- 1School of Population Health, The University of Western Australia, Perth, WA, Australia
- 2Telethon Kids Institute, The University of Western Australia, Perth, WA, Australia
- 3WZB Berlin Social Research Center, Berlin, Germany
- 4Faculty of Health Sciences, Centre for Population Health Research, Curtin University, Perth, WA, Australia
- 5School of Psychology and Speech Pathology, Curtin University, Perth, WA, Australia
- 6Neurosciences Unit, Health Department of Western Australia, Perth, WA, Australia
- 7School of Paediatrics and Child Health, The University of Western Australia, Perth, WA, Australia
Objectives: In this study, we aimed to investigate the long-term associations between breastfeeding duration during infancy, diet quality as measured by a diet score at 1 year of age, and cognitive performance during adolescence.
Methods: Participants (n = 717) were recruited from the West Australian Pregnancy Cohort (Raine) Study, a prospective longitudinal study of 2868 children and their families based in Perth, WA, Australia. Breastfeeding duration and an early diet score at age 1 year were used as the main predictor variables, while a computerized cognitive battery (CogState) was used to assess adolescents’ cognitive performance at 17 years. The diet score, which has seven food group components, was based on a 24-h recall questionnaire completed by the mother at 1 year of age. A higher diet score represents a better, more nutritious eating pattern. Associations between breastfeeding duration, diet score, and cognitive performance were assessed in multivariable regression models.
Results: Higher diet scores at 1 year representing better diet quality were significantly associated with faster reaction times in cognitive performance at 17 years [Detection Task (DET): β = −0.004, 95% CI: −0.008; 0.000, p = 0.036; Identification Task (IDN): β = −0.004, 95% CI: −0.008; 0.000, p = 0.027]. Breastfeeding duration (≥4 months) was also significantly associated with a shorter reaction time, but only for males (DET: β = −0.026, 95% CI: −0.046; −0.006, p = 0.010).
Conclusion: Nutrition in early childhood may have a long-term association with fundamental cognitive processing speed, which is likely to be related to enhanced brain development in the first year of life.
Introduction
It is well known that the first 2 years of life represent a sensitive period for neurodevelopmental processes (1, 2). These processes are genetically programed but are also influenced by environmental factors, including nutrition (1, 3, 4). Optimal nutrition during this critical developmental period may have long-lasting positive effects on individuals’ later cognitive abilities (5).
Breastfeeding is a nutritional factor that has been widely researched and reviewed in relation to cognitive performance in children. Early reviews have not provided compelling evidence linking breastfeeding to cognitive development (6–8). However, a recent review (9) concluded that after adjusting for confounders, which strongly influence breastfeeding including socioeconomic status and maternal education or IQ, there was a consistent positive association between breastfeeding duration and IQ with a difference between breastfed and non-breastfed children of approximately 2–5 points in IQ. Most of the research conducted to date on these associations has examined the effect of breastfeeding on cognitive development during childhood (6–9), while less is known about whether positive longer-term associations between breastfeeding and cognitive capacity are present (10, 11). One study examined longer-term associations between breastfeeding and cognitive performance in children from 8 to 18 years of age in New Zealand. They found that a longer duration of breastfeeding (categorized as: not breastfed; breastfed for <4 months, 4–7 months, and ≥8 months) was positively associated with cognitive and academic performance, as measured by the Wechsler Intelligence Scale for Children, teachers’ rating of school performance, reading and mathematics tests, and high school outcomes (10). Another study, conducted in Northern Ireland, found that 11- to 16-year-old adolescents breastfed for more than 12 weeks, compared to those who were breastfed between 1 and 12 weeks, showed higher verbal, numerical, and reasoning IQ and performed better on the Raven’s Standard Progressive Matrices test (11). The first aim of our study was to evaluate evidence concerning the prospective association between breastfeeding duration and cognitive performance at 17 years.
It is important to determine whether a longer-term association exists between diet in the early years and cognitive capacity in adolescence. This was a secondary aim of our study. While the possible connection between cognitive development and single nutrients has been widely researched in children (5), fewer studies have undertaken a more comprehensive assessment of nutrition in early childhood and related this to cognitive capacity (12–15). Moreover, only one study that we are aware of has explored these links in adolescence (16). In the Avon Longitudinal Study of Parents and Children (ALSPAC), researchers identified four dietary pattern trajectories with respect to food intake between 6 and 24 months of age: “Healthy,” “Discretionary,” “Traditional,” and “Ready-to-Eat.” They found negative associations between the “Discretionary” diet (that included foods such as crisps and chocolate) and the “Traditional” diet (characterized by intake of meat, cooked vegetables, and puddings) and cognitive performance as measured by the Wechsler Abbreviated Scale of Intelligence (WASI) at the age of 15 years (16).
Previously in the Western Australian Pregnancy Cohort (Raine) Study, breastfeeding was positively associated with language development at 6 and 10 years of age (17, 18) and those children who had a better quality diet at 1 year manifested better language and reasoning ability at 10 years (19). In the current study, we hypothesized that longer breastfeeding duration and a better dietary score during infancy would be associated with better cognitive performance in adolescence.
Materials and Methods
Study Population
Participants were recruited from The Western Australian Pregnancy Cohort (Raine) Study. Details of the study are described elsewhere (20). In brief, 2,900 women were initially recruited from King Edward Memorial Hospital (the major tertiary maternity hospital in Perth, WA, Australia) and surrounding private practices between 1989 and 1991. Women between 16 and 20 weeks’ gestation were randomly enrolled into the study to assess the effects of repetitive pregnancy ultrasounds. The initial cohort included a total of 2,868 babies [89.5% full-term (≥37 weeks gestation) and 10.5% pre-term infants (<37 weeks gestation)]. Families were followed up at regular intervals at ages 1, 2, 3, 5, 10, 14, and 17 years. Ethics approvals were obtained from The Human Ethics Committee at King Edward Memorial Hospital and/or Princess Margaret Hospital for Children (Perth, WA, Australia) for every Raine follow-up. In the current study, we analyzed breastfeeding and dietary data from the first 3 years of life as well as cognitive performance scores from the 17-year follow-up (undertaken between 2006 and 2009), when 717 adolescents (89% full-term gestational age) completed a computerized cognitive test battery.
Predictor Variables
Breastfeeding
Breastfeeding data were collected at 1–3 years of age, with data collection being retrospective in the previous year. The method used for collecting these data represents a valid and reliable estimate of breastfeeding initiation and duration (21). The age in months at which breastfeeding was discontinued was recorded, giving a continuous measure of breastfeeding duration. These data were also dichotomized as: <4 months or ≥4 months breastfeeding. We chose to include this binary breastfeeding variable in the analysis because at the time of data collection (1990–1995), the recommendation from the Word Health Organization (WHO) was that all babies should be exclusively breastfed from 4 to 6 months. This differs from the current recommendation that all infants should be exclusively breastfed for 6 months (22). (We did not take into account the introduction of solid foods.)
Diet score
At 1 year of age (i.e., between 1991 and 1993), primary caregivers completed a modified 24-h dietary recall questionnaire during the clinic visit (with assistance provided from a research nurse). Data were collected only once from each caregiver regardless of season. The collected dietary data were entered into the dietary analysis program FoodWorks® (Professional Version 5, 2007, Xyris Software, Brisbane, QLD, Australia). As serving sizes were not consistently recorded, the nutritionists entering the data used each “eating occasion” to represent “one serving of food,” as reported in other publications (23, 24). For our study, a team of three nutritionists categorized 2,262 individual foods and drinks consumed by participants into 100 food groups. These were further categorized into 20 categories according to the Australian Guide to Healthy Eating (25).
The diet score derived from these data include seven dietary components from 20 broad food categories [i. whole grains, ii. vegetables, iii. fruits, iv. meat ratio (numerator: white meat, egg, other protein sources, denominator: red meat, processed meats), v. dairy, vi. snack foods, and vii. sweetened beverages] (Table S1 in Supplementary Material). Scoring was guided by the Youth Healthy Eating Index (YHEI) (26) and adapted to our study data (19, 27). Each of the seven components received a score between 0 and 10 according to the number of eating occasions of each food group. A higher score represented more eating occasions of foods from the categories of wholegrain, vegetables, fruits, protein/meat ratio, and dairy and less frequent consumption from the categories of snack foods and sweetened beverages. The number of age-appropriate servings/eating occasions was based on the Dietary Guidelines for Children and Adolescents in Australia (28), which are similar to the servings used in the YHEI. For example, an infant who consumed a “healthy” food component (such as fruit, vegetables, dairy, wholegrains, or a high meat ratio) three or more times a day (i.e., optimal number of eating occasions) received a score of 10 for that dietary component. If none of these “healthy” foods were consumed, a score of zero was achieved for that component. Conversely, an infant who did not consume snack foods or soft drinks received the highest score for that dietary component, while three or more eating occasions for either of these components received a zero score. These scores were then summed to determine an overall dietary score ranging from 0 to 70, with a higher score representing a more healthy eating pattern (19) (Table S1 in Supplementary Material). This overall score was subsequently rescaled for easier interpretation from 0 to 10, with a higher score representing a healthier eating pattern. In summary, a better diet score indicates higher consumption of fruit, vegetables, wholegrain, dairy, white meat, and legumes and less consumption of red and processed meats, soft drinks, and snack food. The diet score was found to be normally distributed and correlated well with the socio-demographic characteristics of the cohort (27). Furthermore, in a previous study, a better early diet score was associated with an improved cognitive performance in middle childhood (19).
Outcome Variables
The cognitive assessment was undertaken between 2007 and 2009, when participants were a mean age of 17.11 years (range: 16.01–18.32 years; SD: 0.22 years). Cognitive performance was measured by a computerized cognitive battery developed by CogState (CogState Ltd., Melbourne, Australia). CogState is a valid, reliable, and sensitive test designed to assess neurocognitive functioning across the lifespan (29, 30). CogState is suitable for different cultures and requires only minimal language skills (31). It has limited practice effects (32) and correlates well with conventional neuropsychological measures (Pearson’s R in the range.49–0.83) (33). In our study, four tasks were chosen from the CogState battery (summarized in Table S2 in Supplementary Material), from which four main outcome variables were analyzed. The Detection Task (DET) was the least complex task in the test battery; a playing card was presented on the screen and participants were required to indicate by pressing a key as quickly as they could as soon as the card turned over. This task measures basic psychomotor speed. The principal outcome variable was reaction time. The Identification Task (IDN) is a simple choice response task; in this task, the participants were required to indicate whether the card that turned over is red (using “yes” or “no” keys on the computer keyboard). This task assesses psychomotor speed and visual attention. The principal outcome measure was reaction time. In the One Card Learning Task (OCL), participants were required to indicate by pressing a key if they had seen the card that appeared on the screen before within the same task. The task measures visual learning and memory. The principal outcome variable was the proportion of correct responses. In the Continuous Paired Association Learning Task (CPAL), participants were asked to recall the correct spatial location of a previously presented object. This task evaluates visual and spatial learning and memory. The principal outcome measure was the total number of errors made.
Confounding Factors and Covariates
Potential confounding factors included in the regression models were as follows: maternal age, maternal education, family income, and the biological father’s presence in the family. Gender was also included in the regression models.
Maternal data were collected during pregnancy. Maternal age was classified as a continuous variable. Maternal education was categorized as follows: none, trade certificate/apprenticeship, non-degree professional registration, college/TAFE diploma, university degree, and other.
Family data were collected at the 1-year follow-up between 1990 and 1993. Family income was coded into five categories: <AUD $7,000; $7,000–$11,999; $12,000–$23,999; $24,000–$35,000; and ≥$36,000. The presence of the biological father within the family was categorized as: “yes” and “no.”
Statistical Analysis
Data were scrutinized for missing values, invalid data and normality. We excluded participants who failed to complete 75% of the trials and did not pass the integrity checks for the DET, IDN, and OCL tasks. This procedure was followed as per CogState guidelines (29). Integrity check criteria were as follows: DET speed scores <IDN speed scores and DET accuracy >90%; IDN accuracy >80%; OCL accuracy >50%. The distributions of the total error rate for the CPAL task were positively skewed; therefore, a log10 transformation was applied to the data to achieve normality. The reaction time scores for the DET and IDN were also log10 transformed and the square root of the proportion of correct responses for the OCL task was arcsine transformed. These standard procedures with respect to the cognitive date were undertaken by CogState Ltd., Melbourne, VIC, Australia.
We analyzed the data in three steps. Group comparisons, using Pearson chi-square tests for categorical variables and independent sample t-tests for continuous variables, were initially undertaken to compare characteristics of participants who did or did not complete the CogState cognitive battery. Means of outcome variables were compared between breastfeeding categories, using one-way ANOVAs, and correlations were computed between diet scores and the outcome variables. Lastly, General Linear Modeling was implemented to perform multivariable regression analyses to examine the associations between breastfeeding duration (as a continuous variable and categorized as <4 months, ≥4 months) and nutrition in early childhood (measured by the continuous diet score at age 1 year) with the CogState outcomes as described previously. These analyses were performed on each cognitive outcome separately, adjusting for relevant confounders and covariates including gender, maternal age, maternal education, family income, and presence of the biological father in the family. To assess a potential moderating effect of gender, initially gender × diet and gender × breastfeeding interaction terms were included in all models, followed by a separate analysis undertaken for males and females if a significant interaction was present. All analyses were performed using IBM SPSS Statistics 19 with α = 0.05 set as the criterion for statistical significance.
Results
Sample Attrition
Table 1 shows the relevant descriptive statistics concerning the participants who took part in CogState testing. Family income was >AUS$36,000 in 37% of participants at age 1, while 40% of the mothers did not have any formal qualifications and only 13% reported possession of a university degree. Breastfeeding continued for more than 4 months in 69% of the participants. Adolescents who participated in the CogState cognitive battery at the 17-year follow-up were born to mothers who were older (p < 0.001), more educated (p < 0.001), had a greater probability of living with the child’s biological father (p < 0.008), had higher family incomes (p < 0.001), and were more likely to have breastfed their child for a longer period (p < 0.001), than study participants who did not take part (Table S3 in Supplementary Material).
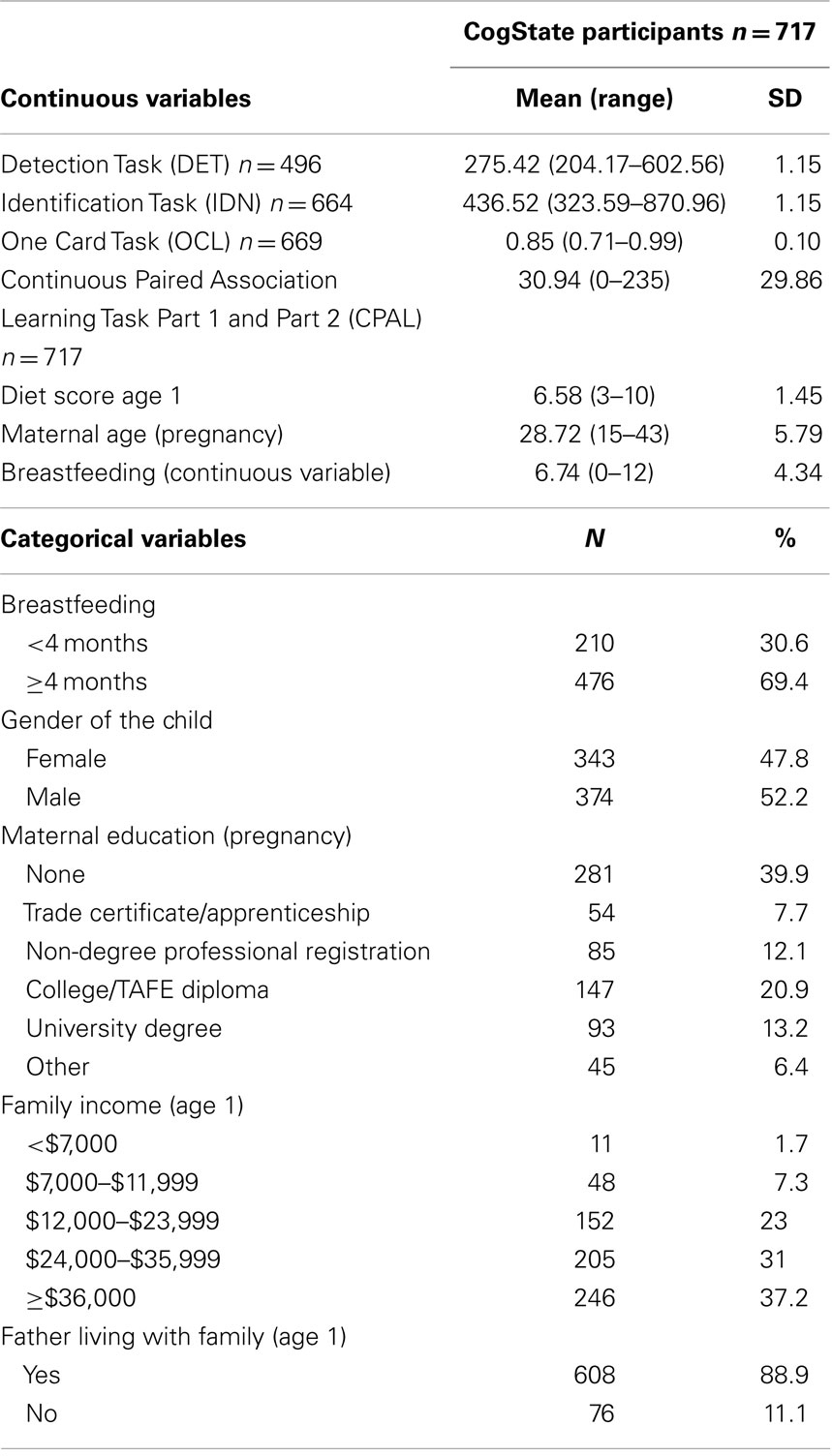
Table 1. Descriptive statistical data for participants in CogState at 17 years of age (n = 717) in the Western Australian Pregnancy Cohort (Raine) Study.
Breastfeeding
Unadjusted mean scores for each cognitive measure according to breastfeeding categories are shown in Table 2. There were significant differences in mean reaction times on the DET (<4 months: 280.67 ms; ≥4 months: 270.33 ms; p = 0.007) and the IDN (<4 months: 448.44 ms; ≥4 months: 434.61 ms; p = 0.010) tasks. In the multivariable regression models, after adjusting for confounders and covariates (Table 3), there was a significant interaction between gender and breastfeeding on the DET task, i.e., whether or not a child was breastfed for 4 months or longer had a differential effect on the reaction time on DET task depending on the child’s gender (F = 4.40, p = 0.037). There was also a significant interaction between gender and breastfeeding on DET task performance when breastfeeding was treated as a continuous variable (F = 4.53, p = 0.034). Therefore, separate analyses for males and females were conducted on the DET task. These gender-specific analyses revealed that for males there was a significant association between breastfeeding for 4 months or longer and faster reaction time on the DET task (β = −0.023, 95% CI: −0.043; −0.002, p = 0.029), but not for females (β = 0.004, 95% CI: −0.013; 0.021, p = 0.642). The associations between diet and breastfeeding as a continuous variable were not significant in either males (β = −0.002, 95% CI: −0.004; 0.000, p = 0.079) or females (β = 0.001, 95% CI: −0.001; 0.003, p = 0.441).
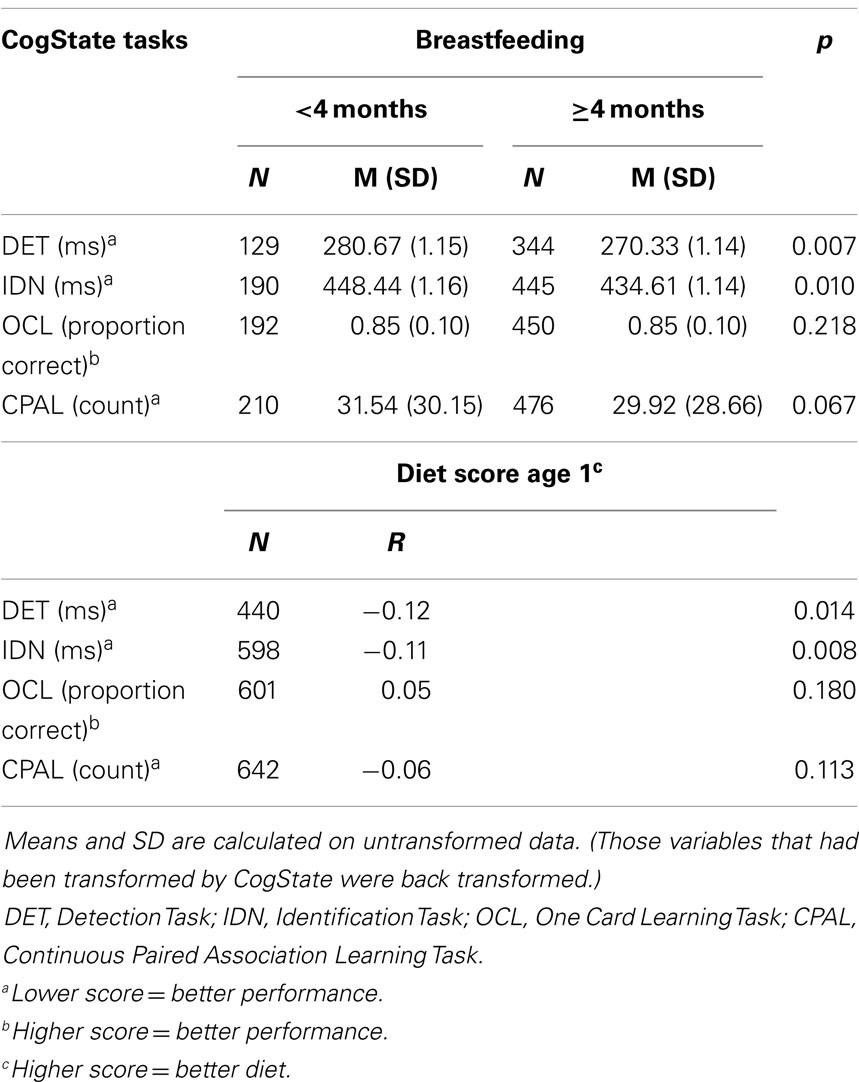
Table 2. Unadjusted mean scores (and SD) for each cognitive measure according to breastfeeding category, and Pearson’s correlation between diet score at age one and each cognitive measure.
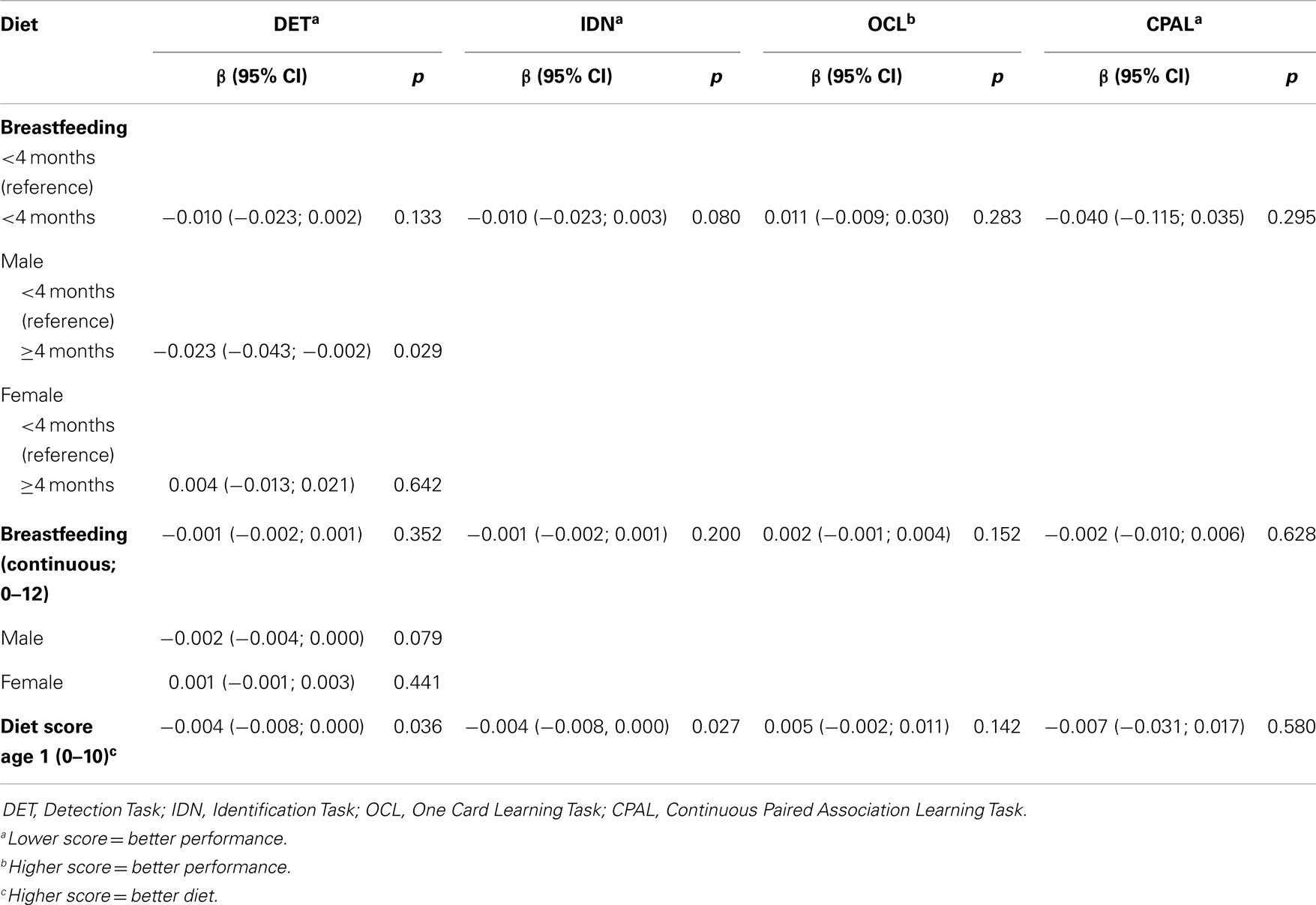
Table 3. Multiple Regression analyses evaluating (a) breastfeeding and cognitive performance at 17 years of age and (b) diet score at 1 year and cognitive performance at 17 years of age in the Western Australian Pregnancy Cohort Study adjusted for sex, family income (age one), father’s presence in the family (age one), maternal age (pregnancy), and maternal education (pregnancy).
Diet Scores at Age 1
Pearson’s correlations between diet score at 1 year and each cognitive measure are presented in Table 2. Negative correlations between the diet score and the response times on the DET (r = −0.117, p = 0.014) and IDN (r = −0.108, p = 0.008) tasks were observed. After adjustment, a higher diet score at 1 year was associated with faster reaction times on the DET (β = −0.004, 95% CI: −0.008; 0.000, p = 0.036) and IDN (β = −0.004, 95% CI: −0.008; 0.000, p = 0.027; Table 3) tasks. Gender × diet interactions were not significant in any models examining diet score.
Discussion
In this study, we examined the long-term impact of breastfeeding and nutrition in early childhood on the cognitive ability of adolescents. We found that boys who had been breastfed for 4 months or longer showed better cognitive performance with regard to psychomotor speed reflected by faster reaction times on the CogState DET. This association remained significant after adjustment for the socioeconomic covariates maternal age and education, family income, and the presence of the biological father in the family. We did not find any association between breastfeeding and cognitive performance in girls. We found that better diet quality at 1 year was associated with faster reaction time on the CogState DET and IDN task in both boys and girls.
The effects of breastfeeding on cognitive capacity in adolescence (11, 34–36) and adulthood have been studied previously (37, 38). Our results are similar to the results reported by Kafouri et al. (34). These researchers showed a positive association between a longer duration of breastfeeding and full scale and performance (but not verbal) IQ, as measured on the Wechsler Intelligence Scale for Children III (WISC-III). The CogState test battery does not measure IQ per se and we were not able to compute an overall composite score of test performance (as the measurement parameters were different across the CogState tasks). However, we found that one of the key CogState outcome variables measuring psychomotor speed (an important element of the “performance” component of intelligence) was significantly associated with breastfeeding duration in boys. In the study conducted by Kafouri et al. (34), a longer breastfeeding duration was associated with higher performance IQ. This component of IQ is measured by tasks that evaluate how accurately and quickly people can perform; this capacity is conceptually linked to psychomotor speed. Therefore, there appears to be consistency between our current findings and those reported by Kafouri et al. (34).
Gender benefits related to breastfeeding have been reported by Isaacs et al. (35). In their study, an association was observed between IQ, brain volume (specifically white matter volume), and breast milk intake in premature infants who were subsequently evaluated during adolescence. This association was highly significant in males, but not in females. Isaacs et al. (35) reported an association predominantly observed in males, on verbal and full scale IQ (measured by the WISC) but not on performance IQ. Gender differences have previously been noted by Oddy et al. (39) in the Raine cohort; i.e., longer breastfeeding duration was significantly associated with better academic performance (mathematics and spelling) at age 10 years in boys only. Our study has shown that this gender difference continues to 17 years with regard to cognitive test performance. It should be noted that, in contrast to the findings of Isaacs et al. (35), the gender difference observed in our study was reflected in a measure conceptually related to “performance” IQ rather than to “verbal” IQ.
Gender differences in neural development, brain structure, and neurocognitive capacity have been reported in numerous studies, and confirmed by neuroimaging (40–42). The difference in males and females’ neurocognitive development may be attributed to sex hormones, as receptors for these hormones can be found across several brain regions (43). Other factors potentially mediating the gender differences observed in our study could include the neuroprotective effect of the sex hormone estradiol in females (44). The lack of estradiol in males is consistent with the notion of greater vulnerability of the male brain to external events and the nutritional environment; therefore, it is plausible to suggest that a shorter duration of breastfeeding would exert a more detrimental effect on boys’ neurocognitive development than on girls’ cognitive capacity.
To our knowledge, there is only one published study that has examined the long-term effect of dietary patterns during the early years on cognitive functioning. Smithers et al. (16) analyzed dietary pattern trajectories from 6 to 24 months of age and cognitive performance at age 8 and 15 years. These researchers observed weak but positive associations between early diet and cognitive functioning measured later in life. These findings are supported by our study, whereby a better diet quality during the infant/toddler years was associated with higher levels of cognitive performance in adolescence.
We showed that performance on the DET was significantly associated with breastfeeding duration in boys and performance on the DET and IDN task was significantly associated with diet at 1 year in the whole cohort. A possible explanation of these results concerns the ontogeny of different neurocognitive functions. Specifically, the DET and IDN tasks are among the most “process pure” tasks in cognitive terms from those tests in the CogState test battery that were applied in the current study. These tasks evaluate elementary psychomotor functioning and attention using reaction time as the key performance parameter. The psychomotor functions underlying reaction time represent some of the earliest capacities to develop, i.e., in terms of the fundamental cognitive building blocks that are subserved by the brain (45). More complex cognitive capacities emerge and develop until late adolescence and early adulthood (46), aligned with the maturation of specific brain areas (for example, the frontal lobes) over a similar time frame (4).
With respect to biological mechanisms underlying the current findings, the long-chain polyunsaturated fatty acids (LCPUFAs) – specifically omega-3 fatty acid docosahexaenoic acid (DHA) in breast milk – are considered to be key factor/s that may increase the intelligence of breastfed individuals. However, recent Cochrane reviews did not support this hypothesis, as significant associations between LCPUFA supplementation and cognitive development in term infants (47) or pre-term infants (48) were not found. In contrast, another review by Hoffman et al. (49) suggested that supplementation with a similar level of DHA to breast milk in formula milk resulted in higher cognitive performance in young children who were born full-term. Further, Issacs et al. (35) proposed that cholesterol (which is a significant component of breast milk) may exert a beneficial influence on children’s cognitive development through breastfeeding. Cholesterol is important in neuronal myelination and has been implicated in adult cognitive performance (35).
We found that a healthier diet during infancy may exert long-lasting cognitive benefits. The mechanism/s underlying these effects are likely to be linked to the high nutritional sensitivity of brain development in the critical early period of life. Specifically, the brain reaches 80% of its adult weight during the first 2 years of life due to relatively fast growth (1, 2). Furthermore, during the first year of life, structural and metabolic changes (including increased glucose metabolism, enhanced capillary density, and dendritic field and white matter myelination) commence in the cortex, reflective of early maturational processes (50–52).
Some of the strengths of our study include the use of a prospective design in a large, well-researched cohort with the ability to study the long-term effects of breastfeeding and nutrition in early childhood on cognitive capacities in adolescence. Cognitive functioning was evaluated using a computerized cognitive battery (CogState) representing a valid, reliable, and sensitive method for detecting differences in cognitive performance (29, 30).
Our study also has some limitations. First, the semi-quantitative dietary data that we obtained may not accurately represent the child’s usual diet. Second, although we did not have access to data on maternal intelligence per se, we adjusted for maternal education, which has been shown to be a reliable surrogate of mother’s intelligence (53). Third, due to the lack of CogState data for the entire cohort, we were only able to analyze data from the subset who completed CogState testing. These individuals differed significantly in socio-demographic characteristics from non-participants as specified earlier. A more representative sample of the cohort would contain greater variation in predictor and outcome variables. Therefore, the impact of breastfeeding and early diet on cognitive function in adolescence may be biased conservatively. We adjusted for the most relevant socio-demographic factors, maternal age, maternal education, family income, and the biological father’s presence in the family, but we also acknowledge other confounding not adjusted for. Finally, because this is an observational study, we cannot infer causality between breastfeeding/diet and cognitive outcomes.
In summary, we observed that nutrition in early life (including breastfeeding and diet at 1 year) manifested a significant association with cognitive performance in adolescents, specifically on more fundamental cognitive tasks. We identified a gender difference, indicating that breastfeeding for 4 months or longer was particularly important for boys in relation to long-term cognitive development. Further research is needed in this important area; for example, using structural and functional neuroimaging to evaluate the putative neurological mechanisms linking nutrition to neurocognitive development, and the specific biological mechanisms underlying the gender differences noted in our study.
Conflict of Interest Statement
The authors declare that the research was conducted in the absence of any commercial or financial relationships that could be construed as a potential conflict of interest.
Acknowledgments
We would like to thank the following: the Raine Study participants and their families for ongoing participation in the study; the Raine Study Team for the co-ordination of the study and for the collection of the data presented here; the National Health and Medical Research Council (NHMRC) of Australia for their contribution to funding the Raine study over the past 22 years; the Telethon Kids Institute (previously known as Telethon Institute for Child Health Research), the University of Western Australia (UWA), the Raine Medical Research Foundation, UWA Faculty of Medicine, Dentistry and Health Sciences, the Women’s and Infant’s Research Foundation (KEMH), Curtin University, and Edith Cowan University for providing funding for the Core Management of the Raine Study. We are very grateful to the Raine Medical Research Foundation and the NHMRC for funding the collection of antenatal data and the 1 year follow-up. We would also like to specifically acknowledge the NHMRC Program and Project Grants that supported the 17-year follow-up of the Raine cohort. We are very grateful to Denise Anderson and Angela Jacques for statistical advice and assistance with the manuscript. Funding: Dr. Anett Nyaradi is supported by an Australian Postgraduate Award and the Western Australian Pregnancy Cohort (Raine) Scholarships. Professor Wendy Oddy is funded by a National Health and Medical Research Council Population Health Research Fellowship. Dr Siobhan Hickling is an Assistant Professor at The UWA. Dr. Jianghong Li is a Senior Scientist at the Social Research Centre in Berlin (Germany), an Adjunct Associate Professor at The UWA and Adjunct Senior Research Fellow at Curtin University. Clinical Professor Jonathan Foster is supported by a Curtin University Senior Research Fellowship and by the Health Department of Western Australia.
Supplementary Material
The Supplementary Material for this article can be found online at http://www.frontiersin.org/Journal/10.3389/fnut.2015.00002/abstract
Abbreviations
CPAL, continuous paired association learning task; DET, detection task; IDN, identification task; OCL, one card learning task.
References
1. Bryan J, Osendarp S, Hughes D, Calvaresi E, Baghurst K, van Klinken J-W. Nutrients for cognitive development in school-aged children. Nutr Rev (2004) 62(8):295–306. doi: 10.1111/j.1753-4887.2004.tb00055.x
Pubmed Abstract | Pubmed Full Text | CrossRef Full Text | Google Scholar
2. Lenroot RK, Giedd JN. Brain development in children and adolescents: insights from anatomical magnetic resonance imaging. Neurosci Biobehav Rev (2006) 30(6):718–29. doi:10.1016/j.neubiorev.2006.06.001
Pubmed Abstract | Pubmed Full Text | CrossRef Full Text | Google Scholar
3. Toga AW, Thompson PM, Sowell ER. Mapping brain maturation. Trends Neurosci (2006) 29(3):148–59. doi:10.1016/j.tins.2006.01.007
4. Giedd J, Stockman M, Weddle C, Liverpool M, Alexander-Bloch A, Wallace G, et al. Anatomic magnetic resonance imaging of the developing child and adolescent brain and effects of genetic variation. Neuropsychol Rev (2010) 20(4):349–61. doi:10.1007/s11065-010-9151-9
Pubmed Abstract | Pubmed Full Text | CrossRef Full Text | Google Scholar
5. Nyaradi A, Li J, Hickling S, Foster J, Oddy WH. The role of nutrition in children’s neurocognitive development, from pregnancy through childhood. Front Hum Neurosci (2013) 7:97. doi:10.3389/fnhum.2013.00097
Pubmed Abstract | Pubmed Full Text | CrossRef Full Text | Google Scholar
6. Rey J. Breastfeeding and cognitive development. Acta Paediatr (2003) 92:11–8. doi:10.1111/j.1651-2227.2003.tb00659.x
7. Drane DL, Logemann JA. A critical evaluation of the evidence on the association between type of infant feeding and cognitive development. Paediatr Perinat Epidemiol (2000) 14(4):349–56. doi:10.1046/j.1365-3016.2000.00301.x
Pubmed Abstract | Pubmed Full Text | CrossRef Full Text | Google Scholar
8. Jain A, Concato J, Leventhal JM. How good is the evidence linking breastfeeding and intelligence? Pediatrics (2002) 109(6):1044–53. doi:10.1542/peds.109.6.1044
Pubmed Abstract | Pubmed Full Text | CrossRef Full Text | Google Scholar
9. Michaelsen KF, Lauritzen L, Mortensen EL. Effects of breast-feeding on cognitive function breast-feeding: early influences on later health. In: Goldberg G, Prentice A, Prentice A, Filteau S, Simondon K, editors. Advances in Experimental Medicine and Biology. (Vol. 639), Springer (2009). p. 199–215.
10. Horwood LJ, Fergusson DM. Breastfeeding and later cognitive and academic outcomes. Pediatrics (1998) 101(1):e9. doi:10.1542/peds.101.1.e9
Pubmed Abstract | Pubmed Full Text | CrossRef Full Text | Google Scholar
11. Greene LC, Lucas A, Livingstone MB, Harland PS, Baker BA. Relationship between early diet and subsequent cognitive performance during adolescence. Biochem Soc Trans (1995) 23(2):376S.
12. Gale CR, Martyn CN, Marriott LD, Limond J, Crozier S, Inskip HM, et al. Dietary patterns in infancy and cognitive and neuropsychological function in childhood. J Child Psychol Psychiatry (2009) 50(7):816–23. doi:10.1111/j.1469-7610.2008.02029.x
Pubmed Abstract | Pubmed Full Text | CrossRef Full Text | Google Scholar
13. Northstone K, Joinson C, Emmett P, Ness A, Paus T. Are dietary patterns in childhood associated with IQ at 8 years of age? A population-based cohort study. J Epidemiol Community Health (2011) 66:624–8. doi:10.1136/jech.2010.111955
Pubmed Abstract | Pubmed Full Text | CrossRef Full Text | Google Scholar
14. Theodore RF, Thompson JMD, Waldie KE, Wall C, Becroft DMO, Robinson E, et al. Dietary patterns and intelligence in early and middle childhood. Intelligence (2009) 37(5):506–13. doi:10.1016/j.intell.2009.07.001
15. Smithers LG, Golley RK, Mittinty MN, Brazionis L, Northstone K, Emmett P, et al. Dietary patterns at 6, 15 and 24 months of age are associated with IQ at 8 years of age. Eur J Epidemiol (2012) 27(7):525–35. doi:10.1007/s10654-012-9715-5
Pubmed Abstract | Pubmed Full Text | CrossRef Full Text | Google Scholar
16. Smithers LG, Golley RK, Mittinty MN, Brazionis L, Northstone K, Emmett P, et al. Do dietary trajectories between infancy and toddlerhood influence IQ in childhood and adolescence? Results from a prospective birth cohort study. PLoS One (2013) 8(3):e58904. doi:10.1371/journal.pone.0058904
Pubmed Abstract | Pubmed Full Text | CrossRef Full Text | Google Scholar
17. Oddy WH, Kendall GE, Blair E, De Klerk NH, Stanley FJ, Landau LI, et al. Breast feeding and cognitive development in childhood: a prospective birth cohort study. Paediatr Perinat Epidemiol (2003) 17(1):81–90. doi:10.1046/j.1365-3016.2003.00464.x
Pubmed Abstract | Pubmed Full Text | CrossRef Full Text | Google Scholar
18. Whitehouse AJO, Robinson M, Li J, Oddy WH. Duration of breast feeding and language ability in middle childhood. Paediatr Perinat Epidemiol (2011) 25(1):44–52. doi:10.1111/j.1365-3016.2010.01161.x
Pubmed Abstract | Pubmed Full Text | CrossRef Full Text | Google Scholar
19. Nyaradi A, Li J, Hickling S, Whitehouse AJO, Foster JK, Oddy WH. Diet in the early years of life influences cognitive outcomes at 10 years: a prospective cohort study. Acta Paediatr (2013) 102(12):1165–73. doi:10.1111/apa.12363
Pubmed Abstract | Pubmed Full Text | CrossRef Full Text | Google Scholar
20. Newnham JP, Evans SF, Michael CA, Stanley FJ, Landau LI. Effects of frequent ultrasound during pregnancy: a randomised controlled trial. Lancet (1993) 342(8876):887–91. doi:10.1016/0140-6736(93)91944-H
Pubmed Abstract | Pubmed Full Text | CrossRef Full Text | Google Scholar
21. Li R, Scanlon KS, Serdula MK. The validity and reliability of maternal recall of breastfeeding practice. Nutr Rev (2005) 63(4):103–10. doi:10.1111/j.1753-4887.2005.tb00128.x
Pubmed Abstract | Pubmed Full Text | CrossRef Full Text | Google Scholar
22. World Health Organization. 55th World Health Assembly: Infant and Young Child Nutrition. Geneva: WHO (2002).
23. McNaughton SA, Ball K, Crawford D, Mishra GD. An index of diet and eating patterns is a valid measure of diet quality in an Australian population. J Nutr (2008) 138(1):86–93.
24. Waijers P, Feskens E, Ocké M. A critical review of predefined diet quality scores. Br J Nutr (2007) 97(02):219–31. doi:10.1017/S0007114507250421
Pubmed Abstract | Pubmed Full Text | CrossRef Full Text | Google Scholar
25. Smith A, Kellett E, Schmerlaib Y. Australian guide to healthy eating. In: Ageing AGDoHa, editor. Background Information for Nutrition Educators. Melbourne, VIC: Commonwealth of Australia (1998).
26. Feskanich D, Rockett HRH, Colditz GA. Modifying the healthy eating index to assess diet quality in children and adolescents. J Am Diet Assoc (2004) 104(9):1375–83. doi:10.1016/j.jada.2004.06.020
Pubmed Abstract | Pubmed Full Text | CrossRef Full Text | Google Scholar
27. Meyerkort CE, Oddy WH, O’Sullivan TA, Henderson J, Pennell CE. Early diet quality in a longitudinal study of Australian children: associations with nutrition and body mass index later in childhood and adolescence. J Dev Orig Health Dis (2012) 3(1):21–31. doi:10.1017/S2040174411000717
Pubmed Abstract | Pubmed Full Text | CrossRef Full Text | Google Scholar
28. National Health and Medical Research Council. Dietary Guidelines for Children and Adolescents in Australia: Incorporating the Infant Feeding Guidelines for Health Workers. Canberra, ACT: Commonwealth of Australia (2003).
29. Collie A, Maruff P, Darby DG, McStephen M. The effects of practice on the cognitive test performance of neurologically normal individuals assessed at brief test-retest intervals. J Int Neuropsychol Soc (2003) 9(03):419–28. doi:10.1017/S1355617703930074
Pubmed Abstract | Pubmed Full Text | CrossRef Full Text | Google Scholar
30. Mollica CM, Maruff P, Collie A, Vance A. Repeated assessment of cognition in children and the measurement of performance change. Child Neuropsychol (2005) 11(3):303–10. doi:10.1080/092970490911306
Pubmed Abstract | Pubmed Full Text | CrossRef Full Text | Google Scholar
31. CogState. CogState Research Melbourne: CogState Limited (2001). Available from: http://www.cogstate.com/go/research
32. Falleti MG, Maruff P, Collie A, Darby DG. Practice effects associated with the repeated assessment of cognitive function using the CogState battery at 10-minute, one week and one month test-retest intervals. J Clin Exp Neuropsychol (2006) 28(7):1095–112. doi:10.1080/13803390500205718
Pubmed Abstract | Pubmed Full Text | CrossRef Full Text | Google Scholar
33. Maruff P, Thomas E, Cysique L, Brew B, Collie A, Snyder P, et al. Validity of the CogState brief battery: relationship to standardized tests and sensitivity to cognitive impairment in mild traumatic brain injury, schizophrenia, and AIDS dementia complex. Arch Clin Neuropsychol (2009) 24(2):165–78. doi:10.1093/arclin/acp010
Pubmed Abstract | Pubmed Full Text | CrossRef Full Text | Google Scholar
34. Kafouri S, Kramer M, Leonard G, Perron M, Pike B, Richer L, et al. Breastfeeding and brain structure in adolescence. Int J Epidemiol (2012) 42(1):150–9. doi:10.1093/ije/dys172
35. Isaacs EB, Fischl BR, Quinn BT, Chong WK, Gadian DG, Lucas A. Impact of breast milk on intelligence quotient, brain size, and white matter development. Pediatr Res (2010) 67(4):357–62. doi:10.1203/PDR.0b013e3181d026da
Pubmed Abstract | Pubmed Full Text | CrossRef Full Text | Google Scholar
36. Rodgers B. Feeding in infancy and later ability and attainment: a longitudinal study. Dev Med Child Neurol (1978) 20(4):421–6. doi:10.1111/j.1469-8749.1978.tb15242.x
37. Mortensen EL, Michaelsen K, Sanders SA, Reinisch J. The association between duration of breastfeeding and adult intelligence. JAMA (2002) 287(18):2365–71. doi:10.1001/jama.287.18.2365
Pubmed Abstract | Pubmed Full Text | CrossRef Full Text | Google Scholar
38. Richards M, Hardy R, Wadsworth ME. Long-term effects of breast-feeding in a national birth cohort: educational attainment and midlife cognitive function. Public Health Nutr (2002) 5(5):631–5. doi:10.1079/PHN2002338
Pubmed Abstract | Pubmed Full Text | CrossRef Full Text | Google Scholar
39. Oddy WH, Li J, Whitehouse AJ, Zubrick SR, Malacova E. Breastfeeding duration and academic achievement at 10 years. Pediatrics (2011) 127(1):e137–45. doi:10.1542/peds.2009-3489
Pubmed Abstract | Pubmed Full Text | CrossRef Full Text | Google Scholar
40. Schmithorst VJ. Developmental sex differences in the relation of neuroanatomical connectivity to intelligence. Intelligence (2009) 37(2):164–73. doi:10.1016/j.intell.2008.07.001
Pubmed Abstract | Pubmed Full Text | CrossRef Full Text | Google Scholar
41. Schmithorst VJ, Holland SK. Functional MRI evidence for disparate developmental processes underlying intelligence in boys and girls. Neuroimage (2006) 31(3):1366–79. doi:10.1016/j.neuroimage.2006.01.010
Pubmed Abstract | Pubmed Full Text | CrossRef Full Text | Google Scholar
42. Gilmore J, Lin W, Prastawa M, Looney C, Vetsa Y, Knickmeyer R, et al. Regional gray matter growth, sexual dimorphism, and cerebral asymmetry in the neonatal brain. J Neurosci (2007) 27(6):1255–60. doi:10.1523/JNEUROSCI.3339-06.2007
Pubmed Abstract | Pubmed Full Text | CrossRef Full Text | Google Scholar
43. De Bellis MD, Keshavan MS, Beers SR, Hall J, Frustaci K, Masalehdan A, et al. Sex differences in brain maturation during childhood and adolescence. Cereb Cortex (2001) 11(6):552–7. doi:10.1093/cercor/11.6.552
Pubmed Abstract | Pubmed Full Text | CrossRef Full Text | Google Scholar
44. Arevalo M-A, Santos-Galindo M, Bellini M-J, Azcoitia I, Garcia-Segura LM. Actions of estrogens on glial cells: implications for neuroprotection. Biochim Biophys Acta (2010) 1800(10):1106–12. doi:10.1016/j.bbagen.2009.10.002
45. Levitt P. Structural and functional maturation of the developing primate brain. J Pediatr (2003) 143(4 Suppl1):35–45. doi:10.1067/s0022-3476(03)00400-1
46. Anderson P. Assessment and development of executive function (EF) during childhood. Child Neuropsychol (2002) 8(2):71–82. doi:10.1076/chin.8.2.71.8724
Pubmed Abstract | Pubmed Full Text | CrossRef Full Text | Google Scholar
47. Simmer K, Patole S, Rao S. Long chain polyunsaturated fatty acid supplementation in infants born at term. Cochrane Database Syst Rev (2011) 12:CD000376. doi:10.1002/14651858.CD000376.pub3
48. Schulzke S, Patole S, Simmer K. Long-chain polyunsaturated fatty acid supplementation in preterm infants. Cochrane Database Syst Rev (2011) 2:CD000375. doi:10.1002/14651858.CD000375.pub4
49. Hoffman DR, Boettcher JA, Diersen-Schade DA. Toward optimizing vision and cognition in term infants by dietary docosahexaenoic and arachidonic acid supplementation: a review of randomized controlled trials. Prostaglandins Leukot Essent Fatty Acids (2009) 81(2–3):151–8. doi:10.1016/j.plefa.2009.05.003
Pubmed Abstract | Pubmed Full Text | CrossRef Full Text | Google Scholar
50. Chugani HT, Phelps ME, Mazziotta JC. Positron emission tomography study of human brain functional development. Ann Neurol (1987) 22(4):487–97. doi:10.1002/ana.410220408
Pubmed Abstract | Pubmed Full Text | CrossRef Full Text | Google Scholar
51. Chugani HT. A critical period of brain development: studies of cerebral glucose utilization with PET. Prev Med (1998) 27(2):184–8. doi:10.1006/pmed.1998.0274
Pubmed Abstract | Pubmed Full Text | CrossRef Full Text | Google Scholar
52. Paus T, Collins DL, Evans AC, Leonard G, Pike B, Zijdenbos A. Maturation of white matter in the human brain: a review of magnetic resonance studies. Brain Res Bull (2001) 54(3):255–66. doi:10.1016/s0361-9230(00)00434-2
Pubmed Abstract | Pubmed Full Text | CrossRef Full Text | Google Scholar
Keywords: nutrition, breastfeeding, cognitive performance, CogState, early childhood, adolescence, Raine study
Citation: Nyaradi A, Oddy WH, Hickling S, Li J and Foster JK (2015) The relationship between nutrition in infancy and cognitive performance during adolescence. Front. Nutr. 2:2. doi: 10.3389/fnut.2015.00002
Received: 12 September 2014; Accepted: 21 January 2015;
Published online: 11 February 2015.
Edited by:
Guido Frank, University of Colorado Anschutz Medical Campus, USACopyright: © 2015 Nyaradi, Oddy, Hickling, Li and Foster. This is an open-access article distributed under the terms of the Creative Commons Attribution License (CC BY). The use, distribution or reproduction in other forums is permitted, provided the original author(s) or licensor are credited and that the original publication in this journal is cited, in accordance with accepted academic practice. No use, distribution or reproduction is permitted which does not comply with these terms.
*Correspondence: Anett Nyaradi, Telethon Kids Institute, PO BOX 855 West, Perth, WA 6872, Australia e-mail:YW5ldHQubnlhcmFkaUB0ZWxldGhvbmtpZHMub3JnLmF1