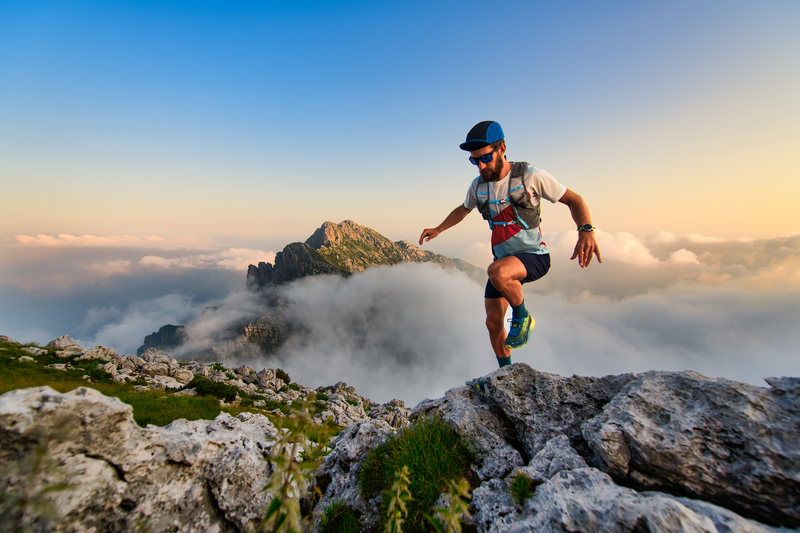
95% of researchers rate our articles as excellent or good
Learn more about the work of our research integrity team to safeguard the quality of each article we publish.
Find out more
ORIGINAL RESEARCH article
Front. Allergy , 15 August 2024
Sec. Asthma
Volume 5 - 2024 | https://doi.org/10.3389/falgy.2024.1451846
Introduction: Recent evidence has demonstrated that the microbiome is a driver of the underlying pathophysiological mechanisms of respiratory disease. Studies have indicated that bacterial metabolites produced in the gut and lung can impact lung inflammation and immune cell activity, affecting disease pathology. Despite asthma being a disease with marked sex differences, experimental work linking microbiomes and asthma has not considered the sex variable.
Methods: To test the hypothesis that the lung and gut microbial composition impacts allergic lung inflammation in a sex-specific manner, we evaluated lung and gut microbiome alterations in a mouse model of allergic inflammation and assessed their association with lung function and inflammation phenotypes. For this, we exposed male and female adult C57BL/6J mice intranasally to 25 µg of a house dust mite extract mix (HDM) daily, or phosphate-buffered saline (PBS) as control, for 5 weeks (n = 4–6/group). DNA from fecal pellets collected before and after the 5-week treatment, and from lung tissue collected at endpoint, was extracted using the ZymoBIOMICS®-96 MagBead DNA Kit and analyzed to determine the 16S microbiome via Targeted Metagenomic Sequencing.
Results: The HDM treatment induced a sex-specific allergic inflammation phenotype with significantly higher neutrophilia, lymphocytosis, inflammatory gene expression, and histopathological changes in females than males following exposure to HDM, but higher airway hyperresponsiveness (AHR) in males than females. In addition, sex-specific lung gene expression and associated pathways were identified HDM mix after challenge. These changes corresponded to sex-specific alterations in the gut microbiome, where the Firmicutes to Bacteroidetes ratio (F:B) was significantly reduced in fecal samples from only male mice after HDM challenge, and alpha diversity was increased in males, but decreased in females, after 5-weeks of HDM treatment.
Discussion: Overall, our findings indicate that intranasal allergen challenge triggers sex-specific changes in both gut and lung microbiomes, and induces sex-specific lung inflammation, AHR, and lung inflammatory gene expression pathways, suggesting a contribution of the lung-gut axis in allergic airway disease.
Asthma is a common respiratory disease, characterized by chronic airway inflammation and airflow limitation, that affects people at all life stages. It is a multifactorial disease that can result from interactions between genetic, environmental, and lifestyle factors (1), as well as epigenetic contributions (2). The prevalence of asthma has been increasing in recent decades. The most recent Global Burden of Disease (GBD) study by The Global Asthma Network estimated that asthma affected around 262 million people and that over 400,000 deaths resulted from the effects of the disease in 2019 alone (3). Notably, the prevalence and severity of asthma symptoms are reported to be greater among adult women when compared to men (4). Many clinical and basic science studies have attributed this disparity to the effect of circulating sex hormones on the physiological and immunological functions of the lung (5). The interaction between hormones and inflammation has been previously implicated in the pathogenesis of asthma (6). However, it remains unclear to what extent sex influences other mediators of respiratory disease and inflammation, such as the microbiome.
Pathophysiology research has suggested that the microbiome is a major driver of disease and illness. The microbiome is the trillions of microbes, including bacteria, viruses, and fungi, that reside in communities within and on the human body, providing metabolic and immune functions (7, 8). These microorganisms are highly influential on the health of their host, and alterations in the composition and diversity of the microbiome have been associated with a wide range of diseases; including autoimmune disorders, cancers, and lung diseases (9). These include tryptophan metabolites which can induce cytokine expression, causing the production of antibacterial peptides by acting as aryl hydrocarbon receptor ligands (10). Immunity and Intestinal barrier function can also be influenced through metabolism of short-chain fatty acids and bile acids (11).
Microbial profiling using the 16S microbiome is a common technique for studying microbial communities in various environments, including in the gut and respiratory tract (7). The 16S microbiome is based on sequencing the 16S ribosomal RNA gene, which is present in all bacteria and is highly conserved across species (12). The technique is used to identify and classify bacterial taxa based on their unique 16S rRNA sequences. Several studies have suggested that gut microbiome alterations may contribute to asthma development and exacerbation (13, 14). Growing evidence suggests that dysbiosis, or disruption, of gut microbiota, is an important contributor to the pathophysiology of inflammatory lung disease (15, 16). Bacteroidetes and Firmicutes are the predominant phyla of bacteria found in the gut microbial community; while Bacteroidetes, Firmicutes, and Actinobacteria are found to predominate in a healthy lung (17, 18). The gut and lungs are anatomically distinct, both organs have anatomical origins and complex pathways that reinforce the potential for the existence of the gut-lung axis (19, 20). Several microbiological and epidemiological studies report the contributions of the communities of the gut microbiome, in addition to the lung microbiome to the development of asthma in children. The gut-lung axis is the bidirectional communication between the gut and the lungs (21). It is an emerging field of research that seeks to understand the complex interactions between the gut microbiome, the mucosal immune system, and lung health. Much evidence suggests that bacterial metabolites produced in the gut can impact lung inflammation and immune cell activity and that the microbiome plays a role in the pathology of asthma, but the mechanisms involved are not clear. The mechanisms by which gut microbiota has been shown to regulate immune responses in the lungs are varied, including the “production of host-accessible metabolites, penetration of mucosal barriers by bacteria and/or toxins, regulation of hematopoiesis or circulating hormones and cytokines, and direct stimulation of migrating immune cells” (22).
Conversely, lung diseases can also affect the gut microbiome. For example, individuals with chronic obstructive pulmonary disease (COPD) also display altered gut microbiome composition and diversity, with an overgrowth of pathogenic bacteria, when compared to healthy individuals (15, 23). Overall, the gut-lung axis represents an important area of research in the respiratory medicine field. A better understanding of the interactions between the gut microbiome, the immune system, and lung health will contribute to the development of improved disease management and prevention strategies that can be used to decrease the global burden of asthma. The current study investigated the sex-specific effects of an environmental allergen challenge on the community and structure of the gut and lung microbiomes’ composition, diversity, and abundance using a mouse (C57BL/6J) model.
The animal study protocol (Protocol 21-012) was approved by Indiana University Bloomington's Institutional Animal Care and Use Committee (BIACUC). This study used an inbred strain, C57BL/6J, mouse model originally from Jackson Laboratories (Bar Harbor, ME), but bred and maintained in-house. Males and Females of 8–10-week-old littermates were randomly assigned (n = 4–6/group) to control or treatment groups. Those assigned to the experimental group were intranasally exposed to 25 µg of the allergen extract from house dust mite mix of D. pteronyssinus, and D. farinae mix (HDM) (Citeq biologics, Groningen, Netherlands) suspended in 50 µl of phosphate-buffered saline (PBS) daily (5 days/week) for 5 total weeks after a light anesthesia with 5% isoflurane using the SomnoSuite device (Kent Scientific) to induce phenotypic allergic airway inflammation, a physiologically relevant model to study mechanisms of inflammation similar to those occurring in some types of human asthma. Those assigned to the control group were intranasally exposed to 50 µl of PBS daily throughout the same treatment period.
Fecal pellet samples were collected from each mouse 1–3 days before the commencement of treatment to measure pre-exposure bacterial composition. Once treatment began, fecal pellet samples were collected from each mouse once weekly until the end of the five-week treatment period. Collections were labeled, weighed, and stored in 2 ml tubes at −80℃ until sent for processing. Fecal pellet samples collected during week 0 (pre-exposure) and week 5 (final exposure) were submitted to Zymo Research, to be processed with ZymoBIOMICSⓇ Service: Targeted Metagenomic Sequencing (Zymo Research, Irvine, CA). DNA was extracted to a 50 µl elution volume using ZymoBIOMICSⓇ-96 MagBead DNA Kit with ZymoBIOMICSⓇ Microbial Community Standard as a positive control. Afterward, the sample was prepared for targeted sequencing with Quick-16STM NGS Library Prep Kit using customized primers from the Quick-16STM Primer Set V3-V4. Final products from polymerase chain reactions (PCR) performed in real-time PCR machines were quantified with qPCR fluorescence readings and pooled together based on equal molarity to prepare a sequencing library. ZymoBIOMICSⓇ Microbial Community DNA Standard was used as a positive control for each target and negative controls were included. The final library was cleaned up using the Select a-Size DNA Clean & Concentrator™ then quantified with TapeStationⓇ (Agilent Technologies, Santa Clara, CA) and QubitⓇ (Thermo Fisher Scientific, Waltham, WA). The library was sequenced on IlluminaⓇ MiSeq™ with a v3 reagent kit (600 cycles). The sequencing was performed with a 10% PhiX spike-in.
At 72 h after the last exposure, Mice were anesthetized with intraperitoneal administration of Ketamine/xylazine and tracheostomized using an 18-gauge cannula, after which the mice were connected to the rodent ventilator system (flexiVent, SCIREQ, Montreal, Canada). To paralyze the mice muscle, we used pancuronium bromide (10 mg/g). The settings used to ventilate the animals include a positive end-expiratory pressure (PEEP) of 3cmH2O, a tidal volume of 10 ml/kg, and a maximum inflation pressure of 30cmH2O. We then administered Methacholine (MCh) (100 mg/ml) using a nebulizer. Resistance (Rrs), Elastance (Ers), Conducting airway resistance (Rn), Tissue resistance (G), and Tissue elastance (H) were determined after methacholine challenge in all the groups (HDM and PBS-treated groups).
Mice were tracheostomized and 1 mm EDTA/PBS solution was used to wash the lungs repeatedly until a solution of 2.5 ml was obtained from each mouse. BALF samples were centrifuged (1,300 rpm for 10 min). Cell pellets were resuspended in 500 ml of 1 mm PBS/EDTA, and 100 ul of the cell suspension was placed in a cytospin slide for differential cell counting using HEMA 3 staining (Fisher # 122-911 protocol HEMA 3 stain set). Cell differentials were counted at least 5 image frames at 20×magnification per slide by three investigators blinded to treatment.
For the lung microbiome study, whole lung tissues were collected from 8-to-10-week-old C57BL/6J male and female mice (n = 4/treatment group) upon sacrifice, and submitted to the University of North Carolina at Chapel Hill Microbiome Core Facility. The lung samples were then collected in a 2 ml tube containing 200 mg of 106/500 μm glass beads (Sigma, St. Louis, MO) and 0.5 ml of Qiagen PM1 (Valencia, CA) buffer was homogenized by mechanical lysis for 40 min. Following 5 min of centrifugation, 0.45 ml of supernatant was aspirated and then transferred into a new tube containing 0.15 ml of Qiagen IRS solution. The suspension was incubated at 4°C overnight. After another 5-minute centrifugation, the supernatant was aspirated and transferred to deep well plates containing 0.45 ml of Qiagen binding buffer supplemented with Qiagen ClearMag Beads. The DNA was purified using the automated KingFisherTM Flex Purification System and eluted in DNase-free water. Total genomic DNA was extracted using RNeasy® PowerMicrobiome® Kit (Qiagen, Valencia, CA) and purified using the automated KingFisher™ Flex Purification System. 16S rRNA gene fragments were amplified using primers F515/R806 to target the V4 region of the bacterial gene 1, 2 in addition to the use of 2× KAPA HiFi HotStart ReadyMix (KAPA Biosystems, Wilmington, MA). Each 16S amplicon was purified using the AMPure XP reagent (Beckman Coulter, Indianapolis, IN). The DNA was then denatured with NaOH, diluted with hybridization buffer, and heat-denatured before loading on the MiSeq reagent cartridge (Illumina, San Diego, CA) and on the MiSeq instrument (Illumina, San Diego, CA). Automated cluster generation and paired–end sequencing with dual reads were performed according to the manufacturer's instructions. Bioinformatics microbiome analyses were conducted as described below.
For lung histology, using another set of mice following the same treatment protocol, mice were anesthetized with ketamine and blood was collected to exsanguinate the animals for lung perfusion. Each mouse chest cavity was opened, the trachea cannulated and 5 µl formalin was placed in a syringe and allowed to perfuse in the lung by gravity. After which, the lung was fixed in formalin overnight. At 24 hrs, the lung was transferred into 70% ethanol solution, then each lobe of the lung was sectioned into half and stained with Alcian blue & Periodic Acid-Schiff (AL-BI-PAS), and Hematoxylin-eosin (HE- collagen). The histology slides were scored using the method used in the previous study (24). All tissues were examined by three investigators blinded to treatments.
Lung tissues were harvested and pulverized, and RNA was extracted using the Direct-zol RNA Miniprep plus kit (Zymo Research Corporation, Irvine, CA). A Nanodrop ND-2000 spectrophotometer (Thermo Scientific Wilmington, DE, USA) was used to analyze the concentration and quality of the RNA extracted in the different groups. Using targeted sequencing-based RNA expression, the mice RNA (n = 3/group) was analyzed, and the result was generated from TempO-Seq. assays (Arctoris Ltd. Oxfordshire, UK).
The QIAGEN Ingenuity Pathway Analysis (QIAGEN Inc., https://digitalinsights.qiagen.com/IPA) (25) was used to construct networks of DEGs in all the groups and to visualize the genes’ associations with canonical pathways implicated in asthma and lung inflammation.
For gut and lung microbiome analysis, unique amplicon sequences were inferred from raw reads using the Dada2 pipeline; additionally, chimeric sequences were removed using the Dada2 pipeline. Taxonomy assignments were performed using Uclust from Qiime v.1.9.1 with the internally designed and curated, Zymo Research Database, used as a reference. Alpha-diversity and beta-diversity analyses were performed with Qiime v.1.9.1. Sequencing output from the Illumina MiSeq PE250 platform was converted to fastq format and demultiplexed using Illumina Bcl2Fastq 2.18.0.12. The resulting reads were processed using QIIME 2. Index and linker primer sequences were trimmed, and the reads were processed with DADA2 through QIIME2 including merging paired ends, quality filtering, error correction, and chimera detection. Each read was then assigned to the GREENGENES database. Firmicutes/Bacteroidetes ratio (F: B) was calculated using GraphPad Prism version 9. The raw amplicon sequence variant (ASV) output from QIIME 2 was used to generate stacked taxa bar plots. Beta diversity analysis is displayed by Nonmetric Multidimensional Scaling (NMDS) plots, using Bray-Curtis distances between samples. This method calculates differences between samples based on the presence or abundance of bacterial ASVs, which is visualized as the distance between samples in the figure. The distances are then used to generate plots in N-dimensional space where samples with smaller distances between them are closer together and those with larger distances are further apart. Bray Curtis was chosen because it is sensitive to differences in relative abundances. The NMDS model then maneuvers the points to where there is the best fit between points being close enough and far enough away from each other. Those points are then given symbols based on their identity and overlaid with the other data to see how similar/different our clusters of samples are based on treatment, time, and sex, where closer clusters indicate more similar samples.
For AHR, immune cell counts, and lung histology, the average values of Rrs, Ers, Rn, G, and H from the methacholine challenge, alongside the prepared slides for both the immune cell analysis and lung histology were examined by three blind investigators and were collated, using the GraphPad prism 10.0.2. Data were expressed in mean ± SEM. 2-way ANOVA was used to compare the mean effect of sex and treatment in the different groups and results were statistically significant at a P-value of <0.05. For microbiome data, the raw ASV was used for permutational ANOVA (PERMANOVA), and ANOVA-like differential expression (ALDEx) analyses in R, using the phyloseq (26), genefilter (27), tidyverse (28), vegan (29), ANCOMBC (30), and ALDEx2 (31) packages, and for generating Bray-Curtis NMDS plots. Finally, for differential gene expression analysis, we used the DESeq2 package (version 1.40.2) in R/Bioconductor (R version 4.3.1) (32). Heatmaps were generated using the R Bioconductor package heatmap (version 1.0.12). Significant differentially expressed genes (DEGs) were determined using a P adj. <0.05. Venn diagrams were generated using the R package Venn Diagram (version 1.2.2). UpSet plots were generated using Complex Upset (version 1.3.3) and UpSetR (version 1.4.0).
AHR was assessed in both male and female mice treated with the HDM mix of D. pteronyssinus, and D. farinae via methacholine challenge (100 mg/ml). The average values of Rrs, Ers, Rn, G, and H from the methacholine challenge in all the groups were analyzed and the values were seen to increase in the HDM-treated groups compared to the PBS-treated groups at P value <0.05 (Figure 1) at the end of the study. Rrs, Rn, and G were significantly increased in the male HDM-treated group compared to the female group. However, Ers and H were not significantly different in the male and female HDM-treated groups.
Figure 1. Sex differences in airway hyperresponsiveness following 5 weeks HDM or PBS exposure. Assessing sex differences in airway hyperresponsiveness using the lung parameters, Total airway resistance (Rrs), Elastance (Ers), Tissue elastance (H), Conducting airway resistance (Rn), and Tissue damping (G) in male and female mice (C57BL/6J) after Methacholine (100 mg/ml) challenge following exposure to PBS or HDM. Data represented as mean ± SEM of at least 4-7 mice per treatment group. *P < 0.05; **P < 0.001, ***P < 0.0001, ****P < 0.00001.
BALF neutrophilia and lymphocytosis were observed in the HDM mix-treated groups, although these were significantly higher in female mice (Figure 2). No significant differences were found in the alveolar macrophages and eosinophil counts among the experimental groups.
Figure 2. Sex differences in immune cell population in the bronchoalveolar lavage fluid following 5 weeks HDM or PBS exposure. Assessing sex differences in the population of immune cells, macrophages, neutrophils, eosinophils, and lymphocytes in male and female mice (C57BL/6J) exposed to PBS or HDM. Data represented as mean ± SEM of at least 5-9 mice per treatment group. *P < 0.05; **P < 0.001.
Histopathological examination of lung tissues showed changes in peribronchial and perivascular inflammation, goblet cells, and hyperplasia in HDM mix-treated mice compared to the PBS-treated group (Figure 3). While peribrochial inflammation and goblet cells were higher in both male and female mice exposed to HDM, perivascular inflammation, inflammation scores, and hyperplasia were only significant in females exposed to HDM vs. PBS. Overall, the female group exposed to HDM displayed a stronger inflammatory phenotype than the male exposed group.
Figure 3. Sex differences in the histopathological analyses of lung tissue following 5 weeks HDM or PBS exposure. (A) Histological images of lung tissues stained with Hematoxylin and eosin (HE) or Alcian Blue and Periodic Acid Schiff (AB-PAS) stains to show the degree of peribronchial and perivascular inflammation, inflammation score, goblet cells, and hyperplasia. 10× magnification images showing lung tissues of PBS-treated and HDM-treated male and female (C57BL/6J) mice at 5 weeks and 72 h of the study (n = 4/group). (B) Graphs representing Slide scoring for each of the groups. Data represented as mean ± SEM of 4 mice per treatment group. *P < 0.05; **P < 0.001.
Using the Tempo-seq, a comprehensive gene expression analysis was performed in the lung samples of HDM-treated and PBS-treated male and female mice (Figures 4, 5). Overall, 2,865 differentially expressed genes (DEGs) were observed in the experimental groups. In PBS-treated mice, 43 DEGs were found in males vs. females at FDR <0.05, of which 40 were downregulated (top 10: Tnfrsf17, Oosp1, Ell3, Gm5128, Magedi, Nlgn3, Itpr1, Tcerg1, Ott, Sprr2a3), and only 3, Ddx3y, Eiy2s3yIY2S3Y and Tnnt2 were upregulated. In the HDM-treated mice, 1,075 DEGs were revealed in male vs. female mice, of which 200 were upregulated (top 10: Ddx3y, Eif2s3y, Klf2, Scnn1 g, Gsta3, Hba-a2, Cyp2b10, Etfb, Htra1, Rela) and 875 were downregulated (top 10: Prc1, Kif20a, Nlgn3, Kif2c, Stxbp1, Myh3, Il11, If135, Prune, Pogk) (Figures 4, 5). When comparing HDM-treated male mice over PBS-treated male mice, only 19 DEGs were revealed, 9 of them upregulated, Tnfrsf13b, Igha, Iglc1, Mmp14, Pik3c2b, Tnfrsf17, Sod1, Fasn, Itgb5, while 10 of them downregulated, Ugt1a5, Hp, Ywhaz, Vapb, Il13ra1, Igf1r, Me2, Ndufs2, Mcl1, Cul1. Interestingly, in the females, HDM-treated mice showed 834 DEGs (604 upregulated and 230 downregulated) vs. PBS-treated ones. Overall, between male and female mice, a total of 851 DEGs were found in HDM-treated over PBS-treated. Of these, 822 DEGs were in females (599 upregulated and 223 downregulated), and 7 DEGs (3 upregulated and 4 downregulated) in males. However, 12 DEGs (6 upregulated and 5 downregulated) were overlapped between the two groups, while only one gene (SOD1) was upregulated in males and downregulated in females.
Figure 4. Total genes upregulated or downregulated in the male and female mice following 5 weeks of HDM or PBS exposure. Venn diagram showing the number of unique and shared genes differentially expressed in the lungs of HDM-treated and PBS-treated male and female mice (n = 4/ treatment group).
Figure 5. Volcano plots showing sex differences in differentially expressed genes among groups. (A) Volcano plot showing differentially expressed genes (DEGs) in the HDM-treated over PBS-treated male mice (n = 4). (B) DEGs in the HDM-treated over the PBS- treated female mice (n = 4). (C) DEGs in male and female mice treated with PBS for 5 weeks (n = 4). (D) DEGs in HDM-treated male and female mice (n = 4).
Ingenuity pathway analysis (IPA) revealed that the top canonical pathways were B-cell activating factor signaling, Tnfs, PI3k/Akt signaling, Tr/Rxr activation, and estrogen receptor signaling between HDM and PBS-treated mice. Pd98059, wortmannin, growth hormone, Nr3c1, and Ly294002 were the top upstream regulators while Tcf7, Lrig1, Zm306416, Bibx1522, and Sema3d were the top casual network revealed in this group of animals. Interestingly, Immunological diseases and inflammatory responses were among the diseases and disorders associated with HDM-treated male mice over those treated with PBS. Most importantly, significant DEGs, related to inflammatory responses in this group, were Itgb5, Tnfrsf13b, Mmp14, Sod1, Igf1r, Mcl1, and Uwhaz.
One of the top canonical pathways revealed in HDM-treated female mice over PBS-treated was the Tp53 pathway. Rara, I-asparaginase sesaminol, Phox2a, Nr5a2, Tnf, Il25, Tp53, and IlL6 were the top upstream regulators. Organismal injury and abnormalities were part of the top diseases revealed to be associated with this group. Most importantly, the molecular and cellular functions revealed in this group were cell morphology cellular function/maintenance, and Pxp/Rxr activation. Significant DEGs related to inflammatory responses in this group were Cryba1, Dbh, Tnfrsf17, Trim71, P2ry6, Cerk, Ccl11, Cdk5r1, Il12b, Grn, and Npm1.
IPA also showed Tp53, Tnf, beta-estradiol lipopolysaccharide, and Tgfb1 as the top upstream regulators in the HDM-treated males over the HDM-treated females. Inflammatory response, Endocrine system disorders, and organismal injury/abnormalities were the top diseases and disorders associated with this group (Figure 6). Also, Smad4, Yap1, Pr0s1, Igfbp3, Sell, Sod1, Sqstm1, Vegfa, Ace, Vwf, Nfe2l2, Vim, Gadd45b, Ghr, Creg1, Rela, Ikbkg, Scp2, Ifngr1, Hsp90b1, Kdr, Tcf4, Bcl2li, Prkca were DEGs related to inflammatory responses that were significantly expressed in the male HDM-treated group vs. females.
Figure 6. Networks analysis predicted by IPA to be activated or inhibited in the HDM-treated males vs. females. Inflammatory response pathway shown to be inhibited in the male HDM-treated group over the female group. Blue lines represent predicted downregulation.
Among the 16 common microbial amplicon sequence variants (ASVs) that were selected as meeting a threshold of 5% abundance in at least one fecal pellet sample, Firmicutes occupied 63%, Actinobacteria 13%, Bacteroidetes 19%, and Verrucomicrobia 6%, of the microbial population in the gut. Among the 13 ASVs that were selected as meeting a threshold of 5% abundance in at least one fecal pellet sample, Phyla- Firmicutes occupied 46%, Proteobacteria, Bacteroidetes, and Actinobacteria occupied 15% each, while Fusobacteria occupied the remaining 8% of the microbial population in the lung. Interestingly, one taxon was found to be shared between the gut and lung microbiome and was present in all sample groups: “Actinobacteria; Actinobacteria; Bifidobacterium pseudolongum” as shown in Figure 7.
Figure 7. Stacked taxa bar plot of relative abundances of most common gut and lung microbial communities (A) ∼18 most common microbial amplicon sequence variants (ASV), selected if they met a threshold of 5% abundance in at least one fecal pellet sample, are displayed in different colors and aggregated together by sample identity (treatment, sex, and time). All other taxa falling below this threshold are grouped together into a ‘rare’ category labeled “<5% Abundance”. (B) Samples grouped by “Identity”, defined by their unique combinations of treatment/time, and sex. Each of the bars shows the abundance of named taxa found to be present at least 5% relative abundance in at least one sample of lung tissue. All other taxa falling below this threshold are aggregated into a single “rare” category and labeled “<5% Abundance” (n = 2–4/ treatment group).
The pre-exposure and after-exposure F:B in the gut and lungs of the HDM-challenged and control groups are shown in Figure 8. Pre-exposed males had an average gut F:B ratio of 2.88 while the females had an average gut F:B of 2.62. These are the presumed basal levels for each sex in this model. After treatment, the male gut F:B ratio decreased to 2.31 in the HDM-challenge group and increased to 3.00 in the control mice, while female gut F:B ratios decreased to 2.34 and 2.56, respectively following HDM and PBS challenges (Figure 8A) In the lungs, the male PBS group showed an F:B ratio close to 4.00, while all other groups had ratios between 2.00 and 3.00 (Figure 8B).
Figure 8. Firmicutes to Bacteroidetes ratio of 16S gut and lung microbiome among the groups. (A) The average ratio of Firmicutes to Bacteroidetes by absolute abundance found in the gut of male and female samples at week 0 and week 5 (HDM or PBS treatment). (B) The average ratio of Firmicutes to Bacteroidetes by absolute abundance found in the lungs of male and female samples after 5 weeks of treatment (HDM or PBS) (n = 2–4/ treatment group).
Alpha diversity analysis at week 0 revealed that males and females have similar median values of roughly 4.8 and 4.7, respectively, and that females also displayed a much larger variance (Figure 9). After 5 weeks of HDM challenge, the median value for males increased to about 5.0 with an extreme outlier on both high and low ends, while the median value for females decreased to 4.5. After 5 weeks the median value for PBS-treated males slightly decreased to about 4.7 with a large variance, while the median value for females increased to a value of roughly 5.1.
Figure 9. Shannon diversity Index plots of 16S gut and lung microbiome. Box-and-whisker plots depicting the diversity of bacteria in each fecal pellet sample (A) and each lung sample (B) grouped by identity variables (sex, treatment, and time). This indicates the number of different bacteria in each fecal pellet sample, while accounting for uniformity (n = 2–4/ treatment group).
Ordination for the fecal samples by beta diversity (Figure 10A) showed clustering of male baseline, while male week 5 HDM and PBS appear to cluster next to each other. From this analysis, it can be thus assumed that the difference in variation between week 0 and week 5 is leading to a significant time effect in the gut PERMANOVA tests. On the other hand, ordination for the lung samples (Figure 10B) showed that HDM treatment doesn't necessarily shift the community structure but rather causes samples to spread in this model. It can be assumed that the difference in variation between the treatments leads to a significant treatment effect in the lung's PERMANOVA tests. This illustrates microbiome dysbiosis in HDM-treated animals when compared to the tight, similar communities of the control (PBS) animals. As indicated in Supplementary Tables 1A,B, there was a non-significant effect of treatment on Bray-Curtis similarity between fecal pellet samples when baseline values are included and excluded (p = 0.071 and 0.303, respectively); meaning tha the different treatments (HDM or PBS) do not have significantly different microbial community structures, irrespective of sex or time. Considering both sex and treatment together (Supplementary Tables 1C,D), treatment was still not predictive of significantly different microbial communities with (p = 0.068) or without the baseline samples (p = 0.260). However, sex was significant in both cases (p = 0.047 and 0.018) accounting for differences in community structure between samples. Furthermore, when sex, treatment, and their interaction are considered (Supplementary Table 1E), sex alone significantly influences community similarity when baseline values are included (p = 0.047) and excluded (p = 0.015). Though, the sex by treatment interaction was not significant in either case (p = 0.071 and p = 0.153). Finally, the variable of time (i.e., week 0 to week 5) had a significant influence on community similarity (p = 0.033), as expressed in Supplementary Table 1G.
Figure 10. Bray-Curtis nonmetric multidimensional scaling (NMDS) plot of 16S gut and lung microbiome. Each point represents a separate fecal sample (A) and lung sample (B) Points have symbols representative of their identity (sex, treatment, time) and are overlaid with each other to form visual regions of clustering that represent the similarity/dissimilarity of the samples (confidence Level = 0.90; a = 0.20, N = 2–4/ group).
Conversely, PERMANOVA tests of lung tissue samples described a significant (p = 0.003) treatment effect on Bray-Curtis similarity between samples (Supplementary Table 2A); the different treatments resulted in significantly different microbial community structures, irrespective of sex or exposure. Sex (Supplementary Table 2B) was not found to be significant (p = 0.397), as was the treatment by sex interaction (p = 0.316); therefore, they do not explain differences in community structure between samples.
In this study, we examined the extent to which chronic exposure to a mix of D. pteronyssinus, and D. farinae HDM in a C57BL/6J mouse model caused sex-specific alterations to the gut and lung microbiomes. Male and female adult C57BL/6J mice were exposed intranasally to either an HDM mix allergen challenge or a PBS control challenge daily for 5 weeks (n = 4–6). A prior study observed that allergic airway disease phenotype was induced in female C67BL/6 mice after only 2 weeks, or 5 weeks, of exposure to HDM (33). This was evident by elevated eosinophilia, peribronchial and perivascular inflammation, and airway resistance, which reverted when treatment lasted for 11 weeks (33). More recently, Mostafa et al. reported sex- and strain-specific differences in eosinophilia, gene expression, and pro-inflammatory cytokine expression after only 2 weeks of exposure to HDM (34). In our 5-week model, we observed a severed AHR in male mice compared to the female mice. Similar models have demonstrated that chronic exposure to HDM causes varied respiratory system responses which are time and strain-dependent. For example, C57BL/6J shows higher resistance to AHR compared to other strains of mice and is attributed to their genetic makeup (35–38). Several studies have already reported that females are susceptible to airway inflammation following allergen exposure (39–45), while others have seen higher AHR in males than females (46–48). The greater response to methacholine challenge seen in the males was associated with differential responses in the airway smooth muscle (46, 48).
Sex dimorphism in HDM-mediated airway inflammation has also been reported. Most studies have reported greater infiltration of eosinophils in females than males exposed to allergen (36, 44). In this study, HDM-mediated neutrophil accumulation was greater in females than in males, suggesting that females had severed inflammation compared to males. Mostafa et al. reported higher HDM-eosinophils in males than in females and suggested that the immune cell population in the lungs following allergen exposure was strain-dependent (34). However, this claim was countered by the non-sex-specific study of Boucher et al., where a similar increase in the infiltration of inflammatory cells to the airway of two different strains of mice after HDM exposure was observed (49). Berndt et al. also observed a non-sex specific time-of-day dependent elevation in neutrophils in the acute HDM-exposed group compared to the control group (35). Male and female animals treated with lipopolysaccharide showed a higher neutrophil count compared with control, but no sex differences were observed (50, 51). In human studies, a non-sex specific neutrophilia was seen in the sputum and BALF of asthmatics (52, 53) which was hypothesized to be mediated by IL-17A (54). Interestingly, the ENFUMOSA cross-sectional studies have highlighted that men are four times less likely to suffer from chronic severe airway diseases than women (55). A recent study reported a similar finding, showing an increase in lymphocytes observed in the female HDM-treated group than in males (56). While Fuseini et al. reported a greater increase in lymphocytes, macrophages, eosinophils, and neutrophils in female BALB/C mice compared to males (57), we only observed a greater increase in neutrophils and lymphocytes in females. The differing outcomes may be due to the choice of strain, the concentration of the HDM used, and/or the duration of the protocol adopted to challenge or sensitize the animals.
Genes known to increase immune responses, Tnfrsf17, Trim71, Cerk, Ccl11, Il12b, and Grn (58–61), were upregulated in the HDM-treated female mice over the PBS-treated group, while only one gene, Npm1 (62), known to decrease immune response was upregulated in the same group. Interestingly, among the upstream regulators shown by IPA in the HDM-treated males over that of the females was Tp53, a gene implicated in the susceptibility to respiratory diseases (63). Tnf and beta-estradiol were inhibited in the male HDM-treated animals over the females. These two genes are positively related to neutrophilic-inflammation (64–66), suggesting reasons for the reduced inflammation observed in HDM-treated males. Vegf-a increased significantly in asthmatics (67, 68), and induced airway inflammation and remodeling in murine mice (69). Also, Hif-1α expression was increased after 1 and 5 weeks of exposing mice to allergens (70), whereas Hif-1α regulates the expression of the gene that encodes Vegf-a (71). One important limitation of this study is that all the data reported comes from sequencing of whole lung tissue homogenates rather than sorting out specific immune cells. Future studies should consider assessing cell-specific gene expression following challenge, as well as their associations with lung and gut microbiome changes.
Our analysis revealed that while the phyla Firmicutes and Bacteroidetes were predominant in the gut microbial community, in the lung microbial community the phyla that were predominant were Firmicutes, Proteobacteria, Bacteroidetes, and Actinobacteria. This was in line with prior reports from other researchers (17, 72, 73). The percentage of abundance of these microbial populations varied in the different groups. Previous studies found a varying composition in gut microbiome in early life due to diversity, complexity, and dominant bacterial taxa. Ege et al. named environmental exposures one major microbiome composition influencer (74). Actinobacteria has been known to colonize the lung in the early stage of life which is thought to protect the individual against allergy (75) and men are believed to possess it more than women which might account for the severe symptoms of asthma in women. Alaskha Alhamwe et al. observed an elevated proinflammatory immune response following repeated administration of Acinetobacter Iwoffi, proving the protective role of the bacteria on the immune system, although the sex of the animals used was not specified (76). Consistent with the previous study, in the gut microbial composition, we observed that the abundance of Actinobacteria was reduced in the male HDM and PBS group when compared to the male pre-exposure group (baseline 0week) whereas it was higher in the lungs of the male PBS group compared to the other groups. The abundance of Proteobacteria has been linked to the exacerbation of asthma (77), and it was higher in the lungs of the female HDM group compared to the other group. Chiu et al. found a reduction in the abundance of Firmicutes in children with asthma and related it to increased asthma risk (78). Interestingly, this agreed with our study as we found it lower in both male and female HDM-challenged groups when compared to the other groups. Recently, the probiotic species, Bifidobacterium was revealed as an anti-inflammatory promoter in clinical studies (79), and was found reduced in the male mice in both the PBS and HDM-challenged groups in this study. Furthermore, most studies have demonstrated that Bacteroidetes exhibit a pro-inflammatory effect, which may also contribute to the severity of asthma in women than in men, as it was found to be lower in male HDM and PBS groups compared to the female groups.
The Firmicutes/Bacteroidetes ratio is a measure of the abundance of the two major phyla of the gut, where an increase or decrease in the ratio is considered dysbiosis (80), it is significantly relevant in the microbial composition of human guts (81). In the gut of the male and female mice, this ratio was decreased following the HDM challenge when compared to the pre-exposure and PBS-challenged groups. In the lung, F: B was decreased in the male HDM vs. PBS group but not in the females. Previous studies reported that F: B evolves from early childhood to adulthood (82).
Alpha diversity refers to the diversity within a single microbial community, typically measured by calculating the number of different microbial taxa (species, genera, etc.) present and the relative abundance of each taxon (83). Alpha diversity is important because it can provide insights into the stability and resilience of a microbial community, as well as its functional capacity (84, 85). The Shannon Diversity Index plots in Figure 6, indicate the number of different bacteria in each fecal pellet sample while accounting for uniformity. The higher the value, the greater the community diversity (86). Bisgaard et al. reported that reduced bacterial diversity is inversely proportional to the risk of allergic sensitization at the first 6-years of life (87). Shannon index was used to measure bacterial diversity within the different samples in this study, which has also been used in similar research (88). We observed dysbiosis in this study, especially in the gut microbiome. The dysbiosis occurred with time, treatment, and sex, and this was associated with the development and exacerbation of allergic asthma (80). We found that the alpha diversity median value increased in the male HDM group and decreased in the female HDM-challenged mice. Interestingly, this observation was reversed in the PBS group.
Scientists have reported higher richness of lung microbiota diversity in asthmatics vs. non-asthmatic patients (13). While some have reported lower richness in the asthmatics compared to control subjects (89, 90), others reported no significant difference between these groups (91, 92). Beta diversity i.e., the differences in microbial community composition between different samples or environments has been used by other researchers (93, 94). These measures compare the presence and abundance of microbial taxa between samples and can provide insights into the factors driving microbial community differences, such as diet, host genetics, and environmental conditions (93, 94). In prior studies, beta diversity, not alpha diversity was significantly different between genders (95).
In summary, our study revealed sex differences in the gut microbial composition in the PBS and HDM-challenged groups, as well as sex-specific inflammatory phenotypes in response to allergic challenge. Researchers have observed sex disparities in the gut microbial composition both in clinical (96) and animal studies (97–100) following ozone exposure, and associated these with differential AHR and inflammatory phenotypes. The microbiome was shown to be sex-biased, and the females were highly diverse compared to the males at puberty (101) in an autoimmune disease context. Most sex-based studies have only considered the gut microbiome leaving the lung microbiome. Few studies, like the ovalbumin (OVA)-induced model of allergic airway inflammation research of Roggenbuck et al., reported a sex disparity in the microbial composition of the lung (102). The current study adds to our body of knowledge about the potential interaction of lung and gut microbiomes in mediating inflammatory and physiological responses to an HDM mix-induced allergic challenge in the lung.
In conclusion, our findings indicate that the gut and lung microbiome experience sex-specific changes in composition and structure due to chronic exposure to an environmental allergen. These may suggest a contribution of the lung-gut axis in mediating inflammatory mechanisms underlying asthma, and thus contribute to our understanding of sex-specific asthma phenotypes.
Sex disparity has been established in respiratory disease; hence it becomes important to highlight sex-specific changes in respiratory disease pathophysiology drivers such as microbiome to identify novel sex-specific therapeutics for disease management. This present study investigated the sex-based alterations in the gut and lung microbiome following allergen exposure. Our findings from this study revealed a sex-specific change in the gut microbial composition while, the lung microbial composition did not display sex-specific alterations and only experienced treatment-induced alterations. Further studies are needed to investigate other drivers of sex disparities in respiratory diseases such as the sex chromosomes and the sex hormones.
The datasets presented in this study can be found in online repositories. The names of the repository/repositories and accession number(s) can be found in the article/Supplementary Material.
The animal study was approved by BIACUC (Bloomington Institutional Animal Care and Use Committee). The study was conducted in accordance with the local legislation and institutional requirements.
CE: Data curation, Formal Analysis, Investigation, Methodology, Visualization, Writing – original draft, Writing – review & editing. RA: Formal Analysis, Investigation, Methodology, Visualization, Writing – original draft, Writing – review & editing. DR: Investigation, Methodology, Writing – original draft, Writing – review & editing. MB: Investigation, Methodology, Writing – original draft, Writing – review & editing. SS: Investigation, Methodology, Writing – original draft, Writing – review & editing. EP: Investigation, Methodology, Writing – original draft, Writing – review & editing. KD: Data curation, Methodology, Writing – original draft, Writing – review & editing. CH: Formal Analysis, Methodology, Visualization, Writing – original draft, Writing – review & editing. DR: Formal Analysis, Methodology, Visualization, Writing – original draft, Writing – review & editing. PS: Conceptualization, Data curation, Formal Analysis, Funding acquisition, Investigation, Methodology, Project administration, Resources, Software, Supervision, Validation, Visualization, Writing – original draft, Writing – review & editing.
The author(s) declare financial support was received for the research, authorship, and/or publication of this article.
This study was supported by the following grants: NIH/NHLBI, Grant/Award Number: R01HL159764 and R03HL141618 (to P.S), UNC Microbiome Core, Cancer Center Support Grant (P30 CA016086), Center for Gastrointestinal Biology and Disease (P30 DK34987), and UNC Center for Environmental Health and Susceptibility (P30 ES010126).
The authors thank Dr. M. Andrea Azcarate-Peril and Lilian Golzarri Arroyo for assistance with microbiome data analysis interpretation.
The authors declare that the research was conducted in the absence of any commercial or financial relationships that could be construed as a potential conflict of interest.
The author(s) declared that they were an editorial board member of Frontiers, at the time of submission. This had no impact on the peer review process and the final decision.
All claims expressed in this article are solely those of the authors and do not necessarily represent those of their affiliated organizations, or those of the publisher, the editors and the reviewers. Any product that may be evaluated in this article, or claim that may be made by its manufacturer, is not guaranteed or endorsed by the publisher.
The Supplementary Material for this article can be found online at: https://www.frontiersin.org/articles/10.3389/falgy.2024.1451846/full#supplementary-material
1. Thomsen SF. Genetics of asthma: an introduction for the clinician. Eur Clin Respir J. (2015) 2(1):24643. doi: 10.3402/ecrj.v2.24643
2. Potaczek DP, Harb H, Michel S, Alhamwe BA, Renz H, Tost J. Epigenetics and allergy: from basic mechanisms to clinical applications. Epigenomics. (2017) 9(4):539–71. doi: 10.2217/epi-2016-0162
3. Global Asthma Network. The global asthma report 2022. Int J Tuberc Lung Dis. (2022) 26(Supp 1):1–104. doi: 10.5588/ijtld.22.1010
4. Pate CA, Zahran HS, Qin X, Johnson C, Hummelman E, Malilay J. Asthma surveillance - United States, 2006–2018. MMWR Surveill Summ. (2021) 70(5):1–32. doi: 10.15585/mmwr.ss7005a1
5. Fuentes N, Silveyra P. Endocrine regulation of lung disease and inflammation. Exp Biol Med. (2018) 243(17-18):1313–22. doi: 10.1177/1535370218816653
6. Tesse R, Schieck M, Kabesch M. Asthma and endocrine disorders: shared mechanisms and genetic pleiotropy. Mol Cell Endocrinol. (2011) 333(2):103–11. doi: 10.1016/j.mce.2010.11.032
7. Hamady M, Knight R. Microbial community profiling for human microbiome projects: tools, techniques, and challenges. Genome Res. (2009) 19(7):1141–52. doi: 10.1101/gr.085464.108
8. Ursell LK, Metcalf JL, Parfrey LW, Knight R. Defining the human microbiome. Nutr Rev. (2012) 70(suppl_1):S38–44. doi: 10.1111/j.1753-4887.2012.00493.x
9. Ogunrinola GA, Oyewale JO, Oshamika OO, Olasehinde GI. The human microbiome and its impacts on health. Int J Microbiol. (2020) 2020:8045646. doi: 10.1155/2020/8045646
10. Gao J, Xu K, Liu H, Liu G, Bai M, Peng C, et al. Impact of the gut microbiota on intestinal immunity mediated by tryptophan metabolism. Front Cell Infect Microbiol. (2018) 8:13. doi: 10.3389/fcimb.2018.00013
11. Lee-Sarwar KA, Lasky-Su J, Kelly RS, Litonjua AA, Weiss ST. Gut microbial-derived metabolomics of asthma. Metabolites. (2020) 10(3):97. doi: 10.3390/metabo10030097
12. Ames NJ, Ranucci A, Moriyama B, Wallen GR. The human microbiome and understanding the 16S rRNA gene in translational nursing science. Nurs Res. (2017) 66(2):184–97. doi: 10.1097/nnr.0000000000000212
13. Huang C, Yu Y, Du W, Liu Y, Dai R, Tang W, et al. Fungal and bacterial microbiome dysbiosis and imbalance of trans-kingdom network in asthma. Clin Transl Allergy. (2020) 10:1–13. doi: 10.1186/s13601-020-00345-8
14. Wang Z, Lai Z, Zhang X, Huang P, Xie J, Jiang Q, et al. Altered gut microbiome compositions are associated with the severity of asthma. J Thorac Dis. (2021) 13(7):4322–38. doi: 10.21037/jtd-20-2189
15. Bowerman KL, Rehman SF, Vaughan A, Lachner N, Budden KF, Kim RY, et al. Disease-associated gut microbiome and metabolome changes in patients with chronic obstructive pulmonary disease. Nat Commun. (2020) 11(1):5886. doi: 10.1038/s41467-020-19701-0
16. Chunxi L, Haiyue L, Yanxia L, Jianbing P, Jin S. The gut microbiota and respiratory diseases: new evidence. J Immunol Res. (2020) 2020:2340670. doi: 10.1155/2020/2340670
17. Hufnagl K, Pali-Schöll I, Roth-Walter F, Jensen-Jarolim E. Dysbiosis of the gut and lung microbiome has a role in asthma. Semin Immunopathol. (2020) 42(1):75–93. doi: 10.1007/s00281-019-00775-y
18. Zhang Q, Cox M, Liang Z, Brinkmann F, Cardenas PA, Duff R, et al. Airway Microbiota in severe asthma and relationship to asthma severity and phenotypes. PLoS One. (2016) 11(4):e0152724. doi: 10.1371/journal.pone.0152724
19. Enaud R, Prevel R, Ciarlo E, Beaufils F, Wieërs G, Guery B, et al. The gut-lung axis in health and respiratory diseases: a place for inter-organ and inter-kingdom crosstalks. Front Cell Infect Microbiol. (2020) 10. doi: 10.3389/fcimb.2020.00009
20. Mjösberg J, Rao A. Lung inflammation originating in the gut. Science. (2018) 359(6371):36–7. doi: 10.1126/science.aar4301
21. Marsland BJ, Trompette A, Gollwitzer ES. The gut-lung axis in respiratory disease. Ann Am Thorac Soc. (2015) 12(Suppl 2):S150–156. doi: 10.1513/AnnalsATS.201503-133AW
22. Alemao CA, Budden KF, Gomez HM, Rehman SF, Marshall JE, Shukla SD, et al. Impact of diet and the bacterial microbiome on the mucous barrier and immune disorders. Allergy. (2021) 76(3):714–34. doi: 10.1111/all.14548
23. Zhang L, Lu J, Liu C. Akkermansia muciniphiladoi: ameliorates lung injury in smoke-induced COPD mice by IL-17 and autophagy. Cell Microbiol. (2023) 2023:4091825. doi: 10.1155/2023/4091825
24. Ashcroft T, Simpson JM, Timbrell V. Simple method of estimating severity of pulmonary fibrosis on a numerical scale. J Clin Pathol. (1988) 41(4):467–70. doi: 10.1136/jcp.41.4.467
25. Krämer A, Green J, Pollard J Jr, Tugendreich S. Causal analysis approaches in ingenuity pathway analysis. Bioinformatics. (2013) 30(4):523–30. doi: 10.1093/bioinformatics/btt703
26. McMurdie PJ, Holmes S. Phyloseq: an R package for reproducible interactive analysis and graphics of microbiome census data. PLoS One. (2013) 8(4):e61217. doi: 10.1371/journal.pone.0061217
27. Gentleman R, Carey V, Huber W, Hahne F. Genefilter: Methods for Filtering Genes from High-Throughput Experiments. R Package Version 1.82.1. Bioconductor. (2023). doi: 10.18129/B9.bioc.genefilter
28. Wickham H, Averick M, Bryan J, Chang W, McGowan LD, François R, et al. Welcome to the tidyverse. Journal of Open Source Software. (2019) 4(43):1686. doi: 10.21105/joss.01686
29. Oksanen J, Simpson G, Blanchet F, Kindt R, Legendre P, Minchin P, et al. Vegan: Community Ecology Package. R Package Version 2.6-4 (2022). Available online at: https://CRAN.R-project.org/package=vegan
30. Lin H, Peddada SD. Analysis of compositions of microbiomes with bias correction. Nat Commun. (2020) 11(1):1–11. doi: 10.1038/s41467-020-17041-7
31. Fernandes AD, Macklaim JM, Linn TG, Reid G, Gloor GB. ANOVA-like differential gene expression analysis of single-organism and meta-RNA-Seq. PLoS ONE. (2013) 8(7):e67019. doi: 10.1371/journal.pone.0067019
32. Love MI, Huber W, Anders S. Moderated estimation of fold change and dispersion for RNA-Seq data with DESeq2. Genome Biol. (2014) 15(12):1–21. doi: 10.1186/s13059-014-0550-8
33. Bracken SJ, Adami AJ, Szczepanek SM, Ehsan M, Natarajan P, Guernsey LA, et al. Long-term exposure to house dust mite leads to the suppression of allergic airway disease despite persistent lung inflammation. Int Arch Allergy Immunol. (2015) 166(4):243–58. doi: 10.1159/000381058
34. Mostafa DH, Hemshekhar M, Piyadasa H, Altieri A, Halayko AJ, Pascoe CD, et al. Characterization of sex-related differences in allergen house dust mite-challenged airway inflammation, in two different strains of mice. Sci Rep. (2022) 12(1):20837. doi: 10.1038/s41598-022-25327-7
35. Berndt A, Leme AS, Williams LK, Smith RV, Savage HS, Stearns TM, et al. Comparison of unrestrained plethysmography and forced oscillation for identifying genetic variability of airway responsiveness in inbred mice. Physiol Genomics. (2011) 43(1):1–11. doi: 10.1152/physiolgenomics.00108.2010
36. Blacquiere M, Hylkema M, Postma D, Geerlings M, Timens W, Melgert B. Airway inflammation and remodeling in two mouse models of asthma: comparison of males and females. Int Arch Allergy Appl Immunol. (2010) 153(2):173–81. doi: 10.1159/000312635
37. Reinhard C, Eder G, Fuchs H, Ziesenis A, Heyder J, Schulz H. Inbred strain variation in lung function. Mamm Genome. (2002) 13:8. doi: 10.1007/s00335-002-3005-6
38. Srinivasan A, Giri A, Duraisamy SK, Alsup A, Castro M, Sundar IK. Acute HDM exposure shows time-of-day and sex-based differences in the severity of lung inflammation and circadian clock disruption. J Allergy Clin Immunol Glob. (2023) 2(4):100155. doi: 10.1016/j.jacig.2023.100155
39. Corteling R, Trifilieff A. Gender comparison in a murine model of allergen-driven airway inflammation and the response to budesonide treatment. BMC Pharmacol. (2004) 4:4. doi: 10.1186/1471-2210-4-4
40. Hayashi T, Adachi Y, Hasegawa K, Morimoto M. Less sensitivity for late airway inflammation in males than females in BALB/c mice. Scand J Immunol. (2003) 57:562–7. doi: 10.1046/j.1365-3083.2003.01269.x
41. Melgert B, Postma D, Kuipers I, Geerlings M, Luinge M, Van Der Strate B, et al. Female mice are more susceptible to the development of allergic airway inflammation than male mice. Clin Exp Allergy. (2005) 35(11):1496–503. doi: 10.1111/j.1365-2222.2005.02362.x
42. E-Lacerda RR, Anhê GF, Page CP, Riffo-Vasquez Y. Sex differences in the influence of obesity on a murine model of allergic lung inflammation. Clin Exp Allergy. (2020) 50(2):256–66. doi: 10.1111/cea.13541
43. Schaefer AL, Ceesay M, Leier JA, Tesch J, Wisenden BD, Pandey S. Factors contributing to sex differences in mice inhaling aspergillus fumigatus. Int J Environ Res Public Health. (2020) 17(23):8851. doi: 10.3390/ijerph17238851
44. Takeda M, Tanabe M, Ito W, Ueki S, Konnno Y, Chihara M, et al. Gender difference in allergic airway remodelling and immunoglobulin production in mouse model of asthma. Respirology. (2013) 18(5):797–806. doi: 10.1111/resp.12078
45. Wang C, Xu Z-B, Peng Y-Q, Zhang H-Y, Yu Q-N, Guo Y-B, et al. Sex differences in group 2 innate lymphoid cell-dominant allergic airway inflammation. Mol Immunol. (2020) 128:89–97. doi: 10.1016/j.molimm.2020.09.019
46. Boucher M, Henry C, Khadangi F, Dufour-Mailhot A, Tremblay-Pitre S, Fereydoonzad L, et al. Effects of airway smooth muscle contraction and inflammation on lung tissue compliance. Am J Physiol Lung Cell Mol Physiol. (2022) 322(2):L294–l304. doi: 10.1152/ajplung.00384.2021
47. Card JW, Carey MA, Bradbury JA, DeGraff LM, Morgan DL, Moorman MP, et al. Gender differences in murine airway responsiveness and lipopolysaccharide-induced inflammation. J Immunol. (2006) 177(1):621–30. doi: 10.4049/jimmunol.177.1.621
48. Gill R, Rojas-Ruiz A, Boucher M, Henry C, Bossé Y. More airway smooth muscle in males versus females in a mouse model of asthma: a blessing in disguise? Exp Physiol. (2023) 108(8):1080–91. doi: 10.1113/ep091236
49. Boucher M, Henry C, Dufour-Mailhot A, Khadangi F, Bossé Y. Smooth muscle hypocontractility and airway normoresponsiveness in a mouse model of pulmonary allergic inflammation. Front Physiol. (2021) 12:698019. doi: 10.3389/fphys.2021.698019
50. Nelson AJ, Roy SK, Warren K, Janike K, Thiele GM, Mikuls TR, et al. Sex differences impact the lung-bone inflammatory response to repetitive inhalant lipopolysaccharide exposures in mice. J Immunotoxicol. (2018) 15(1):73–81. doi: 10.1080/1547691X.2018.1460425
51. Warren KJ, Sweeter JM, Pavlik JA, Nelson AJ, Devasure JM, Dickinson JD, et al. Sex differences in activation of lung-related type 2 innate lymphoid cells in experimental asthma. Ann Allergy Asthma Immunol. (2017) 118(2):233–4. doi: 10.1016/j.anai.2016.11.011
52. Hastie AT, Mauger DT, Denlinger LC, Coverstone A, Castro M, Erzurum S, et al. Baseline sputum eosinophil+ neutrophil subgroups’ clinical characteristics and longitudinal trajectories for NHLBI severe asthma research program (SARP 3) cohort. J Allergy Clin Immunol. (2020) 146(1):222–6. doi: 10.1016/j.jaci.2020.01.039
53. Hudey SN, Ledford DK, Cardet JC. Mechanisms of non-type 2 asthma. Curr Opin Immunol. (2020) 66:123–8. doi: 10.1016/j.coi.2020.10.002
54. Lindén A, Dahlén B. Interleukin-17 cytokine signalling in patients with asthma. Eur Respir J. (2014) 44(5):1319–31. doi: 10.1183/09031936.00002314
55. Group, E. S. The ENFUMOSA cross-sectional European multicentre study of the clinical phenotype of chronic severe asthma. Eur Respir J. (2003) 22(3):470–7. doi: 10.1183/09031936.03.00261903
56. Barrett A, Humeniuk P, Drevinge C, Corciulo C, Weidner J, Rådinger M, et al. Physiological estrogen levels are dispensable for the sex difference in immune responses during allergen-induced airway inflammation. Immunobiology. (2023) 228(3):152360. doi: 10.1016/j.imbio.2023.152360
57. Fuseini H, Newcomb DC. Mechanisms driving gender differences in asthma. Curr Allergy Asthma Rep. (2017) 17(3):19. doi: 10.1007/s11882-017-0686-1
58. Aldhalmi AK, Al-Athari AJH, Makki Al-Hindy HA. Association of tumor necrosis factor-α and myeloperoxidase enzyme with severe asthma: a comparative study. Rep Biochem Mol Biol. (2022) 11(2):238–45. doi: 10.52547/rbmb.11.2.238
59. Randolph AG, Lange C, Silverman EK, Lazarus R, Silverman ES, Raby B, et al. The IL12B gene is associated with asthma. Am J Hum Genet. (2004) 75(4):709–15. doi: 10.1086/424886
60. Scott G, Asrat S, Allinne J, Keat Lim W, Nagashima K, Birchard D, et al. IL-4 and IL-13, not eosinophils, drive type 2 airway inflammation, remodeling and lung function decline. Cytokine. (2023) 162:156091. doi: 10.1016/j.cyto.2022.156091
61. Wu D, Zhou J, Bi H, Li L, Gao W, Huang M, et al. CCL11 As a potential diagnostic marker for asthma? J Asthma. (2014) 51(8):847–54. doi: 10.3109/02770903.2014.917659
62. Kim D, Kim YH, Kang J-H, Park C-S. Increased expression of nucleophosmin is associated with the pathophysiology of chronic rhinosinusitis with nasal polyposis. Auris Nasus Larynx. (2020) 47(5):807–13. doi: 10.1016/j.anl.2020.02.010
63. Yuan L, Wang L, Du X, Qin L, Yang M, Zhou K, et al. The DNA methylation of FOXO3 and TP53 as a blood biomarker of late-onset asthma. J Transl Med. (2020) 18. doi: 10.1186/s12967-020-02643-y
64. Bonds RS, Midoro-Horiuti T. Estrogen effects in allergy and asthma. Curr Opin Allergy Clin Immunol. (2013) 13(1):92–9. doi: 10.1097/ACI.0b013e32835a6dd6
65. Niessen NM, Gibson PG, Simpson JL, Scott HA, Baines KJ, Fricker M. Airway monocyte modulation relates to tumour necrosis factor dysregulation in neutrophilic asthma. ERJ Open Res. (2021) 7(3):00131–2021. doi: 10.1183/23120541.00131-2021
66. Scioscia G, Carpagnano GE, Lacedonia D, Soccio P, Quarato CMI, Trabace L, et al. The role of airways 17β-estradiol as a biomarker of severity in postmenopausal asthma: a pilot study. J Clin Med. (2020) 9:7. doi: 10.3390/jcm9072037
67. Kanazawa H. VEGF, angiopoietin-1 and -2 in bronchial asthma: new molecular targets in airway angiogenesis and microvascular remodeling. Recent Pat Inflamm Allergy Drug Discov. (2007) 1(1):1–8. doi: 10.2174/187221307779815066
68. Sharma S, Murphy A, Soto-Quiros M, Avila L, Klanderman B, Sylvia J, et al. Association of VEGF polymorphisms with childhood asthma, lung function and airway responsiveness. Eur Respir J. (2009) 33(6):1287–94. doi: 10.1183/09031936.00113008
69. Lee CG, Link H, Baluk P, Homer RJ, Chapoval S, Bhandari V, et al. Vascular endothelial growth factor (VEGF) induces remodeling and enhances TH2-mediated sensitization and inflammation in the lung. Nat Med. (2004) 10(10):1095–103. doi: 10.1038/nm1105
70. Byrne AJ, Jones CP, Gowers K, Rankin SM, Lloyd CM. Lung macrophages contribute to house dust mite driven airway remodeling via HIF-1α. PLoS One. (2013) 8(7):e69246. doi: 10.1371/journal.pone.0069246
71. Forsythe JA, Jiang BH, Iyer NV, Agani F, Leung SW, Koos RD, et al. Activation of vascular endothelial growth factor gene transcription by hypoxia-inducible factor 1. Mol Cell Biol. (1996) 16(9):4604–13. doi: 10.1128/MCB.16.9.4604
72. Lal CV, Travers C, Aghai ZH, Eipers P, Jilling T, Halloran B, et al. The airway microbiome at birth. Sci Rep. (2016) 6(1):31023. doi: 10.1038/srep31023
73. Zhang D, Li S, Wang N, Tan HY, Zhang Z, Feng Y. The cross-talk between gut microbiota and lungs in common lung diseases. Front Microbiol. (2020) 11:301. doi: 10.3389/fmicb.2020.00301
74. Ege MJ, Mayer M, Normand AC, Genuneit J, Cookson WO, Braun-Fahrländer C, et al. Exposure to environmental microorganisms and childhood asthma. N Engl J Med. (2011) 364(8):701–9. doi: 10.1056/NEJMoa1007302
75. Barfod KK, Roggenbuck M, Hansen LH, Schjørring S, Larsen ST, Sørensen SJ, et al. The murine lung microbiome in relation to the intestinal and vaginal bacterial communities. BMC Microbiol. (2013) 13:303. doi: 10.1186/1471-2180-13-303
76. Alashkar Alhamwe B, Gao Z, Alhamdan F, Harb H, Pichene M, Garnier A, et al. Intranasal administration of Acinetobacter Iwoffii in a murine model of asthma induces IL-6-mediated protection associated with cecal microbiota changes. Allergy. (2023) 78(5):1245–57. doi: 10.1111/all.15606
77. Huang YJ, Nelson CE, Brodie EL, Desantis TZ, Baek MS, Liu J, et al. Airway microbiota and bronchial hyperresponsiveness in patients with suboptimally controlled asthma. J Allergy Clin Immunol. (2011) 127(2):372–381.e1-3. doi: 10.1016/j.jaci.2010.10.048
78. Chiu KM, Arnaud CD, Ju J, Mayes D, Bacchetti P, Weitz S, et al. Correlation of estradiol, parathyroid hormone, interleukin-6, and soluble interleukin-6 receptor during the normal menstrual cycle. Bone. (2000) 26(1):79–85. doi: 10.1016/s8756-3282(99)00243-4
79. Yao S, Zhao Z, Wang W, Liu X. Bifidobacterium longum: protection against inflammatory bowel disease. J Immunol Res. (2021) 2021. doi: 10.1155/2021/8030297
80. Stojanov S, Berlec A, Štrukelj B. The influence of probiotics on the Firmicutes/Bacteroidetes ratio in the treatment of obesity and inflammatory bowel disease. Microorganisms. (2020) 8:11. doi: 10.3390/microorganisms8111715
81. Ley RE, Turnbaugh PJ, Klein S, Gordon JI. Microbial ecology: human gut microbes associated with obesity. Nature. (2006) 444(7122):1022–3. doi: 10.1038/4441022a
82. Mariat D, Firmesse O, Levenez F, Guimarăes V, Sokol H, Doré J, et al. The Firmicutes/Bacteroidetes ratio of the human microbiota changes with age. BMC Microbiol. (2009) 9:123. doi: 10.1186/1471-2180-9-123
83. Lahti L, Salonen A, Kekkonen RA, Salojärvi J, Jalanka-Tuovinen J, Palva A, et al. Associations between the human intestinal microbiota, lactobacillus rhamnosus GG and serum lipids indicated by integrated analysis of high-throughput profiling data. PeerJ. (2013) 1:e32. doi: 10.7717/peerj.32
84. Ruhl IA, Sheremet A, Smirnova AV, Sharp CE, Grasby SE, Strous M, et al. Microbial functional diversity correlates with Species diversity along a temperature gradient. mSystems. (2022) 7(1):e00991–00921. doi: 10.1128/msystems.00991-21
85. Wang S, Loreau M. Biodiversity and ecosystem stability across scales in metacommunities. Ecol Lett. (2016) 19(5):510–8. doi: 10.1111/ele.12582
86. Kim B-R, Shin J, Guevarra RB, Lee JH, Kim DW, Seol K-H, et al. Deciphering diversity indices for a better understanding of microbial communities. J Microbiol Biotechnol. (2017) 27(12):2089–93. doi: 10.4014/jmb.1709.09027
87. Bisgaard H, Li N, Bonnelykke K, Chawes BLK, Skov T, Paludan-Müller G, et al. Reduced diversity of the intestinal microbiota during infancy is associated with increased risk of allergic disease at school age. J Allergy Clin Immunol. (2011) 128(3):646–52.e1-5. doi: 10.1016/j.jaci.2011.04.060
88. Morgan XC, Huttenhower C. Chapter 12: human microbiome analysis. PLoS Comput Biol. (2012) 8(12):e1002808. doi: 10.1371/journal.pcbi.1002808
89. Pang Z, Wang G, Gibson P, Guan X, Zhang W, Zheng R, et al. Airway microbiome in different inflammatory phenotypes of asthma: a cross-sectional study in northeast China. Int J Med Sci. (2019) 16(3):477–85. doi: 10.7150/ijms.29433
90. Perez-Garcia J, Hernández-Pérez JM, González-Pérez R, Sardón O, Martin-Gonzalez E, Espuela-Ortiz A, et al. The genomics and metagenomics of asthma severity (GEMAS) study: rationale and design. J Pers Med. (2020) 10:3. doi: 10.3390/jpm10030123
91. Goleva E, Jackson LP, Harris JK, Robertson CE, Sutherland ER, Hall CF, et al. The effects of airway microbiome on corticosteroid responsiveness in asthma. Am J Respir Crit Care Med. (2013) 188(10):1193–201. doi: 10.1164/rccm.201304-0775OC
92. Hilty M, Burke C, Pedro H, Cardenas P, Bush A, Bossley C, et al. Disordered microbial communities in asthmatic airways. PLoS One. (2010) 5(1):e8578. doi: 10.1371/journal.pone.0008578
93. Finotello F, Mastrorilli E, Di Camillo B. Measuring the diversity of the human microbiota with targeted next-generation sequencing. Brief Bioinformatics. (2016) 19(4):679–92. doi: 10.1093/bib/bbw119
94. Su H, Zhang D, Antwi P, Xiao L, Zhang Z, Deng X, et al. Adaptation, restoration and collapse of anammox process to la(III) stress: performance, microbial community, metabolic function and network analysis. Bioresour Technol. (2021) 325:124731. doi: 10.1016/j.biortech.2021.124731
95. Yuan X, Chen R, Zhang Y, Lin X, Yang X. Sexual dimorphism of gut microbiota at different pubertal status. Microb Cell Fact. (2020) 19(1):152. doi: 10.1186/s12934-020-01412-2
96. Sheffield PE, Zhou J, Shmool JLC, Clougherty JE. Ambient ozone exposure and children’s acute asthma in New York city: a case-crossover analysis. Environ Health. (2015) 14:1–10. doi: 10.1186/s12940-015-0010-2
97. Cho DY, Hunter RC, Ramakrishnan VR. The microbiome and chronic rhinosinusitis. Immunol Allergy Clin North Am. (2020) 40(2):251–63. doi: 10.1016/j.iac.2019.12.009
98. Cho I, Blaser MJ. The human microbiome: at the interface of health and disease. Nat Rev Genet. (2012) 13(4):260–70. doi: 10.1038/nrg3182
99. Ekpruke CD, Alford R, Parker E, Silveyra P. Gonadal sex and chromosome complement influence the gut microbiome in a mouse model of allergic airway inflammation. Physiol Genomics. (2024) 56(6):417–25. doi: 10.1152/physiolgenomics.00003.2024
100. Kasahara K, Rey FE. The emerging role of gut microbial metabolism on cardiovascular disease. Curr Opin Microbiol. (2019) 50:64–70. doi: 10.1016/j.mib.2019.09.007
101. Yurkovetskiy L, Burrows M, Khan AA, Graham L, Volchkov P, Becker L, et al. Gender bias in autoimmunity is influenced by microbiota. Immunity. (2013) 39(2):400–12. doi: 10.1016/j.immuni.2013.08.013
Keywords: lung microbiome, gut microbiome, sex differences, allergic asthma, lung inflammation
Citation: Ekpruke CD, Alford R, Rousselle D, Babayev M, Sharma S, Parker E, Davis K, Hemmerich C, Rusch DB and Silveyra P (2024) Sex-specific alterations in the gut and lung microbiome of allergen-induced mice. Front. Allergy 5:1451846. doi: 10.3389/falgy.2024.1451846
Received: 19 June 2024; Accepted: 29 July 2024;
Published: 15 August 2024.
Edited by:
Kelly M. Mcnagny, University of British Columbia, CanadaReviewed by:
Daniel P. Potaczek, University of Marburg, Germany© 2024 Ekpruke, Alford, Rousselle, Babayev, Sharma, Parker, Davis, Hemmerich, Rusch and Silveyra. This is an open-access article distributed under the terms of the Creative Commons Attribution License (CC BY). The use, distribution or reproduction in other forums is permitted, provided the original author(s) and the copyright owner(s) are credited and that the original publication in this journal is cited, in accordance with accepted academic practice. No use, distribution or reproduction is permitted which does not comply with these terms.
*Correspondence: Patricia Silveyra, cHNpbHZleXJAaXUuZWR1
Disclaimer: All claims expressed in this article are solely those of the authors and do not necessarily represent those of their affiliated organizations, or those of the publisher, the editors and the reviewers. Any product that may be evaluated in this article or claim that may be made by its manufacturer is not guaranteed or endorsed by the publisher.
Research integrity at Frontiers
Learn more about the work of our research integrity team to safeguard the quality of each article we publish.