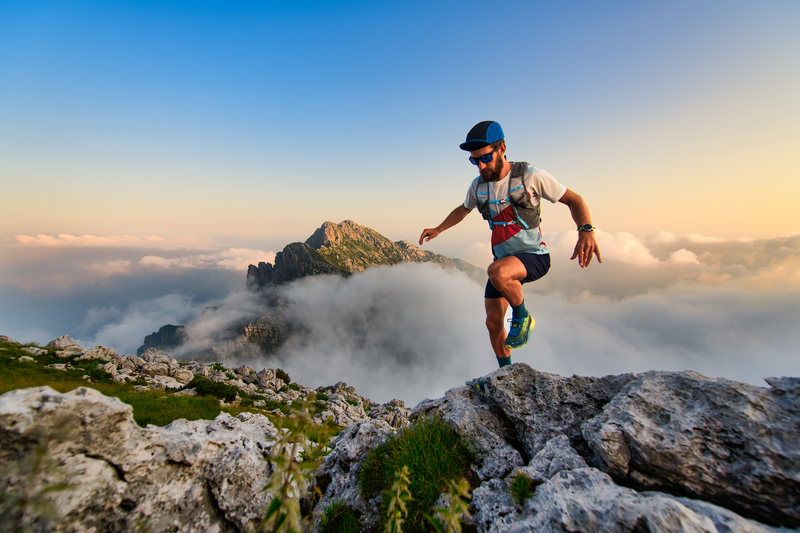
95% of researchers rate our articles as excellent or good
Learn more about the work of our research integrity team to safeguard the quality of each article we publish.
Find out more
ORIGINAL RESEARCH article
Front. Agron. , 10 July 2024
Sec. Weed Management
Volume 6 - 2024 | https://doi.org/10.3389/fagro.2024.1407422
Herbicide resistance has become a major issue in recent decades. Because diagnostics is still expensive, prediction models are helping to assess risks of resistance evolution. In this paper the influence of weed management on the evolution of resistance of the grass Alopecurus myosuroides Huds to ALS-inhibitors is investigated based on field history data from two regions, Hohenlohe in Germany and Champagne in France respectively. Champagne data also comprise information on Lolium spp. Using a random forest method variable importance and performance measures were obtained for a large number of single analyses allowing for a statistical analysis of the four performance measures, type I error, type II error, AUC and accuracy. It could be shown that acceptable predictions can be obtained for training data from Hohenlohe applied to Champagne and vice versa. It turned out that in nearly all analyses false negative classifications are more frequent than false positive classifications. Based on a combined training set of A.myosuroides samples from Hohenlohe and Champagne resistance status of Lolium spp. from the Champagne dataset can be predicted with a good accuracy. This suggest that resistance evolution to ALS-inhibitors of the two grasses are closely related. This work is a first step to set a simple herbicide resistance prediction tool to the users based on field history weed management data.
In the last decades, herbicide resistance has become a major issue for many weeds (Gressel, 2009; Powles and Yu, 2010; Heap, 2024). Weed population dynamics and control is a complex process depending not only on the choice of appropriate herbicides but also on cropping patterns, cultural techniques and other crop management practices (Lutman et al., 2013; Massa et al., 2013; Hawkins et al., 2019). From an economical point of view, the costs of herbicide resistance management of A. myosuroides were significantly higher than pro-active measures aiming to mitigate the evolution of resistance (Gerhards et al., 2016).
Resistance development is a long-term process but can be mitigated by the introduction of integrated weed management (IWM) which combines agronomy and chemical weed control (Moss, 2017). Weed population dynamics is influenced by variables such as weather conditions, spatial inhomogeneity of the seed bank, initial frequency of resistant biotypes and spray distribution patterns in a random manner (Zwerger et al., 2017). Therefore, it is not surprising, that with closely related, but not similar, field history, some farmers observed resistant weeds in their fields and others not. Modelling of herbicide resistance evolution can be a very helpful approach in helping farmers to define the best strategies to be adopted to control weeds in a given location and environment. Nevertheless, reliable models are still very difficult to define, in particular due to the multiple mechanisms involved in weed resistance (Comont et al., 2020). The assumption that the use of herbicide mixtures was mitigating the evolution of weed resistance is mainly true when the resistance mechanism present is target-site resistance (TSR) i.e. where the structure or/and the expression of the chemical’s target is altered (Powles and Yu, 2010). Most of the models are defined according to TSR evolution, which provide a specific resistance (Bourguet et al., 2013; Comont et al., 2020). Today there is increasing evidence that non-target site resistance (NTSR) is more and more widespread (Bobadilla and Tranel, 2024). NTSR involves several mechanisms such as herbicide detoxification, inhibition of uptake and transport, or vacuole sequestration. It usually involves multiple genes and can confer a broad resistance spectrum to chemicals representing several modes of action, a generalist resistance (Délye et al., 2013; Délye, 2013; Comont et al., 2020; Bobadilla and Tranel, 2024). Modelling NTSR is extremely difficult considering the number of different mechanisms and the multiple genes involved. There are mainly two ways to assess appropriate management schemes. One way is based on mathematical process models (cf. Renton et al., 2014; Richter et al., 2016). The advantages of process models are that they are based explicitly on population dynamics and genetics and are capable of analyzing underlying mechanisms. The disadvantage is that these models request a large input of physiological parameters like e.g., seed emergence rates, number of seeds, seed survival, vertical transport parameters due to soil cultivation, competition coefficients with respect to crops as well as parameters of statistical distributions of environmental variables. The second approach is based on statistical methods notably on techniques of artificial intelligence. Crop protection can greatly benefit of random forest approach. It was shown that a Markov random field model has the potential to model the evolution of herbicide resistance in rye grass in a spatial context (Ip et al., 2018). This was confirmed by Oliveira et al. (2021) who applied a random forest approach to the analysis of the evolution of glyphosate and PPO-inhibitor resistance in Palmer amaranth. The advantage of machine learning methods is that they are capable of detecting patterns in huge bodies of data allowing to identify the driving forces for resistance development. Disadvantages are that large data bases comprehending long term field histories are needed requiring the compliance of the farmers partaking in the study. Furthermore, outcomes are frequently difficult to interpret in terms of the underlying mechanisms.
Black-grass (Alopecurus myosuroides Huds) mostly occurs in Central and Western Europe, where it has established as one of the most problematic weed (Moss et al., 2007). It has fast evolved resistance to acetyl-CoA carboxylase (ACCase, HRAC group 1)-, acetolactate synthase (ALS, HRAC group 2)- and photosynthetic (PSII, HRAC group 5)-inhibitors, all post-emergence herbicides (Moss et al., 2007). This has a strong economic impact, impairing the yield of cereal based cropping systems (Varah et al., 2020). Today only pre-emergence herbicides, like HRAC group 15, remain as an effective chemical tool in locations where high resistance has evolved to post-emergence herbicides (Dücker et al., 2019a, 2019b, 2020).
In a previous study, blackgrass field samples were collected as well as field history data comprising a period of 6 years in a region of Southern Germany, region of Hohenlohe, and herbicide resistance was assessed (Herrmann, 2016). A similar study was performed in Northern France, region of Champagne (Lepke et al., 2020). Based on this material, we performed extensive studies with a random forest approach to assess the driving variables and their rankings in both regions. The availability of data from two regions with comparable crop management, prompted us to test, whether the patterns found in Hohenlohe data are specific for this region or whether they are transferable not only to another region, in that case Champagne (and vice versa) but also to another grass weed, in that case Lolium spp (rye-grass), important worldwide weed to be controlled (Powles and Yu, 2010; Dücker et al., 2019b) and which has evolved herbicide resistance (Heap, 2024). Descriptive data analyses were presented and some results of single analyses were already discussed (Lepke et al., 2020).
In this study, we report the results obtained by the application of the random forest method, based on field history parameters, on the evolution of herbicide resistance in black-grass. Variable importance and performance measures were studied in a systematic way for a large number of single analyses allowing for a statistical analysis of the four performance measures, type I error, type II error, AUC and accuracy. This is a first step showing that reliable weed resistance management prediction can be based on a random forest model approach. In addition, the model developed in one region can be successfully used in another one (Hohenlohe versus Champagne). Furthermore the model developed for black-grass can be extended to another grass weed, in that case rye-grass.
Field history data from two earlier studies were used. A first data set related to A. myosuroides Huds (blackgrass) includes the field histories and resistance status of 98 fields from the Hohenlohe area in Germany (Herrmann, 2016) and 131 from the Champagne area in France (Lepke et al., 2020). For the Champagne area, a second data set related to Lolium spp. (rye-grass) was obtained for 49 fields with resistance status and field history information. Predictor variables comprise in particular crop species rotation, number of crops used over a 6-year period, number of winter and summer crops respectively, seeding date, soil cultivation like ploughing or shallow tillage and herbicide applications. In total, there are 20 predictors as described in Table 1. For each field management data were recorded for a period of 6 years, e.g. crops, plant protection products, and soil cultivation. This is denoted as field history. From these data an input vector for the random forest procedure was generated by allocating scores. For example, for ploughing 1 or 0 was assigned depending on whether the tillage method was plough or another respectively. The variable no. of crops is the number of unique crops cultivated within the observation period. All other variables were treated in the same manner (cf. Table 1).
Table 1 List of predictor variables [based on Herrmann (2016)].
The predictor variable management diversity considers the number of different measures specific for weed control within a year (Herrmann, 2016). These comprise delayed seeding, ploughing, summer crops and the use of multiple modes of action. If a measure is applied, the respective score takes the value of 1 otherwise the value of 0. If all measures are applied, the maximum value of management diversity is 4, if none is applied the score is 0. The index ranges therefore between 0 and 4. For example in a winter wheat field, using two modes of action (score 1) and ploughing (score 1) results in a management diversity value of 2, while shallow tillage (score 0) and only one mode of action (score 0) give a management diversity value of 0. Management diversity values are averaged for the time frame of the 6yrs being considered. Descriptive data analysis showed that the data sets of both countries have a similar structure. Details are described in Lepke et al., 2020.
Black-grass seed samples were harvested in 98 fields in Hohenlohe and in 131 fields in Champagne region. In addition, samples of rye-grass seeds were harvested in 49 fields in France Champagne region. Resistance to mesosulfuron and iodosulfuron (ALS-inhibitors) as well as to pinoxaden (ACCase-inhibitor) was assessed in the greenhouse as described in details (Herrmann, 2016; Herrmann et al., 2019; Lepke et al., 2020). In summary, herbicides were applied at BBCH 11–13, at 0.5 kg/ha (Atlantis WG, mesosulfuron/iodosulfuron) and at 1.2 L/ha (Axial 50, pinoxaden) in a total volume of 200L water using a Teejet-8002-EVS nozzle and a Track Sprayer Generation III. A visual assessment was done 21 days after spraying and the number of dead and living plants counted. A population was considered sensitive when the herbicide efficacy was higher than 90%. The resistance was considered in development when the efficacy of the herbicide was found between 50% and 89% and the resistance was considered as established when the herbicide efficacy was below 50%. Furthermore, when necessary, ALS- and ACCase-target site mutations were determined by pyrosequencing as described previously (Beffa et al., 2012). Detoxification of the herbicides was analyzed by HPLC separation after incubating individual plants using respectively C14 - radiolabeled mesosulfuron (ALS-inhibitor) and fenoxaprop-ethyl (ACCase-inhibitor) (Beffa et al., 2012).
Fields were classified as resistant if target-site resistance or metabolic resistance or both were detected in the samples and/or survivals were observed in the greenhouse (Herrmann et al., 2016).
All calculations were carried out in R 1.1.456 (R Core Team, 2018). For the classification problem with 20 predictor variables the random forest method (Breiman, 2001) was applied using the randomForest package (v4.6.14; Liaw and Wiener, 2002). Unless otherwise described, the data has been split into training data (75%) and test data (25%) using the caret package (v6.0.81; Kuhn et al., 2018). Importance measures were analyzed, to test which predictor variables have a significant impact on the response. Usual importance measures are the Gini index, the mean decrease accuracy index and the area under the Receiver Operating Characteristic curve (AUC) (Hastie et al., 2017). Note that as the name already implies the random forest outcomes are random due to random sampling of training data points when building trees and the random selection of subsets of features considered when splitting nodes. This intrinsic randomness affects the stability of feature importance measures (Wang et al., 2016). Therefore, the rating of features based on a single run is not robust especially if predictor variables are correlated. To consider randomness, a ranking of predictor variables was generated based on 50 repeated runs.
The most common ways of handling missing values are: a) delete cases with missing values b) fill in missing values with the median (for numerical values) or mode (for categorical values) obtained from all complete cases. In case of a) available information is lost. The second method can cause significant losses of accuracy for data sets with many gaps and significant structure. In the study done in that report, alternative methods such as filling in missing values by the median of k-nearest neighbours (Gupta, 2015) are recommended. In our study, method b) was analyzed for the common A. myosuroides dataset from both Hohenlohe and Champagne (229 fields).
Data gaps were generated randomly and the missing values in the new created datasets were replaced by the median of the remaining data. Figure 1 shows several measures of performance in dependence of the number of gaps. Accuracy is defined as the number of true positives + true negatives divided by the total number of predictions. AUC is the area under the receiver operation curve (ROC). ROC is a plot of the positive rate (TPR) versus the false positive rate (FPR) for different parameters of a classification rule (Gareth et al., 2021). The AUC can assume values between zero (worst classifier) and one (perfect classifier).
Figure 1 Performance measures accuracy, AUC, type I error and type II error in dependence of the percentage of missing values.
One can see a significant drop of AUC and an increase of the type I error (false positive classification) when a threshold of 40% missing values is surpassed. Type II errors (false negative classification) and accuracies are only slightly affected. The same pattern occurs, if missing values are generated only for the 4 variables with the highest Gini rank. Figure 2 shows the dependence of the accuracy on the sample size. The relationship turns out to be linear.
The transferability of the random forest model was analyzed in three steps.
1. Champagne and Hohenlohe data sets were analyzed separately.
2. Training and test data were interchanged, e.g. Hohenlohe data were used to train the random forest and Champagne data were predicted.
3. Champagne and Hohenlohe data were merged and used as training data set. Test data sets were taken from Champagne and Hohenlohe data, from Champagne data only and from Hohenlohe data only respectively.
To assess the importance of prediction variables analyses with both datasets (Hohenlohe and Champagne) separately and in combination a random splitting of training and test data, with a ratio of 75% and 25% respectively was used. Statistics of performance measures were generated by 50 simulation runs respectively.
To assess the importance of the soil variables an analysis with the Hohenlohe dataset (with and without soil information) was performed with random splitting of training and test data, with a ratio of 75% and 25% respectively. Performance measures based on 50 runs showed only slight differences between the median values. However, the variance of the accuracy obtained with soil information is lower than the accuracy obtained without soil information. Since no soil information was available for the Champagne data set and it was shown in the Hohenlohe data set that the soil did not have major influence, no soil features were considered further.
Table 2 show the frequencies of prediction variables falling into the group of the six highest ranked importance measures in 50 random forest runs with randomly split training and test data sets for the Hohenlohe data (a), the Champagne data (b), and the combined data set (c). Table 2 shows the frequencies of prediction variables obtained by this data set falling into the group of the six highest ranked importance measures. E.g., the variable management diversity is in all runs among the six highest ranked, whereas the variable Summer crops appears only once. The variables with the highest importance in the Hohenlohe data set are management diversity, ploughing, products group 2 (HRAC group 2), late seeding and no. of active ingredients used during the 6 years.
Table 2 Frequency of prediction variables under the six highest ranked after 50 runs for the Hohenlohe data (A), Champagne data (B) and combined Champagne and Hohenlohe data (C).
In a second step, the performance of RF was investigated under omission of variables. The sequence of variable reduction was guided by the Gini importance. Figure 3 shows the selection of the variables for the Hohenlohe data set. The dashed lines mark groups of variables, that were omitted subsequently. For each reduction 50 runs of the random forest model were performed with fixed training and test data sets. Reduction 1 means that the last group with lowest Gini importance was omitted, reduction 2 means that additionally group 2 was omitted, etc. The curves represent the mean values for the performance indices accuracy, AUC, type I and type II error. In addition, the standard deviations are also shown. Up to reduction 2, the results remain stable. If more variables are omitted, the results become more and more unstable with increasing standard deviations. For the Champagne data set (Figure 3B) the same pattern occurs. The analysis of the combined data set shows, that the performance of the random forest model is increased, when only variables with high Gini Index values are used (Figure 3C).
Figure 3 Simulation results of Random Forest performance measures under omission of variables. The left column shows the Gini importance measures for the Hohenlohe (A), Champagne (B) and the combined data set (C) respectively. The dashed lines mark groups of variables which were omitted. The right column shows the corresponding measures accuracy, AUC, type I and type II errors. The colors on the left side of the figure correspond to the colors on the right hand side. Legends of performance measure as in Figure 1.
A further analysis with the combined dataset was performed with random splitting of training and test data. Here, the performance for the following cases was compared
a. all prediction variables
b. highest ranked variable
c. four best ranked variables
Figure 4 shows box plots for several performance measures based on 50 runs. If the set of prediction variables is reduced to the variable with the highest rank (case b), the performance scores show large variances compared to case a, when all prediction variables are employed. With the exception of the type I error, median values do not change much. It is interesting that the performance obtained with the four best ranked variables only slightly differs from the results obtained for case a both with respect to the median values and the variation.
Figure 4 Box plots for four performance measures, type I error, type II error, AUC and accuracy obtained from 50 runs on the basis of all prediction variables (A) and highest ranked variable (B).
An interesting question is if RF is able to differentiate between the field histories of Hohenlohe and Champagne regions. Although both data sets are similar at a first glance (cf. section 3.2), it is surprising that the random forest produces a clear-cut distinction between field histories of both regions with an accuracy of about 93%. The four highest ranked predictor variables are late seeding, products HRAC group 2 (ALS-inhibitors), products HRAC group 1 (ACCase-inhibitors), and number of dicot Crops in the 6 years rotation.
It is important to note that high ranked variables for the prediction of resistance, e.g. management diversity, have only a low rank concerning the differences between the two regions.
Separate analyses of the data sets of both regions as described in material and methods (1. step) gave similar results for both the accuracy as well as the type I and type II errors. In the second step we found that prediction accuracies are more different than in step one, if training and test data between Champagne and Hohenlohe are exchanged. However, when merging the data sets as described above (3. step) all combinations yielded similar results comparable to those obtained in step one. Two general features are apparent. In all combinations, type I are larger than type II errors. These results indicate that in both regions a closely related patterns of weed management are likely to develop resistance. This confirmed preliminary data obtained by using the random forest model under different combinations of training and test data (Lepke et al., 2020).
The most striking feature of the Gini index is the high rank of the variable management diversity (Figure 5). Large differences in ranking occur for the variable summer cereals (proportion of summer cereals in the crop rotation). However, for all data sets, 4 variables out of the first six places are identical. These are the number of ALS-Inhibitor applications against A. myosuroides [ALOMY group 2 (B)], management diversity, the number of different group 2 (B) products used [products group 2 (B)], and the number of different active ingredients which were applied (no. of active ingredients). The mean decrease of accuracy measure gives similar results (Figure 6): the variables ALOMY group 2 (B) and products group 2 (B) and variables pertaining to crop rotation are highly ranked.
Figure 5 Comparison of the Gini importance measure for the four training data sets. (A) Training data set consists of 75% of the Hohenlohe data set. (B) Training data set consists of 75% of the Champagne data set. (C) The Hohenlohe data set was combined with the Champagne data set and 75% of the data was used in the training data set. (D) The training data sets from a and b were combined to a new training data set. Note that 4 variables out of the first six places are identical: Alomy group 2, management diversity, no. of active ingredients, products group 2.
For A. myosuroides the results show that in most combinations type II errors are lower than type I errors, i.e. false positive classifications are more frequent. There are two possible explanations:
i. The misclassified sensitive field has features similar to the features of resistant fields, but resistance has not developed as yet or has not been found in the plant samples.
ii. There are other factors not considered e.g. soil properties and weather patterns.
The results clearly show that the main factors promoting the evolution of A. myosuroides resistance are frequent use of herbicides of HRAC group 2 (ALS inhibitors), and low diversity of management. The importance of these factors is seen in all combinations of the data sets. For the Hohenlohe data, the factor ploughing turned out to be most important. Here, we see a possible conflict between soil conservation and avoidance of resistance. In the analysis based on the Champagne data and also on the combined data ploughing has only a minor importance. This could be explained by higher use of conservation cropping in Champagne. Finally for the Champagne data, the variable ALOMY group 2 (B, ALS inhibitors) is most important.
Employing the Champagne data set and the merged dataset of Champagne and Hohenlohe respectively, a random forest model was applied to Lolium spp. data from Champagne. Note that this data set comprises only resistant cases so the results have to be interpreted with caution. With the combined Hohenlohe-Champagne data as training set only one case was misclassified as sensitive (Lepke et al., 2020).
In our study it is shown that a machine learning algorithm to predict the risk of herbicide resistance evolution established in a certain region for black-grass, in that case Hohenlohe in Germany, is valid in another region belonging to another country, i.e. Champagne in France. Moreover, the same algorithm can be successfully extended to another grass species i.e. rye-grass. This strongly suggests that the same main parameters (agronomical and chemical) are of importance in the evolution of weed resistance for both grass species.
In conclusion, machine learning based approaches enable the identification of decisive factors for the evolution of resistance. Based on this knowledge, management schemes can be recommended for resistance mitigation. Although AI based approaches act as a black box, but with early understanding of the importance of the different parameters, their results help to formulate hypotheses on the underlying mechanisms, which might be captured in comprehensive mechanistic models. Even if additional parameters such as temperature, precipitation, etc could improve the random forest algorithm, our study shows that the prediction of resistance evolution is accurate in a majority of cases. In addition it corroborates the recommendations issued by many authors: to prevent resistance development it is important to define an overall integrated weed management approach by combining as many management practices comprising the use of different herbicides, diversity in crop rotations and cultivation as possible including cover crops, false seed bed, delayed sowing date, seed destruction, and other non-agronomic practices when appropriate (i.e. Beckie, 2006, Norsworthy et al., 2012; Byrne et al., 2018). A key advantage of a machine learning based approach to predict herbicide resistance risk evolution is that it does not require to develop specific mechanistic models based on parameters difficult to assess or requiring time consuming experiments, like e.g. soil seed bank, or soil composition analyses (Metcalfe et al., 2019). In addition once established, field history data, easy to determine, are enough to run the random forest model. It does not require tedious measurement of the TSR mutation frequencies and/or the analyses of the herbicide(s) detoxification in the plant (Bobadilla and Tranel, 2024). The evolution of specialist and generalist resistance (Comont et al., 2020) and the increasing of resistance cases (Heap, 2024) requires more and more to define pro-actively the adequate strategies to use herbicides (Bobadilla and Tranel, 2024) and to provide Integrated Weed Management solutions to farmers, applicators, retailors and advisors. Modelling will be an essential tool to contribute to that. Our study showed also that related species, in our cases, rye-grass and black-grass can be assessed with the same model as well as different locations, in that case two regions in two different countries. Finally, our results of the current study showed that few parameters, 4 to 6, related to agronomic and chemical practices can be useful to develop a random forest prediction tool for resistance evolution which can facilitate the decision of the farmers on the best IWM strategy to sustainably control weeds and mitigate the evolution of resistance. This will help to mitigate herbicide resistance evolution which impact farmer’s benefit (Staples, 2021) and increase the sustainability use of herbicides in IWM strategies (Moss, 2017).
The raw data supporting the conclusions of this article will be made available by the authors, without undue reservation.
JL: Writing – review & editing, Writing – original draft, Formal analysis, Methodology, Software. JH: Writing – review & editing, Writing – original draft, Conceptualization, Data curation. NR: Data curation, Writing – original draft, Writing – review & editing. RB: Data curation, Writing – original draft, Writing – review & editing, Conceptualization, Methodology, Project administration. OR: Conceptualization, Writing – original draft, Writing – review & editing, Supervision.
The author(s) declare financial support was received for the research, authorship, and/or publication of this article. This research was partly funded by Bayer CropScience.
Author JH is employed by the company Agris42 GmbH.
The remaining authors declare that the research was conducted in the absence of any commercial or financial relationships that could be construed as a potential conflict of interest.
All claims expressed in this article are solely those of the authors and do not necessarily represent those of their affiliated organizations, or those of the publisher, the editors and the reviewers. Any product that may be evaluated in this article, or claim that may be made by its manufacturer, is not guaranteed or endorsed by the publisher.
Beckie H. J. (2006). Herbicide-resistant weeds: management tactics and practices. Weed Technol. 20, 793–814. doi: 10.1614/WT-05-084R1.1
Beffa R., Figge A., Lorentz L., Hess M., Laber B., Ruiz-Santaella J. P., et al. (2012). Weed resistance diagnostic technologies to detect herbicide resistance in cereal growing areas. A review. Julius-Kühn-Archiv 434, 75–80. doi: 10.5073/jka.2012.434.008
Bobadilla L. K., Tranel P. J. (2024). Predicting the unpredictable: the regulatory nature and promiscuity of herbicide cross resistance. Pest Manage. Sci. 80, 235–244. doi: 10.1002/ps.7728
Bourguet D., Delmotte F., Franck P., Guillemaud T., Reboud X., Vacher C., et al. (2013). Heterogeneity of selection and the evolution of resistance. Trends Ecol. Evol. 28, 110–118. doi: 10.1016/j.tree.2012.09.001
Byrne R., Spink J., Freckleton R., Neve P., Barth S. (2018). A critical review of integrated grass weed management in Ireland. Irish J. Agric. Food Res. 57, 15–28. doi: 10.1515/ijafr-2018-0003
Comont D., Lowe C., Hull R., Crook L., Hicks H. L., Onkokesung N., et al. (2020). Evolution of generalist resistance to herbicide mixtures reveals a trade-off in resistance management. Nat. Commun. J. 11, 3086–3094. doi: 10.1038/s41467-020-16896-0
Délye C. (2013). Unravelling the genetic bases of non-target-site-based resistance (NTSR) to herbicides: a major challenge for weed science in the forthcoming decade. Pest Manage. Sci. 69, 176–187. doi: 10.1016/j.tig.2013.06.001
Délye C., Jasieniuk M., Le Corre V. (2013). Deciphering the evolution of herbicide resistance in weeds. Trends Genet. 29, 649–658. doi: 10.1016/j.tig.2013.06.001
Dücker R., Parcharidou E., Beffa R. (2020). Flufenacet activity is affected by GST inhibitors in blackgrass (Alopecurus myosuroides) populations with reduced flufenacet sensitivity and higher expression levels of GSTs. Weed Sci. 68, 451–459. doi: 10.1017/wsc.2020.54
Dücker R., Zöllner P., Lümmen P., Ries S., Collavo A., Beffa R. (2019b). Glutathione transferase plays a major role in flufenacet resistance of ryegrass (Lolium spp.) filed populations. Pest Manage. Sci. 75, 3084–3092. doi: 10.1002/ps.5425
Dücker R., Zöllner P., Parcharidou E., Ries S., Lorentz L., Beffa R. (2019a). Enhanced metabolism causes reduced flufenacet sensitivity in black-grass (Alopecurus myosuroides Huds.) field populations. Pest Manage. Sci. 75, 2996–3004.
Gareth J., Witten D., Hastie T., Tibshirani R. (2021). An introduction to statistical learning with applications in R. 2nd Edition (New York: Springer).
Gerhards R., Dentler J., Gutjahr C., Auburger S., Bahrs E. (2016). An approach to investigate the costs of herbicide-resistant Alopecurus myosuroides. Weed Res. 56, 407–414. doi: 10.1111/wre.12228
Gressel J. (2009). Evolving understanding of evolution of herbicide resistance. Pest Manage. Sci. 65, 1164–1173. doi: 10.1002/ps.1842
Gupta A. (2015). Overcoming Missing Values In A Random Forest Classifier (New York: The Airbnb Tech Blog). Available at: https://medium.com/airbnb-engineering/overcoming-missing-values-in-a-random-forest-classifier-7b1fc1fc03ba.
Hastie T., Tibshirani R., Friedman J. (2017). The Elements of Statistical Learning. 2nd ed (New York: Springer Verlag), 593 p.
Hawkins N. J., Bass C., Dixon A., Neve P. (2019). The evolutionary origins of pesticide resistance. Biol. Rev. 94, 135–155. doi: 10.1111/brv.12440
Heap I. (2024). The International Herbicide-Resistant Weed Database. Available online at: https://www.weedscience.org (Accessed May 15, 2024).
Herrmann J. (2016). Analysis of the spatial and temporal dynamics of herbicide resistance to ACCase- and ALS-Inhibitors in Alopecurus myosuroides Huds. and their causes. PhD thesis., TU Braunschweig: University of Technology Braunschweig. doi: 10.24355/dbbs.084-201701240925-0
Herrmann J., Hess M., Strek H., Richter O., Beffa R. (2016). Linkage of current ALS-resistance status with field history information of multiple fields infested with blackgrass (Alopecurus myosuroides Huds.) in southern Germany. Julius-Kühn-Archiv 452, 42–49. doi: 10.5073/jka.2016.452.006
Herrmann J., Hess M., Wagner J. (2019). Infestation and herbicide sensitivity in selected regions of Germany: results of a weed resistance monitoring 2019. Julius-Kühn-Archiv 464, 333–338.
Ip R. H. L., Ang L.-M., Seng K. P., Broster J. C., Pratley J. E. (2018). Big data and machine learning for crop protection. Comput. Electron. Agric. 151, 376–383. doi: 10.1016/j.compag.2018.06.008
Kuhn M., Wing J., Weston S., Williams A., Keefer C., Engelhardt A., et al. (2018). Caret: classification and regression training. R package version 6.0-81. Available online at: https://CRAN.R-project.org/package=caret.
Lepke J., Beffa R., Richter O., Herrmann J. (2020). Transferability of a random forest model for resistance prediction between different regions in Europe. Julius-Kühn-Archiv 464, 490–497. doi: 10.5073/jka.2020.464.074
Liaw A., Wiener M. (2002). Classification and regression by RandomForest. R News 2, 18–22. doi: 10.4236/ijcns.2016.95010
Lutman P. J. W., Moss S. R., Cook S., Welham S. J. (2013). A review of the effects of crop agronomy on the management of Alopecurus myosuroides. Weed Res. 53, 299–310. doi: 10.1111/wre.12024
Massa D., Kaiser Y. I., Andújar-Sánchez D., Carmona-Aleférez R., Mehrtens J., Gerhards R. (2013). Development of a geo-referenced database for weed mapping and analysis of agronomic factors affecting herbicide resistance in Apera spica-venti L. Beauv. (silky windgrass). Agronomy 3, 13–27. doi: 10.3390/agronomy3010013
Metcalfe H., Milne A. E., Coleman K., Murdoch A. J., Storkey J. (2019). Modelling the effect of spatially variable soil properties on the distribution of weeds. Ecol. Model. 396, 1–11. doi: 10.1016/j.ecolmodel.2018.11.002
Moss S. R. (2017). Black-grass (Alopecurus myosuroides): why has this weed become such a problem in western Europe and what are the solutions. Outlooks Pest Manage. 28, 207–2012. doi: 10.1564/v28_oct_04
Moss S. R., Perryman S. A. M., Tatnell L. V. (2007). Managing herbicide resistance in black-grass (Alopecurus myosuroides): Theory and practice. Weed Technol. 21, 300–309. doi: 10.1614/WT-06-087.1
Norsworthy J., Ward S. M., Shaw D. R., Llewellyn R. S., Nichols R. L., Webster T. M., et al. (2012). Reducing the risk of herbicide resistance: best management practices and recommendations. Weed Sci. 60, 31–62. doi: 10.1614/WS-D-11-00155.1
Oliveira M., Giacomini D., Arsenijevic N., Vieira G., Tranel P., Werle R. (2021). Distribution and validation of genotypic and phenotypic glyphosate and PPO-inhibitor resistance in Palmer amaranth (Amaranthus palmeri) from southwestern Nebraska. Weed Technol. 35, 65–76. doi: 10.1017/wet.2020.74
Powles S. B., Yu Q. (2010). Evolution in action: plants resistant to herbicides. Annu. Rev. Plant Biol. 61, 317–347. doi: 10.1146/annurev-arplant-042809-112119
R Core Team. (2018). R: A Language and Environment for Statistical Computing (Vienna, Austria: R Foundation for Statistical Computing). Available at: https://www.R-project.org/.
Renton M., Busi R., Neve P., Thornby D., Vila-Aiub M. (2014). Herbicide resistance modelling: past, present and future. Pest Manage. Sci. 70, 1394–1404. doi: 10.1002/ps.3773
Richter O., Langemann D., Beffa R. (2016). Genetics of metabolic resistance. Math. Biosci. 279, 71–82. doi: 10.1016/j.mbs.2016.07.005
Varah A., Ahodo K., Coutts S. R., Hicks H. L., Comont D., Crook L., et al. (2020). The costs of human-induced evolution in an agricultural system. Nat. Sustain. 3, 63–71. doi: 10.1038/s41893-019-0450-8
Wang H., Yang F., Luo Z. (2016). An experimental study of the intrinsic stability of random forest variable importance measures. BMC Bioinf. 17, (60). doi: 10.1186/s12859-016-0900-5
Keywords: artificial intelligence, black-grass, herbicide resistance prediction, geographical variation, rye-grass, resistance management
Citation: Lepke J, Herrmann J, Remy N, Beffa R and Richter O (2024) Weed resistance prediction: a random forest analysis based on field histories. Front. Agron. 6:1407422. doi: 10.3389/fagro.2024.1407422
Received: 26 March 2024; Accepted: 18 June 2024;
Published: 10 July 2024.
Edited by:
Ali Ahsan Bajwa, La Trobe University, AustraliaReviewed by:
Ahmet Uludag, Çanakkale Onsekiz Mart University, TürkiyeCopyright © 2024 Lepke, Herrmann, Remy, Beffa and Richter. This is an open-access article distributed under the terms of the Creative Commons Attribution License (CC BY). The use, distribution or reproduction in other forums is permitted, provided the original author(s) and the copyright owner(s) are credited and that the original publication in this journal is cited, in accordance with accepted academic practice. No use, distribution or reproduction is permitted which does not comply with these terms.
*Correspondence: Otto Richter, by5yaWNodGVyQHR1LWJzLmRl
Disclaimer: All claims expressed in this article are solely those of the authors and do not necessarily represent those of their affiliated organizations, or those of the publisher, the editors and the reviewers. Any product that may be evaluated in this article or claim that may be made by its manufacturer is not guaranteed or endorsed by the publisher.
Research integrity at Frontiers
Learn more about the work of our research integrity team to safeguard the quality of each article we publish.