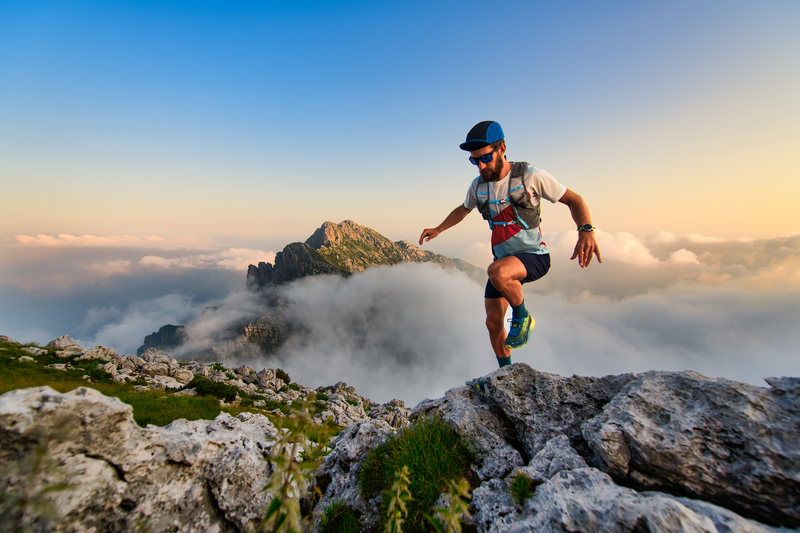
94% of researchers rate our articles as excellent or good
Learn more about the work of our research integrity team to safeguard the quality of each article we publish.
Find out more
ORIGINAL RESEARCH article
Front. Artif. Intell.
Sec. AI in Business
Volume 8 - 2025 | doi: 10.3389/frai.2025.1554325
This article is part of the Research Topic Advancing Knowledge-Based Economies and Societies through AI and Optimization: Innovations, Challenges, and Implications View all articles
The final, formatted version of the article will be published soon.
You have multiple emails registered with Frontiers:
Please enter your email address:
If you already have an account, please login
You don't have a Frontiers account ? You can register here
In business-to-business (B2B) companies, marketing and sales teams face significant challenges in identifying, qualifying, and prioritizing a large number of leads. Lead prioritization is a critical task for B2B organizations because it allows them to allocate resources more effectively, focus their sales force on the most viable and valuable opportunities, optimize their time spent qualifying leads, and maximize their B2B digital marketing strategies. This article addresses the topic by presenting a case study of a B2B software company's development of a lead scoring model based on data analytics and machine learning under the consumer theory approach. The model was developed using real lead data generated between January 2020 and April 2024, extracted from the company's CRM, which were analyzed and evaluated by fifteen classification algorithms, where the results in terms of accuracy and ROC AUC showed a superior performance of the Gradient Boosting Classifier over the other classifiers. At the same time, the feature importance analysis allowed the identification of features such as "source" and "lead status", which increased the accuracy of the conversion prediction. The developed model significantly improved the company's ability to identify high quality leads compared to the traditional methods used. This research confirms and complements existing theories related to understanding the application of consumer behavior theory and the application of machine learning in the development of B2B lead scoring models. This study also contributes to bridging the gap between marketers and data scientists in jointly understanding lead scoring as a critical activity because of its impact on overall marketing strategy performance and sales revenue performance in B2B organizations.
Keywords: Lead scoring, digital marketing, B2B sales, Business-to-business, Marketing automation, Lead qualification, Lead Conversion, CRM
Received: 01 Jan 2025; Accepted: 17 Feb 2025.
Copyright: © 2025 González Flores, Rubiano-Moreno and Sosa Gómez. This is an open-access article distributed under the terms of the Creative Commons Attribution License (CC BY). The use, distribution or reproduction in other forums is permitted, provided the original author(s) or licensor are credited and that the original publication in this journal is cited, in accordance with accepted academic practice. No use, distribution or reproduction is permitted which does not comply with these terms.
* Correspondence:
Guillermo Sosa Gómez, Facultad de Ciencias de la Salud, Universidad Panamericana, Mexico City, Mexico
Disclaimer: All claims expressed in this article are solely those of the authors and do not necessarily represent those of their affiliated organizations, or those of the publisher, the editors and the reviewers. Any product that may be evaluated in this article or claim that may be made by its manufacturer is not guaranteed or endorsed by the publisher.
Research integrity at Frontiers
Learn more about the work of our research integrity team to safeguard the quality of each article we publish.