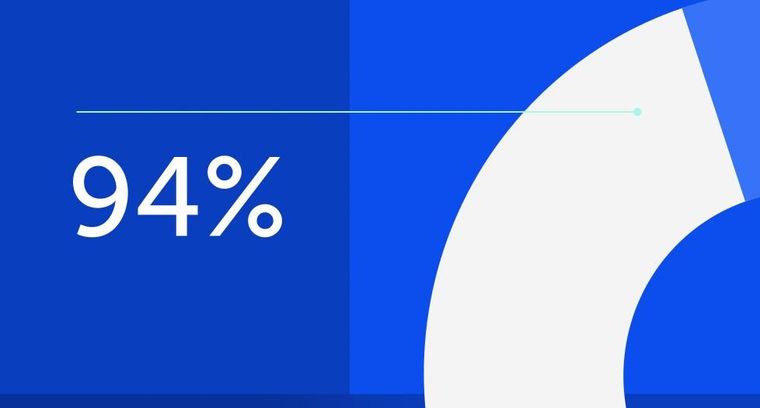
94% of researchers rate our articles as excellent or good
Learn more about the work of our research integrity team to safeguard the quality of each article we publish.
Find out more
ORIGINAL RESEARCH article
Front. Vet. Sci., 05 December 2024
Sec. Comparative and Clinical Medicine
Volume 11 - 2024 | https://doi.org/10.3389/fvets.2024.1497817
Assessing the pathogenicity of a disease-associated genetic variant in animals accurately is vital, both on a population and individual scale. At the population level, breeding decisions based on invalid DNA tests can lead to the incorrect inclusion or exclusion of animals and compromise the long-term health of a population, and at the level of the individual animal, lead to incorrect treatment and even life-ending decisions. Criteria to determine pathogenicity are not standardized, i.e., no guidelines for animal variants are available. Here, we aimed to develop and validate guidelines to be used by the community for Mendelian disorders in domestic animals to classify variants in categories based on standardized criteria. These so-called animal variant classification guidelines (AVCG) were based on those developed for humans by The American College of Medical Genetics and Genomics (ACMG). In a direct comparison, 83% of the pathogenic variants were correctly classified with ACMG, while this increased to 92% with AVCG. We described methods to develop datasets for benchmarking the criteria and identified the most optimal in silico variant effect predictor tools. As the reproducibility was high, we classified 72 known disease-associated variants in cats and 40 other disease-associated variants in eight additional species.
The pace at which disease-associated genetic variants in animals are discovered is increasing and is associated with technological advancement (1) (Figure 1). Genetic tests for these variants have become very accessible as well, also for a layman audience thanks to, e.g., the direct-to-consumer genetic testing companies. The challenge of the interpretation of DNA variants and the translation to the clinic is well known in human medicine (2–5). The functional mechanisms of identified disease-associated DNA variants are often unclear and the associated diseases may have variable expression and incomplete penetrance, which leads to ambiguous interpretation of the pathogenicity of given variants. In animals, these challenges have also been recognized, nevertheless, no standardized evaluation protocol of variant pathogenicity has been developed (6–8). The potential consequences associated with misinterpretation of the importance of genetic variants however are far reaching (9–11). Ranging from incorrect treatment to even euthanasia, individual animals can suffer directly, however, the consequences can even negatively affect the entire population by impacting breeding decisions (9–11). As genetic diversity in several cat and dog breeds is low compared to the general human population, the exclusion of animals based on invalid associations can drive a further increase in other disease prevalences, and substantiate the concerns linked to animal welfare (12–18).
Figure 1. Number of new variants published per five year-period for the three species with currently over >100 published disease-causing variants in OMIA (1). While the extent is species-dependent, an increase can be seen in all three.
The American College of Medical Genetics and Genomics (ACMG) developed, and updates, widely used guidelines in human genetics to provide guidance when interpreting the potential pathogenicity of genetic variants (2, 19–28). While these ACMG guidelines have been considered a few times more recently in animal genetics, their implementation is overall very limited, and concerns about the appropriateness of some criteria have led to the exclusion of certain criteria in some publications (29–32). More recently, applying the ACMG guidelines has been objectively shown to lead to misclassification of variants in cats (6). Currently, no guidelines have been established that assist the process of variant classification in animals, which implies that decisions on pathogenicity are not standardized and are based on individual investigator experience alone.
We present guidelines tailored for variant classification in domestic animals and demonstrate their superiority on animal variant classification relative to the ACMG guidelines. To benchmark these animal variant classification guidelines (AVCG), a reference dataset was created, with allele frequencies (AF) derived from a large population study. An evaluation of in silico variant effect predictor tools was performed. The reproducibility of labelling variants in a five-category classification system was examined and variants in nine species were evaluated.
Samples were non-invasive buccal swabs, collected by the animal owner or in veterinary practices, or EDTA whole blood, collected by veterinary clinics, in accordance with international standards for animal care and research. In some cats, blood was obtained as part of routine clinical procedures for diagnostic purposes, at the request and with the consent of the owner. As these samples were from client-owned cats for which no harmful invasive procedures were performed, there was no animal experimentation according to the legal definition in Europe (Subject 5f of Article1, Chapter I of the Directive 2010/63/UE of the European Parliament and of the Council). All cat owners provided consent for the use of their cat’s DNA sample in scientific research. Written informed consent was obtained from the owners for the participation of their animals in this study.
The feline AF dataset was based on non-invasive cheek swab samples collected by cat owners, and either blood or cheek swab samples collected at certified veterinary clinics, for submission to commercial DNA testing. The samples submitted for MyCatDNA™/Optimal Selection™ Feline (Wisdom Panel, Helsinki, Finland and Wisdom Panel, Vancouver, WA, USA, respectively) DNA genotyping based on a custom-designed Illumina® Infinium HD array between September 2016 and November 2023 consist of 30,577 individual cat samples, of these 11,036 samples (36%) have been previously published (33) and 19,541 samples are new entries. The samples submitted for Antagene DNA genotyping consist of 32,841 individual cat samples (Antagene, La Tour de Salvagny, France). The owners provided written consent for data use in research upon submission of samples for commercial genetic testing. All tests are routinely run and offered commercially. Geographically, these samples originate from all over the world, with the majority from Northern America (USA, Canada) and Europe (>20 countries) and some samples from Africa and Asia.
The breed of a cat was reported by its owner typically with additional accompanying information confirming registration under The International Cat Association, Fédération Internationale Féline, Livre Officiel des Origines Félines, The Cat Fanciers’ Association, or World Cat Federation standards. Additional breeds not yet recognized by any major breed registry but with an established community of breed hobbyists were also considered breeds for the purposes of this study. A cautionary note specifying examples regarding this aspect was added (Supplementary Table S1). The non-pedigreed cat sample set consisted of mixed breeds, breed crosses, or random-bred cats.
In agreement with recommendations assisting the development of guidelines, the following steps were taken (34, 35). Prior to the actual development process, the scope and starting point were decided on. The starting point for this process were the original 2015 ACMG guidelines used in human medicine (Supplementary Table S2) (2). Throughout the manuscript, when a criterion from the original ACMG guidelines is mentioned, the criterion name will always be preceded by ACMG. If this is not the case, the criterion mentioned is part of the newly developed AVCG.
A multidisciplinary group was composed including veterinarians, geneticists from universities, and from commercial laboratories offering DNA-tests, and fell within the recommended 6 to 15 members (35). Individuals from this multidisciplinary group have worked and/or published on various species, including cats, dogs, horses and chickens. The development process was divided into three phases: the pre-meeting preparatory phase (phase one), a group decision phase (phase two), and the optimization phase (phase three) (34, 35). The first phase was an individual evaluation phase, whereas the focus group approach was used in phase two and three. In the first phase, each member of the multidisciplinary team independently provided remarks and voted (accept, revise, or remove) on each of the ACMG criteria, as well as had an opportunity to propose new criteria. The remarks and votes from phase one were shared anonymously in the decision phase in a powerpoint presentation (phase two). Decisions to retain, revise, or exclude ACMG criteria for AVCG were made by anonymous voting [using the Wooclap platform (wooclap.com)] and were based on a two-thirds majority rule. Modifications were linked to the text of the criterion and/or the weight assigned to that criterion. Finally, in the optimization phase, individuals who worked with the guidelines could propose changes, which were again voted on anonymously by the entire group (phase three). All group meetings (phase two and phase three) were recorded.
While various classification development approaches exist, the focus group approach was chosen in phases two and three as the goal was to opt for a method that allowed (1) a group discussion to stimulate new ideas and insights (requirement one), and (2) a safe decision environment in which personal opinions can be reflected (requirement two) (36). While focus groups allow a thorough discussion and enrichment of ideas, potential drawbacks are the lack of confidentiality and power relations (36). To resolve that and fulfil requirement two, voting was anonymous in phases two and three and participants were invited to submit remarks prior to the decision phase, and these were also included anonymously in the presentations used in phase two. During the entire process, the stepwise development was tracked.
To evaluate (1) the performance of both the ACMG and the AVCG, and, (2) the accuracy of in silico tools, datasets of “true” disease-causing variants and benign variants had to be created. A systematic review according to the PRISMA guidelines was conducted to evaluate the existing methodologies for selecting pathogenic and benign variants in studies involving these tools (37). The process is explained in Supplementary Data S1, graphically represented in Supplementary Figure S1 and methods are summarized in Table 1.
Based on the summarized methods (Table 1), the following procedure was followed to collect a set of pathogenic variants. Every geneticist was asked to independently “provide at least five variants that he/she considers to be pathogenic without a doubt.” A list was compiled, removing all duplicates. Subsequently, these variants were manually checked and were excluded if they met any of the following exclusion criteria: somatic variants associated with cancer, variants (risk/protective) associated with complex traits, structural variants (defined as sequence variants >50 bp in size (38)). Next, variants were removed if (1) the original paper could not be retrieved and/or (2) if there were errors in the paper (e.g., if the effect of the variant did not match the current annotation or the annotation that was used at the time of publication) and/or if (3) the paper was considered to be too old to make a proper judgment (e.g., some papers predated the public availability of genome sequences, as such it was not always possible to link the sequence data published in the paper to any of the current resources), and/or if (4) the variant caused a non-disease Mendelian phenotype.
To be retained in the final list, variants had to pass all the aforementioned exclusion criteria and consistent and independent labelling as “without a doubt pathogenic” by at least three experienced (defined as >10 years of experience in the field) geneticists. If a variant was put on the list independently by three geneticists during the collection process, the variant was considered pathogenic. If a variant was proposed by less than three geneticists, other geneticists than the ones that put it on the list, were asked to review the variant. If the variant was only reported once, two other geneticists were consulted, if it was reported twice, then only one other geneticist was consulted. Variants were allocated to reviewing geneticists randomly and during the entire process (submission for the initial list and reviewing of the remainder) no instructions were given on the methodology, i.e., every geneticist did this at their own discretion. The concordance of the independent evaluations was tracked. If a variant was not consistently labelled by at least three geneticists as “without a doubt pathogenic,” it was not included in the “truth” pathogenic dataset. At that moment, however, it was eligible for the subgroup of variants that were not consistently considered pathogenic by all three evaluators, which were used to assess inter-evaluator agreement of classification (see “2.7 Evaluation of the performance of variant classification guidelines: inter-evaluator agreement of classification”).
This dataset was completed with AF data as this is an important part of the evaluation process in the ACMG guidelines. To provide a standardized and as complete dataset as possible, allelic frequency data from two commercial laboratories (Antagene and Wisdom Panel) were added for every variant that was routinely tested (for details: see “2.2 Samples”).
Similarly, based on the results of the systematic review according to the PRISMA guidelines (strategy detailed in Supplementary Data S1, graphically represented in Supplementary Figure S1 and methods summarized in Table 1), the following selection strategy was used to retrieve variants that were most likely benign (37). First, a random selection of a variant with a certain effect was done from BioMart (39). The following settings were used: (1) Database: Ensembl Variation 111, Cat short variants (SNPs and indels excluding flagged variants) (Felis_catus_9.0); (2) Filters: Region: none; General variant filters: none; Gene associated variant filters: “missense_variant,” “frameshift_variant,” “stop gained,” (3) Attributes: variant associated information: variant name, variant source, chromosome/scaffold name, chromosome/scaffold position start (bp), chromosome/scaffold position end (bp) (default settings). The results were exported and a random selection was performed in R version 4.3.2 using a custom script. Second, the gene in which the selected variant resides was checked for a reported association with disease in Online Mendelian Inheritance in Animals (OMIA) (1). Third, a homology-based search through ConVarT was used to identify whether a variant in humans was reported to be pathogenic or likely pathogenic according to ClinVar (40). Only when OMIA yielded no result and the variant was not classified pathogenic or likely pathogenic in ClinVar, the variant was retained in the list. This process was continued until a number equal to the number of pathogenic (missense/nonsense/frameshift) variants was achieved. This list is available in Supplementary Table S3. For splice sites, this approach was not used. As there is no functional proof that a random splice variant from the database will actually result in altered splicing, neither that the absence of such a variant will not, it would not be possible to check whether a tool correctly predicts the effect. However, as the splice site variants from the pathogenic dataset contained both the creation and removal of donor as well as acceptor sites and functional proof for the effect (i.e., cDNA/mRNA sequence evaluation) for seven out of eight variants was provided, these were used to assess the predictions of the tools.
The pathogenic and benign dataset were restricted to one species to avoid potential bias due to species differences; species transferability of AVCG was evaluated in a subsequent dataset (see “2.8. Evaluation of the performance of variant classification guidelines: cross-species classification”). From the nine species mentioned individually on OMIA, the species selected had to have a sufficiently large number of disease-associated variants published (>100) and to ensure maximum confidence in the dataset, the geneticists involved in the variant evaluation, had to have ample experience with that species.
A systematic review according to the PRISMA guidelines was conducted to obtain a list of in silico tools (37). The procedure is described in Supplementary Data S2 and graphically represented in Supplementary Figure S2. After the initial list was compiled, all tools were subsequently evaluated for (1) easy accessibility, defined as whether there is an online interface available (i.e., no download is necessary to use the tool), and (2) whether non-model species were supported. The remaining tools were all included in the benchmark analyses. Two of the remaining tools (PredictSNP and MetaSNP) are metapredictors (i.e., tools that use the output of other tools to predict the effect of variants). An overview of all tools used, is provided in Table 2.
For missense, nonsense and frameshift variants, to evaluate performance, all corresponding variants from the “truth” pathogenic dataset and benign dataset were used, resulting in a balanced design, i.e., an equal number of pathogenic and benign variants was included during benchmarking. For each tool, the number of variants that gave a result, as well as the accuracy, sensitivity, and specificity were calculated. The default settings were used. While most tools dichotomize classification, PANTHER and PolyPhen2 have subcategories (probably/possibly benign or damaging, respectively), which were collapsed into pathogenic or benign (41–43). For splice sites, reporting is slightly different: the output of the tools is not pathogenic or benign, but whether a donor or acceptor splice site is created or not. As such, the overall accuracy is reported.
Whenever >1 tool was available to assess a specific category of variants, the most optimal combination of two tools was identified to adhere to the in silico criterion as mentioned in ACMG and the AVCG. This step was restricted to tools that assessed all variants in their category for two reasons: (1) to achieve general applicability, more versatile tools that score more variants can be more widely used, and (2) to avoid biased accuracy estimates because the omitted variants might be more difficult or easier to score. For missense, nonsense and frameshift variants, most optimal was defined as the combination of tools that led to the least consistent misclassification, i.e., the least false positives (variants from the benign list that were allocated a “pathogenic” label) and false negatives (variants from the pathogenic list that were allocated a “benign” label) relative to the total number of consistent classifications. If there was a tie, the combination that classified most variants correctly, was preferred. For splice sites, the best combination of tools was defined as the two tools that together consistently predicted most often correctly the creation/removal of donor/acceptor sites, respectively.
The variants in the aforementioned “truth” pathogenic variant list were classified twice: once with the traditional ACMG guidelines and once with the newly developed AVCG guidelines (2), based on the data provided in the original publications (with the publications used per variant specified in Supplementary Table S5). This data was complemented with the new criteria added to AVCG (see below) and for the criteria linked with the in silico tools, only the results from those tools that adequately performed (based on “2.5 Selection, analysis, and optimization of the in silico variant effect predictor tools”), were used. To avoid the tendency to look for additional criteria supporting pathogenic classification if the threshold would not be met, the reviewers were only asked to check the criteria and not to calculate the final label. No variants were reviewed by the same investigator who made the original variant discovery. Furthermore, the work was divided, where each time two geneticists reviewed the in silico tools, two others reviewed the newly added criteria and the final two reviewed the remaining criteria. Label assignment was finally done by a different geneticist who adhered to the decision table exactly.
Practically this entails that for each variant, all the evidence of the various criteria that were fulfilled, was weighted and counted to determine a classification. While the decision-making process changed slightly from ACMG to AVCG, a five-category-based classification remained (Table 3) (2). The categories/labels are: pathogenic (P), likely pathogenic (LP), variant of unknown significance (VUS), likely benign (LB) and benign (B). Each category has consequences for clinical decision making and for whether a variant should be included in breeding and/or screening programs. As primarily differences between P/LP and the other three categories might lead to differences in medical management and/or breeding strategies, these are specifically mentioned in subsequent analyses, aside from general classification overviews (3).
Table 3. Description and consequences of each variant pathogenicity classification in the five-category system.
Five evaluators independently assessed a random subset of an overlapping set of variants with the newly developed guidelines. The goal was to provide a set that encompassed variants with various effects (missense/ nonsense/ frameshift/ splice sites) and that vary from easy to more difficult to classify [variants belonging to the set of pathogenic variants (n = 6, see “2.4 Obtaining a dataset of pathogenic and benign variants for benchmarking”); variants that were submitted for the set of pathogenic variants but that were not consistently considered pathogenic by all three evaluators (n = 5, see “2.4 Obtaining a dataset of pathogenic and benign variants for benchmarking”); and a set of variants for which the ACMG criteria turned out to be unsuitable, resulting in a wide range of classifications back then (n = 6)] (6). To ensure that classification differences were linked to differences in the interpretation of the guidelines rather than differences in terms of access to data linked to the variant, all evaluators based their classification on the same papers. In total, 17 variants were evaluated by three geneticists independently.
To evaluate the inter-evaluator agreement, all pairwise combinations were checked. Classifications that might lead to medical management differences (i.e., P/LP versus B/LB/VUS) and disagreements less likely to affect clinical decision-making (P versus LP; B versus LB; VUS versus LB/B), were evaluated.
While these guidelines were initially tested on a set of feline variants, the goal was to develop a set of variant classification guidelines capable of classifying variants across a wide range of domestic species. Aside from the cat, a first step towards assessing the applicability of AVCG across species was taken for all eight other species (dog, cattle, pig, sheep, horse, chicken, rabbit, and goat) mentioned in OMIA (1). Practically, for every additional species, five variants were evaluated, if available, and the evaluator was asked to answer two questions: (1) whether there was an incompatibility of any of the criteria in that specific species in which that variant was evaluated and (2) whether they encountered difficulties not seen in the cat. To avoid any bias, no evaluator was allowed to check a variant published by his/her own group.
The scope was defined as the development of guidelines that are used by the community for Mendelian disorders in domestic animals to classify variants in categories based on standardized criteria. Somatic variants associated with cancer, variants (risk/protective) associated with complex traits, and structural variants (defined as a variant larger than 50 bp) (38) were considered outside the scope of these guidelines.
The development of the AVCG was conducted in three steps. After the preparatory phase, seven ACMG criteria were removed, while six were altered in phase two. In phase three, one additional criterion was altered. Overall, of the 28 initial criteria from the original ACMG guidelines published in 2015, half were removed or altered (Supplementary Table S2) (2). The final set of criteria can be found in Table 4, clarifications for several criteria can be found in Supplementary Data S3.
Table 4. The final set of criteria used to classify pathogenic and benign variants in domestic animals, based on the original American College of Medical Genetics and genomics guidelines (2).
An overview of the removed criteria is provided in Table 5. From the seven criteria that were removed, three were associated with AF (ACMG PM2, ACMG BA1, ACMG BS1). The rationale for removal of these three criteria was based on a combination of observations. First, the large variant databases used by human geneticists, seldom exist in other species, hence the data will often not be available. Secondly, animal geneticists must consider breed population structures and dynamics. Breeds are not always consistently defined across breed registries, and their populations experience the effect of (a combination of) breeding practices like population bottlenecks, the founder effect, popular sire effects, and inbreeding, which all influence AF. The human criteria have not been designed for those situations. Thirdly, while methods have been developed to calculate AF cutoffs, they rely on estimates of prevalence and penetrance, which can lead to debate and different results (44, 45). This causes undesired subjectivity in the criteria. While the first reason will become less important when more data is generated, the other two could currently not be resolved, hence the criteria were removed. Two criteria linked to the authority of a reputable source (e.g., investigators from an experienced laboratory make a statement about a variant without showing the data, ACMG PP5 and ACMG BP6) have also been removed, due to the absence of reviewable evidence supporting the claim. Information must be available to allow independent checks. One criterion (ACMG PM6) was removed as it became redundant due to the rewording of another criterion. The final one (ACMG BP1) was deemed too restrictive without sufficient evidence.
Table 5. Overview of the criteria present in the American College of Medical Genetics and Genomics (ACMG) guidelines that were removed to develop the animal variant classification guidelines.
Two new criteria were proposed in phase one, their concept was accepted in phase two and their text was optimized and assigned a supporting weight in phase three. These two criteria are essentially the pathogenic and benign version of an approach integrating conservation and clinical data from other species (Table 4, criteria PP1/BP1). As such, the newly developed guidelines (Table 4) contain 23 criteria, of which 14 are linked to pathogenicity and nine support benign classification, and these are combined to assign pathogenicity labels as detailed in Table 6. This also implies that there are 16 changes in AVCG versus the ACMG guidelines (i.e., 14 criteria removed or altered, two new criteria added).
As the cat is one of the top three domestic species for which disease-associated variants are published and several geneticists are dedicated feline experts, the cat was the species of choice for this analysis (1). The systematic review conducted to identify criteria used to search for candidate pathogenic variants in variant effect predictor benchmark studies (Supplementary Figure S1 for the PRISMA flow diagram), led to a set of 61 descriptions on how to obtain pathogenic variants (Supplementary Table S4). Summarizing the approaches led to three selection methods (Table 1), of which two were found suitable and were combined. To increase the stringency further, it was decided that independent literature reviews had to be conducted by at least three experienced (>10 years of experience) geneticists. Ultimately, 53 feline variants, encompassing a variety of mechanisms, inheritance patterns, and phene classes were considered pathogenic “without a doubt” by at least three geneticists and were used as a “truth set” (46, 47). The characteristics of this pathogenic dataset are summarized in Table 7 and a detailed description per variant is provided in Supplementary Table S5.
Importantly for classification purposes, but also part of our recommendations on how variants should be reported (Supplementary Data S4), population data could be collected for 44 of the 53 variants, with sample sizes ranging from 994 to 48,949 (median: 31,559 samples), tripling the sample size from the largest feline population study so far (33). The AFs retrieved for these 44 variants ranged from 0% (i.e., not found in the population, 30/44 variants) to >5% (the variant responsible for factor XI deficiency) (Supplementary Table S5) (48). Seven variants were reported for the first time in at least one new breed (Supplementary Table S1) (49–53). Of the remaining nine variants, AFs for six of them were found in the original publication, leading to a total of 50 out of 53 variants with AF data (median sample size: 125, range: 28—597 samples). The complete list of variants and their overall and breed-specific AFs are provided in Supplementary Tables S5 and S1, respectively.
For several categories of variants (i.e., missense, frameshift, and nonsense variants, but not for splice site variants), in silico tools aim to predict whether they are disease-causing or not. To allow benchmarking of the performance of those tools, the aforementioned systematic review was conducted to identify criteria used to select candidate-benign variants (also described as “neutral,” “nondamaging” or “tolerated” in literature) for benchmark studies (Supplementary Figure S1 for the PRISMA flow diagram) (54). A set of 61 descriptions was obtained describing how to obtain benign variants (Supplementary Table S4). These descriptions could be summarized in four selection methods (Table 1) but only one method was found suitable. The benign dataset can be found in Supplementary Table S3 and contains 21 missense variants, 13 nonsense variants and 11 frameshift variants.
The systematic review returned 78 publications, identifying altogether 128 variant effect predictor tools (Supplementary Table S6). From this initial list of 128 tools, 114 were excluded because they did not allow analysis of genetic variants of cats and/or did not have a working online tool, leading to 14 tools that were ultimately retained. Of these, nine can be used solely to evaluate missense variants (55–63), one for nonsense and missense variants (55), one for nonsense variants and frameshifts (64), and three for splice sites (65–67), respectively. An overview of their performance (i.e., accuracy, sensitivity and specificity) and technical summary are provided in Table 2.
For 42 missense variants, from the 10 remaining tools, six scored all 42 variants and the remaining four a subset. Most variants that were not scored were benign (25 times a benign variant versus six times a pathogenic variant). While the overall accuracy ranged between 79 and 90%, the best scores were for MutPred2 (90%), and List-S2 (88%). Two tools were available for analysis of 26 nonsense variants, PANTHER and MutpredLOF. MutpredLOF did not score one variant and PANTHER did not score 15 out of 26 variants. Again, more benign variants were not scored (12 times a benign variant versus four times a pathogenic variant). The overall accuracy ranged between 48 and 55%. For the 22 frameshift variants, only MutpredLOF was available. This tool scored all except one benign variant and the accuracy was 52%. For the eight splice site variants, three tools were available, with accuracies ranging between 63 and 100%.
Practically, if the results of two in silico tools are consistent, PP3 or BP4 are fulfilled. Otherwise, the result is not considered. An overview of the combinations and their accuracy can be found in Supplementary Table S7. For missense variants, of 15 possible combinations, the combination that scored best was the combination of MutPred2 and List-S2 (34 out of 35 correctly classified). For nonsense and frameshift variants, this comparison was impossible as no tools scored all variants or only one tool was available, respectively. For splice site variants, the best combination was SSPnn and Spliceator.
Based on the data provided by the original publications, complemented with the in silico data from 3.3 and, for AVCG, the data from criterion PP1/BP1, the 53 pathogenic variants were all classified with the ACMG criteria and AVCG. Overall, with the ACMG guidelines, 38 were classified as P (72%), six as LP (11%), four as VUS (8%), and five as B (9%), which implies that 83% of the variants were correctly classified. Overall, AVCG classified 42 variants as P (79%), seven as LP (13%), and the remaining four as VUS (8%); 92% of the variants were thus correctly classified, i.e., misclassification halved. The final classification for every variant is denoted in Supplementary Table S5.
An in-depth evaluation was performed to assess why six variants were classified differently (Table 8) (48–50, 52, 68, 69). For each of these, ACMG classified at least one category more in the direction of B. Five of these classification differences might affect clinical decision making and screening/breeding programs. An assessment of the differences demonstrated that this was linked to the removed AF criteria (ACMG BA1, five times negative effect), the newly added PP1 (two times positive effect), and the altered PM2 and PS5 (one and three times positive effect, respectively). In more detail, PKD1:c.9882C > A, TRPV4:c.1024G > T, UROS:c.140C > T, CEP290:g.112522818C > A and F11:g.17176154G > A all had an AF >0.05 in at least one breed, which is heavily penalized by ACMG (Supplementary Table S1). In addition, TRPV4:c.1024G > T and UROS:c.140C > T are conserved and a ClinVar entry at that position indicates that the variant is pathogenic, i.e., PP1 is fulfilled. This criterion has no counterpart in ACMG and supports a pathogenic classification with AVCG. Furthermore, a similar missense variant as UROS:c.140C > T has deemed to be pathogenic in other species, with the other species being the driver for the different result. In AVCG, this is sufficient to invoke PM2, while this is not taken into account in ACMG. Finally, while the wording of the criterion is the same, the weight has increased from supporting to strong for PS5. Hence, more weight is given to classify PKD1:c.9882C > A, DMD:g.27988938G > A and F11:g.17176154G > A as pathogenic.
Table 8. Variants classified differently with the American College of Medical Genetics and Genomics (ACMG) guidelines and the Animal Variant Classification Guidelines (AVCG).
An evaluation of the incorrectly classified variants (i.e., that did not get the label LP/ P) led to a total of nine variants that need to be scrutinized (Tables 8, 9). From these nine variants, five are uniquely misclassified with the ACMG guidelines (Table 8), while the other four were consistently misclassified as VUS (Table 9) (48–50, 52, 68, 70–73). All five variants that were uniquely misclassified by ACMG were all directly caused by the AF criterion ACMG BA1 (Table 5 and Supplementary Table S2) which is removed in AVCG.
Table 9. Variants consistently misclassified with the American College of Medical Genetics and Genomics (ACMG) guidelines and the animal variant classification guidelines (AVCG).
An analysis of the four VUS misclassified by ACMG and AVCG indicates that all four are missense variants with some criteria supporting pathogenic classification, however, not enough to be considered LP (Table 9). Another similarity is that they were all present in one unique case, i.e., there were no additional affected individuals or family members. As 15 other variants with n = 1 were correctly classified (Supplementary Table S5), a detailed analysis was conducted to identify what sets them apart. What differs for the majority is their effect at the protein level: in 10/15, the variant is of a type invoking PVS1, a very strong criterion that requires limited additional criteria to result in an LP/P classification. The five remaining missense variants with n = 1 were correctly classified (i.e., all as LP). For these five, this LP classification was due to an additional strong criterion [i.e., functional studies (PS3, n = 2), an identical or other amino-acid change as a previously established pathogenic change (PS1, n = 1; PM2, n = 1, respectively)] or a combination of a sufficient number of moderate and supporting criteria being fulfilled.
The overall inter-evaluator agreement was based on a novel dataset comprising three subgroups of variants (Supplementary Table S8). As each of these 17 variants were assessed with the AVCG by three individual evaluators, there were 51 classifications assigned.
Overall, 39 of 51 classifications (76%) matched. While there were a total of 12 of 51 disagreements on classification (24%), for only 2/51 (4%), this potentially might influence clinical decision-making (i.e., these were differences between P and VUS). The remaining differences were linked to the strength of pathogenicity [8/51 (16%) P vs. LP] or VUS versus benign classification (2/51, 4%).
Practically, for 11 variants, the classifications were thus three times consistent, while for six variants the classification of each time one person did not match that of the other two (Supplementary Table S8). There were no variants that received three different labels. Among the aforementioned six variants, eight criteria were not consistently used (i.e., PS1, PS3, PS4, PS5, PM1, PM4, PP4 and BS2). Half of these criteria (PS1, PS5, PM1 and BS2) were used differently in only one variant, the other half (PS3, PS4, PM4 and PP4) were used differently for two variants. For the variant in which the classification might affect clinical decision-making (i.e., CYP27B1:g.86180281del, VUS vs. VUS vs. P), the difference was linked to a different evaluation of criterion PS3, i.e., whether there was sufficient in vitro or in vivo functional data to support a damaging effect.
Among the three groups of variants included in this reproducibility evaluation, clear differences in agreement were noticed (Supplementary Table S8). For the variants that passed the inclusion criteria for the pathogenic reference data set, the agreement was strongest [16/18 (89%)] and there were no misclassifications that might potentially lead to differences in medical management/ breeding/screening programs because they ranged between P and LP. Among the variants that were included because ACMG classification was difficult, the agreement was in the same range [14/18 (78%)] with also no misclassifications affecting clinical management. Among the variants that were included because there was a disagreement among evaluators whether they should be included in the pathogenic dataset, the agreement was lower [9/15 (60%)] and 2/15 differences (13%) might lead to altered clinical approaches as they ranged between VUS and P. For these five variants, this is still an increase in agreement compared to the 5/15 (33%) agreement based on subjective decision-making on pathogenicity prior to the development of the guidelines.
Five variants per species, when available, were classified and the evaluators were asked to note (1) any incompatibilities, and/or (2) difficulties not seen in the cat. For both questions, the answer was “no” for every single variant across eight additional species (i.e., dog, horse, cattle, pig, goat, sheep, rabbit, and chicken).
As there were no issues encountered, several of the remaining variants submitted for the feline pathogenic variant dataset that did not pass all criteria, were additionally evaluated, resulting in 72 feline variants or 53% (= 72/136) of the disease-associated variants currently known in cats, classified. Population data was collected for five of these additional variants, but all were observed to be homozygous wildtype. Altogether, we have now provided a classification for over 110 variants (Supplementary Table S9).
Breeding strategies, especially in companion animals, significantly impact the health of their offspring and the overall population. Selective breeding that emphasizes specific traits too narrowly can result in reduced genetic diversity, ultimately potentially increasing the population’s susceptibility to diseases. Furthermore, prioritizing phenotypic traits over health can adversely affect animal welfare. Responsible breeding practices that emphasize health, genetic diversity, and animal welfare can lead to healthier and more resilient animal populations (74). Especially in inbred populations with a small effective population size, the use of validated genetic testing is essential. Therefore, we have developed and tested the AVCG, aiming not only to provide guidance to the practitioner who sees the individual animal in a clinical setting but also to assist in improving the health of the population, by objectifying when there is sufficient evidence for the pathogenicity of a variant. This is the first step in determining whether a variant can be used for screening and in breeding programs. This development process is not finished with the publication of the current set of guidelines.
Common to both ACMG and AVCG and considered good practice, guidelines are subject to change. Since the publication of the original ACMG guidelines, several of the criteria have been modified, clarified, or are considered for removal, and a thorough analysis demonstrates a considerable overlap with the decisions made here for AVCG. From the seven criteria that were removed in the process to develop the AVCG, the two linked to expert opinion (i.e., ACMG PP5 and ACMG BP6, see Table 5 and Supplementary Table S2) have also been recommended for removal from ACMG, with a rationale similar to ours: actual data should be preferred over opinion (19). For three others linked to AF (ACMG PM2, ACMG BS1, and ACMG BA1, see Table 5 and Supplementary Table S2), parallel observations have been made, but different actions have been undertaken, probably driven by the difference in occurrence. In more detail, ACMG has recognized that, for some variants, the stand-alone criterion for an AF of 5% is too strict, which has been tackled by a (very short) exception list, and it has been suggested to reduce the moderate weight of the allelic frequency criterion supporting pathogenic classification (20, 21). Here, 9% (5/53) of the feline variants in the pathogenic list have AFs >5% overall or in subpopulations (with subpopulation being a breed or a variety within a breed, depending on the registry, see Supplementary Table S1 for examples) and even higher percentages were found in other population studies in cats (17%, 9/52) (33) and dogs (18%, 46/250) (75), indicating an exception list is not feasible for species or populations in which genetic diversity is reduced. For AVCG, we opted to omit the three criteria linked to AF, as explained above, and this led to a marked improved classification. Apart from questions about the appropriateness of AF cut-offs for animals, the key to use these criteria is also the availability of (public) databases for a wide range of species. While this is an ongoing effort for several species, data is often lacking. As small databases can lead to biased estimates and the absence of a database makes it difficult to assess the impact of certain breeding strategies as the AF is unknown, we encourage community efforts to build new and expand the currently available databases. The dataset presented here helps by providing breed-specific and overall AFs for a large set of variants (Supplementary Table S1 and S5, respectively).
Clarifications were also deemed necessary for the criteria linked to de novo variants (ACMG PS2 and ACMG PM6) (22). These were also considered unclear here and led to a modification of criterion PS2 and removal of criterion PM6 (see Table 5 and Supplementary Table S2). As such, for six out of seven criteria that were removed, at least a modification was also deemed necessary after the original ACMG guidelines were published. A change implemented by ACMG, but not adopted for AVCG, was the update on PVS1 (23). The rationale for a more extensive explanation for this criterion for ACMG was that it is the only criterion with a very strong weight, hence it can influence the classification of a variant easily. The proposed change is an extensive flow chart, which is complex and should be re-evaluated as the AVCG evolve. Published during this study, the updated ACMG recommendations linked to all criteria associated with splice variants (PVS1, PS1, PP3, BP4, BP6) could not be included in the evaluation process (24). Nevertheless, except for small additions to PVS1 (explicitly stating that this criterion can also be used across species) and PP3/BP4 (explicitly stating the number of tools that should be used for in silico criteria), the group considered the criteria linked to splicing sufficiently clear. Similarly, criteria PS3/BS3, PP4/BS4, the criterion formally known as PP1 but renamed to PS5 here as the weight was increased, and PM3 were also considered clear, while additional clarifications were published for ACMG (25–27).
The final recommendation dealt with PP3 and BP4, i.e., the performance of in silico tools (28). Remarkably, while there are several benchmark studies, there are none for any of the species evaluated here. While we wanted to provide guidance, this posed significant challenges as there are, to our knowledge, no reference datasets for any of the domestic animals evaluated here. To identify the most optimal strategy to develop such a dataset, systematic literature reviews were conducted. Surprisingly, the number of different strategies was limited, and especially for the benign variants, only one method remained. As the most common methodology was AF-based, with all its limitations in species with limited genetic diversity, we opted for a different strategy (6, 29). This strategy has the additional benefit that it does not rely on any of the criteria used to classify variants later on, which is positive as this would have created some sort of circularity in reasoning. While this is important for the development of the AVCG, it is also a common issue when in silico tools are tested. Circularity occurs when there is an overlap between training and test datasets, which is common in humans, and can lead to biased performance estimates (54, 76). As none of the tools used feline genetic variants during the development process, circularity is no issue here.
Overall, we observed large performance differences between and within categories of variant types (Table 2, Supplementary Table S7). Similarly, benchmarks in literature indicate even gene- and phenotype-specific difference (41–43). While individual tools might outperform one another in specific cases, we aimed to provide an overview of the combination of tools that generally demonstrated the best accuracy, which turned out to be the combination of MutPred2 and LIST-S2 for missense variants (56, 62). The tools in this combination differ in underlying methodology and how they were trained, which is an advantage as this also implies less dependency on the same information, that is, a more independent analysis and conclusion. While a satisfactory performance was achieved for missense variants and splice sites, this was not the case for frameshift and nonsense variants. As information from variant effect predictor tools can be readily generated, we, in general, encourage the use of these kind of tools if performance is adequate, which is thus limited to missense variants and splice sites based on the current benchmark.
Combined, classification of animal variants with AVCG outperformed classification with ACMG. From a breeding and/or veterinary practice perspective, one example illustrating the potential consequences of a different category, is linked to polycystic kidney disease, associated with the PKD1:c.9882C > A variant (52). It is a widespread variant, standardly incorporated in screening and these screening programs have successfully reduced the prevalence of the disease (33). In contrast, based on the ACMG classification, this variant should be disregarded when breeding decisions, screening and clinical decisions are made, which implies a standstill for a disease that was originally found in 40% of the Persian cats (77).
Aside from a good classification, when implemented in practice, guidelines ideally also have an additional characteristic, i.e., a high reproducibility or concordance between evaluators. If this is not the case, results tend to change between laboratories or even between evaluators within a laboratory. While interpretation differences have been reported several times, variant classification reproducibility has been demonstrated to be rather low with and without the ACMG guidelines (34% for both) (3–5). A cautionary note on this result is that a disagreement of 66% does not necessarily imply also that clinical management will be affected in two out of three cases. Looking at differences that might affect clinical management (L/LP versus VUS/LB/B), the number reduced to 22%. The difference with AVCG is remarkable: an exact agreement of 76% and only 4% of the differences might have practical consequences. While an obvious explanation might be that only variants that were easy to classify were included, this was not the case. In fact, even when the comparison solely includes the group of variants among which there was debate, the agreement was still twice as high with the AVCG (ACMG: 34% versus AVCG: 60%).
With this study, we not only provide methods to develop datasets for benchmarking, but we also classify and provide AFs, and in silico data for over half of the currently known feline pathogenic variants. Overall, nearly all variants classified here got an LP or P label, and for several of the ones that did not (e.g., three hypertrophic cardiomyopathy-associated variants), this was anticipated (6, 29, 78, 79). The high number of LPs/Ps was expected as the study was based on OMIA, a database that focuses on disease-associated variants. The range of phenotypes, inheritance patterns, penetrance, and species in which the AVCG were successfully applied, indicates that the central scope, i.e., providing guidelines that can be used in general for Mendelian disorders, seems to be fulfilled. However, the classification of these variants is not written in stone. Similarly to the guidelines themselves and the results obtained with the in silico tools, the classification of a variant can change when new information becomes available (33). One example is the UROS:c.140C > T variant. In the original publication that was used to classify the variant here, one cat was homozygous for two UROS variants (UROS:c.140C > T and UROS:c.331G > T) (68). While functional studies indicated a potential effect for both variants (with a more pronounced effect for the latter), in that study the effect of the two could not be separated (68). Recently, several cats without symptoms but homozygous for the UROS:c.140C > T variant were identified, indicating variant disassociation with disease (33). This finding suggests the need to re-evaluate the pathogenicity of this variant (33). It also substantiates the importance of follow-up and identifying additional cases, whenever possible.
This study raises interesting questions for future research. First, for both frameshift and nonsense variants, the accuracy of in silico tools was lower than for the splice site and missense variants. This has the consequence that for two large groups of variants, the in silico criteria (PP3/BP4) can currently not be used. While these criteria have only moderate support, nonsense and frameshift variants benefit from the strong PVS1 criterion, which reduces the additional evidence needed to end up with a classification as P/LP. However, an in silico evaluation is easily generated once good tools are available. Hence, we encourage the development of new tools but also to not limit them to model species as humans and the mouse, as has happened for several tools in the past. Second, during the analysis process of the variants in the pathogenic dataset, a positive finding was that in vitro and/or in vivo validation had already been performed for several variants. While it was outside the scope for the current study to do this for all the remaining variants, functional studies provide significantly more evidence. This is also reflected by the strong weight given to these criteria, and we strongly encourage these steps. Third, the effect of the person evaluating variants, is important. To develop a pathogenic dataset, three geneticists checked each variant independently, which is more than what is routinely mentioned in literature (Table 1, Supplementary Table S4). While this already implies quite a workload, it can always be argued that increasing the number of evaluators, might have led to an even better (i.e., more robust) pathogenic dataset. In taking these kind of decisions, it is of course important to balance feasibility and performance. Also important is tracking inconsistencies. In more detail, during the evaluation of variants that did not receive a consistent classification by multiple evaluators with AVCG, certain criteria were identified that were interpreted differently and for some, this occurred more than once. Future studies should keep track of these kind of disagreements and take action to improve the criteria, where needed. Fourth, while the across-species results of AVCG are preliminary evidence for a use in other species, further research should follow these initial steps as the demand for objective guidelines to, e.g., provide a first proof of evidence on when to include a variant for screening, is widespread (80). Fifth, as some disease-causing variants were classified as VUS with AVCG, further optimization and tailoring, especially for the n = 1 situation, should be a future focus. Furthermore, while a large set of variants was thoroughly checked, the aim should be to try to classify all variants currently published. This will undoubtedly identify additional areas of improvement and potential classification difficulties. Importantly, while striving for perfect accuracy in terms of classification, we also want to stress that there will likely always be exceptions that do not follow the rules. Finally, we agree with the view of ACMG and that is why these guidelines focus specifically on Mendelian diseases and why variants associated with complex diseases, somatic variants, and structural variants larger than 50 bp, were excluded (2). While the excluded variants currently represent a minority of the total number of disease-associated variants, more are likely to be discovered in the future, requiring solutions for classification for them as well. As such, we also support the foundation of expert groups, an initiative which is currently underway under the umbrella of the International Society for Animal Genetics, to take on the challenge of (1) further improving guidelines, (2) keeping track of new data and, whenever necessary, updating variant classification, and (3) developing guidelines for the variants that were outside the current scope. Furthermore, steps are undertaken to make these variant classifications publicly available in databases like OMIA.
In short, we provide the AVCG, tailored for variant classification in domestic animals, and demonstrate a substantially improved classification, as well as reproducibility, even when used on animal variants the ACMG guidelines struggle with or on animal variants which led to individual conflicting assessments.
The datasets presented in this study can be found in the Supplementary material.
Ethical approval was not required for the studies involving animals in accordance with the local legislation and institutional requirements because samples were non-invasive buccal swabs, collected by the owner or in veterinary practices, or whole blood on EDTA, collected by veterinary clinics, in accordance with international standards for animal care and research. In some cats, blood was obtained as part of routine clinical procedures for diagnostic purposes, at the request and with the consent of the owner. As these samples were from client-owned cats for which no harmful invasive procedures were performed, there was no animal experimentation according to the legal definition in Europe (Subject 5f of Article1, Chapter I of the Directive 2010/63/UE of the European Parliament and of the Council). All cat owners provided consent for the use of their cat’s DNA sample in scientific research. Written informed consent was obtained from the owners for the participation of their animals in this study.
FB: Data curation, Formal analysis, Investigation, Writing – review & editing. MA: Data curation, Formal analysis, Investigation, Writing – review & editing. HA: Data curation, Formal analysis, Investigation, Resources, Writing – review & editing. IC: Data curation, Formal analysis, Investigation, Writing – review & editing. CD: Data curation, Formal analysis, Investigation, Resources, Writing – review & editing. JHa: Data curation, Formal analysis, Investigation, Software, Writing – review & editing. JHä: Formal analysis, Investigation, Writing – review & editing. MK: Investigation, Writing – review & editing. EL: Investigation, Writing – review & editing. IL: Data curation, Formal analysis, Investigation, Writing – review & editing. ML: Data curation, Formal analysis, Investigation, Writing – review & editing. LL: Data curation, Formal analysis, Investigation, Writing – review & editing. ÅO: Data curation, Formal analysis, Investigation, Writing – review & editing. LP: Investigation, Writing – review & editing. PS: Data curation, Formal analysis, Investigation, Writing – review & editing. TV: Investigation, Writing – review & editing. FS: Data curation, Formal analysis, Investigation, Writing – original draft, Writing – review & editing. BB: Conceptualization, Data curation, Formal analysis, Funding acquisition, Investigation, Methodology, Project administration, Resources, Software, Supervision, Validation, Writing – original draft, Writing – review & editing.
The author(s) declare that financial support was received for the research, authorship, and/or publication of this article. This study was partially funded by the Bijzonder Onderzoeksfonds (BOF) starting Grant (01N04119).
HA is an employee of Wisdom Panel Mars Petcare Science & Diagnostics, a company that offers canine and feline DNA testing as a commercial service. CDdC is an employee of Antagene, a DNA testing and genetic analysis company for dogs, cats, horses, and wildlife. The following authors interact (= discuss genetic tests, variants that have or will be published, …) on a regular basis with commercial companies: BJGB (Van Haeringen laboratorium, Laboklin, Wisdom Panel Mars Petcare Science & Diagnostics, Antagene), MA (Antagene, Wisdom Panel Mars Petcare Science & Diagnostics, Felome, Genindexe), JJH (Embark), ML (Vetogene, Genefast), however, there are no fees involved. None of the authors have received speaking fees from commercial genetic testing companies. None of the authors have performed paid consultant services for commercial genetic testing companies.
All claims expressed in this article are solely those of the authors and do not necessarily represent those of their affiliated organizations, or those of the publisher, the editors and the reviewers. Any product that may be evaluated in this article, or claim that may be made by its manufacturer, is not guaranteed or endorsed by the publisher.
The Supplementary material for this article can be found online at: https://www.frontiersin.org/articles/10.3389/fvets.2024.1497817/full#supplementary-material
1. Nicholas, FW . Online Mendelian inheritance in animals (OMIA): a comparative knowledgebase of genetic disorders and other familial traits in non-laboratory animals. Nucleic Acids Res. (2003) 31:275–7. doi: 10.1093/nar/gkg074
2. Richards, S, Aziz, N, Bale, S, Bick, D, Das, S, Gastier-Foster, J, et al. Standards and guidelines for the interpretation of sequence variants: a joint consensus recommendation of the American College of Medical Genetics and Genomics and the Association for Molecular Pathology. Genet Med. (2015) 17:405–24. doi: 10.1038/gim.2015.30
3. Amendola, LM, Jarvik, GP, Leo, MC, McLaughlin, HM, Akkari, Y, Amaral, MD, et al. Performance of ACMG-AMP variant-interpretation guidelines among nine Laboratories in the Clinical Sequencing Exploratory Research Consortium. Am J Hum Genet. (2016) 98:1067–76. doi: 10.1016/j.ajhg.2016.03.024
4. Rehm, HL, Berg, JS, Brooks, LD, Bustamante, CD, Evans, JP, Landrum, MJ, et al. ClinGen-the clinical genome resource. N Engl J Med. (2015) 372:2235–42. doi: 10.1056/NEJMsr1406261
5. Yorczyk, A, Robinson, LS, and Ross, TS. Use of panel tests in place of single gene tests in the cancer genetics clinic. Clin Genet. (2015) 88:278–82. doi: 10.1111/cge.12488
6. Boeykens, F, Abitbol, M, Anderson, H, Dargar, T, Ferrari, P, Fox, PR, et al. Classification of feline hypertrophic cardiomyopathy-associated gene variants according to the American College of Medical Genetics and Genomics guidelines. Front Vet Sci. (2024) 11:1327081. doi: 10.3389/fvets.2024.1327081
7. Shaffer, LG, Sundin, K, Geretschlaeger, A, Segert, J, Swinburne, JE, Royal, R, et al. Standards and guidelines for canine clinical genetic testing laboratories. Hum Genet. (2019) 138:493–9. doi: 10.1007/s00439-018-1954-4
8. Shaffer, LG, Geretschlaeger, A, Ramirez, CJ, Ballif, BC, and Carl, C. Quality assurance checklist and additional considerations for canine clinical genetic testing laboratories: a follow-up to the published standards and guidelines. Hum Genet. (2019) 138:501–8. doi: 10.1007/s00439-019-02013-9
9. Broeckx, BJG . The dog 2.0: lessons learned from the past. Theriogenology. (2020) 150:20–6. doi: 10.1016/j.theriogenology.2020.01.043
10. Moses, L, Niemi, S, and Karlsson, E. Pet genomics medicine runs wild. Nature. (2018) 559:470–2. doi: 10.1038/d41586-018-05771-0
11. Lyons, LA, and Buckley, RM. Direct-to-consumer genetic testing for domestic cats. Vet Clin North Am Small Anim Pract. (2020) 50:991–1000. doi: 10.1016/j.cvsm.2020.05.004
12. Leroy, G . Genetic diversity, inbreeding and breeding practices in dogs: results from pedigree analyses. Vet J. (2011) 189:177–82. doi: 10.1016/j.tvjl.2011.06.016
13. Wijnrocx, K, Franc, L, Stinckens, A, Janssens, S, and Buys, N. Half of 23 Belgian dog breeds has a compromised genetic diversity, as revealed by genealogical and molecular data analysis. J Anim Breed Genet. (2016) 133:375–83. doi: 10.1111/jbg.12203
14. Leroy, G, and Rognon, X. Assessing the impact of breeding strategies on inherited disorders and genetic diversity in dogs. Vet J. (2012) 194:343–8. doi: 10.1016/j.tvjl.2012.06.025
15. Calboli, FCF, Sampson, J, Fretwell, N, and Balding, DJ. Population structure and inbreeding from pedigree analysis of purebred dogs. Genetics. (2008) 179:593–601. doi: 10.1534/genetics.107.084954
16. Leroy, G, Verrier, E, Meriaux, JC, and Rognon, X. Genetic diversity of dog breeds: within-breed diversity comparing genealogical and molecular data. Anim Genet. (2009) 40:323–32. doi: 10.1111/j.1365-2052.2008.01842.x
17. Leroy, G, Vernet, E, Pautet, MB, and Rognon, X. An insight into population structure and gene flow within pure-bred cats. J Anim Breed Genet. (2014) 131:53–60. doi: 10.1111/jbg.12043
18. van Steenbeek, FG, Hytönen, MK, Leegwater, PAJ, and Lohi, H. The canine era: the rise of a biomedical model. Anim Genet. (2016) 47:519–27. doi: 10.1111/age.12460
19. Biesecker, LG, and Harrison, SM. The ACMG/AMP reputable source criteria for the interpretation of sequence variants. Genet Med. (2018) 20:1687–8. doi: 10.1038/gim.2018.42
20. Ghosh, R, Harrison, SM, Rehm, HL, Plon, SE, and Biesecker, LG. Updated recommendation for the benign stand-alone ACMG/AMP criterion. Hum Mutat. (2018) 39:1525–30. doi: 10.1002/humu.23642
21. ClinGen Sequence Variant Interpretation Working Group (2020). ClinGen sequence variant interpretation recommendation for PM2 - version 1.0. Available at: https://www.clinicalgenome.org/docs/pm2-recommendation-for-absence-rarity/ (Accessed May 16, 2024).
22. ClinGen Sequence Variant Interpretation Working Group . (2021). ClinGen sequence variant interpretation recommendation for de novo criteria (PS2/PM6) - version 1.1. Available at: https://clinicalgenome.org/docs/ps2-pm6-recommendation-for-de-novo-ps2-and-pm6-acmg-amp-criteria-version-10/ (Accessed May 16, 2024).
23. Abou Tayoun, AN, Pesaran, T, DiStefano, MT, Oza, A, Rehm, HL, Biesecker, LG, et al. Recommendations for interpreting the loss of function PVS1 ACMG/AMP variant criterion. Hum Mutat. (2018) 39:1517–24. doi: 10.1002/humu.23626
24. Walker, LC, De La, HM, Wiggins, GAR, Lindy, A, Vincent, LM, Parsons, MT, et al. Using the ACMG/AMP framework to capture evidence related to predicted and observed impact on splicing: recommendations from the ClinGen SVI splicing subgroup. Am J Hum Genet. (2023) 110:1046–67. doi: 10.1016/j.ajhg.2023.06.002
25. Brnich, SE, Abou Tayoun, AN, Couch, FJ, Cutting, GR, Greenblatt, MS, Heinen, CD, et al. Recommendations for application of the functional evidence PS3/BS3 criterion using the ACMG/AMP sequence variant interpretation framework. Genome Med. (2019) 12:3. doi: 10.1186/s13073-019-0690-2
26. Biesecker, LG, Byrne, AB, Harrison, SM, Pesaran, T, Schäffer, AA, Shirts, BH, et al. ClinGen guidance for use of the PP1/BS4 co-segregation and PP4 phenotype specificity criteria for sequence variant pathogenicity classification. Am J Hum Genet. (2024) 111:24–38. doi: 10.1016/j.ajhg.2023.11.009
27. ClinGen Sequence Variant Interpretation Working Group (2019). ClinGen sequence variant interpretation recommendation for in trans criterion (PM3) - version 1.0. Available at: https://www.clinicalgenome.org/docs/pm3-recommendation-for-in-trans-criterion-pm3-version-10/ (Accessed May 16, 2024).
28. Pejaver, V, Byrne, AB, Feng, BJ, Pagel, KA, Mooney, SD, Karchin, R, et al. Calibration of computational tools for missense variant pathogenicity classification and ClinGen recommendations for PP3/BP4 criteria. Am J Hum Genet. (2022) 109:2163–77. doi: 10.1016/j.ajhg.2022.10.013
29. Schipper, T, Ohlsson, Å, Longeri, M, Hayward, JJ, Mouttham, L, Ferrari, P, et al. The TNNT2:c.95-108G>a variant is common in Maine coons and shows no association with hypertrophic cardiomyopathy. Anim Genet. (2022) 53:526–9. doi: 10.1111/age.13223
30. Van Poucke, M, Stee, K, Sonck, L, Stock, E, Bosseler, L, Van Dorpe, J, et al. Truncating SLC12A6 variants cause different clinical phenotypes in humans and dogs. Eur J Hum Genet. (2019):27. doi: 10.1038/s41431-019-0432-3
31. Schipper, T, Van Poucke, M, Sonck, L, Smets, P, Ducatelle, R, Broeckx, BJG, et al. A feline orthologue of the human MYH7 c.5647G>a (p.(Glu1883Lys)) variant causes hypertrophic cardiomyopathy in a domestic shorthair cat. Eur J Hum Genet. (2019) 27:1724–30. doi: 10.1038/s41431-019-0431-4
32. Van Poucke, M, Stee, K, Vanhaesebrouck, A, Bosseler, L, Peelman, LJ, and Van Ham, L. The novel homozygous KCNJ10 c.986T>C (p.(Leu329Pro)) variant is pathogenic for the SeSAME/EAST homologue in Malinois dogs. Eur J Hum Genet. (2017) 25:222–6. doi: 10.1038/ejhg.2016.157
33. Anderson, H, Davison, S, Lytle, KM, Honkanen, L, Freyer, J, Mathlin, J, et al. Genetic epidemiology of blood type, disease and trait variants, and genome-wide genetic diversity in over 11,000 domestic cats. PLoS Genet. (2022) 18:e1009804. doi: 10.1371/journal.pgen.1009804
34. Moher, D, Schulz, KF, Simera, I, and Altman, DG. Guidance for developers of health research reporting guidelines. PLoS Med. (2010) 7:e1000217. doi: 10.1371/journal.pmed.1000217
35. Shekelle, PG, Woolf, SH, Eccles, M, Grimshaw, J, and Los, AW. Clinical guidelines Developing guidelines. BMJ. (1999) 318:593–6. doi: 10.1136/bmj.318.7183.593
36. McAllister, M, Payne, K, Nicholls, S, MacLeod, R, Donnai, D, and Davies, LM. Improving service evaluation in clinical genetics: identifying effects of genetic diseases on individuals and families. J Genet Couns. (2007) 16:71–83. doi: 10.1007/s10897-006-9046-3
37. Page, MJ, McKenzie, JE, Bossuyt, PM, Boutron, I, Hoffmann, TC, Mulrow, CD, et al. The PRISMA 2020 statement: an updated guideline for reporting systematic reviews. BMJ. (2021) 372:n71. doi: 10.1136/bmj.n71
38. Ho, SS, Urban, AE, and Mills, RE. Structural variation in the sequencing era. Nat Rev Genet. (2020) 21:171–89. doi: 10.1038/s41576-019-0180-9
39. Kinsella, RJ, Kähäri, A, Haider, S, Zamora, J, Proctor, G, Spudich, G, et al. Ensembl BioMarts: a hub for data retrieval across taxonomic space. Database. (2011) 2011:bar030. doi: 10.1093/database/bar030
40. Pir, MS, Bilgin, HI, Sayici, A, Coşkun, F, Torun, FM, Zhao, P, et al. ConVarT: a search engine for matching human genetic variants with variants from non-human species. Nucleic Acids Res. (2022) 50:D1172–8. doi: 10.1093/nar/gkab939
41. Prado, MJ, Ligabue-Braun, R, Zaha, A, Rossetti, MLR, and Pandey, AV. Variant predictions in congenital adrenal hyperplasia caused by mutations in CYP21A2. Front Pharmacol. (2022) 13:931089. doi: 10.3389/fphar.2022.931089
42. Tarnovskaya, SI, Kostareva, AA, and Zhorov, BS. In silico analysis of TRPM4 variants of unknown clinical significance. PLoS One. (2023) 18:e0295974. doi: 10.1371/journal.pone.0295974
43. Leong, IUS, Stuckey, A, Lai, D, Skinner, JR, and Love, DR. Assessment of the predictive accuracy of five in silico prediction tools, alone or in combination, and two metaservers to classify long QT syndrome gene mutations. BMC Med Genet. (2015) 16:34. doi: 10.1186/s12881-015-0176-z
44. Whiffin, N, Minikel, E, Walsh, R, O’Donnell-Luria, AH, Karczewski, K, Ing, AY, et al. Using high-resolution variant frequencies to empower clinical genome interpretation. Genet Med. (2017) 19:1151–8. doi: 10.1038/gim.2017.26
45. Broeckx, BJG, Peelman, L, Saunders, JH, Deforce, D, and Clement, L. Using variant databases for variant prioritization and to detect erroneous genotype-phenotype associations. BMC Bioinform. (2017) 18:535. doi: 10.1186/s12859-017-1951-y
46. Ong, E, Xiang, Z, Zhao, B, Liu, Y, Lin, Y, Zheng, J, et al. Ontobee: a linked ontology data server to support ontology term dereferencing, linkage, query and integration. Nucleic Acids Res. (2017) 45:D347–52. doi: 10.1093/nar/gkw918
47. Smith, CL, and Eppig, JT. The mammalian phenotype ontology: enabling robust annotation and comparative analysis. Wiley Interdiscip Rev Syst Biol Med. (2009) 1:390–9. doi: 10.1002/wsbm.044
48. Kuder, H, Dickeson, SK, Brooks, MB, Kehl, A, Müller, E, Gailani, D, et al. A common missense variant causing factor XI deficiency and increased bleeding tendency in Maine coon cats. Genes (Basel). (2022) 13:792. doi: 10.3390/genes13050792
49. Menotti-Raymond, M, Deckman, KH, David, V, Myrkalo, J, O’Brien, SJ, and Narfström, K. Mutation discovered in a feline model of human congenital retinal blinding disease. Invest Ophthalmol Vis Sci. (2010) 51:2852–9. doi: 10.1167/iovs.09-4261
50. Gandolfi, B, Alamri, S, Darby, WG, Adhikari, B, Lattimer, JC, Malik, R, et al. A dominant TRPV4 variant underlies osteochondrodysplasia in Scottish fold cats. Osteoarthr Cartil. (2016) 24:1441–50. doi: 10.1016/j.joca.2016.03.019
51. Cogné, B, Latypova, X, Senaratne, LDS, Martin, L, Koboldt, DC, Kellaris, G, et al. Mutations in the Kinesin-2 motor KIF3B cause an autosomal-dominant ciliopathy. Am J Hum Genet. (2020) 106:893–904. doi: 10.1016/j.ajhg.2020.04.005
52. Lyons, LA, Biller, DS, Erdman, CA, Lipinski, MJ, Young, AE, Roe, BA, et al. Feline polycystic kidney disease mutation identified in PKD1. J Am Soc Nephrol. (2004) 15:2548–55. doi: 10.1097/01.ASN.0000141776.38527.BB
53. Abitbol, M, Hitte, C, Bossé, P, Blanchard-Gutton, N, Thomas, A, Martignat, L, et al. A COLQ missense mutation in sphynx and Devon rex cats with congenital myasthenic syndrome. PLoS One. (2015) 10:e0137019. doi: 10.1371/journal.pone.0137019
54. Grimm, DG, Azencott, CA, Aicheler, F, Gieraths, U, Macarthur, DG, Samocha, KE, et al. The evaluation of tools used to predict the impact of missense variants is hindered by two types of circularity. Hum Mutat. (2015) 36:513–23. doi: 10.1002/humu.22768
55. Tang, H, and Thomas, PD. PANTHER-PSEP: predicting disease-causing genetic variants using position-specific evolutionary preservation. Bioinformatics. (2016) 32:2230–2. doi: 10.1093/bioinformatics/btw222
56. Malhis, N, Jacobson, M, Jones, SJM, and Gsponer, J. LIST-S2: taxonomy based sorting of deleterious missense mutations across species. Nucleic Acids Res. (2020) 48:W154–61. doi: 10.1093/nar/gkaa288
57. Yates, CM, Filippis, I, Kelley, LA, and Sternberg, MJE. SuSPect: enhanced prediction of single amino acid variant (SAV) phenotype using network features. J Mol Biol. (2014) 426:2692–701. doi: 10.1016/j.jmb.2014.04.026
58. Bendl, J, Stourac, J, Salanda, O, Pavelka, A, Wieben, ED, Zendulka, J, et al. PredictSNP: robust and accurate consensus classifier for prediction of disease-related mutations. PLoS Comput Biol. (2014) 10:e1003440. doi: 10.1371/journal.pcbi.1003440
59. Capriotti, E, Calabrese, R, and Casadio, R. Predicting the insurgence of human genetic diseases associated to single point protein mutations with support vector machines and evolutionary information. Bioinformatics. (2006) 22:2729–34. doi: 10.1093/bioinformatics/btl423
60. Capriotti, E, Altman, RB, and Bromberg, Y. Collective judgment predicts disease-associated single nucleotide variants. BMC Genomics. (2013) 14:S2. doi: 10.1186/1471-2164-14-S3-S2
61. Calabrese, R, Capriotti, E, Fariselli, P, Martelli, PL, and Casadio, R. Functional annotations improve the predictive score of human disease-related mutations in proteins. Hum Mutat. (2009) 30:1237–44. doi: 10.1002/humu.21047
62. Pejaver, V, Urresti, J, Lugo-Martinez, J, Pagel, K, Lin, G, Nam, H, et al. Inferring the molecular and phenotypic impact of amino acid variants with MutPred2. Nat Commun. (2020) 11:5918. doi: 10.1038/s41467-020-19669-x
63. Adzhubei, I, Jordan, DM, and Sunyaev, SR. Predicting functional effect of human missense mutations using PolyPhen-2. Curr Protoc Hum Genet. (2013):1–41. doi: 10.1002/0471142905.hg0720s76
64. Pagel, KA, Pejaver, V, Lin, GN, Nam, HJ, Mort, M, Cooper, DN, et al. When loss-of-function is loss of function: assessing mutational signatures and impact of loss-of-function genetic variants. Bioinformatics. (2017) 33:i389–98. doi: 10.1093/bioinformatics/btx272
65. Burge, C, and Karlin, S. Prediction of complete gene structures in human genomic DNA. J Mol Biol. (1997) 268:78–94. doi: 10.1006/jmbi.1997.0951
66. Scalzitti, N, Kress, A, Orhand, R, Weber, T, Moulinier, L, Jeannin-Girardon, A, et al. Spliceator: multi-species splice site prediction using convolutional neural networks. BMC Bioinform. (2021) 22:561. doi: 10.1186/s12859-021-04471-3
67. REESE, MG, EECKMAN, FH, KULP, D, and HAUSSLER, D. Improved splice site detection in genie. J Comput Biol. (1997) 4:311–23. doi: 10.1089/cmb.1997.4.311
68. Clavero, S, Bishop, DF, Giger, U, Haskins, ME, and Desnick, RJ. Feline congenital erythropoietic porphyria: two homozygous UROS missense mutations cause the enzyme deficiency and porphyrin accumulation. Mol Med. (2010) 16:381–8. doi: 10.2119/molmed.2010.00038
69. Hilton, S, Christen, M, Bilzer, T, Jagannathan, V, Leeb, T, and Giger, U. Dystrophin (DMD) missense variant in cats with Becker-type muscular dystrophy. Int J Mol Sci. (2023) 24:3192. doi: 10.3390/ijms24043192
70. Mauler, DA, Gandolfi, B, Reinero, CR, O’Brien, DP, Spooner, JL, Lyons, LA, et al. Precision medicine in cats: novel Niemann-pick type C1 diagnosed by whole-genome sequencing. J Vet Intern Med. (2017) 31:539–44. doi: 10.1111/jvim.14599
71. Kopke, MA, Diane Shelton, G, Lyons, LA, Wall, MJ, Pemberton, S, Gedye, KR, et al. X-linked myotubular myopathy associated with an MTM1 variant in a Maine coon cat. J Vet Intern Med. (2022) 36:1800–5. doi: 10.1111/jvim.16509
72. Christen, M, Janzen, N, Fraser, A, Sewell, AC, Jagannathan, V, Guevar, J, et al. L2HGDH missense variant in a cat with L-2-hydroxyglutaric aciduria. Genes (Basel). (2021) 12:682. doi: 10.3390/genes12050682
73. Owens, SL, Downey, ME, Pressler, BM, Birkenheuer, AJ, Chandler, DW, and Scott-Moncrieff, JC. Congenital adrenal hyperplasia associated with mutation in an 11β-hydroxylase-like gene in a cat. J Vet Intern Med. (2012) 26:1221–6. doi: 10.1111/j.1939-1676.2012.00971.x
74. Keijser, SFA, Fieten, H, Vos-Loohuis, M, Piek, CJ, Anderson, H, Donner, J, et al. Heterozygosity testing and multiplex DNA panel screening as a potential tool to monitor health and inbreeding in a small, closed dog population. Canine Genet Epidemiol. (2018) 5:12. doi: 10.1186/s40575-018-0068-6
75. Donner, J, Freyer, J, Davison, S, Anderson, H, Blades, M, Honkanen, L, et al. Genetic prevalence and clinical relevance of canine Mendelian disease variants in over one million dogs. PLoS Genet. (2023) 19:e1010651. doi: 10.1371/journal.pgen.1010651
76. Mahmood, K, Jung, CH, Philip, G, Georgeson, P, Chung, J, Pope, BJ, et al. Variant effect prediction tools assessed using independent, functional assay-based datasets: implications for discovery and diagnostics. Hum Genomics. (2017) 11:10. doi: 10.1186/s40246-017-0104-8
77. Barrs, VR, Gunew, M, Foster, SF, Beatty, JA, and Malik, R. Prevalence of autosomal dominant polycystic kidney disease in Persian cats and related-breeds in Sydney and Brisbane. Aust Vet J. (2001) 79:257–9. doi: 10.1111/j.1751-0813.2001.tb11977.x
78. Wess, G, Schinner, C, Weber, K, Küchenhoff, H, and Hartmann, K. Association of A31P and A74T polymorphisms in the myosin binding protein C3 gene and Hypertrophic cardiomyopathy in Maine coon and other breed cats. J Vet Intern Med. (2010) 24:527–32. doi: 10.1111/j.1939-1676.2010.0514.x
79. Longeri, M, Ferrari, P, Knafelz, P, Mezzelani, A, Marabotti, A, Milanesi, L, et al. Myosin-binding protein C DNA variants in domestic cats (A31P, A74T, R820W) and their association with hypertrophic cardiomyopathy. J Vet Intern Med. (2013) 27:275–85. doi: 10.1111/jvim.12031
Keywords: (clinical) genetic testing, genetic variant datasets, interpretation, reproducibility, in silico variant effect predictor tools, across-species classification, pathogenic, neutral
Citation: Boeykens F, Abitbol M, Anderson H, Casselman I, de Citres CD, Hayward JJ, Häggström J, Kittleson MD, Lepri E, Ljungvall I, Longeri M, Lyons LA, Ohlsson Å, Peelman L, Smets P, Vezzosi T, van Steenbeek FG and Broeckx BJG (2024) Development and validation of animal variant classification guidelines to objectively evaluate genetic variant pathogenicity in domestic animals. Front. Vet. Sci. 11:1497817. doi: 10.3389/fvets.2024.1497817
Received: 17 September 2024; Accepted: 18 November 2024;
Published: 05 December 2024.
Edited by:
Claire Rebecca Sharp, Murdoch University, AustraliaReviewed by:
Alex Silva Da Cruz, Pontifícia Universidade Católica de Goiás, BrazilCopyright © 2024 Boeykens, Abitbol, Anderson, Casselman, de Citres, Hayward, Häggström, Kittleson, Lepri, Ljungvall, Longeri, Lyons, Ohlsson, Peelman, Smets, Vezzosi, van Steenbeek and Broeckx. This is an open-access article distributed under the terms of the Creative Commons Attribution License (CC BY). The use, distribution or reproduction in other forums is permitted, provided the original author(s) and the copyright owner(s) are credited and that the original publication in this journal is cited, in accordance with accepted academic practice. No use, distribution or reproduction is permitted which does not comply with these terms.
*Correspondence: Bart J. G. Broeckx, YmFydC5icm9lY2t4QHVnZW50LmJl
†These authors have contributed equally to this work and share last authorship
Disclaimer: All claims expressed in this article are solely those of the authors and do not necessarily represent those of their affiliated organizations, or those of the publisher, the editors and the reviewers. Any product that may be evaluated in this article or claim that may be made by its manufacturer is not guaranteed or endorsed by the publisher.
Research integrity at Frontiers
Learn more about the work of our research integrity team to safeguard the quality of each article we publish.