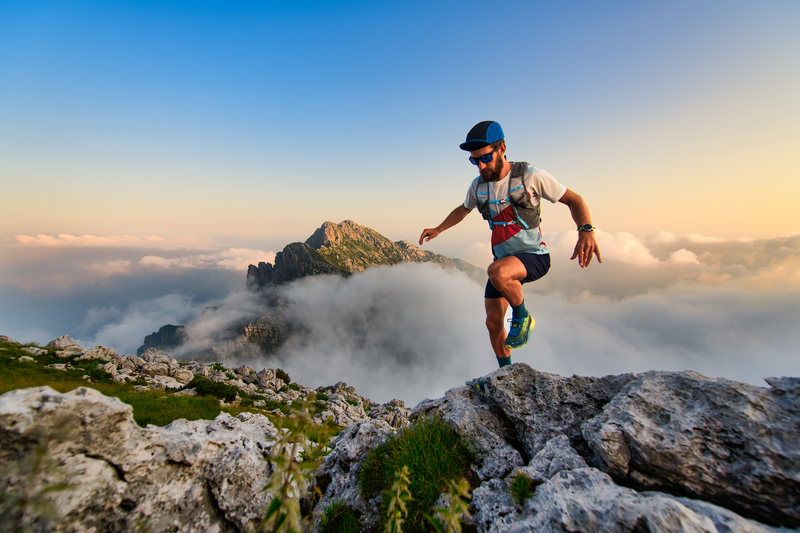
94% of researchers rate our articles as excellent or good
Learn more about the work of our research integrity team to safeguard the quality of each article we publish.
Find out more
ORIGINAL RESEARCH article
Front. Artif. Intell.
Sec. AI in Finance
Volume 8 - 2025 | doi: 10.3389/frai.2025.1566197
The final, formatted version of the article will be published soon.
You have multiple emails registered with Frontiers:
Please enter your email address:
If you already have an account, please login
You don't have a Frontiers account ? You can register here
There is a growing concern about the sustainability of artificial intelligence, in terms of Environmental, Social and Governance (ESG) factors. We contribute to the debate measuring the impact of ESG factors on one of the most relevant applications of AI in finance: credit rating. There is not yet conclusive evidence on whether EGS factors impact on credit rating. In this paper,we propose several machine learning models to measure such impact, and a set of metrics that can improve their ability to do so. In this way, machine learning models and, more generally, decisions based on artificial intelligence, can become more sustainable.
Keywords: Explainability, ESG scores, Credit ratings, gradient boosting, Shapley values
Received: 24 Jan 2025; Accepted: 17 Feb 2025.
Copyright: © 2025 Giudici and Wu. This is an open-access article distributed under the terms of the Creative Commons Attribution License (CC BY). The use, distribution or reproduction in other forums is permitted, provided the original author(s) or licensor are credited and that the original publication in this journal is cited, in accordance with accepted academic practice. No use, distribution or reproduction is permitted which does not comply with these terms.
* Correspondence:
Paolo Giudici, University of Pavia, Pavia, Italy
Disclaimer: All claims expressed in this article are solely those of the authors and do not necessarily represent those of their affiliated organizations, or those of the publisher, the editors and the reviewers. Any product that may be evaluated in this article or claim that may be made by its manufacturer is not guaranteed or endorsed by the publisher.
Research integrity at Frontiers
Learn more about the work of our research integrity team to safeguard the quality of each article we publish.