, ei, ej are task name embeddings, namei, namej are the task name tokens, modi, modj are task modalities (image, text, audio, etc.), and cati, catj are task categories (detection, summarization, classification, etc.). J is the Jaccard similarity, and cos is the cosine similarity of embeddings.
Dataset similarity: Dataset consists of modality calculated using Equation 1. They do not have category as a semantic property as a given dataset might be suitable for two task categories such as segmentation and detection. Therefore, dataset similarity is calculated as
where di, di are any two dataset nodes, ei, ej are dataset name embeddings, namei, namej are the dataset name tokens, modi, modj are dataset modalities (image, text, audio, etc.) of the dataset names, urli, urlj are dataset URLs, and U is token-based URL similarity metric that quantifies the degree of resemblance between two URLs.
Model similarity: Model similarity is computed using the given semantic property class such as CNN and GPT. The URL given by the sources is also used as in some sources it aids in capturing the root of the model origin (Example: HuggingFace)
where mi, mi are any two model nodes, ei, ej are model name embeddings, namei, namej are model name tokens, and classi, classj are model classes (transformers, CNN, GRU, etc.).
We found through empirical experiments that a combination of embedding and keyword similarity offers the best results. For example, embedding similarity captures that “fault” and “anomaly” are synonyms. Simultaneously, in Figure 3A, segmentation tasks must be closer than classification tasks. Similarly, in Figure 3B, image-based tasks need to be closer than text-based tasks. These semantics are not captured by the embedding similarity but through keyword-based similarity of semantic properties computed for pipeline components. The ability to design and implement meta-similarity based on sets and the proximity of textual embeddings is a unique differentiator compared to existing methods such as Achille et al. (2019).
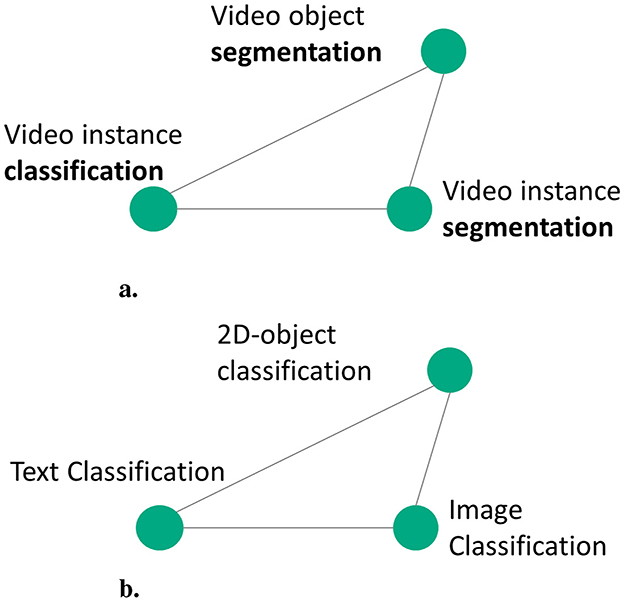
Figure 3. Illustration to show the necessity of embedding and keyword similarity. (A) Task category semantics (B) Task modality semantics.
4.2 Relevant pipeline recommendation using graph learning
In contrast to the recommender discussed in the section above, this section proposes a custom graph embedding learning model that retrieves relevant pipelines to user input queries given in natural language.
4.2.1 Problem formulation
The goal is to learn a common embedding space for the natural language query and its corresponding pipeline graph to retrieve relevant pipelines. AIMKG graph consists of several pipeline graphs {P1, P2, P3, …Pn}. Each Pi = {N, E}, where N is the set of nodes and E is the set of edges. The nodes N = {p, s, e, a, d, m, met, f, r, t} represent different elements: p is pipeline, s is stages, e is executions, a is artifacts, d is datasets, m is model, met is metrics, f is framework, r is reports, and t is tasks. For each pipeline, we have a set of queries Qi = {q1, q2, …, qn}. The goal is to learn a common embedding space for graph embedding gei that takes Pi = {N, E} as input and query embedding qei that takes in one-sentence query qi as input.
4.2.2 Query generation
Since there is no ground truth information, ChatGPT was used to generate a one-sentence query that describes the pipeline, which can simulate a user query to search for a pipeline. Similar to studies that involve the Retrieval Augmented Generation (RAG) approach (Jadon and Kumar, 2023; Guo and Chen, 2024), we utilized ChatGPT API to generate queries for a given pipeline based on the name and description of node entities such as pipeline, model, task, dataset, and metrics. These generated queries are different from the title of the paper from Papers-with-Code or title of the report or model cards from HuggingFace. The detailed analysis on queries generated can be found in Supplementary material. The following prompt was used to generate one-sentence description for each pipeline:
PROMPT:
Generate a vague two-line query summarizing
the pipeline
information below, utilizing pipeline
description, list
of tasks, list of datasets and list of
methods. Avoid forming
the query as a question. Generate these
queries as if a user
is searching for a pipeline based on the
following pipeline
information. Note, these queries should be
very different
from the pipeline name given below.
Return the query as
bullets numbered as 1., 2., and 3.
Pipeline Description:
{data['pipeline_description']}
Pipeline Name: {data['pipeline_name']}
List of Tasks:
{task_string}
List of Datasets:
{dataset_string}
List of Models:
{model_string}
4.2.3 Dataset
For this evaluation, we randomly picked 5,000 pipelines from Papers-with-Code and HuggingFace each, totaling 10,000 pipelines. Only the pipelines with complete information such as model, dataset, task, and metrics were chosen. The pipelines from Papers-with-Code and HuggingFace are more descriptive which is essential for query generation. For example, Papers-with-Code has abstract, dataset description, task description, and so on. Similarly, HuggingFace has model cards, dataset description, and so on. Such descriptive information was not found in OpenML pipelines, and so they are omitted for this evaluation. For each pipeline, on an average of two queries were generated by ChatGPT using the prompt mentioned in Section 4.2.2
4.2.4 Model architecture
In this section, we propose a custom model described in Algorithm 2 that utilizes self-attention based aggregation to learn embedding for each pipeline graphs as described in Figure 4. For each node in N, where N = {p, s, e, a, d, m, met, f, r, t}, the name and description present as text are converted to 768-dimensional embedding using sentence transformer. Using the semantic properties computed for each pipeline graph nodes (Section 3), we create a knowledge string. The knowledge is then passed to a sentence transformer to create embedding for knowledge. Similarly, the generated queries are passed to the sentence transformer to generate respective embeddings. Through empirical analysis, we found that the sentence transformer embeddings perform better compared to a learnable embedding layer with one-hot embeddings.
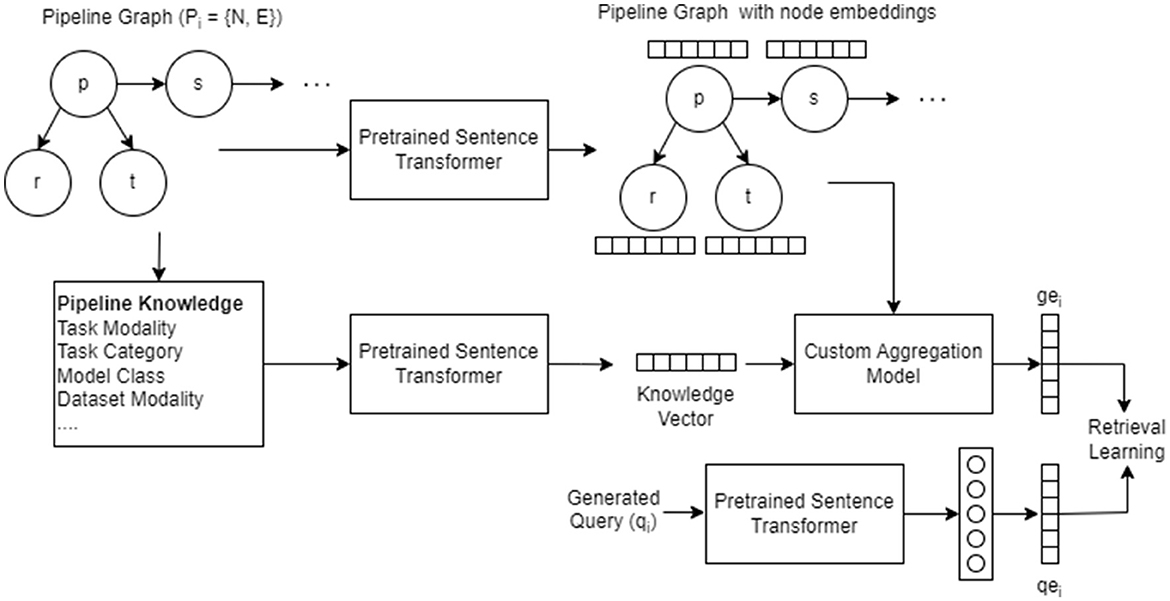
Figure 4. Architecture and workflow of the custom aggregation model utilized to learn the graph embedding.
Then, these embeddings are given as an input to the self-attention block (Algorithm 3) to generate an intermediate graph embedding of 1024-dimensional vector. Similarly, the embeddings generated for knowledge vector are also transformed into 1024-dimensional vector using a learnable fully connected layer. The learnt embeddings of the nodes and the knowledge vector are combined using a weighted sum to generate final graph embedding gei. We present the results of the model with and without knowledge embedding in Table 5. The embeddings generated for query vector using sentence transformer embedding are also transformed into 1024-dimensional vector to obtain qei. The objective function described in Section 4.2.5 trains the model to such that gei and qei are closer in the embedding space.
In the case of AIMKG, 1.6 million pipeline graphs follow the graph structure described by CMO (Section 3.2). To add, the textual information present in the nodes holds the most information compared to the graph structure. While the connectivity between models, datasets, tasks, and other nodes of the pipeline is essential to learning an appropriate graph embedding, graph-based models such as graph convolutional neural networks or graph attention neural networks prioritize learning graph topology compared to node features (Section 6.4). For this reason, a custom aggregation model was proposed to learn embedding for each pipeline graph. Table 5 shows the necessity of representing pipelines as a graph.
4.2.5 Objective function
To train the query embedding qei and the corresponding graph embedding gei to be closer in the embedding space, we use noise contrastive estimation (NCE) loss (Chen et al., 2020). NCE loss has the ability to normalize large probability distributions making it effective for scalable training datasets. The equation for NCE loss is as follows:
where N is the batch size, gei and qei are the embeddings for the i-th instance in the batch, and k is the number of negative samples which is N − 1 where i ≠ j.
5 Experimentation
In this section, we define evaluation metrics performed to test the robustness of AIMKG and recommendation ability of AIMKG.
5.1 AIMKG robustness
To evaluate the reliability of our knowledge graph construction, we employ a statistical technique called bootstrapping (Anirudh and Thiagarajan, 2019). We take a random sample of 75% of the data and utilize it to create a partial knowledge graph using our proposed approach (AIMKG). We repeat this process 10 times to generate 10 distinct knowledge graphs. A robust knowledge graph construction method should show low variance in node properties within partial graphs. We measure variance in node degrees and other distributional properties, comparing these with knowledge graphs built using the MLSchema ontology. The performance improvements are detailed in the result section.
5.2 Custom heuristics: qualitative analysis
Through user evaluation, we evaluate the ability of custom heuristic function to rank similar tasks for a given unknown task and return its associated pipelines. Due to the abscence of ground truth, we rely on domain experts to evaluate the relevance of results returned by the recommender. For comparison, a knowledge graph constructed using same data but using state-of-the-art MLSchema ontology is used. Using the custom heuristic function, the tasks in AIMKG and MLSchema-based KG are ranked and associated pipelines are returned to the domain experts for evaluation. The task nodes in AIMKG contain properties such as Name, Modality, Category, and Description. Modality and Category are computed using NLP techniques. In contrast, MLSchema-based task nodes only have properties such as Name, Description, and custom user-reported properties. Therefore, Smod and Scat from Equation 2 are always 0 for the recommender that uses MLSchema-based KG whereas cos(ei, ej) and J(Ti, Tj) are calculated using the same procedure. We configure the recommender to return the top-k relevant tasks and n pipelines for each task, where k and n are set to 3. We randomly select query tasks from various AI fields from AIMKG (Table 4) and drop the query task node to simulate unknown query task. This ensures the validity of the query task names. Eighteen domain experts aged between 24 and 50 participated in the evaluation study, each assigned 10 query tasks to determine the relevance of the recommendations provided.
5.3 Graph embedding learning
5.3.1 Implementation details
We utilize sentence transformer all-mpnet-base-v2 to generate the text encodings for node features, semantic properties (knowledge), and queries generated by ChatGPT. The default embedding size of 768 was used. Each of these text encodings are transformed into 1024-dimensional vector using a fully connected layer, one for knowledge vector and another for query encodings. We initialize the network with random weights for training. During training, for each pipeline, we randomly sample one query from the available generated queries. The batch size is set to 512. The Adam optimizer (Kingma and Ba, 2014) was used with learning rate 10-4 and weight decay set to 1e-5. We employ early stopping to prevent the model from overfitting and train it for several epochs until it converges.
5.3.2 Evaluation protocols
The custom aggregation model learns a common embedding space to retrieve a process graph given a natural language query. These can be considered two modalities of data, namely, graph and text. Therefore, we evaluate the custom aggregation model described in Section 4.2.4 using retrieval metrics reported by Salvador et al. (2017). For a given query embedding qei, we retrieve the k closest graph embeddings ge1…k using cosine similarity and present the results for k = 1, 3, and 5. We perform retrieval evaluation for 1,000 data samples and report results in Table 5. The definition of models reported is as follows:
• GCN: A graph convolutional neural network that takes pipeline graph Pi with node encodings Nei to generate gei obtained using global mean pool of learned node embeddings.
• GAT: A graph attention neural network that takes pipeline graph Pi with node encodings Nei to generate gei obtained using global mean pool of learned node embeddings.
• Sent_Trans: Use pretrained sentence transformer to generate gei using pipeline name and description. Use query text to generate qei. Both gei and qei are 768-dimensional vector as that is the default embedding size for sentence transformers.
• Sent_Trans_Finetune: Use qei and gei from Sent_Trans model and transform them into 1024-dimensional vector using a learnable fully connected layer.
• Custom_Agg: Model described in Algorithm 3 that takes in node encodings Nei for each pipeline graph Pi to learn graph embedding hei (equivalent of gei for this model).
• Custom_Agg_Knowledge: Model described in Algorithm 2. It takes the output from Custom_Agg hei and transformed knowledge vector kei to learn gei = α.hei + β.kei where α and β are learnable weights.
6 Result and discussion
6.1 AIMKG overview
The statistical overview of AIMKG can be found in Table 2. The AIMKG consisting of knowledge graphs KG1, KG2, and KG3 contains 8 million nodes and 25 million relationships in label property graph (LPG) format. There are ~78 million triples in RDF format which include the vector embeddings computed as properties. There are 11 types of nodes that represent each component of AI pipeline metadata and 13 types of relationships among those entities. Currently, the knowledge graph consists of 1.6 million AI pipelines executed for ~10 k tasks with ~53 k datasets and ~270k models. The knowledge graph is currently growing in size to include more pipelines and additional knowledge. A sample pipeline present in AIMKG is described in Supplementary material. The details of system maintenance and performance are also included in Supplementary material.
6.2 AIMKG robustness
The first row in Table 3 shows the results obtained from knowledge graph constructed using MLSchema ontology. It is evident that the variance in node degrees is higher compared to AIMKG. AIMKG demonstrates lower variance, confirming the robustness of the knowledge graph construction scheme. Furthermore, we observe that when using the MLSchema ontology, only 71% of the nodes are part of the largest connected component in the knowledge graph, while the remaining nodes are part of other disconnected components. In contrast, AIKMG includes 93% of the nodes in the largest connected component, indicating a more coherent graph structure for performing downstream tasks.
6.3 Relevant pipeline recommendation using custom heuristics
The results of user agreement on the relevance of recommendations provided for query tasks are summarized in Table 4. The goal is to return relevant pipelines for a given query of unknown task. For each areas such as computer vision, natural language processing, audio/speech, and video, 20 query tasks were evaluated. Due to limited number of pipelines in AIMKG, 10 queries were evaluated for multimodal and other areas that includes graphs, reasoning, and game-related learning. In total, 100 queries were evaluated for each recommender. According to domain experts, the recommender utilizing AIMKG achieved relevant results for 78% of the queries, while the MLSchema-based recommender had a lower success rate of 51%. The Cohen's kappa score computed for the subset (25%) of the queries was found to be 0.657, which is considered a substantial agreement between the domain experts on the recommendation relevance. The computed semantic properties utilized by the custom heuristic function (Equation 2) played a significant role in understanding task nature and capturing synonyms. For example, a query for Dialogue Interpretation returned Dialogue Understanding as a relevant task, showcasing the recommender's ability to recognize synonyms.
In computer vision queries, there was a notable difference in relevance scores due to significant number of challenging queries which did not explicitly mention the word “image.” For example, 3D object detection and 3D human pose estimation do not have the word image in it, but they are image-based tasks. Similarly, NLP-based tasks also benefited from semantic enhancements present in AIMKG. Video-based tasks are extensions of computer vision-based tasks that includes temporal factor. Therefore, like computer vision-based queries, a significant amount of video-based queries did not explicitly have the word “video” in it. Some examples include motion detection and human movement detection. The category other was challenging for both recommenders as the vocabulary curated for these areas is relatively small to identify modalities of these tasks.
When AIMKG is deployed as an open-source platform, it serves as a curated knowledge repository of open-source AI innovations that are searchable, discoverable, and executable. Users can search among 280 k models, 53 k datasets used for 10 k tasks at one place. It is an AI exploration and experimentation platform that hosts, serves, and refreshes state-of-the-art open-source AI innovations. This enables the reproduction of AI pipelines, including data preprocessing, pretraining, fine-tuning, and model deployment, which are impactful across various use cases. The broader practical impacts of AIMKG in fields such as healthcare, finance, and legal for pipeline optimization through relevant pipeline recommendation can be found in Supplementary material.
6.4 Relevant pipeline recommendation using graph learning
From Table 5, it can be observed that our Custom_Agg_Knowledge model performed the best and Custom_Agg performed second best against other baseline approaches. The difference is that the former model utilizes semantic properties computed for various pipeline graph component entities as described in Section 3.4.2. Furthermore, the proposed method demonstrated statistically significant improvements over baseline methods, confirmed by both Friedman's test (p < 0.01) and pairwise Wilcoxon signed-rank tests (p < 0.01). The results of sensitivity analysis and ablation study can be found in Supplementary material. GCN and GAT models weigh in more on learning the topological structure of the graphs compared to node features. In AIMKG, all pipeline graphs follow similar graph structure defined by CMO. To add, most information about the pipeline is present as text in the node features. Due to this, the text information gets diluted over graph structure in GCN and GAT models. As expected, these models have the least retrieval scores. The sentence transformer model was evaluated with and without fine-tuning to test whether pipeline descriptions (abstract, model-card) suffice for relevant retrieval. The fine-tuned model performed better for the HuggingFace and Combined datasets but not for Papers-with-Code, likely due to its detailed description on pipelines already present in abstract. Fine-tuning may have caused embedding instability for Papers-with-Code, while it improved accuracy for HuggingFace and the Combined dataset.
In summary, the Sent_Trans and Sent_Trans_Finetune results show that pipeline graphs are essential for effective retrieval, capturing relationships between datasets, models, tasks, and entities. Descriptions from Papers-with-Code and HuggingFace are limited. Traditional graph models such as GCN and GAT underperformed on AIMKG due to their focus on topology over node features. The proposed custom aggregation model, emphasizing node features, outperformed others with added knowledge-boosting results.
6.5 Pipeline optimization
In this section, we present the results on utilizing recommendations from AIMKG to seed the AI pipeline experimentations. Existing work Pedretti et al. (2023) demonstrated the use of novel in-memory accelerator engines to speed-up the inference of tree-based machine learning models for heterogeneous (tabular) data, the most widely used type of data across various industries. We employed seven widely used real-world tabular datasets for binary/multi-class classification and regression problems from research papers. In this section, we demonstrate the improvements in executing the hyperparameter optimization AI pipelines for gradient-boosted trees (XGBoost; Chen and Guestrin, 2016) on several binary and multi-class classification problems from that paper. Concretely, we collected results from hyperparameter optimization pipelines for four datasets (Eye Movement, Gas Concentration, Gesture Phase Segmentation, and Rossmann Stores Sales). We then imported the pipeline performance data into the AIMKG using one of the developed parses and asked it to recommend pipeline configurations for new, previously unseen, similar problems—churn modeling, telco customer churn, and forest cover type. We then used these recommendations from AIMKG to warm-up the Bayesian (TPE—tree-structured Parzen Estimators; Bergstra et al., 2011) hyperparameter optimization. We compared results with the reference results where no warm-up initialization was made. Table 6 shows three datasets. For each dataset, we report observed speed-up (wall time) to optimize hyperparameters of respective models to same or lower loss. In addition, we observed that the final loss was lower compared to experiments without warm-up initialization.
6.6 Additional attributes of AIMKG
6.6.1 Search using semantic enrichment and graph traversal
We illustrate the potential of AIMKG to perform complex queries that utilizes combination of custom heuristic functions in Section 4.1 and graph traversal to return desired results through Figure 5. We queried recommender to return datasets and models used for image detection task. Since this task does not exist in the repository, it identifies 2d-object detection and 3d object detection as similar tasks using the heuristic function in Equation 2. Even though the task names did not have explicit mention of the word “image,” they are identified as image-based tasks due to the semantic property modality. In addition, the recommender traverses the path from Task → Pipeline → Stage → Execution → Artifact → Dataset and Task → Pipeline → Stage → Execution → Artifact → Model to retrieve models and datasets. More sample queries and their results can be found at Venkataramanan (2023).
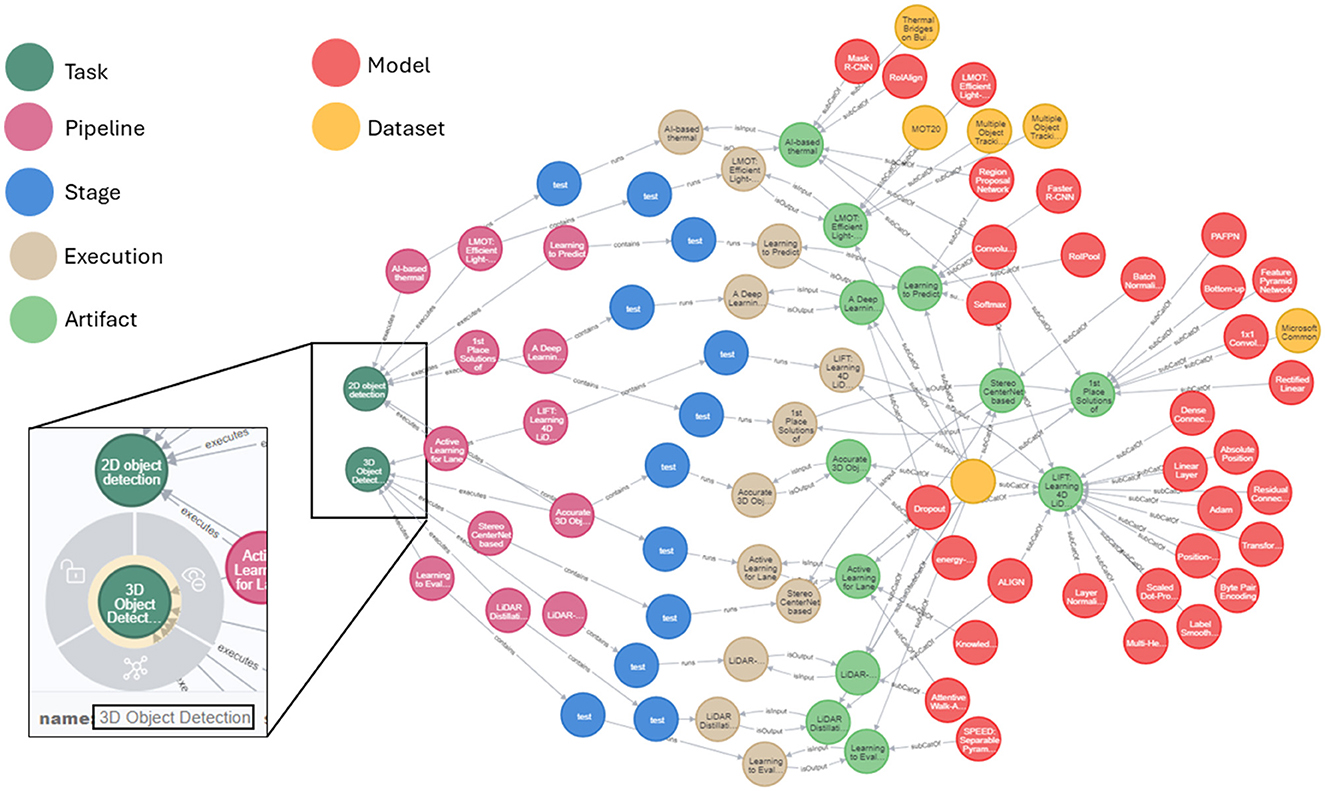
Figure 5. Sample query: list all the image detection pipelines with dataset and evaluations. The tasks “3d object detection” and “2d object detection” are returned by AIMKG even though no explicit mention of “image.” To add, the graph traversed from task to datasets and models to identify models and datasets used for image detection task. More sample queries of pipelines with hyperparameters can be found at Venkataramanan (2023).
6.6.2 Relevant pipeline recommendation using graph traversal
In addition to Equation 3, similar datasets can also be obtained through graph traversal as shown in Figure 6. The query is to return datasets similar to Awesome-chatgpt-prompts. Using the inference that if the datasets are used for the same task, they can be similar in certain aspects, we performed graph traversal query, and the resulting graph is shown in Figure 6. To perform the same query, that is to return similar datasets to a given dataset, other kinds of inferences can be used such as (i) if the datasets are used in the same pipeline, they can be considered; (ii) if the datasets are used in the same pipeline with same model, they can be considered similar and so on.
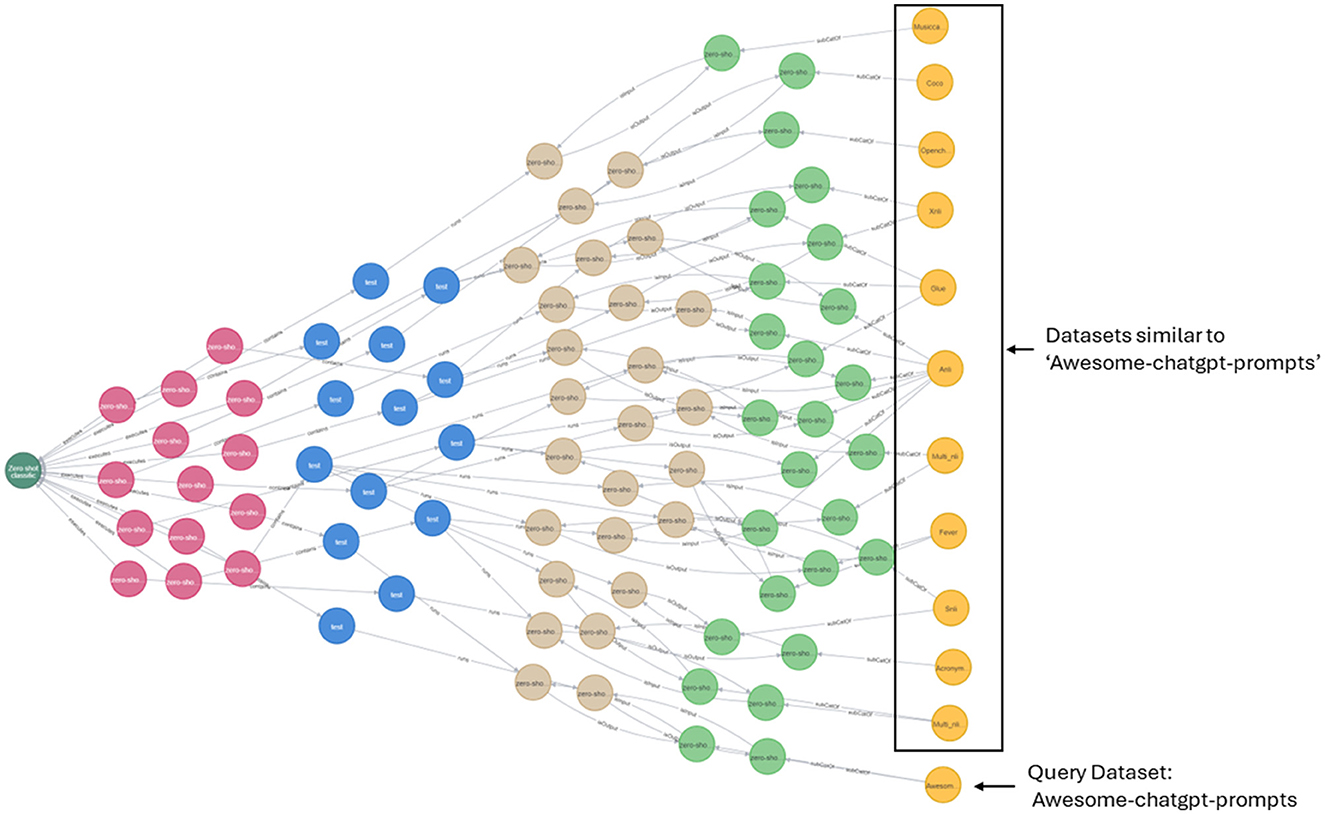
Figure 6. Query: identify datasets similar to Awesome-chatgpt-prompts. In this example, similar datasets were identified based on graph traversal. That is, if the datasets are used for the same task, they might be similar in certain aspects.
6.6.3 AIMKG: dynamic AI pipeline knowledge repository
AIMKG is a constantly evolving graph that updates itself periodically by fetching data from Papers-with-Code, OpenML, and HuggingFace. We are also working toward including other metadata sources mentioned in Table 1. This iterative process of periodic updates involves continuous monitoring, ensuring that the graph remains current and reflective of the evolving information landscape. Given that AI domain is ever changing with new models being introduced and manuscripts being published, it is imminent that AIMKG is live and dynamic. We demonstrate the importance of maintaining a live pipeline for AIMKG using the example described in Table 7.
We query AIMKG to return pipelines and models for the task Question Answering. Before the integration of most recent models from HuggingFace, AIMKG returned pipelines that were published in 2021 and 2020, respectively. Each of these pipelines used two models in their experimentation. When the same query was ran at a different timestamp, after integrating the most recent models, it returned bert-finetuned-squad and xlm-roberta-base-vn-dplat as the models used for Question Answering along with their pipelines. These models were published in 2023. The result from AIMKG now contains most recent models used for Question Answering. This self-updating mechanism not only enhances the graph's comprehensiveness but also ensures that it consistently serves as a reliable and up-to-date resource for users seeking the latest insights and connections within the represented domain. System maintenance and performance details are included in Supplementary material.
6.6.4 Integration of multiple data sources
As mentioned in Section 3, AIMKG consists of pipeline metadata obtained from multiple sources such as Papers-with-Code, OpenML, and HuggingFace. It is worth noting that Papers-with-Code and HuggingFace have information overlap to certain degree. While the overlap has been identified and unified, it has also helped in pipeline completion in certain cases. For example, in Figure 7, AIMKG had pipeline name and report from Papers-with-Code. For these pipelines, the model and dataset information is not available via Papers-with-Code API as they were not recorded by users explicitly. On the other hand, HuggingFace had model and dataset information for these pipelines. By utilizing paper arxiv ID and paper title, our AIMKG construction pipeline identified that these two are from the same pipeline by mapping them to CMO. While there are several such example, a few of them are included in Figure 7 to demonstrate the concept of integration of data sources that can aid in completion of pipeline metadata. Ontologies and knowledge graphs excel at the task of recognizing identical concepts present in various data sources. The ability of ontologies and knowledge graphs to discern shared meanings has enabled AIMKG to identify identical concepts from disparate data contexts.
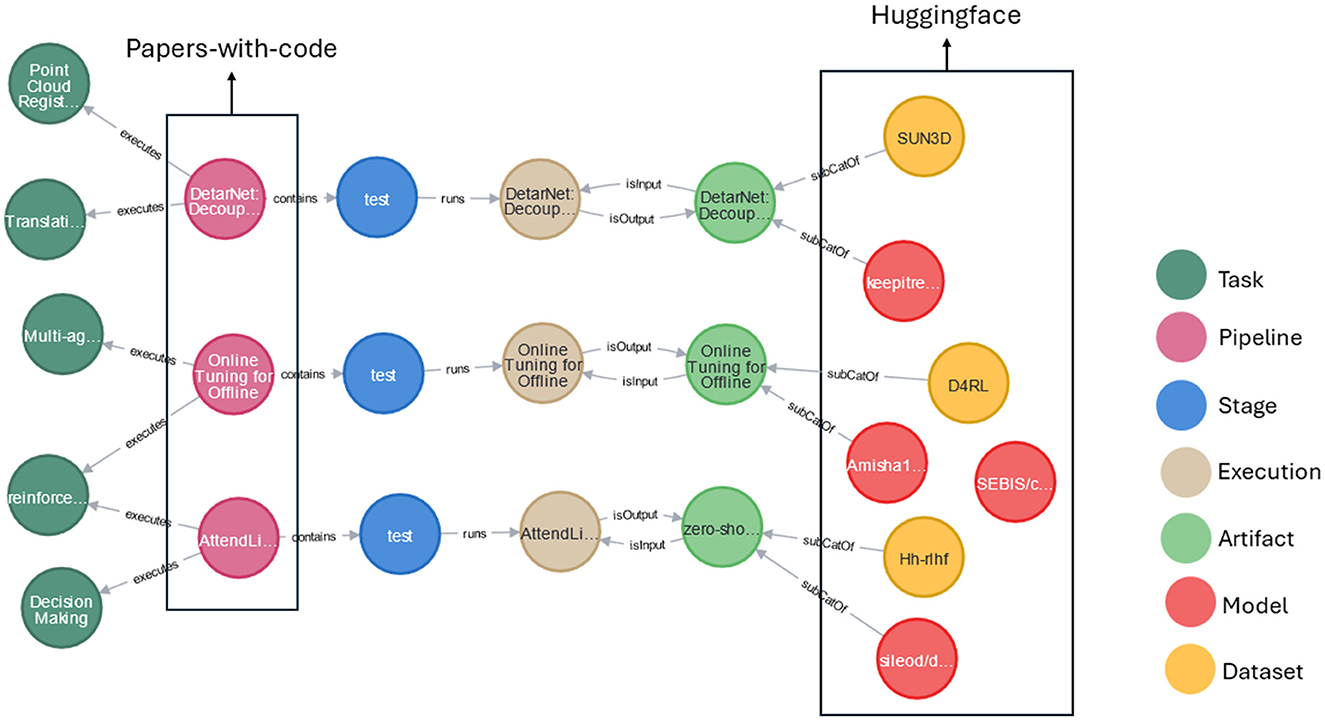
Figure 7. Sample of pipeline completion done by AIMKG by identifying identical concepts from Papers-with-Code and Huggingface.
6.7 Comparison with ChatGPT
As Large Language Models (LLMs) have been known to perform several tasks, we compared our AI pipeline recommendation task with ChatGPT-3.5 (OpenAI, 2022). We queried both our AIMKG recommender and ChatGPT to return pipelines executed for the task Supervised Anomaly Detection and reported the results in Table 8. Anomaly detection is the task of detecting data instances that significantly deviate from the majority of the data points (Pang et al., 2021). ChatGPT offers an interactive framework for querying, and it returns the models and their papers for common tasks such as 3D-aware image synthesis. The model seems to generate relevant information for familiar tasks from computer vision or natural language processing. However, the model seems to generate non-existent information for less familiar tasks such as anomaly detection or sequence-to-sequence speech generation. ChatGPT was prompted with sample query and sample expected response as mentioned in Table 8. The model generated similar response as given in the prompt. However, the pipelines returned by ChatGPT, in terms of papers, seem to be non-existent in literature. That is, ChatGPT exhibited instances of hallucination, generating information that does not exist in a convincingly realistic manner which is a well-known issue (Zuccon et al., 2023). In the response presented in Table 8, all of GitHub links return 404 when attempted to reach. For all the three recommended papers, the arxiv link is the same and it does not belong to any of the papers recommended. Paper 1 that was recommended with the title “DeepOne-Class: Outlier Detection with Deep Learning” does not exist. This means the model and dataset generated in the response are also in question. For paper 2 and paper 3, the titles are partially correct but papers with exact titles do not exist. Similarly, the datasets mentioned in paper 2 and paper 3 are not the datasets used in the actual paper. It is evident that the recommendations from ChatGPT are not reliable to reproduce or train an AI pipeline. More example responses along with explanations can be found in Supplementary material.
On the other hand, AIMKG returns a list of relevant pipelines for Supervised Anomaly Detection tasks as the exact match of the task is unavailable. For brevity, the responses currently include the paper and git repository from which the pipeline can be reproduced. However, AIMKG can also list associated datasets, models, and metrics for some of these pipelines. It is noteworthy that the first recommendation task, Weakly Supervised Defect Detection, did not explicitly mention the word anomaly. However, our recommender captured that defect detection is synonymous with anomaly detection in the domain of AI by just using a model pretrained for generic tasks. This also demonstrates the efficiency of embedding and semantic property-based ranking functions described in Equation 2. To add, the AIMKG recommender is explainable by design and the results are explainable.
To summarize, AIMKG produces relevant explainable results and also ensures the reproducibility of the recommended pipelines. While ChatGPT may respond with relevant models for familiar tasks, it hallucinates for many other cases, making it unreliable. Though ChatGPT has access to the data sources AIMKG is constructed with, it cannot construct an AI pipeline from the information available to it. Therefore, the construction of AIMKG enhanced with semantic knowledge is essential to recommend relevant pipelines to users.
7 Conclusion
In this study, we proposed Common Metadata Ontology (CMO) to construct an Artificial Intelligence pipeline Metadata Knowledge Graph (AIMKG), a first-of-its-kind knowledge graph for AI pipelines. AI pipeline metadata from open sources such as Papers-with-Code, OpenML, and HuggingFace are integrated to AIMKG, resulting in 1.6 million pipelines with semantic enhancements. The semantic enhancements incorporated in AIMKG capture implicit knowledge (Figure 5) and enhance reasoning capabilities. AIMKG can also store multimodal data types such as embeddings of task, dataset, model and pipeline nodes, supporting text, and numeric and vector data types. Using the computed semantic properties and embeddings, we introduced a custom heuristic ranking metric to rank relevant pipelines for recommendations using task, dataset, or model. The custom heuristic ranking function captured the underlying semantics of the pipeline entities, resulting in more relevant recommendations than the MLSchema-based recommender. The semantic properties also enhance search, as shown in Figure 5. To enable natural language queries for pipelines, we proposed a custom graph embedding aggregation model to retrieve and recommend relevant pipelines. We also demonstrated the potential of AIMKG in optimizing pipelines by seeding them with relevant recommendations. Therefore, AIMKG is an atlas for navigating the rapidly evolving artificial intelligence world.
Currently, not all tasks and datasets in AIMKG have computed semantic properties such as modalities and categories. To address this, we plan to leverage reports and manuscripts associated with pipelines to automatically compute these properties, reducing the biases associated with manually curated vocabularies. In addition, we intend to calculate further semantic and statistical properties for datasets and models, such as dataset image size, color scale, number of classes, data points per class, and model type. We aim to integrate metadata from other open-source repositories, such as Kaggle and the Common Metadata Framework (Koomthanam et al., 2024), into AIMKG. To enrich recommendations and ensure completeness for all 1.6 million pipelines, we plan to utilize fine-tuned language models for extracting information from research papers. Although community-driven sources such as Papers-with-Code, OpenML, and Hugging Face are widely used, they may contain metadata inaccuracies. To improve accuracy and reliability, we will implement robust metadata validation techniques (Soedarmadji et al., 2019; Aggour et al., 2017). In the future, we also envision interfacing AIMKG with large language models (LLMs), enabling users to query pipeline lineage, models, datasets, tasks, and other components through an interactive interface.
Data availability statement
The code repository for AI pipeline Metadata Knowledge Graph can be found at - https://github.com/HewlettPackard/ai-metadata-knowledge-graph. Further inquiries can be directed to the corresponding author.
Author contributions
RV: Conceptualization, Data curation, Formal analysis, Funding acquisition, Investigation, Methodology, Project administration, Resources, Software, Supervision, Validation, Visualization, Writing – original draft, Writing – review & editing. AT: Conceptualization, Data curation, Funding acquisition, Project administration, Resources, Supervision, Writing – review & editing. TK: Conceptualization, Investigation, Methodology, Supervision, Validation, Writing – original draft, Writing – review & editing. SS: Formal analysis, Investigation, Methodology, Resources, Supervision, Validation, Writing – original draft, Writing – review & editing. AJ: Data curation, Methodology, Project administration, Supervision, Writing – review & editing. ASha: Data curation, Resources, Validation, Writing – review & editing. SB: Conceptualization, Funding acquisition, Investigation, Methodology, Project administration, Resources, Supervision, Writing – review & editing. MF: Conceptualization, Funding acquisition, Project administration, Resources, Supervision, Validation, Writing – review & editing. PF: Funding acquisition, Project administration, Resources, Supervision, Writing – review & editing. KR: Conceptualization, Validation, Writing – review & editing. AShe: Project administration, Resources, Supervision, Writing – review & editing.
Funding
The author(s) declare financial support was received for the research, authorship, and/or publication of this article. This work was partly supported by National Science Foundation Award 2119654 “RII Track 2 FEC: Enabling Factory to Factory (F2F) Networking for Future Manufacturing.”
Acknowledgments
We thank the cloud services team at Hewlett Packard Labs for their continued support in providing GPUs and CPUs, crucial in hosting our graph and running evaluations. We also thank Hong Yung Yip who shared his expertise on knowledge graph construction. The authors acknowledge the use of ChatGPT-4, an AI language model developed by OpenAI, to assist in rephrasing text, checking grammar, and improving the overall clarity of the manuscript. The authors affirm that no original content was generated by the AI, and all ideas and conclusions are solely those of the authors.
Conflict of interest
RV, AT, TK, SS, AJ, AS, SB, MF, and PF were employed at Hewlett Packard Enterprise Labs.
The remaining authors declare that the research was conducted in the absence of any commercial or financial relationships that could be construed as a potential conflict of interest.
Publisher's note
All claims expressed in this article are solely those of the authors and do not necessarily represent those of their affiliated organizations, or those of the publisher, the editors and the reviewers. Any product that may be evaluated in this article, or claim that may be made by its manufacturer, is not guaranteed or endorsed by the publisher.
Author disclaimer
Any opinions, findings, and conclusions or recommendations expressed in this material are those of the authors and do not necessarily reflect the views of the National Science Foundation.
Supplementary material
The Supplementary Material for this article can be found online at: https://www.frontiersin.org/articles/10.3389/fdata.2024.1476506/full#supplementary-material
References
Abdelaziz, I., Dolby, J., McCusker, J., and Srinivas, K. (2021). “A toolkit for generating code knowledge graphs,” in Proceedings of the 11th on Knowledge Capture Conference, 137–144. Available at: https://dl.acm.org/doi/abs/10.1145/3460210.3493578
Achille, A., Lam, M., Tewari, R., Ravichandran, A., Maji, S., Fowlkes, C. C., et al. (2019). “Task2Vec: Task embedding for meta-learning,” in Proceedings of the IEEE/CVF International Conference on Computer Vision (Seoul), 6430–6439. Available at: https://openaccess.thecvf.com/content_ICCV_2019/html/Achille_Task2Vec_Task_Embedding_for_Meta-Learning_ICCV_2019_paper.html
Aggour, K. S., Williams, J. W., McHugh, J., and Kumar, V. S. (2017). Colt: concept lineage tool for data flow metadata capture and analysis. Proc. VLDB Endow. 10, 1790–1801. doi: 10.14778/3137765.3137783
Akhtar, M., Benjelloun, O., Conforti, C., Gijsbers, P., Giner-Miguelez, J., Jain, N., et al. (2024). “Croissant: a metadata format for ML-ready datasets,” in Proceedings of the Eighth Workshop on Data Management for End-to-End Machine Learning (Santiago), 1–6. Available at: https://dl.acm.org/doi/abs/10.1145/3650203.3663326
Anirudh, R., and Thiagarajan, J. J. (2019). “Bootstrapping graph convolutional neural networks for autism spectrum disorder classification,” in ICASSP 2019-2019 IEEE International Conference on Acoustics, Speech and Signal Processing (ICASSP) (Brighton: IEEE), 3197–3201.
Berahmand, K., Daneshfar, F., Salehi, E. S., Li, Y., and Xu, Y. (2024). Autoencoders and their applications in machine learning: a survey. Artif. Intell. Rev. 57:28. doi: 10.1007/s10462-023-10662-6
Bergstra, J., Bardenet, R., Bengio, Y., and Kégl, B. (2011). Algorithms forhyper-parameter optimization. Adv. Neural Inform. Process. Syst. 2011:24.
Brack, A., Hoppe, A., and Ewerth, R. (2021). “Citation recommendation for research papers via knowledge graphs,” in Linking Theory and Practice of Digital Libraries: 25th International Conference on Theory and Practice of Digital Libraries, TPDL 2021, Virtual Event, September 13–17, 2021, Proceedings 25 (Berlin: Springer), 165–174.
Chen, T., and Guestrin, C. (2016). “XGboost: a scalable tree boosting system,” in Proceedings of the 22nd ACM SIGKDD International Conference on Knowledge Discovery and Data Mining (Sanfrancisco), 785–794. Available at: https://dl.acm.org/doi/abs/10.1145/2939672.2939785
Chen, T., Kornblith, S., Norouzi, M., and Hinton, G. (2020). “A simple framework for contrastive learning of visual representations,” in International Conference on Machine Learning, 1597–1607. Available at: https://proceedings.mlr.press/v119/chen20j.html
Dessì, D., Osborne, F., Reforgiato Recupero, D., Buscaldi, D., Motta, E., and Sack, H. (2020). “AI-KG: an automatically generated knowledge graph of artificial intelligence,” in The Semantic Web–ISWC 2020: 19th International Semantic Web Conference, Athens, Greece, November 2–6, 2020, Proceedings, Part II 19 (Berlin: Springer), 127–143.
Elsken, T., Metzen, J. H., and Hutter, F. (2019). Neural architecture search: a survey. J. Machine Learn. Res. 20, 1997–2017. doi: 10.1007/978-3-030-05318-5_11
Färber, M., and Lamprecht, D. (2021). The data set knowledge graph: creating a linked open data source for data sets. Quant. Sci. Stud. 2, 1324–1355. doi: 10.1162/qss_a_00161
Färber, M., and Leisinger, A.-K. (2021). “Datahunter: a system for finding datasets based on scientific problem descriptions,” in Proceedings of the 15th ACM Conference on PWC Systems (Amsterdam), 749–752. Available at: https://dl.acm.org/doi/abs/10.1145/3460231.3478882?casa_token=gV35uoxEs_QAAAAA:vv7apDXE9B-qbS4ifhlfSPh6kDiERRFK70CDje2M_c6PW_LLWw_1BzCu9epm7gaotMNmcV3ac5jtiw
Guo, X., and Chen, Y. (2024). Generative AI for synthetic data generation: methods, challenges and the future. arXiv preprint arXiv:2403.04190. doi: 10.48550/arXiv.2403.04190
Guo, X., van Stein, B., and Bäck, T. (2019). “A new approach towards the combined algorithm selection and hyper-parameter optimization problem,” in 2019 IEEE Symposium Series on Computational Intelligence (SSCI) (Xiamen: IEEE), 2042–2049.
Hariharan, A., Zhang, T., Motz, M., and Weinhardt, C. (2024). “Accessible data lineage: a scoping review on open-source data lineage platforms,” in ICIS 2024 Proceedings, 5. Available at: https://aisel.aisnet.org/icis2024/data_soc/data_soc/5
He, X., Zhao, K., and Chu, X. (2021). AutoML: a survey of the state-of-the-art. Knowl. Bas. Syst. 212:106622. doi: 10.1016/j.knosys.2020.106622
Helali, M., Mansour, E., Abdelaziz, I., Dolby, J., and Srinivas, K. (2022). A scalable automl approach based on graph neural networks. Proc. VLDB Endow. 15, 2428–2436. doi: 10.14778/3551793.3551804
Humm, B. G., and Zender, A. (2021). “An ontology-based concept for meta automl,” in Artificial Intelligence Applications and Innovations: 17th IFIP WG 12.5 International Conference, AIAI 2021, Hersonissos, Crete, Greece, June 25–27, 2021, Proceedings 17 (Berlin: Springer), 117–128.
Jadon, A., and Kumar, S. (2023). “Leveraging generative ai models for synthetic data generation in healthcare: balancing research and privacy,” in 2023 International Conference on Smart Applications, Communications and Networking (SmartNets) (Istanbul: IEEE), 1–4. Available at: https://ieeexplore.ieee.org/abstract/document/10215825
Kannan, A. V., Fradkin, D., Akrotirianakis, I., Kulahcioglu, T., Canedo, A., Roy, A., et al. (2020). “Multimodal knowledge graph for deep learning papers and code,” in Proceedings of the 29th ACM International Conference on Information and Knowledge Management, 3417–3420. Available at: https://dl.acm.org/doi/abs/10.1145/3340531.3417439
Karmaker, S. K., Hassan, M. M., Smith, M. J., Xu, L., Zhai, C., and Veeramachaneni, K. (2021). Automl to date and beyond: challenges and opportunities. ACM Comput. Surv. 54, 1–36. doi: 10.1145/3470918
Kingma, D. P., and Ba, J. (2014). Adam: a method for stochastic optimization. arXiv preprint arXiv:1412.6980. doi: 10.48550/arXiv.1412.6980
Koomthanam, A. J., Tripathy, A., Serebryakov, S., Nayak, G., Foltin, M., and Bhattacharya, S. (2024). Common metadata framework: integrated framework for trustworthy artificial intelligence pipelines. IEEE Intern. Comput. 28, 37–44. doi: 10.1109/MIC.2024.3377170
Kumar, T., Shah, A., Mishra, A., Bhattacharya, S., Mahendran, A., Dunning, T., et al. (2023). “From roots to fruits: exploring lineage for dataset recommendations,” in Proceedings of the Second ACM Data Economy Workshop (Seattle, WA), 41–47. Available at: https://dl.acm.org/doi/abs/10.1145/3600046.3600053?casa_token=9KFjuOA11hkAAAAA:LC3fQhjgI_-szqmMM6T9wivHN6s0ByEWJ9hKWWbZorp6CGqELY_aoz_ruBOiXErJqrvcRrRQ4HCKLg
Mathew, A., Amudha, P., and Sivakumari, S. (2021). “Deep learning techniques: an overview,” in Advanced Machine Learning Technologies and Applications: Proceedings of AMLTA 2020, 599–608. Available online at: https://link.springer.com/chapter/10.1007/978-981-15-3383-9_54
Menghani, G. (2023). Efficient deep learning: a survey on making deep learning models smaller, faster, and better. ACM Comput. Surv. 55, 1–37. doi: 10.1145/3578938
Mohammadi, M., Tajik, E., Martinez-Maldonado, R., Sadiq, S., Tomaszewski, W., and Khosravi, H. (2024). Artificial Intelligence in Multimodal Learning Analytics: A Systematic Literature Review.
Müllner, P., Schmerda, S., Theiler, D., Lindstaedt, S., and Kowald, D. (2022). “Towards employing PWC systems for supporting data and algorithm sharing,” in Proceedings of the 1st International Workshop on Data Economy (Rome), 8–14. Available at: https://dl.acm.org/doi/abs/10.1145/3565011.3569055
Musto, C., Narducci, F., Lops, P., De Gemmis, M., and Semeraro, G. (2016). “Explod: a framework for explaining recommendations based on the linked open data cloud,” in Proceedings of the 10th ACM Conference on Recommender Systems (Boston, MA), 151–154. Available at: https://dl.acm.org/doi/abs/10.1145/2959100.2959173?casa_token=Xlgy_FvbnL8AAAAA:m_Eg7vQEsT2CXlGugie6lrjvGSzFqY3oq4CxlAsnsx1-Pr4Q-MMvt1hG0YzDLacw50IK9Pz1I1Pljg
Pang, G., Shen, C., Cao, L., and Hengel, A. V. D. (2021). Deep learning for anomaly detection: a review. ACM Comput. Surv. 54, 1–38. doi: 10.1145/3439950
Pedretti, G., Moon, J., Bruel, P., Serebryakov, S., Roth, R. M., Buonanno, L., et al. (2023). X-time: an in-memory engine for accelerating machine learning on tabular data with CAMs. arXiv preprint arXiv:2304.01285. doi: 10.48550/arXiv.2304.01285
Publio, G. C., Esteves, D., Ławrynowicz, A., Panov, P., Soldatova, L., Soru, T., et al. (2018). ML-schema: exposing the semantics of machine learning with schemas and ontologies. arXiv preprint arXiv:1807.05351. doi: 10.48550/arXiv.1807.05351
Salvador, A., Hynes, N., Aytar, Y., Marin, J., Ofli, F., Weber, I., et al. (2017). “Learning cross-modal embeddings for cooking recipes and food images,” in Proceedings of the IEEE Conference on Computer Vision and Pattern Recognition (Honolulu, HI), 3020–3028. Available at: https://openaccess.thecvf.com/content_cvpr_2017/html/Salvador_Learning_Cross-Modal_Embeddings_CVPR_2017_paper.html
SBERT Documentation (2023). SBERT Pretrained Models Documentation. Available at: https://www.sbert.net/docs/pretrained_models.html (accessed September 15, 2023).
Shen, Y., Song, K., Tan, X., Li, D., Lu, W., and Zhuang, Y. (2023). HuggingGPT: solving AI tasks with ChatGPT and its friends in huggingface. arXiv preprint arXiv:2303.17580. doi: 10.48550/arXiv.2303.17580
Shrestha, A., and Mahmood, A. (2019). Review of deep learning algorithms and architectures. IEEE Access 7, 53040–53065. doi: 10.1109/ACCESS.2019.2912200
Soedarmadji, E., Stein, H. S., Suram, S. K., Guevarra, D., and Gregoire, J. M. (2019). Tracking materials science data lineage to manage millions of materials experiments and analyses. NPJ Comput. Mater. 5:79. doi: 10.1038/s41524-019-0216-x
Thornton, C., Hutter, F., Hoos, H. H., and Leyton-Brown, K. (2013). “Auto-WEKA: combined selection and hyperparameter optimization of classification algorithms,” in Proceedings of the 19th ACM SIGKDD International Conference on Knowledge Discovery and Data Mining (Chicago, IL), 847–855. Available at: https://dl.acm.org/doi/abs/10.1145/2487575.2487629?casa_token=S3NSpj33lH8AAAAA:XOutkr8nKKkoiSIEQruB86E-ZRT2AlfaxWKcofLaDu_x9n9gTxEwRH1ZkXMfyLAroZBwwHfHJwFIoA
Vanschoren, J., Van Rijn, J. N., Bischl, B., and Torgo, L. (2014). OpenML: networked science in machine learning. ACM SIGKDD Expl. Newsl. 15, 49–60. doi: 10.1145/2641190.2641198
Venkataramanan, R. (2023). Construction of AIMKG. Available at: https://github.com/revathyramanan/ai-pipeline-recommender (accessed December 9, 2024).
Venkataramanan, R. (2024). Common Metadata Framework—Common Metadata Ontology. Available at: https://hewlettpackard.github.io/cmf/common-metadata-ontology/readme/ (accessed November 13, 2024).
Viswanathan, V., Gao, L., Wu, T., Liu, P., and Neubig, G. (2023). DataFinder: Scientific dataset recommendation from natural language descriptions. arXiv preprint arXiv:2305.16636. doi: 10.48550/arXiv.2305.16636
Zaharia, M., Chen, A., Davidson, A., Ghodsi, A., Hong, S. A., Konwinski, A., et al. (2018). Accelerating the machine learning lifecycle with mlflow. IEEE Data Eng. Bull. 41, 39–45.
Zuccon, G., Koopman, B., and Shaik, R. (2023). “ChatGPT hallucinates when attributing answers,” in Proceedings of the Annual International ACM SIGIR Conference on Research and Development in Information Retrieval in the Asia Pacific Region (Beijing), 46–51. https://dl.acm.org/doi/abs/10.1145/3624918.3625329?casa_token=iHqvsj9eDawAAAAA:NKqAHeJ_knPXKJ535R7dQ-I9rZnCnZmaN-BCsRqJmETLZHHPdv7UcvLGWu2dzJkc5Gd7QWnnhLA70Q
Keywords: AI pipeline metadata, graph learning, graph recommendation, AIMKG, metadata knowledge graphs, AI pipeline optimization
Citation: Venkataramanan R, Tripathy A, Kumar T, Serebryakov S, Justine A, Shah A, Bhattacharya S, Foltin M, Faraboschi P, Roy K and Sheth A (2025) Constructing a metadata knowledge graph as an atlas for demystifying AI pipeline optimization. Front. Big Data 7:1476506. doi: 10.3389/fdata.2024.1476506
Received: 05 August 2024; Accepted: 27 November 2024;
Published: 07 January 2025.
Edited by:
Suresh Kallam, Jain University, IndiaReviewed by:
Kamal Berahmand, Institute for Advanced Studies in Basic Sciences (IASBS), IranCristobal Rodolfo Guerra-Tamez, University of Monterrey, Mexico
Copyright © 2025 Venkataramanan, Tripathy, Kumar, Serebryakov, Justine, Shah, Bhattacharya, Foltin, Faraboschi, Roy and Sheth. This is an open-access article distributed under the terms of the Creative Commons Attribution License (CC BY). The use, distribution or reproduction in other forums is permitted, provided the original author(s) and the copyright owner(s) are credited and that the original publication in this journal is cited, in accordance with accepted academic practice. No use, distribution or reproduction is permitted which does not comply with these terms.
*Correspondence: Revathy Venkataramanan, cmV2YXRoeUBlbWFpbC5zYy5lZHU=