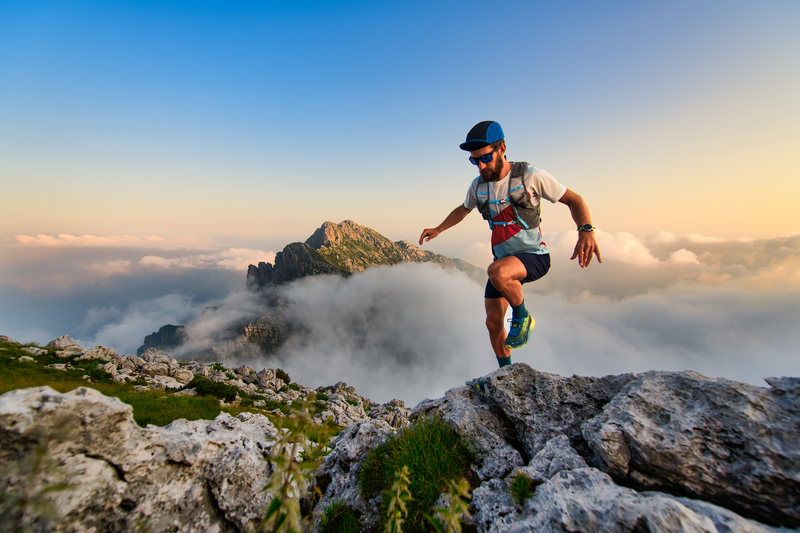
94% of researchers rate our articles as excellent or good
Learn more about the work of our research integrity team to safeguard the quality of each article we publish.
Find out more
ORIGINAL RESEARCH article
Front. Vet. Sci. , 07 November 2023
Sec. Veterinary Infectious Diseases
Volume 10 - 2023 | https://doi.org/10.3389/fvets.2023.1280161
This article is part of the Research Topic Insights in Emerging or Re-emerging Zoonoses View all 14 articles
The deletion of orphan response regulator CovR reduces the growth rate of Streptococcus suis serotype 2 (S. suis 2). In this study, metabolome and transcriptome profiling were performed to study the mechanisms underlying the poor growth of S. suis 2 caused by the deletion of orphan response regulator CovR. By comparing S. suis 2 (ΔcovR) and S. suis 2 (SC19), 146 differentially accumulated metabolites (upregulated: 83 and downregulated: 63) and 141 differentially expressed genes (upregulated: 86 and downregulated: 55) were identified. Metabolome and functional annotation analysis revealed that the growth of ΔcovR was inhibited by the imbalance aminoacyl tRNA biosynthesis (the low contents of L-lysine, L-aspartic acid, L-glutamine, and L-glutamic acid, and the high content of L-methionine). These results provide a new insight into the underlying poor growth of S. suis 2 caused by the deletion of orphan response regulator CovR. Metabolites and candidate genes regulated by the orphan response regulator CovR and involved in the growth of S. suis 2 were reported in this study.
Streptococcus suis (S. suis) is one of the main pathogenic bacteria on swine farms around the world (1). S. suis is also an emerging zoonotic pathogen that can cause a wide range of diseases (e.g., septicemia, endocarditis, arthritis, meningitis, and pneumonia) (2, 3). S. suis mainly causes death in 5- to 10-week-old post-weaned piglets, resulting in significant economic losses to the pig farming industry (4). According to the differences in capsular polysaccharide (CPS) antigenicity, 35 serotypes of S. suis strains were found and defined (5). Among these 35 serotypes, the S. suis 2 strain not only has the highest isolation rate but also poses a serious threat to the development of pig farming and human health (6).
Two-component systems (TCSs) play a crucial role in bacterial infection and response to changes in the external living environment, which have been identified in the vast majority of bacteria (7). The vast majority of TCSs in bacteria consist of a histidine kinase (HK) that receives stimulus signals and a cognate response regulator (RR) that transfers stimulus signals. Once HK receives external stimulus signals, a histidine residue located on the HK will be phosphorylated, and then the phosphoryl group is transferred to the aspartic acid residue on the RR. Subsequently, the phosphorylated RR will interact with the promoter areas of the target genes associated with the response to the environmental stimuli (8). TCSs can determine the survival and pathogenicity of bacteria by regulating various cellular processes, including competition, metabolism, antibiotic resistance, biofilm formation, pathogenicity, and stress response. Therefore, TCSs are viewed as attractive candidates for the development of new strategies for preventing bacterial infections (7, 9).
The CovR/S system consists of a sensor kinase gene (covS) and a RR gene (covR), which directly or indirectly regulate 15% of genes connected with bacterial pathogenesis in Streptococcus spp. (10–12). Due to the impact of CovR/S on a variety of critical virulence factors, it is considered the most essential TCS system for group A Streptococcus (GAS, Streptococcus pyogenes) (13). The CovR that can regulate the virulence of bacteria has been reported in many studies. In Streptococcus agalactia, for example, the ΔcovR strain has stronger hemolytic activity, adherence ability to epithelial cells, and higher lethality in the neonate rat sepsis model (14). Previous studies reported that CovR can bind to the promoter areas of the majority of virulence factor genes in GAS (13). These reports suggested that CovR can negatively regulate bacterial virulence. In bacteria, protein tyrosine kinases are linked to pathogenicity and exopolysaccharide synthesis (15). In Edwardsiella piscicida, adenylosuccinate synthase has been reported to have an inhibition effect on the host's nuclear factor-kappa B (NF-κB) signaling pathway, which is a common and important immune signaling pathway (16). Hemolysin is the key virulence component of S. suis 2, which creates holes in the target cell membrane and activates the inflammasome NLRP3 in order to release cytoplasmic K+ efflux and cause Streptococcal toxic shock-like syndrome (17). Initiation factors IF1, IF2, and IF3 allow Shine-Dalgarno (SD) sequence-lead mRNAs initiate transcription (18). Ser/Thr kinase (STK) controls a number of cellular activities, including stress response, biofilm formation, membrane production, sporulation, metabolism, pathogenicity, and growth processes in bacteria. Recently, STK has been reported to be related to the virulence of S. suis (19). However, it is still unclear how the CovR/S system regulates genes and metabolites to promote the survivability of S. suis 2.
In recent years, transcriptomics and metabolomics have been extensively utilized to explain the survival and pathogenic mechanism of pathogenic bacteria. To reveal the mechanism by which the orphan RR factor CovR regulates the survivability of S. suis 2, liquid chromatography-tandem mass spectrometry (LC-MS) was employed to dig for the differentially expressed metabolites between S. suis 2 (wild type) and ΔcovR (mutant) strains. Furthermore, the assessment of the differentially expressed genes (DEGs) was carried out using RNA-Seq and qRT-PCR. This study will not only reveal the genes and metabolites that are regulated by the orphan RR factor CovR involved in the survivability of S. suis 2 but also provide some potential targets for preventing and controlling S. suis 2 infections.
The S. suis 2 SC19 strain was donated by Prof. Chen Tan of Huazhong Agricultural University. SC19 is plated on Tryptose Soya agar (TSA) or cultured in Tryptose Soya broth (TSB) (Difco Laboratories, Detroit, MI, USA) with 10% (v/v) newborn bovine serum (Sijiqing Biological Engineering Materials Co., Ltd., Hangzhou, China) at 37°C. ΔcovR mutant and complemented CΔcovR strains are stored in our laboratory and cultured as described above (20).
The growth characteristics of wild-type SC19, ΔcovR mutant, and complemented CΔcovR strains were determined by transferring overnight bacterial cultures into fresh preheated TSB supplemented with 10% newborn bovine serum at a ratio of 1:1000. The bacteria were grown at 37°C, accompanied by shaking at 180 rpm for 12 h, and OD600 was determined by an Automatic Microbial Growth Curve Analyzer (MGC-200, Ningbo Scientz Biotechnology Co., Ltd., Ningbo, China) for every hour. One-way ANOVA test was used to verify the significance of growth characteristics of SC19, ΔcovR, and CΔcovR for every hour.
The total RNA was extracted using the TRIzol reagent (Takara, Dalian, China) from SC19 (n = 3) and ΔcovR (n = 3) as described by Rio et al. (21), and the genomic DNA in the total RNA was removed by using DNase I (Takara). A total of 2 μg of RNA was used to build the RNA-seq transcriptome library by using the TruSeqTM RNA sample preparation Kit (Illumina, San Diego, CA). Briefly, the Ribo-Zero Magnetic Kit (epicenter) was used to exhaust ribosomal RNA (rRNA), and then the fragmented mRNA was broken into short fragments (200 bp) by using a fragmentation buffer. The mRNA fragment was performed by RT-PCR using the SuperScript Double-Stranded cDNA Synthesis Kit (Invitrogen, CA) with random hexamer primers (Illumina). The dTTP was replaced with dUTP when the second strand was synthesized. Then, after end-repair and phosphorylation, the synthesized cDNA was treated with “A” base addition according to the protocol of Illumina's introduction. The UNG enzyme was used to recognize and degrade those second-strand cDNA with dUTP. The library was constructed using 200-bp cDNA target fragments of 2% low-range ultra-agarose. Subsequently, these fragments were amplified by performing 15 PCR cycles with Phusion DNA polymerase and quantified using TBS380. Finally, the paired-end RNA-seq sequencing library was constructed using Illumina Novaseq (2 × 150 bp read length).
The raw data collected from Illumina Hiseq sequencing were converted into raw data using base calling, and the results were saved in FASTQ file format. For mapping assembly sequences and distinct genes to reference sequences (GenBank: CP020863.1), Bowtie with default parameters (http://bowtiebio.sourceforge.net/index.shtml) was employed. FPKM (fragments per kilobase million) was used to quantify the quantity of genes and transcripts (http://deWeylab.biostat.wisc.edu/rsem/, https://ccb.jhu.edu/software/stringtie/). The transcriptome data were analyzed by the cloud platform of Shanghai Majorbio Bio-Pharm Technology Co., Ltd. (Shanghai, China). Additionally, the DEGs between ΔcovR and S. suis 2 strains were detected using the DESeq2 software. To control the probability of error with multiple test corrections (Benjamini/Hochberg, BH), the p-values were adjusted. DEGs were identified as their fold change ≥1.2 or ≤ 0.8 and adjusted p-values < 0.05. The GO analysis for DEGs was performed using the Goatools software, while the KEGG analysis was performed using R script.
Overnight, SC19 (n = 4) and ΔcovR (n = 4) cultures were diluted at a 1:1000 ratio into TSB supplemented with 10% newborn bovine serum, and the mixture was then incubated to the logarithm phase. A total of 100 ml of the bacterium was transferred to a clean tube and centrifuged at 12,000g at 4°C for 10 min, and then the supernatant was removed. The metabolites were extracted from the bacterium (SC19 and ΔcovR) by a 400-μl isovolumetric methanol and acetonitrile solution and then sonicated by an ultrasonic cleaner (SBL-10TD, Ningbo Xinzhi Biotechnology Co., Ltd.) at 40 kHz for 30 min at 5°C. After being placed at −20°C for 30 min, the mixture was centrifuged at 13,000g at 4°C for 15 min. The supernatant was carefully transferred to a new microtube and evaporated to dry under a gentle stream of nitrogen. Then, the samples were resuspended with 100 μl of loading isovolumetric acetonitrile and water solution by short sonication using an ultrasonic cleaner (Ningbo Scientz Biotechnology Co., Ltd.) in a 5°C water bath. The extracted metabolites were centrifuged at 13,000g for 15 min at 4°C and then UHPLC-MS/MS analysis was performed. The same volumes of all samples were mixed to be used as a pooled quality control.
The LC-MS System (UHPLC-Q Exactive HF-X System, Thermo Fisher Scientific, Shanghai, China) was used to analyze the extracted metabolites. First, the sample was injected into a Waters HSS T3 column (100 mm × 2.1 mm i.d., 1.8 μm), which was followed by mass spectrometry detection. The mobile phases consisted of two solvents: solvent A, which consisted of 95% 0.1% formic acid and 5% acetonitrile, and solvent B, which consisted of 47.5% 0.1% formic acid in acetonitrile with 47.5% isopropanol and 5% water. The solvent gradient employed the following conditions: First, the gradient changed from 0 B to 24.5% B at a flow rate of 0.4 ml/min for 3.5 min; then, the gradient changed from 24.5 B to 65% B for 1.5 min; the gradient changed from 65 B to 100% B for 0.5 min; the gradient remained at 100% B for 1.9 min with the flow rate being increased from 0.4 to 0.6 ml/min; the gradient changed from 100 B to 51.5% B for 0.2 min; the gradient changed from 51.5 B to 0% B for 0.2 min at a flow rate of 0.6 to 0.5 ml/min; the gradient remained at 0% B for 0.2 min at a flow rate of 0.5 to 0.4 ml/min; the system equilibration was performed as the gradient remained at 0% B for 1 min at a flow rate of 0.4 ml/min. The sample injection volume was 2 μl at a flow rate of 0.4 ml/min. The column temperature was maintained at 40°C throughout the analysis, and the samples were stored at 4°C. The mass spectrometric data were collected using continuous scanning in either positive or negative ion modes. Data acquisition was performed in the data-dependent acquisition mode. The full MS resolution was set at 60,000, and the MS/MS resolution was set at 7,500. The heater temperature and capillary temperature were set at 425°C and 325°C, respectively. The sheath gas flow rate was set at 50 arb and the aux gas was set at 13 arb. The ion-spray voltage floating was −3,500 V in negative mode and 3,500 V in positive mode. Normalized collision energy for MS/MS was set at 20-40-60 V rolling. The detection range for mass spectrometry was from 70 to 1,050 m/z.
A three-dimensional data matrix that included sample information, metabolite name, and mass spectral response intensity in CSV format was obtained by analyzing metabolite data with Progenesis QI (Waters Corporation, Milford, USA). Internal standard peaks, as well as any known false positive peaks that include noise, column bleed, and derivatized reagent peaks, were removed from the data matrix, which was then de-redundant and peak pooled. The metabolic mass spectra features were recognized by accurate mass, MS/MS fragment spectra, and isotope ratio differences were searched in reliable biochemical databases such as the Human Metabolome Database (HMDB) (http://www.hmdb.ca/) and the METLIN database (https://metlin.scripps.edu/).
After searching in the database, these data were uploaded to the Majorbio cloud platform (https://cloud.majorbio.com) for analysis. In each set of samples, at least 80% of the metabolic features detected were retained. The metabolic levels in specific samples that fell below the lower limit of quantitation were imputed with minimum metabolite values, and each metabolic feature was normalized by sum. The response intensity of the sample mass spectrum peaks was normalized using the sum normalization method to obtain the normalized data matrix. Those QC samples with variables that had a relative standard deviation >30% were removed, and a logarithmic transformation (log10) was applied to the final data matrix for subsequent analysis.
Next, principal component analysis (PCA) and orthogonal least squares partial discriminant analysis (OPLS-DA) were conducted on the preprocessed data array using the R package (Version 1.6.2). The stability of the model was evaluated through seven cycles of cross-validation. To identify significantly different metabolites, VIP values obtained from the OPLS-DA model and Student's t-test were used, with VIP >1 and p < 0.05 indicating significance. Metabolic pathway annotation was carried out using the KEGG data (https://www.kegg.jp/kegg/pathway.html) to determine the pathways with which the differential metabolites were associated. Pathway enrichment analysis was performed using the Python package scipy.stats, and Fisher's exact test was used to identify the most relevant biological pathways to the experimental treatments. The raw data were finally uploaded to MetaboLights, where they were given the unique identifier MTBLS8495 (www.ebi.ac.uk/metabolights/MTBLS8495).
In this study, the accuracy of RNA-seq data was validated by performing qRT-PCR on the selected DEGs. Among them, B9H01_RS09035 is glutamine ABC transporter substrate-binding protein; B9H01_RS06585 is glutamine ABC transporter permease; B9H01_RS06580 is glutamine ABC transporter permease; B9H01_RS05040 is peptide ABC transporter ATP-binding protein; B9H01_RS05035 is amino acid ABC transporter permease; B9H01_RS09790 is ABC transporter ATP-binding protein; and B9H01_RS01490, B9H01_RS06570, and B9H01_RS09040 are ABC transporters. To begin, RNA extraction was followed as described in the preceding part of the text, and 1 μg of RNA was reserved for transcription using a PrimeScript® RT reagent Kit and gDNA Eraser (Takara, Dalian, China). Next, a 7500 Fast Real-Time PCR System (Applied Biosystems, Foster City, CA, USA) was used to perform the PCR reaction using Taq Pro Universal SYBR qPCR Master Mix (Q712, Vazyme, Nanjing, China). The 16S RNA gene served as the control gene, and all mRNA transcription levels were reported as 2−ΔΔCt. The primers in Table 1 were designed according to the genomic sequence of SC19 and used for qRT-PCR.
The data in this study were analyzed using SPSS (Version 17.0, SPSS Inc., Chicago, IL, USA) and GraphPad Prism 8. Unpaired t-tests between two groups or one-way ANOVA among multiple groups were used to obtain p-values (*p < 0.05, **p < 0.01, ***p < 0.001; NS, not significant).
As shown in Figure 1, the growth of ΔcovR was significantly slower compared to that of SC19 and the complemented CΔcovR strains after 3 h.
Figure 1. The growth characteristics of SC19, ΔcovR, and complemented CΔcovR strains. (A) The growth curves of SC19, ΔcovR, and complemented CΔcovR strains. (B) The clones of SC19, ΔcovR, and complemented CΔcovR strains. Compare SC19 and ΔcovR; ns, no significance; *p < 0.5, **p < 0.01, ***p < 0.001.
For the purpose of determining the poor growth of mutant ΔcovR, metabolome profiling via untargeted LC-MS was performed on SC19 and mutant ΔcovR. The metabolites identified from LC-MS were presented in Supplementary Table S1. There was a total of 514 metabolites identified, which were classified into 11 categories. Supplementary Figure S1 provides the PCA of SC19, ΔcovR, and QC samples, which present significant separation. The coloration of each sample was analyzed using metabolite concentration data and shown in Supplementary Figure S1, which suggests that different samples were distinguished significantly. This means that the metabolome data were highly reliable. These results also suggested that SC19 and ΔcovR samples had substantially different metabolite profiles.
The uniquely mapped read ratio of all samples was higher than 96%, which means the reference sequence was reliable. To acquire Differentially accumulated metabolites (DAMs) between SC19 and mutant ΔcovR strains, parameters such as p-values <0.05, |Log2 Fold Change| >1, and variable importance in the projection (VIP) >1 were used to analyze all metabolite data. In total, 146 DAMs (83 were upregulated and 63 were downregulated) were acquired (Figures 2A, B, Supplementary Table S2). The results of the Kyoto Encyclopedia of Genes and Genomes (KEGG, Figure 2C) analysis showed that arginine biosynthesis (map00220), pyrimidine metabolism (map00240), aminoacyl-tRNA biosynthesis (map00970), alanine, aspartate and glutamate metabolism (map00250), ABC transporters (map02010), and lysine biosynthesis (map00300) were significantly enriched. Further analysis results showed that DMAs in these enrichment pathways were likely involved in aminoacyl-tRNA biosynthesis, energy metabolism, and amino acid biosynthesis and metabolism (Table 2). In addition, the 146 DMAs could be categorized into >10 classes (Figure 2D). These analysis results suggest that DAMs involved in aminoacyl-tRNA biosynthesis, energy metabolism, and amino acid biosynthesis and metabolism may play a key role in slowing down the growth rate of the mutant ΔcovR strain.
Figure 2. Differentially accumulated metabolites between SC19 and mutant ΔcovR strains. (A) Volcano plots displayed DAMs that showed significant change (upregulated and downregulated) or no significant change between SC19 and mutant ΔcovR strains. (B) The heat map of mutant ΔcovR and two SC19 strains. (C) KEGG enrichment analysis of DAMs. (D) Pie chart depicting the categories of DAMs.
The DEGs between SC19 and mutant ΔcovR strains were analyzed. There were 145,666,522 (SC19, 72,699,732; mutant ΔcovR, 72,966,790) total reads generated by RNA-seq. A total of 141,860,342 (SC19, 70,781,282; mutant ΔcovR, 71,079,060) clean reads were obtained by eliminating the low-quality reads. A total of 141,860,342 (SC19, 69,990,276; mutant ΔcovR, 70,298,114) mapped reads were collected by aligning the high-quality reads to the reference genome (two SC19 chromosomes, complete genome, GenBank: CP020863.1). The correlation analysis showed significantly different relationships between ΔcovR and SC19 strains (Figure 3A).
Figure 3. DEGs between mutant ΔcovR and SC19 strains. (A) The heat map of mutant ΔcovR and SC19 strains. (B) Go annotation analysis. (C) The KEGG enrichment pathways of 141 DEGs.
To explore the candidate genes responsible for poor growth of ΔcovR strains, DEGs (|Log2 Fold Change| >1.2 or <0.8) were identified between SC19 and mutant ΔcovR strains. A total of 141 DEGs (86 were upregulated and 55 were downregulated) were identified. The GO annotation analysis result showed that the 141 DEGs were classified into three categories (Figure 3B), among which the integral component of membrane (36, 25.5%) was the largest group; the second largest group was ATP binding (26, 18.4%); only a few were related to metal ion binding (3, 2.1%) and iron-sulfur cluster assembly (3, 2.1%). As shown in Figure 3C, the enrichment pathways in KEGG terms were ABC transporters, glycerolipid metabolism, propanoate metabolism, carbon fixation pathways in prokaryotes, valine, leucine, and isoleucine degradation, and glycosaminoglycan degradation (Supplementary Table S3).
To validate the accuracy of the identified DEGs by RNA-seq, nine DEGs were validated by qRT-PCR (Figure 4). Consistent with the RNA-Seq results (Supplementary Table S3), qRT-PCR results showed that the expression levels of the identified genes in the mutant ΔcovR strain were significantly increased compared with those in the SC19 strain. Further research showed that all the gathered data from metabolome and transcriptome profiles had high reliability according to the correlation analysis (Figure 5). We could conclude that variations in metabolite accumulation between SC19 and mutant ΔcovR strains were closely regulated by different gene expressions.
Figure 4. The qRT-PCR validation of genes related to the growth of S. suis 2. (A) Gene expression levels of B9H01_RS09035. (B) Gene expression levels of B9H01_RS06570. (C) Gene expression levels of B9H01_RS09040. (D) Gene expression levels of B9H01_RS01490. (E) Gene expression levels of B9H01_RS09790. (F) Gene expression levels of B9H01_RS05040. (G) Gene expression levels of B9H01_RS05035. (H) Gene expression levels of B9H01_RS06585. (I) Gene expression levels of B9H01_RS06580. *p < 0.05, **p < 0.01, ***p < 0.001, ****p < 0.0001.
Figure 5. Correlation analysis of DEGs and DAMs in the mutant ΔcovR and SC19 strains. The levels of correlation of each sample were indicated by the color, from high (red) to low (blue), and the correlation coefficient between samples was represented by the Z-score. *p < 0.05, **p < 0.01, ***p < 0.001.
Prior studies have reported the importance of the TCS CovR in S. suis 2 virulence and growth characteristics (14, 22–25). However, the mechanism by which CovR deletion affects the growth of S. suis 2 is not yet clear.
First, we constructed the covR deletion mutant strain. We discovered that the mutant covR grew significantly more slowly than the parental strain SC19 on the growth curve (Figure 1A) and it also growth slow on the TSA plate (Figure 1B). This phenomenon was also presented by Pan et al. (22). To further understand the mechanism of poor growth of the ΔcovR mutant, transcriptome and metabolome profiling were performed.
Energy metabolism, including the tricarboxylic acid cycle (TCA), pentose phosphate pathway, and glycolysis pathway, is one of the most important metabolic pathways in the growth of microorganisms. The TCA is the main pathway by which many substrates, including glycogen, fatty acids, and proteins, enter central carbon metabolism via acetyl-coenzyme A (acetyl-CoA) (26). It is also the main pathway that provides energy for the biosynthesis of complex macromolecules such as glycogen, amino acids, and proteins (27). As we all know, acetyl-CoA and oxalosuccinic acid are the intermediate substances in the TCA, and phosphopantothenate is considered a precursor to the synthesis of coenzyme A (28).
In our study, the metabolism profile showed that oxalosuccinic acid and acetyl-CoA were up-accumulated and D-4'-Phosphopantothenate was down-accumulated. However, the transcriptome profile showed that there were no significant changes in gene expression levels of acetyl-CoA and oxalosuccinic acid between mutant ΔcovR and SC19 strains. The reason for the up-accumulation of acetyl-CoA and oxalosuccinic acid may be due to less consumption rather than excessive production. The D-4'-Phosphopantothenate was down-accumulated in metabolism and down-expression in the transcriptome, which means there were fewer precursors of coenzyme A, but the level of acetyl-CoA was still up-accumulated. Less consumption is the main reason for the up-accumulation of acetyl-CoA and oxaloacetylsuccinic acid in the mutant ΔcovR strain. Therefore, the mutant ΔcovR consumed less acetyl-CoA compared to SC19 and caused less energy supply to the biosynthesis of complex macromolecules, which resulted in poor growth.
Amino acid synthesis and metabolism play a key role in bacterial growth. There are over 300 naturally occurring amino acids, and only 22 constitute the monomer units of proteins, which can be classified as acidic or basic and hydrophilic or hydrophobic according to their respective side chain R (29). 1-aminocyclopropanecarboxylic acid (ACC) is a cyclopropane-containing non-proteinogenic amino acid isolated from various fruits and plant tissues and is considered the immediate precursor of the plant hormone ethylene (30). Recently, ACC has been found to perform similar synthesis machinery to plants in microorganisms (31, 32). Citrulline and ornithine, as we all know, are the intermediate substances of the urea cycle and have recently been reported to be involved in the metabolism of arginine in different bacteria (33). N-acetylglutamate in prokaryotes is also the first intermediate in arginine biosynthesis (34). As we all know, the level of amino acids can influence the amount of aminoacyl-tRNA. Several reports have shown that, in E. coli and S. typhimurium, the levels of aminoacyl-tRNA synthetases change with the rate of growth in different media (35). Our study found that seven metabolites related to amino acid biosynthesis and metabolism were differently accumulated, including two up-accumulated (1-aminocyclopropanecarboxylic acid and allysine) and five down-accumulated (citrulline, L-ornithine, N-acetylaspartate, N-acetyl-L-aspartic acid, and N-acetylglutamic acid) in mutant ΔcovR compared to S. suis 2 SC19. The down-accumulation of citrulline, ornithine, and N-acetylglutamate were all involved in arginine metabolism, which means that arginine may play a key role in the growth of SC19.
The argF, as a gene code ornithine carbamoyltransferase, was downregulated in mutant ΔcovR compared to SC19 in the transcriptome. Ornithine carbamoyltransferase can catalyze the condensation of ornithine with carbamoyl phosphate to form the amino acid citrulline, which is subsequently delivered to the cytosol (36). In bacteria, urea and ornithine produced by the decomposition of arginine can be further utilized as nitrogen and carbon sources (37). In other words, the mutant ΔcovR produced less arginine than S. suis 2 SC19, which decreased the availability of nitrogen for the biosynthesis of amino acids. Thus, the CovR deletion influenced the rate of amino acid biosynthesis, resulting in poor growth of S. suis 2.
Aminoacyl-tRNA synthetases play a key role in the process of aminoacyl-tRNA biosynthesis, which produces aminoacyl-tRNA, AMP, and PPi by esterifying an amino acid to the 3′ end of a tRNA and hydrolyzing one molecule of ATP (38). Previous studies showed that translation elongation, which is a process of the aminoacyl-tRNA translocation of the ribosome, is an essential step in protein synthesis (39), and translation is the key to defining the growth rate (40). Therefore, the quantity of aminoacyl-tRNA will have an impact on the pace of bacterial growth.
In our study, the aminoacyl-tRNA biosynthesis related to metabolism (L-methionine, L-lysine, L-aspartic Acid, L-glutamate, and L-glutamine) is down-accumulated except L-methionine in the mutant ΔcovR strain. Meanwhile, the amino acid ABC transporter substrate-binding protein genes (B9H01_RS09940) and the amino acid ABC transporter permease gene (B9H01_RS09935) were downregulated, and the amino acid ABC transporter ATP-binding protein genes (B9H01_RS06570, B9H01_RS09040, and B9H01_RS05040) were upregulated in mutant ΔcovR compared to SC19. The amino acid ABC transporter is a kind of active transporter for amino acid uptake (41). Therefore, we assume that mutant ΔcovR has less amino acid ABC transporters that involve in amino acid uptake, thus expressing more ATP-binding proteins to transport more amino acids to maintain homeostasis, but still making the imbalance of aminoacyl tRNA biosynthesis, ultimately leading to slower growth.
The mechanism of slow growth of ΔcovR was proposed in Figure 6 can be explained as follows: first, the mutant ΔcovR exhibits reduced consumption of acetyl-CoA, resulting in a decrease in ATP production for biosynthesis. This decrease in ATP production, in turn, leads to a shortage of nitrogen resources due to reduced arginine biosynthesis, which consequently slows down the rate of amino acid biosynthesis. Ultimately, the combination of decreased ATP and amino acid production results in a reduced availability of aminoacyl tRNA, leading to poor growth in mutant ΔcovR.
Figure 6. Mechanisms of the poor growth of Streptococcus suis serotype 2 after orphan response regulator CovR deletion. (A) Synthesis of aminoacyl tRNAs in SC19 in ΔcovR strain. (B) Synthesis of aminoacyl tRNAs in ΔcovR strain.
The original contributions presented in the study are publicly available. This data can be found at: https://www.ebi.ac.uk/metabolights, MTBLS8495 and https://www.ncbi.nlm.nih.gov/, PRJNA879942.
YZ: Data curation, Funding acquisition, Writing—original draft, Writing—review & editing. BZ: Data curation, Formal analysis, Writing—original draft, Writing—review & editing. YX: Data curation, Formal analysis, Writing—original draft, Methodology, Writing—review & editing. RL: Writing—review & editing, Data curation. HL: Data curation, Formal analysis, Writing—review & editing. PW: Methodology, Writing—review & editing. XY: Writing—review & editing.
The author(s) declare financial support was received for the research, authorship, and/or publication of this article. This work was supported by grants from the National Natural Science Foundation of China (32102674 and 32202814), Hubei Province Education Department Scientific Research Item (Q20211604), School Research Projects of Wuhan Polytechnic University (2020Y16), Research Funding (Doctoral research start-up funding) of Wuhan Polytechnic University 2021R2020, and Open Project Of Hubei Key Laboratory of Animal Nutrition and Feed Science, Wuhan Polytechnic University (202314).
We thank our students and technicians for their contributions to this research. We also thank the research platform provided by the School of Animal Science and Nutritional Engineering, Wuhan Polytechnic University, and the Kanehisa Laboratory grant permission to reproduced KEGG database IMAGE(S) (SIC) in the published article (KEGG Copyright Permission 230017).
The authors declare that the research was conducted in the absence of any commercial or financial relationships that could be construed as a potential conflict of interest.
All claims expressed in this article are solely those of the authors and do not necessarily represent those of their affiliated organizations, or those of the publisher, the editors and the reviewers. Any product that may be evaluated in this article, or claim that may be made by its manufacturer, is not guaranteed or endorsed by the publisher.
The Supplementary Material for this article can be found online at: https://www.frontiersin.org/articles/10.3389/fvets.2023.1280161/full#supplementary-material
1. Feng Y, Zhang H, Wu Z, Wang S, Cao M, Hu D, et al. Streptococcus suis infection: an emerging/reemerging challenge of bacterial infectious diseases? Virulence. (2014) 5:477–97. doi: 10.4161/viru.28595
2. Zhang S, Sella M, Sianturi J, Priegue P, Shen D, Seeberger PH, et al. Discovery of oligosaccharide antigens for semi-synthetic glycoconjugate vaccine leads against Streptococcus suis serotypes 2, 3, 9 and 14. Angewandte Chemie. (2021) 60:14679–92. doi: 10.1002/anie.202103990
3. Wang Y, Wang Y, Sun L, Grenier D, Yi L. Streptococcus suis biofilm: regulation, drug-resistance mechanisms, and disinfection strategies. Appl Microbiol Biotechnol. (2018) 102:9121–9. doi: 10.1007/s00253-018-9356-z
4. Segura M, Calzas C, Grenier D, Gottschalk M. Initial steps of the pathogenesis of the infection caused by Streptococcus suis: fighting against nonspecific defenses. FEBS Lett. (2016) 590:3772–99. doi: 10.1002/1873-3468.12364
5. Okura M, Osaki M, Nomoto R, Arai S, Osawa R, Sekizaki T, et al. Current taxonomical situation of Streptococcus suis. Pathogens. (2016) 5:45. doi: 10.3390/pathogens5030045
6. Goyette-Desjardins G, Auger JP, Xu J, Segura M, Gottschalk M. Streptococcus suis, an important pig pathogen and emerging zoonotic agent-an update on the worldwide distribution based on serotyping and sequence typing. Emerg Microb Inf. (2014) 3:e45. doi: 10.1038/emi.2014.45
7. He LY, Le YJ, Guo Z, Li S, Yang XY. The role and regulatory network of the ciarh two-component system in Streptococcal species. Front Microbiol. (2021) 12:693858. doi: 10.3389/fmicb.2021.693858
8. Gao R, Stock AM. Biological insights from structures of two-component proteins. Ann Rev Microbiol. (2009) 63:133–54. doi: 10.1146/annurev.micro.091208.073214
9. Gotoh Y, Eguchi Y, Watanabe T, Okamoto S, Doi A, Utsumi R, et al. Two-component signal transduction as potential drug targets in pathogenic bacteria. Curr Opin Microbiol. (2010) 13:232–9. doi: 10.1016/j.mib.2010.01.008
10. Langshaw EL, Pandey M, Good MF. Cellular interactions of covr/s mutant group A Streptococci. Microbes Infection. (2018) 20:531–5. doi: 10.1016/j.micinf.2017.12.009
11. Dmitriev A, Mohapatra SS, Chong P, Neely M, Biswas S, Biswas I, et al. CovR-controlled global regulation of gene expression in Streptococcus mutans. PLoS ONE. (2011) 6:e20127. doi: 10.1371/journal.pone.0020127
12. Horstmann N, Tran CN, Brumlow C, DebRoy S, Yao H, Nogueras Gonzalez G, et al. Phosphatase activity of the control of virulence sensor kinase covs is critical for the pathogenesis of group A Streptococcus. PLoS Pathog. (2018) 14:e1007354. doi: 10.1371/journal.ppat.1007354
13. Horstmann N, Myers KS, Tran CN, Flores AR, Shelburne Iii SA. CovS inactivation reduces covr promoter binding at diverse virulence factor encoding genes in group A Streptococcus. PLoS Pathog. (2022) 18:e1010341. doi: 10.1371/journal.ppat.1010341
14. Lamy MC, Zouine M, Fert J, Vergassola M, Couve E, Pellegrini E, et al. Covs/covr of group B Streptococcus: a two-component global regulatory system involved in virulence. Mol Microbiol. (2004) 54:1250–68. doi: 10.1111/j.1365-2958.2004.04365.x
15. Ilan O, Bloch Y, Frankel G, Ullrich H, Geider K, Rosenshine I, et al. Protein tyrosine kinases in bacterial pathogens are associated with virulence and production of exopolysaccharide. EMBO J. (1999) 18:3241–8. doi: 10.1093/emboj/18.12.3241
16. Hu F, Zhang Y, Liu Q, Wang Z. PurA facilitates Edwardsiella piscicida to escape NF-κB signaling activation. Fish Shellfish Immunol. (2022) 124:254–60. doi: 10.1016/j.fsi.2022.04.001
17. Li X, Li Q, Zhang Z, Wang C, Huo X, Lai H, et al. Canagliflozin inhibited the activity of hemolysin and reduced the inflammatory response caused by Streptococcus suis. Int J Mol Sci. (2023) 24:13074. doi: 10.3390/ijms241713074
18. Rodnina MV. Translation in Prokaryotes. Cold Spring Harb Perspect Biol. (2018) 10:a032664. doi: 10.1101/cshperspect.a032664
19. Li W, Yin Y, Meng Y, Ma Z, Lin H, Fan H, et al. The phosphorylation of phosphoglucosamine mutase GlmM by Ser/Thr kinase STK mediates cell wall synthesis and virulence in Streptococcus suis serotype 2. Vet Microbiol. (2021) 258:109102. doi: 10.1016/j.vetmic.2021.109102
20. Zhang YY, Li R, Li Q, Zhu YW, Yang XP, Zhao D, et al. Orphan response regulator CovR plays positive regulative functions in the survivability and pathogenicity of Streptococcus suis serotype 2 isolated from a pig. BMC Vet Res. (2023).
21. Rio DC, Ares M, Hannon GJ, Nilsen TW. Purification of RNA using TRIzol (TRI reagent). Cold Spring Harb Protoc. (2010) 6:pdb.prot5439. doi: 10.1101/pdb.prot5439
22. Pan X, Ge J, Li M, Wu B, Wang C, Wang J, et al. The orphan response regulator CovR: a globally negative modulator of virulence in Streptococcus suis serotype 2. J Bacteriol. (2009) 191:2601–12. doi: 10.1128/JB.01309-08
23. Jiang SM, Cieslewicz MJ, Kasper DL, Wessels MR. Regulation of virulence by a two-component system in group B Streptococcus. J Bacteriol. (2005) 187:1105–13. doi: 10.1128/JB.187.3.1105-1113.2005
24. Alam FM, Turner CE, Smith K, Wiles S, Sriskandan S. Inactivation of the covr/s virulence regulator impairs infection in an improved murine model of Streptococcus pyogenes naso-pharyngeal infection. PLoS ONE. (2013) 8:e61655. doi: 10.1371/journal.pone.0061655
25. Lun S, Willson PJ. Expression of green fluorescent protein and its application in pathogenesis studies of serotype 2 Streptococcus suis. J Microbiol Methods. (2004) 56:401–12. doi: 10.1016/j.mimet.2003.11.012
26. Khomyakova M, Bükmez Ö, Thomas LK, Erb TJ, Berg IAA. Methylaspartate cycle in haloarchaea. Science. (2011) 331:334–7. doi: 10.1126/science.1196544
28. Thomès L, Lescure A. Mosaic evolution of the phosphopantothenate biosynthesis pathway in bacteria and archaea. Genome Biol Evol. (2021) 13:262. doi: 10.1093/gbe/evaa262
29. Bullwinkle TJ, Ibba M. Translation quality control is critical for bacterial responses to amino acid stress. Proc Nat Acad Sci U S A. (2016) 113:2252–7. doi: 10.1073/pnas.1525206113
30. Wessjohann LA, Brandt W, Thiemann T. Biosynthesis and metabolism of cyclopropane rings in natural compounds. Chem Rev. (2003) 103:1625–48. doi: 10.1021/cr0100188
31. Zha L, Jiang Y, Henke MT, Wilson MR, Wang JX, Kelleher NL, et al. Colibactin assembly line enzymes use s-adenosylmethionine to build a cyclopropane ring. Nat Chem Biol. (2017) 13:1063–5. doi: 10.1038/nchembio.2448
32. Maruyama C, Chinone Y, Sato S, Kudo F, Ohsawa K, Kubota J, et al. C-methylation of s-adenosyl-l-methionine occurs prior to cyclopropanation in the biosynthesis of 1-amino-2-methylcyclopropanecarboxylic acid (norcoronamic acid) in a bacterium. Biomolecules. (2020) 10:775. doi: 10.3390/biom10050775
33. Eick S, Lussi A. Arginine: a weapon against cariogenic biofilm? Oral Biofilms. (2021) 29:80–90. doi: 10.1159/000510203
34. Caldovic L, Tuchman M. N-acetylglutamate and its changing role through evolution. Biochem. J. (2003) 372:279–90. doi: 10.1042/BJ20030002
35. Parker J, Neidhardt FC. Metabolic regulation of aminoacyl-tRNA synthetase formation in bacteria. Biochem Biophys Res Commun. (1972) 49:495–501. doi: 10.1016/0006-291X(72)90438-X
36. Wraith J. Ornithine carbamoyltransferase deficiency. Arch Dis Child. (2001) 84:84–8. doi: 10.1136/adc.84.1.84
37. Hernández VM, Arteaga A, Dunn MF. Diversity, properties and functions of bacterial arginases. FEMS Microbiol Rev. (2021) 45:304. doi: 10.1093/femsre/fuab034
38. Gomez MAR, Ibba M. Aminoacyl-trna synthetases. RNA. (2020) 26:910–36. doi: 10.1261/rna.071720.119
39. Xu B, Liu L, Song G. Functions and regulation of translation elongation factors. Front. Mol. Biosci. (2021) 8:816398. doi: 10.3389/fmolb.2021.816398
40. Belliveau NM, Chure G, Hueschen CL, Garcia HG, Kondev J, Fisher DS, et al. Fundamental limits on the rate of bacterial growth and their influence on proteomic composition. Cell Syst. (2021) 12:924–44. doi: 10.1016/j.cels.2021.06.002
Keywords: Streptococcus suis type 2, orphan response regulator CovR, ΔcovR, metabolome profiling, transcriptome sequencing, poor growth, aminoacyl tRNA
Citation: Zong B, Xiao Y, Li R, Li H, Wang P, Yang X and Zhang Y (2023) Transcriptome and metabolome profiling to elucidate the mechanism underlying the poor growth of Streptococcus suis serotype 2 after orphan response regulator CovR deletion. Front. Vet. Sci. 10:1280161. doi: 10.3389/fvets.2023.1280161
Received: 19 August 2023; Accepted: 10 October 2023;
Published: 07 November 2023.
Edited by:
Santiago Mirazo, University of the Republic, UruguayReviewed by:
German Matias Traglia, CENUR Litoral Norte, Universidad de La Republica, Salto, UruguayCopyright © 2023 Zong, Xiao, Li, Li, Wang, Yang and Zhang. This is an open-access article distributed under the terms of the Creative Commons Attribution License (CC BY). The use, distribution or reproduction in other forums is permitted, provided the original author(s) and the copyright owner(s) are credited and that the original publication in this journal is cited, in accordance with accepted academic practice. No use, distribution or reproduction is permitted which does not comply with these terms.
*Correspondence: Yanyan Zhang, zhangyanyana305@163.com
†These authors have contributed equally to this work
Disclaimer: All claims expressed in this article are solely those of the authors and do not necessarily represent those of their affiliated organizations, or those of the publisher, the editors and the reviewers. Any product that may be evaluated in this article or claim that may be made by its manufacturer is not guaranteed or endorsed by the publisher.
Research integrity at Frontiers
Learn more about the work of our research integrity team to safeguard the quality of each article we publish.