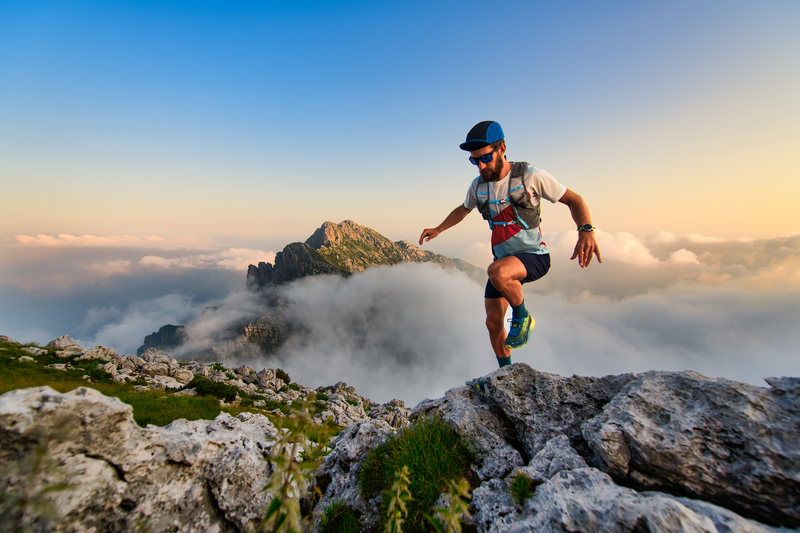
95% of researchers rate our articles as excellent or good
Learn more about the work of our research integrity team to safeguard the quality of each article we publish.
Find out more
ORIGINAL RESEARCH article
Front. Vet. Sci. , 16 December 2021
Sec. Livestock Genomics
Volume 8 - 2021 | https://doi.org/10.3389/fvets.2021.788316
This article is part of the Research Topic Marbling Fat in Livestock View all 13 articles
The enzyme long-chain acyl-CoA synthetase 1 (ACSL1) is essential for lipid metabolism. The ACSL1 gene controls unsaturated fatty acid (UFA) synthesis as well as the formation of lipid droplets in bovine adipocytes. Here, we used RNA-Seq to determine lncRNA and mRNA that regulate UFA synthesis in bovine adipocytes using RNA interference and non-interference with ACSL1. The corresponding target genes of differentially expressed (DE) lncRNAs and the DE mRNAs were found to be enriched in lipid and FA metabolism-related pathways, according to GO and KEGG analyses. The differentially expressed lncRNA- differentially expressed mRNA (DEL-DEM) interaction network indicated that some DELs, such as TCONS_00069661, TCONS_00040771, TCONS_ 00035606, TCONS_00048301, TCONS_001309018, and TCONS_00122946, were critical for UFA synthesis. These findings assist our understanding of the regulation of UFA synthesis by lncRNAs and mRNAs in bovine adipocytes.
As a member of the ACSL family (ACSL1, 3, 4, 5, and 6), long-chain acyl-CoA synthase 1 (ACSL1) is needed for the activation, transportation, and degradation of fatty acids (FAs), as well as lipid synthesis (1, 2). ACSL1 is found on the outer mitochondrial membrane (2) and converts long-chain FAs into fatty acyl-CoA to synthesize triglycerides (3), stimulate FA deposition, activate FAs (1), and finally to enter the β-oxidation pathway (4). Previous research has found that the presence of long-chain unsaturated FAs in beef, such as DHA (22: 6, n−3) and EPA (20: 5, n−3), aids in the prevention of cardiovascular disease, atherosclerosis, and the promotion of fetal brain and visual growth (5–8). A quantitative trait locus (QTL) study revealed that ACSL1 influences the relative content of UFAs, omega-3 FAs, polyunsaturated FAs, docosapentaenoic acid, and DHA (9). Furthermore, overexpression of ACSL1 activates and transports FA for the synthesis of diglycerides and phospholipids rather than cholesterol esters (10). In adipocytes, the arachidonic acid levels were further stimulated by the overexpression of ACSL1 (11). Furthermore, four transcription factors, E2F1, E2F4, Sp1, and KLF15, symbiotically control ACSL1 expression, suggesting that ACSL1 controls UFA synthesis in cells (12). We have also observed, using RNA interference (13) and gene overexpression (14), that ACSL1 controlled UFA synthesis and the formation of lipid droplets in bovine adipocytes.
Long non-coding RNAs (lncRNAs) are noncoding RNA molecules > 200 nt that are found in the nucleus/cytoplasm and undergo eukaryotic transcription (15). Earlier, lncRNAs were regarded as a byproduct of the transcription of RNAP II and thought to have minimal physiological activity. However, numerous studies have shown that they act as multi-level regulators of protein-coding gene expression, by epigenetic (16, 17), transcriptional (18, 19), and post-transcriptional regulation (20, 21). RNA-seq is effective for identifying differentially expressed (DE) genes as well as quantifying transcriptomes (22, 23). This technique has been frequently used in the functional analysis of lncRNAs/mRNAs in adipose tissue in cattle (24–27), sheep (28), pigs (29), and chickens (30). Jiang et al. (25) isolated 3,716 candidate lncRNAs from fat samples from calves and adult cattle and confirmed their crucial role in cattle of various ages. Liu et al. (26) demonstrated that genes, such as PLIN1, PLIN2, and PPARGC1A regulated various lipid-related pathways. Cai et al. (31) identified a novel lncRNA, BADLNCR1, that suppressed bovine adipogenesis by inhibiting GLRX5 expression. However, the roles of lncRNA/mRNAs in UFA synthesis in bovine adipocytes are poorly understood. Here, we used RNA-Seq to examine the lncRNAs/mRNA levels in bovine adipocytes by RNA interference and non-interference of ACSL1. We screened both lncRNAs and mRNAs in terms of UFA synthesis to understand their role in the regulation of UFA synthesis in bovine adipocytes.
A healthy 1-day-old calf was born and raised at the animal farm of Gansu Agricultural University (LanZhou, China) and humanely slaughtered in a slaughterhouse, followed by the collection of the perirenal adipose tissue along with heart, spleen, kidney, liver, lung, and leg muscle tissues. The study protocol was approved by the Gansu Agricultural University, China, as well as the Ministry of Science and Technology of the People's Republic of China (Approval number 2006–398). Animal experimentation, including sample collection, was performed in agreement with the ethical commission's guidelines of Gansu Agricultural University. Furthermore, the experimental protocol complied with the local animal welfare guidelines.
The perirenal adipose tissue of 1-day-old calf (from the animal farm of Gansu Agricultural University) was collected and shredded in PBS, followed by the addition of type I collagenase (200 U/mL). The solution was shaken at 37°C for 1 h, and enzymatic digestion was stopped by the addition of an equal volume of complete medium. The digested product was serially filtered through 100 μm and 40 μm mesh filters, followed by centrifugation (1,500 rpm, 10 min). The erythrocyte lysate was mixed with the pellet and centrifuged (1,500 rpm, 10 min). After discarding the supernatant, a complete medium was added to the pellet, mixed, and centrifuged twice (1,500 rpm, 10 min). The pellet was resuspended, and the cells were seeded in a 10 cm cell culture dish and cultured in 5% CO2 at 37°C. The medium was refreshed after 12 h to remove the non-adherent cells and was refreshed every 48 h. At 90% confluence, the cells were removed with trypsin and frozen in liquid nitrogen.
Bovine pre-adipocytes were grown and passaged to the F3 generation, and cells were isolated on differentiation days 0, 2, 4, 6, and 8. We performed qRT-PCR to assess ACSL1 expression at different stages of differentiation.
The complete sequence of bovine ACSL1 (GenBank No. 537161) was used to design and synthesize three siRNA sequences (Guangzhou Ruibo Biological Company). Table 1 shows the sequence information.
Next, the synthesized siRNA-ACSL1 and the corresponding siRNA-Non-specific Control (siRNA-NC) were transfected into bovine adipocytes. After 48 h of culture, we performed qRT-PCR to determine ACSL1 mRNA expression to screen the siRNA-ACSL1 with the highest interference efficiency.
Next, we sequenced the bovine adipocytes in which ACSL1 expression was silenced and those that exhibited normal expression.
We used TRIzol reagent to isolate total RNA from siRNA-ACSL1 or siRNA-NC bovine adipocytes, followed by strict quality control of the RNA samples. First, 1% agarose gel electrophoresis (AGE) was used to analyze RNA integrity and the presence of DNA contamination. RNA purity was measured using an IMPLEN NanoPhotometer® spectrophotometer and quantified using an RNA analysis kit and a Qubit®2.0 Fluorometer. Finally, the RNA Nano 6000 Assay Kit was used to assess RNA integrity using the Agilent Bioanalyzer 2100 system.
The cDNA library was prepared using the NEBNext Ultra Directional RNA Library Prep Kit for Illumina sequencing using a combination of equal amounts of total RNA of two parallel bovine adipocytes cultures in equal ratios of siRNA-ACSL1/siRNA-NC. The total RNA was from 6 dishes of bovine adipocytes, of which 3 dishes were treated with ACSL1 gene interference, and the other 3 dishes were the control group. The Epicenter Ribo-zero rRNA Removal kit was used for the removal of the rRNA, followed by the construction of six libraries, which were labeled si_ACSL1_1, si_ACSL1_2, si_ACSL1_3, NC_ACSL1_1, NC_ACSL1_2, and NC_ACSL1_3, respectively. Briefly, poly-T oligo-attached magnetic beads were used to obtain mRNA, followed by fragmentation using divalent cations at high temperatures in NEBNext First Strand Synthesis Reaction Buffer (5X). First-strand cDNA was prepared using a random hexamer primer and M-MuLV Reverse Transcriptase (RNaseH-), followed by the application of DNAPI and RNase H. These activities facilitated the conversion of the remaining overhangs into blunt ends. The NEBNext adaptor with hairpin loop structure was ligated to prepare for hybridization after adenylation of the 3′ ends of the DNA fragments. The Beckman Coulter AMPure XP system was used to obtain cDNA fragments of 150 ~ 200 bp in length. These fragments were treated with 3 μL of USER Enzyme at 37°C for 15 min, followed by 5 min at 95°C before PCR. Next, Phusion High-Fidelity DNA polymerase, Universal PCR primers, and Index (X) Primer were used for PCR analysis. Finally, the Agilent Bioanalyzer 2100 system was used to assess the quality of the library generated. The index-coded samples were clustered using a cBot Cluster Generation System for TruSeq PE Cluster Kit v3-cBot-HS (Illumina). An Illumina HiSeq PE150 platform was used to sequence the library at Novogene Bioinformatics Institute (Beijing, China), resulting in the generation of data on the 150 bp paired-end reads.
In-house Perl scripts were used to analyze the raw reads. Clean reads were obtained after filtering the raw data for low-quality reads/adaptors/polyNs, and at the same time, we obtained the Q20, Q30, and GC information. The STAR (v2.5.1b) software (32) was used to create a list of the reference genome, and the corresponding paired-end clean reads were aligned to the cattle reference genome (Bos_taurus_Ensembl_94). We used HTSeq v0.6.0 to assess the read counts mapped to each gene. The gene lengths and read counts aligned to the genes were calculated using FPKM. Normalization of the expression of lncRNAs/mRNAs removed the impact of sequencing depth, gene length, and sample difference on gene expression. The R package DESeq2 was used to identify DE-lncRNAs (DELs) and protein-coding genes (33) following a negative binomial distribution. Genes and lncRNAs with an adjusted P < 0.05 (P-adjust) were labeled as differentially expressed.
Cuffcompare software was used to screen the lncRNA transcripts. The assembled transcripts were filtered to determine probable lncRNAs to reduce the false-positive rate using the following steps: (1) New transcripts were identified by comparison with reference annotations; (2) Transcripts with exon numbers ≥ 2 and lengths > 200 nt were selected for the exon number screening and transcript length screening of the transcripts; (3) Transcripts were assessed for coding potential by integration using coded potential calculator 2 (CPC2) (34), Pfam (35), and Coding-Non-Coding-Index (CNCI) (36) to predict the coding potential and the intersection of the non-coding transcripts, allowing selection of the candidate lncRNA dataset; (4) The candidate novel lncRNAs were finally screened using the HUGO Gene Nomenclature Committee (HGNC) naming guidelines for long non-coding RNA (37) and named based on their positional relationships with coding genes.
The target genes for the lncRNAs in the RNA-seq results were predicted based on cis-and trans-regulation. We investigated the coding genes within 100 kb of the lncRNAs to determine their target genes (38). The trans role implied an expression-based association between the lncRNA and target genes. We determined the correlation between the lncRNAs and coding genes with custom scripts, and the genes with Pearson correlation coefficient > 0.95 were identified as the target genes of the lncRNAs (39).
The GOseq R package was used to perform Gene Ontology (GO) analysis of the target genes of differentially expressed mRNAs (DEMs)/lncRNA target genes, with corrected gene length bias (40). The KEGG database was used to evaluate the functional significance of the biological systems (cells, organisms, and the ecosystem) based on genome sequencing and other high-throughput experimental data. The cluster Profiler R package was used for KEGG pathway analysis of the DEMs/lncRNA target genes. A P < 0.05 indicated a significant relationship between the terms and the DE-lncRNA target genes.
Next, we obtained the differentially expressed lncRNAs (DELs) and their target genes from the cis and trans prediction results, respectively, to further explore the interactions between the DEMs and DELs. These were incorporated with the DEMs from the sequencing results. We screened the lipogenic-related mRNAs based on literature searches to improve the visibility of the results and finally constructed a DEL-DEM co-expression network using Cytoscape (v3.6.0) (41).
We randomly selected 12 significant DEMs and 7 DELs and analyzed their expression using qRT-PCR. The Evo-MLV kit was used to synthesize the RNA extracted from bovine adipocytes. First, we used Primer 5.0 to design specific primers for mRNAs and lncRNAs. Tables 2, 3 show the primers of DEMs and DELs used, respectively. The PCR products were analyzed using 1.5% AGE and then sequenced using the Sanger method. Then, we verified the mRNAs/lncRNAs from bovine adipocytes by comparing the amplified sequences of the PCR products with the sequences of the specific mRNAs and lncRNAs obtained from RNA-Seq using MEGA 5.0. Second, the Applied Biosystems QuantStudio®6 Flex was used to perform qRT-PCR using the TB Green® Premix Ex Taq™ II, and the bovine GAPDH gene as the internal reference. The 2−ΔΔCT method was used to calculate relative expression with GAPDH as the internal control (42). Also, the expression of mRNAs and lncRNAs from both the techniques were expressed as Log2 (fold change) for the si-treated group in comparison with the NC-treated group. Paired t-tests using GraphPad Prism v6 were used for data analysis. Data were expressed as the mean ± SE deviation of triplicate experiments. A P < 0.05 indicated a significant difference.
Next, the lncRNA levels were determined in various bovine tissues to further verify the role of lncRNA in the production of bovine UFAs. Briefly, we used TRIzol to isolate total RNA from the collected heart, spleen, lung, liver, kidney, leg muscle, and perinephric fat, followed by reverse transcription to generate cDNA. Finally, the expression of lncRNAs was determined using qRT-PCR. The 2−ΔΔCT method was used to determine the relative expression using GAPDH as the internal control. The paired t-test using GraphPad Prism v6 was done for data analysis. Data were expressed as the mean ± SE deviation of triplicate experiments.
The cultured F3 generation bovine pre-adipocytes were collected on differentiation days 0, 2, 4, 6, and 8, and qRT-PCR was performed to determine the relative expression of ACSL1. The rise in ACSL1 levels was followed by a reduction, with the maximum expression on day 4 (P < 0.01). Therefore, day-4 bovine adipocytes were used for subsequent experiments (13).
We transfected three pairs of siRNAs into the bovine adipocytes and determined their expression after 48 h to screen the most efficient siRNA fragments for silencing ACSL1. There was a >70% reduction in the mRNA expression of ACSL1, with si3-ACSL1 showed the highest efficiency of interference (86%; P < 0.01). Therefore, si3-ACSL1 was selected for subsequent experiments (13). Next, we transfected differentiation day 4 adipocytes with si3-ACSL1 (si-treated group) (13), followed by sequencing of both the si-treated cells and NC-treated cells.
We constructed six cDNA libraries for the NC-treated group (n = 3) and si-treated group (n = 3) to explore the regulation of UFA synthesis by mRNAs and lncRNAs. After sequencing, the raw reads were acquired using the Illumina HiSeq PE150 Platform and deposited in GenBank (accession numbers: SRR13358757-13358762). Next, we tested the correlation between the expression of different samples (Figure 1A). In total, we obtained 98,462,108–117,507,866 and 93,238,078–110,700, 276 raw reads from the libraries from the NC-treated group and si-treated group, respectively, which resulted in 96,857,744–115,542,046 and 91,106,662–109,206,440 clean reads after removing low-quality/adaptor sequences, resulting in ~15 GB data/sample. The GC contents were 51.54–56.29%. Q20 was >96%, and Q30 was >90% (Table 4). More than 87.76% of clean reads aligned to the reference genome, resulting in 173,090 assembled transcripts (Supplementary File 1). Our study identified 5,432 putative lncRNAs, which included 65.2% lincRNAs, 20.7% antisense lncRNAs, and 14.1% sense_overlapping lncRNAs (Figures 1B,C). At the transcriptional level, 15,547 mRNAs were obtained, of which 454 were novel mRNAs.
Figure 1. Sequencing data after manipulation of ACSL1 expression in bovine adipocytes. (A) Pearson correlation coefficients between samples. (B) LncRNA were screened using Cuffcompare software. (C) Classification of lncRNA.
We analyzed the gene structure and expression to determine the dissimilarities between the lncRNAs and mRNAs obtained by RNA-seq. The results showed that mRNAs were expressed at a relatively higher degree with the lncRNAs, based on the FPKM values (Figure 2A). The mRNAs (5,321 bp on average) were substantially longer than the lncRNAs (1,813 bp on average) (Figure 2B). The mRNAs had a higher number of exons (6.04 on average) than those of the lncRNAs (2.91 on average). Also, 61.23% of mRNAs had four or more exons, while 81.41% of lncRNAs had three or fewer exons (Figure 2C). Furthermore, most of the mRNAs (402 bp on average) had longer ORFs than the lncRNAs (147 bp on average) (Figure 2D).
Figure 2. Genomic expression of lncRNAs and mRNAs. (A) The expression of lncRNA and mRNA. (B–D) Comparison of length, exon count, and ORF length between lncRNAs and mRNAs. (E,F) Cluster heatmap of DEMs and DELs. The FPKM array was centered and scaled in the row direction by R package pheatmap (v1.0.8). Red indicates higher expression and blue represents lower expression. The log10 (FPKM+1) value was converted (scale number) and clustered. (G,H) Volcano plot of DELs and DEMs.
We detected 75 DELs and 107 DEMs in the si-treated group compared with the NC-treated group (P-adjusted <0.05). We performed differential mRNA cluster analysis to examine the clustering pattern of DEMs under varying experimental conditions. We found that the same group of DEMs was clustered together (Figure 2E), supporting the accuracy and reliability of the samples. Furthermore, we found that in the si-treated group, 31 lncRNAs were significantly upregulated, and 44 lncRNAs were significantly downregulated (P-adjusted <0.05). Figure 2F shows the clustering results. Similarly, we observed significantly upregulated expression of 47 mRNAs and downregulated expression of 60 mRNAs in the si-treated group (P-adjusted <0.05). The volcano plots (Figures 2G,H) and the Supplementary Files 2, 3 show the DEL and DEMs, respectively.
The results of the GO analysis revealed that the significant DEMs were classified into 386 functional groups (P < 0.05; Supplementary File 4). Of these, 64 terms were significantly enriched in molecular function (GO-MF), and metalloendopeptidase inhibitor activity (GO: 0008191; P = 0.0017) was the most significantly enriched GO term, followed by EP4 subtype prostaglandin E2 receptor binding (GO: 0031867; P = 0.0019) and oxidoreductase activity (GO: 0016671; P = 0.0034). For the cellular component (GO-CC), 33 terms were significantly enriched, with the extracellular region (GO: 0005576; P = 0.0001) the most significantly enriched, followed by the invadopodium membrane (GO: 0071438; P = 0.0004). For the biological processes (GO-BP), 289 GO terms were related to various processes or regulation, such as glycosaminoglycan metabolic process (GO: 0030203; P = 0.0009), aminoglycan metabolic process (GO: 0006022; P = 0.0014), and positive regulation of MAPK cascade (GO: 0043410; P = 0.0055). Figure 3A shows the top 50 functional GO annotations for the DEMs. The KEGG pathway analysis showed that the DEMs were involved in 116 signaling pathways (Supplementary File 5). Figure 3B shows the top 20 signaling pathways, and the PPAR signaling pathway showed the highest level of significance (P < 0.05), followed by the Rap1 signaling pathway and PI3K-Akt signaling pathway. Our results indicated that these pathways were probably involved in UFA synthesis.
Figure 3. GO and KEGG analysis of DEMs. (A) GO analysis of DEMs showing the relevant categories. (B) Top 20 of KEGG pathways of DEMs.
We identified 20,724 protein-coding genes as the nearest neighbors of 5,423 out of 5,432 lncRNAs using 100 kb as the cutoff (Supplementary File 6). Subsequent GO analysis revealed 435 significantly enriched GO terms (P < 0.05; Supplementary File 7). Figure 4A shows the top 50 GO functional annotations of the DEL target genes. The top five GO terms were embryonic skeletal system development, anterior/posterior pattern specification, sequence-specific DNA binding, regionalization, and embryonic skeletal system morphogenesis. The results of KEGG analysis indicated the involvement of seven pathways (P < 0.05; Supplementary File 8), and Figure 4B shows the top 20 KEGG pathways, such as MAPK signaling pathway, PPAR signaling pathway, Ribosome biogenesis in eukaryotes, and ether lipid metabolism.
Figure 4. GO and KEGG analyses of cis and trans target genes of DELs. (A) The GO analysis of top 50 cis target genes with the corresponding categories. (B) Top 20 of KEGG pathways analysis of cis target genes. (C) Top 50 GO analysis of trans target genes along with the categories of BP, CC, and MF. (D) The KEGG pathways of the top 20 trans target genes.
Next, we identified 5,432 lncRNAs and 698,719 genes to investigate the trans target genes of lncRNAs using the Pearson correlation ≥ 0.95 as the cutoff (Supplementary File 9). Figure 4C shows the top 50 GO functional annotations of the DEL target genes. GO analysis identified 1,154 significantly enriched GO terms (Supplementary File 10), and the top five GO terms were enzyme binding, kinase regulator activity, rRNA binding, G-protein coupled nucleotide receptor activity, and G-protein coupled purinergic nucleotide receptor activity. We also found that 274 KEGG pathways were involved in the trans-target genes for lncRNAs (Supplementary File 11), such as the Ribosome, Citrate cycle (TCA cycle), protein digestion and absorption, FA metabolism, and FA degradation (Figure 4D).
We obtained the DELs and their targets from the cis and trans prediction results, respectively, and incorporated them with the DEMs from the sequencing results (Supplementary File 12). Then, we screened lipogenic-related mRNAs based on literature searches and finally constructed a DEL-DEM co-expression network (Supplementary File 13). The DEL-DEM co-expression network involved 14 DELs and 22 trans-targets (Figure 5A), as well as 2 DELs and 2 cis-targets (Figure 5B). The co-expression network results showed that some lncRNAs interacted with multiple DEMs; for example, 14 DEMs co-expressed with TCONS_00069661, and 2 DEMs co-expressed with TCONS_00142457, TCONS_00040771, and TCONS_00074138, respectively, indicating that these lncRNAs and mRNAs belonged to the core non-coding/coding RNAs and had important regulatory effects on the synthesis of UFAs.
Figure 5. The DEL-DEM interaction network. (A) DEL and trans-target interaction network. The trans-target was a DEM as well. (B) DEL and cis-target interaction network. The cis-target was a DEM as well. The circle type and inverted triangles type represent a lncRNA and mRNA, respectively. Red represents upregulation, and the green represents downregulation.
We randomly chose 12 and 7 genes from DEMs and DELs, respectively, to analyze the validity of the RNA-seq data by qRT-PCR. The results were comparable to the results of mRNA and lncRNA expression derived from the RNA-seq data (Figures 6A,B). Furthermore, the sequence of the qRT-PCR-amplified fragments of the specific primers were consistent with the sequences obtained by RNA-seq (Supplementary File 14).
Figure 6. qRT-PCR of DEMs and DELs. (A) Changes in mRNA expression between the NC and si-treated groups. (B) qRT-PCR verification of changes in lncRNA expression between the NC and si-treated groups. (C) qRT-PCR analysis of DEL TCONS-00069661 in heart, liver, spleen, lung, kidney, leg muscles, and perirenal fat. Three biological and technical replicates were used for each group. The qRT-PCR data were determined using the 2−ΔΔCt method with GAPDH as the internal reference. The verification data of mRNA and lncRNA sequencing results were further normalized to log2 (foldchange). Data represent means ± standard error.
We used qRT-PCR to study the expression of lncRNA TCONS_00069661, located at the center of the co-expression network and expressed in the kidney, spleen, heart, liver, lung, leg muscle, and perirenal fat of cattle. We found that TCONS_00069661 was highly expressed in the adipose tissue of cattle (Figure 6C). Moreover, our previous studies showed that ACSL1 gene was also highly expressed in bovine adipose tissue (12). Thus, these results substantiated the reliability of the co-expression network, and suggested the regulatory roles played by DELs and DEMs in UFA synthesis.
In recent years, transcriptome sequencing has been frequently used for gene expression analysis and to reveal biological characteristics. Here, we investigated the expression of ACSL1, a key gene involved in the production of UFAs in adipocytes, to explore the molecular mechanism of UFAs synthesis. After different manipulations of ACSL1, we performed strand-specific RNA sequencing to identify mRNAs and lncRNAs in bovine adipocytes systematically.
In this study, 15,547 mRNAs and 5,432 lncRNA transcripts were identified in the NC-treated group and si-treated group, of which 107 mRNAs and 75 lncRNA transcripts were differentially expressed, respectively. The qRT-PCR results confirmed the strong correlation between lncRNA and mRNA expression and the transcriptome data. Compared with other transcriptomic studies in beef cattle, fewer mRNAs and lncRNAs were identified in this study. We speculated that it was probably because our study focused specifically on bovine adipocytes. Most of the current research on beef cattle has used specific tissues for sequencing; however, the results were not sufficiently targeted (24, 43). Fewer exons were tested within the 5,432 lncRNAs identified here, which had a lower expression and shorter lengths compared to those of mRNAs. Our results are consistent with the results of other recent studies in other mammals (24, 28, 44). These results on lncRNA expression suggest conservative regulation in mammals.
Next, GO and KEGG analyses were used for determining the biological functions of the mRNAs identified by our sequencing data. The GO analysis classified the significantly enriched DEMs into 386 functional groups (including 289 BPs (P < 0.05), 33 CCs, and 64 MFs). Some GO terms were closely related to the production of UFAs and fats, such as positive regulation of MAPK cascade (GO: 0043410; P = 0.00545), adiponectin-mediated signaling pathway (GO: 0033211; P = 0.025288), long-chain FA transport (GO: 0015909; P = 0.030592), and positive regulation of PPAR signaling pathway (GO: 0035360; P = 0.031822).
MAPK signaling pathways have been shown to control various biological processes via various cellular mechanisms and might involve activation/inhibition of the molecules involved (45). Wu et al. identified C1qTNF-related factor as one of the adipokines Protein 6 (CTRP6), which promoted the differentiation of subcutaneous fat in pigs through the MAPK signaling pathway (46). Adiponectin (APN) is a hormone protein that is specifically secreted from human and animal adipose tissue and plays an important role in adipogenesis (47, 48). Only after specific binding of adiponectin to its receptor, can it activate the intracellular signaling cascade to exert its biological effects (49). Additionally, ADIPOQ is also known to play a critical role in adipocyte development, which affects the composition of UFAs and lipid production (50). The GO analysis also identified various candidate genes, several of which were demonstrated to be related to UFA synthesis, such as ACSL1, FABP4, and PTGIS. Additionally, the positive regulation of the MAPK cascade identified eight background genes, including BMPER, FGFR2, and CSPG4. Although they are not marker genes of UFA synthesis, the regulatory effect of MAPK on adipogenesis indicated that they were probably potential candidates for UFA synthesis.
The results of the KEGG pathway analysis showed that the DEMs were substantially enriched in 13 pathways (P < 0.05). Some DEMs were considerably enriched in the PPAR signaling pathway, PI3K-Akt signaling pathway, Rap1 signaling pathway, Ras signaling pathway, and ECM-receptor interaction. The PPAR signaling pathway is a crucial pathway closely related to FA and sterol metabolism as well as adipogenic differentiation (51). The PPAR family involves three subtypes PPAR-α/-β/-δ/-γ, with variable roles (52). PPARα activates FA oxidation; PLIN1, PLIN3, LIPE, and FABP4 are the known targets of PPAR (53). FA-binding protein 4 (FABP4) has the ability to predict marbling (54). PPARβ/δ participates in adipogenic differentiation and adipogenesis (52). In this study, the PPAR signaling pathway was significantly enriched with 6 DEMs: ACSL1, CPT1A, FABP4, GK2, OLR1, and PTGIS. These genes were all known to be essential genes for lipid production (1, 55).
The PI3K-Akt signaling pathway is a classic insulin-related signaling pathway (56). PI3Ks are involved in the phosphorylation of proteins and the PI3K-Akt pathway might also be involved in adipogenesis (57–59). The insulin-mediated PI3K/AKT signaling pathway plays a vital role within adipocytes of obese patients and leads to an excess of lipids that has to be appropriately stored in the fat tissue (57). In the present study, ANGPT1, COL4A5, EFNA5, FGF13, FGFR2, ITGA3, ITGA4, KITLG, and XLOC_000377 were all considerably enriched in the PI3K-Akt signaling pathway, suggesting that differential expression of these genes might induce the FA-related metabolism pathway in beef cattle.
Rap1, a small G protein, is known to be involved in regulating cell survival/proliferation via the regulation of the PI3-AKT pathway (60). Studies have shown that Ras proteins act as molecular switches to play various regulatory functions in cell growth and survival (61). The Ras signaling pathway has been shown to activate the MAPK cascade; also, the PI3K-Akt pathway is known to regulate cell growth and apoptosis (60). We obtained a total of 14 DEMs from the Rap1 and Ras signaling pathways, which were observed to play vital roles in UFA synthesis. The ECM is essential for tissue architecture and has an important role in adipogenesis (62). ECM is substantially enriched in bovine adipose tissue and may regulate specific genes to participate in adipogenesis (63). In this study, the ECM-receptor interaction was substantially enriched in 3 DEMs, including ITGA3, ITGA4, and COL4A5. These three genes were more closely related to diseases (64–66), but studies have found that ITGA3, ITGA4, and COL4A1 may be involved in the composition of adipocyte extracellular matrix and the differentiation of adipocytes (67).
For a more in-depth understanding of the DELs potential function, we performed GO and KEGG analyses of its cis and trans target genes. In this study, we identified 75 DELs and predicted their target genes, which were primarily enriched in the GO terms associated with the regulation of FA biosynthesis and metabolism (P < 0.05), such as glycerol-3-phosphate biosynthetic process (GO: 0046167), negative regulation of plasma membrane long-chain FA transport (GO: 0010748), FA catabolic process (GO: 0009062), lipid oxidation (GO: 0034440), and FA oxidation (GO: 0019395). These findings suggested that the DELs' target genes are involved in functions from the synthesis to metabolism of FAs, indicating the influence the DELs may have on the synthesis and metabolism of UFAs and lipid. Similarly, some lncRNAs are known to play important roles in lipid synthesis in cattle (25, 31, 68). The KEGG analysis results of the target genes of DELs also showed significant involvement (P < 0.05) of pathways related to UFA synthesis, such as the PPAR signaling pathway, MAPK signaling pathway, Ether lipid metabolism, and Citrate cycle (TCA cycle). The TCA cycle involves various critical metabolic steps, and ether lipid metabolism is another vital FA metabolism-related pathway (69, 70).
To improve the screening out of lncRNAs and mRNAs that may be related to UFA synthesis more intuitively and in-depth, we constructed a DEL-DEM co-expression network. The DEL-DEM co-expression network involved 14 DELs and 22 trans-targets, as well as two DELs and two cis-targets to demonstrate their potential in UFAs production. In the results of the co-expression network, lncRNA TCONS_00069661 was of particular interest, as it was predicted to simultaneously target 14 DEMs including ACSL1, such as FABP4, OLR1, and COL4A5. We found that FABP4 was a downregulated DEM, which not only regulated the differentiation of bovine adipocytes in the PPAR signaling pathway and supported the transport of extracellular FAs but was also involved in lipolysis and FA transport in different tissues (71, 72). A recent study on the FABP4 gene of Yanbian cattle showed that a SNP in FABP4 substantially affected the fat content, protein content, and marbling level (73). Li et al. (74) found that with increasing age, the concentration of SFA in Yanbian cattle decreased, and the concentration of MUFA increased proportionally with an increase in the percentage of intramuscular lipids leading, in turn, to an increase in the expression of the FABP4 gene. A SNP (g.8232C > A) in the oxidized low-density lipoprotein receptor 1 (OLR1) has been shown to be related to rump fat thickness and weaning weight in Nelore cattle (75–78), indicating its importance in regulating fat deposition. In our study, the upregulated lncRNA TCONS_00069661 was predicted to target the downregulated gene OLR1. We speculate that TCONS_00069661 targets the 3'-UTR of OLR1, thereby preventing its translation. Current research on COL4A5 has been related to disease, and some of the genes in this family have been studied in cattle. Previous studies have confirmed the role of COL5A3, COL6A2, and COL3A1 in inducing CD44 expression, followed by upregulation of the cellular expression of docosanoic acid, palmitic acid, and trans-oleic acid and downregulation of the cellular expression of tridecanoic acid, stearic acid, and cis-5, 8, 11, 14-EPA (79). Liu et al. (26) found that COL6A1, COL6A2, COL4A2, COL1A1, COL4A6, COL4A5, COL6A3, COL1A2, and other genes were strongly related to fat metabolism in the transcriptomic study of the muscles of Shandong black cattle and Luxi cattle.
Additionally, our co-expression network results showed that not only did one lncRNA target multiple mRNAs but multiple lncRNAs targeted one mRNA. For example, TCONS_00040771, TCONS_00035606, and TCONS_00048301 simultaneously targeted SLC26A7, and TCONS_00130715 and TCONS_00122946 simultaneously targeted GK2. However, our results of the co-expression network diagram showed dissimilar upward and downward trends of lncRNAs and mRNAs. Thus, we speculate that there is a direct relation between some lncRNAs and mRNAs (21, 31), with some combining with miRNAs to perform the function of ceRNA, leading to the degradation of mRNA targets or translational repression, and the mode of action involved lncRNA-miRNA-mRNA network (80, 81). Further studies should involve the verification of the lncRNA-mRNA targeting relationship pairs that might exist in subsequent studies to explore their mode of action and specific roles in the synthesis of UFAs.
This study used RNA-seq to determine lncRNA and mRNA levels by interference and comparing ACSL1 expression with controls in bovine adipocytes. GO and KEGG analyses showed that the target genes of the DELs and DE-genes were enriched in the relevant FA and lipogenesis-related pathways. Based on the above results, we constructed a DEL-DEM interaction network. The results of this study expand our knowledge of the molecular mechanisms used by lncRNAs as well as the genes involved in the regulation of UFA synthesis in bovine adipocytes.
The datasets presented in this study can be found in online repositories. The names of the repository/repositories and accession number(s) can be found in the article/Supplementary Material.
The animal study was reviewed and approved by Ethical Commission of Gansu Agricultural University as well as the Ministry of Science and Technology of the People's Republic of China.
YB, HT, and ZZ: conceptualization. YB, HT, BS, and ZZ: methodology. YB, XLi, JL, YM, and ZC: validation. YB, BS, XLiu, and SL: formal analysis. ZZ, HT, and YB: investigation. YL, JH, and JW: resources. YB: writing—original draft preparation. YB, ZZ, and SR: writing—review and editing. ZZ and XH: supervision. ZZ: project administration. ZZ and JH: funding acquisition. All authors have read and agreed to the published version of the manuscript.
This research was supported by the National Natural Science Foundation (31860631), Young Doctor Fund Project of Gansu Provincial Department of Education (2021QB-027), and Scientific research start-up funds for openly-recruited doctors (GSAU-RCZX201711).
The authors declare that the research was conducted in the absence of any commercial or financial relationships that could be construed as a potential conflict of interest.
All claims expressed in this article are solely those of the authors and do not necessarily represent those of their affiliated organizations, or those of the publisher, the editors and the reviewers. Any product that may be evaluated in this article, or claim that may be made by its manufacturer, is not guaranteed or endorsed by the publisher.
We thank LetPub (www.letpub.com) for its linguistic assistance during the preparation of this manuscript.
The Supplementary Material for this article can be found online at: https://www.frontiersin.org/articles/10.3389/fvets.2021.788316/full#supplementary-material
Supplementary File 1. The sequencing results were compared to the reference genome.
Supplementary Files 2, 3. Details of DELs and DEMs.
Supplementary File 4. Details of GO analysis of DEMs.
Supplementary File 5. Details of KEGG pathway analysis of DEMs.
Supplementary File 6. Cis-regulation of the lncRNAs target genes.
Supplementary File 7. GO analysis of DELs cis-regulated target genes.
Supplementary File 8. KEGG pathway analysis of DELs cis-regulated target genes.
Supplementary File 9. Trans-regulation of the lncRNAs target genes.
Supplementary File 10. GO analysis of DELs trans-regulated target genes.
Supplementary File 11. KEGG pathway analysis of DELs trans-regulated target genes.
Supplementary File 12. The cis-regulated target genes and the trans-regulated target genes of DELs intersect with DEMs, respectively.
Supplementary File 13. Literature screening information on lipogenesis-related mRNAs.
Supplementary File 14. The result of the alignment between the sequence of the amplified fragments of qRT-PCR and the sequence in RNA-Seq.
1. Coleman RA, Lewin TM, Muoio DM. Physiological and nutritional regulation of enzymes of triacylglycerol synthesis. Annu Rev Nutr. (2000) 20:77–103. doi: 10.1146/annurev.nutr.20.1.77
2. Soupene E, Kuypers FA. Mammalian long-chain acyl-coa synthetases. Exp Biol Med. (2008) 233:507–21. doi: 10.3181/0710-MR-287
3. Zhan T, Poppelreuther M, Ehehalt R, Fullekrug J. Overexpressed fatp1, acsvl4/fatp4 and acsl1 increase the cellular fatty acid uptake of 3t3-l1 adipocytes but are localized on intracellular membranes. PLoS ONE. (2012) 7:e45087. doi: 10.1371/journal.pone.0045087
4. Ellis JM, Li LO, Wu PC, Koves TR, Ilkayeva O, Stevens RD, et al. Adipose acyl-coa synthetase-1 directs fatty acids toward beta-oxidation and is required for cold thermogenesis. Cell Metab. (2010) 12:53–64. doi: 10.1016/j.cmet.2010.05.012
5. Lopez-Huertas E. Health effects of oleic acid and long chain omega-3 fatty acids (epa and dha) enriched milks. A review of intervention studies. Pharmacol Res. (2010) 61:200–7. doi: 10.1016/j.phrs.2009.10.007
6. Russo GL. Dietary n-6 and n-3 polyunsaturated fatty acids: from biochemistry to clinical implications in cardiovascular prevention. Biochem Pharmacol. (2009) 77:937–46. doi: 10.1016/j.bcp.2008.10.020
7. Simopoulos AP. Omega-3 fatty acids in health and disease and in growth and development. Am J Clin Nutr. (1991) 54:438–63. doi: 10.1093/ajcn/54.3.438
8. Barcelo-Coblijn G, Murphy EJ. Alpha-linolenic acid and its conversion to longer chain n-3 fatty acids: benefits for human health and a role in maintaining tissue n-3 fatty acid levels. Prog Lipid Res. (2009) 48:355–74. doi: 10.1016/j.plipres.2009.07.002
9. Widmann P, Nuernberg K, Kuehn C, Weikard R. Association of an acsl1 gene variant with polyunsaturated fatty acids in bovine skeletal muscle. BMC Genet. (2011) 12:96. doi: 10.1186/1471-2156-12-96
10. Li LO, Mashek DG, An J, Doughman SD, Newgard CB, Coleman RA. Overexpression of rat long chain acyl-coa synthetase 1 alters fatty acid metabolism in rat primary hepatocytes. J Biol Chem. (2006) 281:37246–55. doi: 10.1074/jbc.M604427200
11. Cao Y, Wang S, Liu S, Wang Y, Jin H, Ma H, et al. Effects of long-chain fatty acyl-coa synthetase 1 on diglyceride synthesis and arachidonic acid metabolism in sheep adipocytes. Int J Mol Sci. (2020) 21:2044. doi: 10.3390/ijms21062044
12. Zhao ZD, Zan LS, Li AN, Cheng G, Li SJ, Zhang YR, et al. Characterization of the promoter region of the bovine long-chain acyl-coa synthetase 1 gene: roles of e2f1, sp1, klf15, and e2f4. Sci Rep. (2016) 6:19661. doi: 10.1038/srep19661
13. Tian HS, Su XT, Han XM, Zan LS, Luo YZ, Wang JQ, et al. Effects of silencing ACSL1 gene by siRNA on the synthesis of unsaturated fatty acids in adipocytes of qinchuan beef cattle. J Agric Biotechnol. (2020) 10:1722–32. doi: 10.3969/j.issn.1674-7968.2020.10.002
14. Zhao Z, Abbas RS, Tian H, Shi B, Luo Y, Wang J, et al. Effects of overexpression of acsl1 gene on the synthesis of unsaturated fatty acids in adipocytes of bovine. Arch Biochem Biophys. (2020) 695:108648. doi: 10.1016/j.abb.2020.108648
15. Anderson DM, Anderson KM, Chang CL, Makarewich CA, Nelson BR, McAnally JR, et al. A micropeptide encoded by a putative long noncoding rna regulates muscle performance. Cell. (2015) 160:595–606. doi: 10.1016/j.cell.2015.01.009
16. Bertani S, Sauer S, Bolotin E, Sauer F. The noncoding rna mistral activates hoxa6 and hoxa7 expression and stem cell differentiation by recruiting mll1 to chromatin. Mol Cell. (2011) 43:1040–6. doi: 10.1016/j.molcel.2011.08.019
17. Zhao J, Sun BK, Erwin JA, Song JJ, Lee JT. Polycomb proteins targeted by a short repeat rna to the mouse x chromosome. Science. (2008) 322:750–6. doi: 10.1126/science.1163045
18. Sheik MJ, Gaughwin PM, Lim B, Robson P, Lipovich L. Conserved long noncoding rnas transcriptionally regulated by oct4 and nanog modulate pluripotency in mouse embryonic stem cells. RNA. (2010) 16:324–37. doi: 10.1261/rna.1441510
19. Xiao T, Liu L, Li H, Sun Y, Luo H, Li T, et al. Long noncoding rna adinr regulates adipogenesis by transcriptionally activating c/ebpalpha. Stem Cell Rep. (2021) 16:1006–8. doi: 10.1016/j.stemcr.2021.03.024
20. Cai R, Tang G, Zhang Q, Yong W, Zhang W, Xiao J, et al. A novel lnc-rna, named lnc-ora, is identified by rna-seq analysis, and its knockdown inhibits adipogenesis by regulating the pi3k/akt/mtor signaling pathway. Cells. (2019) 8:477. doi: 10.3390/cells8050477
21. Li H, Yang J, Jiang R, Wei X, Song C, Huang Y, et al. Long non-coding rna profiling reveals an abundant mdncr that promotes differentiation of myoblasts by sponging mir-133a. Mol Ther Nucleic Acids. (2018) 12:610–25. doi: 10.1016/j.omtn.2018.07.003
22. Mortazavi A, Williams BA, McCue K, Schaeffer L, Wold B. Mapping and quantifying mammalian transcriptomes by rna-seq. Nat Methods. (2008) 5:621–8. doi: 10.1038/nmeth.1226
23. Miao X, Qin QL. Genome-wide transcriptome analysis of mrnas and micrornas in dorset and small tail han sheep to explore the regulation of fecundity. Mol Cell Endocrinol. (2015) 402:32–42. doi: 10.1016/j.mce.2014.12.023
24. Gao Y, Li S, Lai Z, Zhou Z, Wu F, Huang Y, et al. Analysis of long non-coding rna and mrna expression profiling in immature and mature bovine (bos taurus) testes. Front Genet. (2019) 10:646. doi: 10.3389/fgene.2019.00646
25. Jiang R, Li H, Huang Y, Lan X, Lei C, Chen H. Transcriptome profiling of lncrna related to fat tissues of qinchuan cattle. Gene. (2020) 742:144587. doi: 10.1016/j.gene.2020.144587
26. Liu R, Liu X, Bai X, Xiao C, Dong Y. Different expression of lipid metabolism-related genes in shandong black cattle and luxi cattle based on transcriptome analysis. Sci Rep. (2020) 10:21915. doi: 10.1038/s41598-020-79086-4
27. Song C, Huang Y, Yang Z, Ma Y, Chaogetu B, Zhuoma Z, et al. Rna-seq analysis identifies differentially expressed genes insubcutaneous adipose tissuein qaidamford cattle, cattle-yak, angus cattle. Animals. (2019) 9:1077. doi: 10.3390/ani9121077
28. Ma L, Zhang M, Jin Y, Erdenee S, Hu L, Chen H, et al. Comparative transcriptome profiling of mrna and lncrna related to tail adipose tissues of sheep. Front Genet. (2018) 9:365. doi: 10.3389/fgene.2018.00365
29. Xing K, Zhao X, Ao H, Chen S, Yang T, Tan Z, et al. Transcriptome analysis of mirna and mrna in the livers of pigs with highly diverged backfat thickness. Sci Rep. (2019) 9:16740. doi: 10.1038/s41598-019-53377-x
30. Xing S, Liu R, Zhao G, Liu L, Groenen M, Madsen O, et al. Rna-seq analysis reveals hub genes involved in chicken intramuscular fat and abdominal fat deposition during development. Front Genet. (2020) 11:1009. doi: 10.3389/fgene.2020.01009
31. Cai H, Li M, Jian W, Song C, Huang Y, Lan X, et al. A novel lncrna badlncr1 inhibits bovine adipogenesis by repressing glrx5 expression. J Cell Mol Med. (2020) 24:7175–86. doi: 10.1111/jcmm.15181
32. Dobin A, Davis CA, Schlesinger F, Drenkow J, Zaleski C, Jha S, et al. Star: ultrafast universal rna-seq aligner. Bioinformatics. (2013) 29:15–21. doi: 10.1093/bioinformatics/bts635
33. Anders S, Huber W. Differential expression analysis for sequence count data. Genome Biol. (2010) 11:R106. doi: 10.1186/gb-2010-11-10-r106
34. Kang YJ, Yang DC, Kong L, Hou M, Meng YQ, Wei L, et al. Cpc2: a fast and accurate coding potential calculator based on sequence intrinsic features. Nucleic Acids Res. (2017) 45:W12–6. doi: 10.1093/nar/gkx428
35. Finn RD, Bateman A, Clements J, Coggill P, Eberhardt RY, Eddy SR, et al. Pfam: the protein families database. Nucleic Acids Res. (2014) 42:D222–30. doi: 10.1093/nar/gkt1223
36. Sun L, Luo H, Bu D, Zhao G, Yu K, Zhang C, et al. Utilizing sequence intrinsic composition to classify protein-coding and long non-coding transcripts. Nucleic Acids Res. (2013) 41:e166. doi: 10.1093/nar/gkt646
37. Wright MW. A short guide to long non-coding rna gene nomenclature. Hum Genomics. (2014) 8:7. doi: 10.1186/1479-7364-8-7
38. Ghanbarian AT, Hurst LD. Neighboring genes show correlated evolution in gene expression. Mol Biol Evol. (2015) 32:1748–66. doi: 10.1093/molbev/msv053
39. Liao Q, Liu C, Yuan X, Kang S, Miao R, Xiao H, et al. Large-scale prediction of long non-coding rna functions in a coding-non-coding gene co-expression network. Nucleic Acids Res. (2011) 39:3864–78. doi: 10.1093/nar/gkq1348
40. Young MD, Wakefield MJ, Smyth GK, Oshlack A. Gene ontology analysis for rna-seq: accounting for selection bias. Genome Biol. (2010) 11:R14. doi: 10.1186/gb-2010-11-2-r14
41. Shannon P, Markiel A, Ozier O, Baliga NS, Wang JT, Ramage D, et al. Cytoscape: a software environment for integrated models of biomolecular interaction networks. Genome Res. (2003) 13:2498–504. doi: 10.1101/gr.1239303
42. Livak KJ, Schmittgen TD. Analysis of relative gene expression data using real-time quantitative pcr and the 2(-delta delta c(t)) method. Methods. (2001) 25:402–8. doi: 10.1006/meth.2001.1262
43. Li H, Wei X, Yang J, Dong D, Huang Y, Lan X, et al. Developmental transcriptome profiling of bovine muscle tissue reveals an abundant gosb that regulates myoblast proliferation and apoptosis. Oncotarget. (2017) 8:32083–100. doi: 10.18632/oncotarget.16644
44. Huang W, Zhang X, Li A, Xie L, Miao X. Differential regulation of mrnas and lncrnas related to lipid metabolism in two pig breeds. Oncotarget. (2017) 8:87539–53. doi: 10.18632/oncotarget.20978
45. Yue J, Lopez JM. Understanding mapk signaling pathways in apoptosis. Int J Mol Sci. (2020) 21:2346. doi: 10.3390/ijms21072346
46. Wu W, Zhang J, Zhao C, Sun Y, Pang W, Yang G. Ctrp6 regulates porcine adipocyte proliferation and differentiation by the adipor1/mapk signaling pathway. J Agric Food Chem. (2017) 65:5512–22. doi: 10.1021/acs.jafc.7b00594
47. Ghadge AA, Khaire AA, Kuvalekar AA. Adiponectin: a potential therapeutic target for metabolic syndrome. Cytokine Growth Factor Rev. (2018) 39:151–8. doi: 10.1016/j.cytogfr.2018.01.004
48. Katsiki N, Mantzoros C, Mikhailidis DP. Adiponectin, lipids and atherosclerosis. Curr Opin Lipidol. (2017) 28:347–54. doi: 10.1097/MOL.0000000000000431
49. Deepa SS, Zhou L, Ryu J, Wang C, Mao X, Li C, et al. Appl1 mediates adiponectin-induced lkb1 cytosolic localization through the pp2a-pkczeta signaling pathway. Mol Endocrinol. (2011) 25:1773–85. doi: 10.1210/me.2011-0082
50. Gao Y, Li F, Zhang Y, Dai L, Jiang H, Liu H, et al. Silencing of adipoq efficiently suppresses preadipocyte differentiation in porcine. Cell Physiol Biochem. (2013) 31:452–61. doi: 10.1159/000343381
51. Farmer SR. Regulation of ppargamma activity during adipogenesis. Int J Obes. (2005) 29 (Suppl. 1):S13–6. doi: 10.1038/sj.ijo.0802907
52. Khan WS, Adesida AB, Tew SR, Longo UG, Hardingham TE. Fat pad-derived mesenchymal stem cells as a potential source for cell-based adipose tissue repair strategies. Cell Prolif. (2012) 45:111–20. doi: 10.1111/j.1365-2184.2011.00804.x
53. Gaudel C, Schwartz C, Giordano C, Abumrad NA, Grimaldi PA. Pharmacological activation of pparbeta promotes rapid and calcineurin-dependent fiber remodeling and angiogenesis in mouse skeletal muscle. Am J Physiol Endocrinol Metab. (2008) 295:E297–304. doi: 10.1152/ajpendo.00581.2007
54. Cai G, Zhang X, Weng W, Shi G, Xue S, Zhang B. Associations between pparg polymorphisms and the risk of essential hypertension. PLoS ONE. (2017) 12:e181644. doi: 10.1371/journal.pone.0181644
55. Chmurzynska A. The multigene family of fatty acid-binding proteins (fabps): function, structure and polymorphism. J Appl Genet. (2006) 47:39–48. doi: 10.1007/BF03194597
56. Pessin JE, Saltiel AR. Signaling pathways in insulin action: molecular targets of insulin resistance. J Clin Invest. (2000) 106:165–9. doi: 10.1172/JCI10582
57. Sharma BR, Kim HJ, Rhyu DY. Caulerpa lentillifera extract ameliorates insulin resistance and regulates glucose metabolism in c57bl/ksj-db/db mice via pi3k/akt signaling pathway in myocytes. J Transl Med. (2015) 13:62. doi: 10.1186/s12967-015-0412-5
58. Kim SP, Ha JM, Yun SJ, Kim EK, Chung SW, Hong KW, et al. Transcriptional activation of peroxisome proliferator-activated receptor-gamma requires activation of both protein kinase a and akt during adipocyte differentiation. Biochem Biophys Res Commun. (2010) 399:55–9. doi: 10.1016/j.bbrc.2010.07.038
59. Yun J, Jin H, Cao Y, Zhang L, Zhao Y, Jin X, et al. Rna-seq analysis reveals a positive role of htr2a in adipogenesis in yan yellow cattle. Int J Mol Sci. (2018) 19:1760. doi: 10.3390/ijms19061760
60. Yang C, Zhang J, Wu T, Zhao K, Wu X, Shi J, et al. Multi-omics analysis to examine gene expression and metabolites from multisite adipose-derived mesenchymal stem cells. Front Genet. (2021) 12:627347. doi: 10.3389/fgene.2021.627347
61. Goodsell DS. The molecular perspective: the ras oncogene. Oncologist. (1999) 4:263–264 doi: 10.1634/theoncologist.4-3-263
62. Mariman EC, Wang P. Adipocyte extracellular matrix composition, dynamics and role in obesity. Cell Mol Life Sci. (2010) 67:1277–92. doi: 10.1007/s00018-010-0263-4
63. Lee HJ, Jang M, Kim H, Kwak W, Park W, Hwang JY, et al. Comparative transcriptome analysis of adipose tissues reveals that ecm-receptor interaction is involved in the depot-specific adipogenesis in cattle. PLoS ONE. (2013) 8:e66267. doi: 10.1371/journal.pone.0066267
64. Attia HR, Ibrahim MH, El-Aziz S, Hassan NM, Osman RA, Hagag HA, et al. Itga4 gene methylation status in chronic lymphocytic leukemia. Future Sci OA. (2020) 6:O583. doi: 10.2144/fsoa-2020-0034
65. Naylor RW, Morais M, Lennon R. Complexities of the glomerular basement membrane. Nat Rev Nephrol. (2021) 17:112–27. doi: 10.1038/s41581-020-0329-y
66. Tian L, Chen M, He Q, Yan Q, Zhai C. Microrna199a5p suppresses cell proliferation, migration and invasion by targeting itga3 in colorectal cancer. Mol Med Rep. (2020) 22:2307–17. doi: 10.3892/mmr.2020.11323
67. Ullah M, Sittinger M, Ringe J. Extracellular matrix of adipogenically differentiated mesenchymal stem cells reveals a network of collagen filaments, mostly interwoven by hexagonal structural units. Matrix Biol. (2013) 32:452–65. doi: 10.1016/j.matbio.2013.07.001
68. Li M, Sun X, Cai H, Sun Y, Plath M, Li C, et al. Long non-coding rna adncr suppresses adipogenic differentiation by targeting mir-204. Biochim Biophys Acta. (2016) 1859:871–82. doi: 10.1016/j.bbagrm.2016.05.003
69. Huang Y, Zhang C, Wang Y, Sun X. Identification and analysis of mirnas in the normal and fatty liver from the holstein dairy cow. Anim Biotechnol. (2020) 24:1–12. doi: 10.1080/10495398.2020.1804919
70. Salleh MS, Mazzoni G, Hoglund JK, Olijhoek DW, Lund P, Lovendahl P, et al. Rna-seq transcriptomics and pathway analyses reveal potential regulatory genes and molecular mechanisms in high- and low-residual feed intake in nordic dairy cattle. BMC Genomics. (2017) 18:258. doi: 10.1186/s12864-017-3622-9
71. Goszczynski DE, Papaleo-Mazzucco J, Ripoli MV, Villarreal EL, Rogberg-Munoz A, Mezzadra CA, et al. Genetic variation in fabp4 and evaluation of its effects on beef cattle fat content. Anim Biotechnol. (2017) 28:211–19. doi: 10.1080/10495398.2016.1262868
72. Guo B, Kongsuwan K, Greenwood PL, Zhou G, Zhang W, Dalrymple BP. A gene expression estimator of intramuscular fat percentage for use in both cattle and sheep. J Anim Sci Biotechnol. (2014) 5:35. doi: 10.1186/2049-1891-5-35
73. Yin BZ, Fang JC, Zhang JS, Zhang LM, Xu C, Xu HY, et al. Correlations between single nucleotide polymorphisms in fabp4 and meat quality and lipid metabolism gene expression in yanbian yellow cattle. PLoS ONE. (2020) 15:e234328. doi: 10.1371/journal.pone.0234328
74. Li XZ, Yan CG, Gao QS, Yan Y, Choi SH, Smith SB. Adipogenic/lipogenic gene expression and fatty acid composition in chuck, loin, and round muscles in response to grain feeding of yanbian yellow cattle. J Anim Sci. (2018) 96:2698–709. doi: 10.1093/jas/sky161
75. Chen KC, Hsieh IC, Hsi E, Wang YS, Dai CY, Chou WW, et al. Negative feedback regulation between microrna let-7g and the oxldl receptor lox-1. J Cell Sci. (2011) 124:4115–24. doi: 10.1242/jcs.092767
76. Fonseca PD, de Souza FR, de Camargo GM, Gil FM, Cardoso DF, Zetouni L, et al. Association of adipoq, olr1 and ppargc1a gene polymorphisms with growth and carcass traits in nelore cattle. Meta Gene. (2015) 4:1–7. doi: 10.1016/j.mgene.2015.02.001
77. Kaneda M, Lin BZ, Sasazaki S, Oyama K, Mannen H. Allele frequencies of gene polymorphisms related to economic traits in bos taurus and bos indicus cattle breeds. Anim Sci J. (2011) 82:717–21. doi: 10.1111/j.1740-0929.2011.00910.x
78. Kataoka H, Kume N, Miyamoto S, Minami M, Morimoto M, Hayashida K, et al. Oxidized ldl modulates bax/bcl-2 through the lectinlike ox-ldl receptor-1 in vascular smooth muscle cells. Arterioscler Thromb Vasc Biol. (2001) 21:955–60. doi: 10.1161/01.ATV.21.6.955
79. Hu ZL, Park CA, Reecy JM. Developmental progress and current status of the animal qtldb. Nucleic Acids Res. (2016) 44:D827–33. doi: 10.1093/nar/gkv1233
80. Yue B, Li H, Liu M, Wu J, Li M, Lei C, et al. Characterization of lncrna-mirna-mrna network to reveal potential functional cernas in bovine skeletal muscle. Front Genet. (2019) 10:91. doi: 10.3389/fgene.2019.00091
Keywords: lncRNA, ACSL1, bovine adipocytes, unsaturated fatty acids, RNA-Seq
Citation: Bai Y, Li X, Chen Z, Li J, Tian H, Ma Y, Raza SHA, Shi B, Han X, Luo Y, Hu J, Wang J, Liu X, Li S and Zhao Z (2021) Interference With ACSL1 Gene in Bovine Adipocytes: Transcriptome Profiling of mRNA and lncRNA Related to Unsaturated Fatty Acid Synthesis. Front. Vet. Sci. 8:788316. doi: 10.3389/fvets.2021.788316
Received: 02 October 2021; Accepted: 17 November 2021;
Published: 16 December 2021.
Edited by:
Min Du, Washington State University, United StatesReviewed by:
Ran Di, Institute of Animal Sciences, Chinese Academy of Agricultural Sciences (CAAS), ChinaCopyright © 2021 Bai, Li, Chen, Li, Tian, Ma, Raza, Shi, Han, Luo, Hu, Wang, Liu, Li and Zhao. This is an open-access article distributed under the terms of the Creative Commons Attribution License (CC BY). The use, distribution or reproduction in other forums is permitted, provided the original author(s) and the copyright owner(s) are credited and that the original publication in this journal is cited, in accordance with accepted academic practice. No use, distribution or reproduction is permitted which does not comply with these terms.
*Correspondence: Zhidong Zhao, emhhb3pkQGdzYXUuZWR1LmNu
Disclaimer: All claims expressed in this article are solely those of the authors and do not necessarily represent those of their affiliated organizations, or those of the publisher, the editors and the reviewers. Any product that may be evaluated in this article or claim that may be made by its manufacturer is not guaranteed or endorsed by the publisher.
Research integrity at Frontiers
Learn more about the work of our research integrity team to safeguard the quality of each article we publish.