- 1Wadsworth Center, New York State Department of Health, Albany, NY, United States
- 2The Johns Hopkins University School of Medicine, Baltimore, MD, United States
Introduction
Tuberculosis has been a bane of humanity for centuries and, still to this day, is estimated to affect more than two billion people worldwide (1). The slow growth rate of Mycobacterium tuberculosis (MTB) is a major challenge for timely diagnosis and appropriate treatment of cases (2, 3). Diagnostic delays can lead to increased disease burden, cost increases and risk of treatment failures (4). A crucial aspect for clinical assay validations is the availability of well-characterized samples to assess the specificity and sensitivity of the methodology being tested (10). Due to logistical constraints, the nature and availability of specimens, and geographic diversity of the strains, many laboratories struggle with access to adequate clinical MTB specimens for their validation needs (11). Consequently, validation studies may not include representative of the myriad of clinical samples and drug resistant profiles that a clinical laboratory may receive. Therefore, diverse and well-characterized datasets for standardized next generation sequencing (NGS) assay validations for MTB NGS tests are needed. Reference datasets of clinical TB samples and synthetic genomes were released in the past with limited phenotypic drug susceptibility testing (DST) information for research, development and proficiency testing purposes (5, 6). Here, we have assembled a comprehensive dataset of well-characterized whole genome sequences (WGS) from Mycobacterium tuberculosis strains to aid in the development of clinical assays for this pathogen. This dataset includes complete whole genome sequences paired-end read sets obtained through Illumina MiSeq sequencing, along with detailed profiles of drug susceptibility patterns and mutations known to be associated with antimicrobial resistance (AR) to nine MTB drugs. This dataset has been curated to be inclusive of a broad range of lineage diversity, drug susceptibility profiles, and mutation types. As such, this dataset only contains two separate pairs of strains that are phylogenetically closely related (iValiD-TB-S22 and iValiD-TB-S23 with 0 SNP differences and iValiD-TB-S6 and iValiD-TB-S46 with 6 SNPs differences) based on our pipeline results. A complete SNP matrix has been included in Supplementary Table 3. The sequence reads dataset has been made available for bioinformatics pipeline development, and for clinical assay validation of the bioinformatic analysis pipeline, serving as a valuable resource to advance research and enhance the development of clinical MTB NGS assays.
A total of 50 members of the Mycobacterium tuberculosis complex (MTBC) were sequenced, which includes 47 strains of Mycobacterium tuberculosis, one Mycobacterium caprae strain, one Mycobacterium bovis strain and one Mycobacterium bovis-BCG strain (Figure 1). These strains were part of our collection of samples obtained from New York State patients since the implementation in 2013 of our clinical diagnostic and reporting TB NGS assay (7). Of the MTBC, 6 strains are from Lineage 1, 10 from Lineage 2, seven from Lineage 3, 21 from Lineage 4, and 1 representative of each of Lineages 5, 6, and 9. Of the 50 samples, 14 were determined to be pan-susceptible to nine drugs (rifampin, isoniazid, ethambutol, pyrazinamide, streptomycin, ethionamide, fluoroquinolones, kanamycin, and amikacin) by phenotypic drug susceptibility testing, 18 were mono-resistant, 17 multi-drug resistant (MDR) and one was extensively drug resistant (XDR; Figure 1, Supplementary Table 1). A total of 1,073 different mutations present in the 53 screened loci (Supplementary Table 2) characterized by WGS in this dataset, of which, 107 mutations were identified to be associated with drug resistance, most of which are part of the World Health Organization 2023 Catalog of mutations in Mycobacterium tuberculosis (8). The New York State Department of Health implemented this assay for clinical diagnostic before the WHO catalog was released and as such, we are using our own susceptibility interpretation criteria. Consequently, the users of this dataset will have to use their own decision criteria based on their individual workflow characteristics and interpretations. The characterized mutations included single nucleotide polymorphisms (SNP), stop codons, promotor mutations, small insertion and deletions (indels), and large genomic deletions. The locations of the mutations, types, effect on drug resistance, as well as DST results, lineage information, spoligotype and expected mapping statistics are all listed in an individual report card for each sample (Figure 2). The release of this fully characterized dataset will facilitate the development and benchmarking of bioinformatics tools for MTB NGS diagnostics and aide in the validation of these clinical assays. The read sequences are accessible from the NCBI SRA Bioproject PRJNA980174. The associated AR reports cards for the 50 samples are available in Dryad at: https://doi.org/10.5061/dryad.4j0zpc8m8.
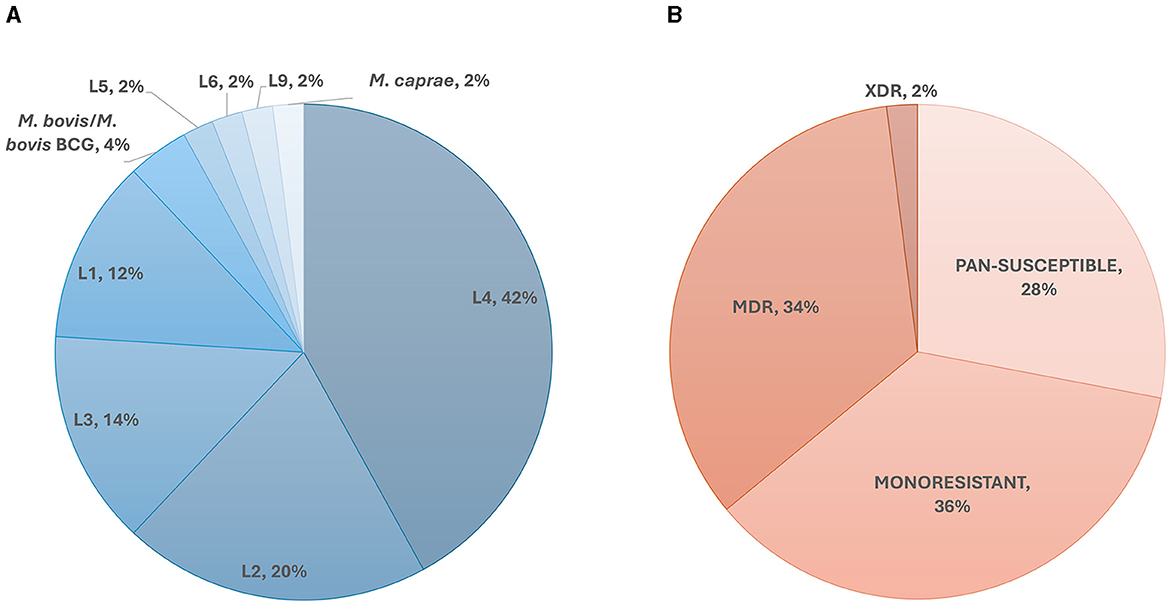
Figure 1. Overall dataset composition. (A) Lineage and species diversity of the dataset. The species identifications were confirmed by real-time PCR and through Kraken as described in Shea et al. (7). The lineages were determined in-silico using lineage-specific genomic markers. (B) Phenotypic drug susceptibility representation of the samples in our dataset. MDR, Multidrug-Resistant; XDR, Extensively Drug-Resistant.
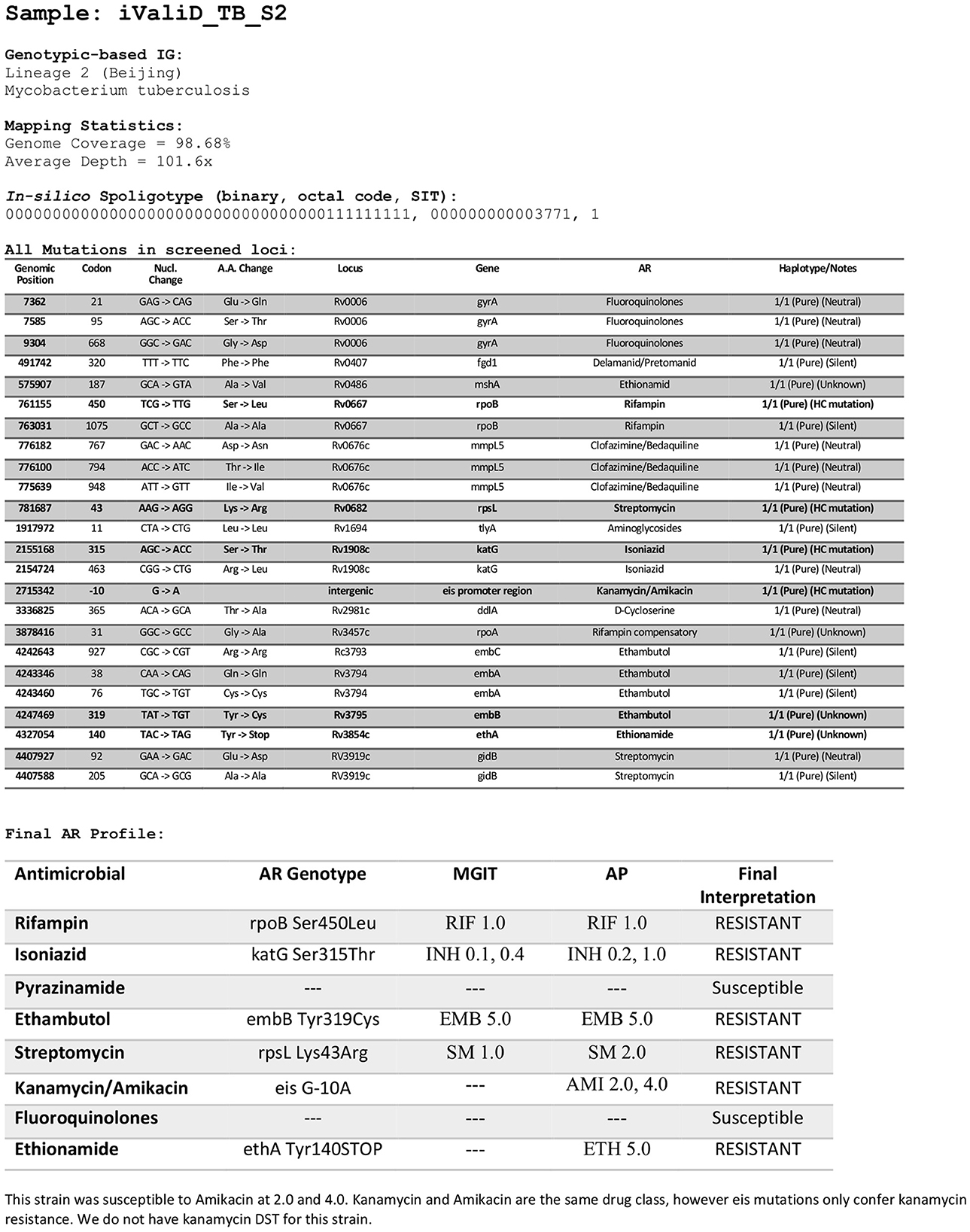
Figure 2. Sample report card. Example of report card for one of the samples in the dataset listing the locations of the mutations, mutation types, drug susceptibility testing results, the final interpretation of the drug resistance, lineage information, spoligotype, and expected mapping metrics. The report cards are available in Dryad at: https://doi.org/10.5061/dryad.4j0zpc8m8. RIF, Rifampin; INH, Isoniazid; EMB, Ethambutol; SM, Streptomycin; AMI, Amikacin; ETH, Ethionamide, PZA, Pyrazinamide; FQ, Fluoroquinolones. DSTs concentrations are in μg/ml.
Methods
Genomic DNA extraction, sequencing library preparation and bioinformatics pipeline methods were described in Shea et al. (7). DSTs were determined by either the agar proportion method on solid 7H10 agar or Becton Dickinson 960 system MGIT SIRE-P assay according to the Clinical and Laboratory Standards Institute's recommendations (9). The following concentrations were used for DST determinations: Streptomycin 1.0 μg/ml, Isoniazid 0.1, 0.2, 0.4, and 1.0 μg/ml, Rifampin 1.0 μg/ml, Ethambutol 5.0 and 10.0 μg/ml, Pyrazinamide 100 μg/ml, Kanamycin 5.0 μg/ml, and ofloxacin (1.0, 2.0, and 4.0 μg/ml). Ofloxacin is used in our laboratory as a representative of the fluoroquinolone (FQ) drug class. Genotypic identification, mapping statistics and in-silico spoligotyping were done as described in Shea et al. (7).
Data availability statement
The datasets presented in this study can be found in online repositories. The data is available in Dyrad at: https://doi.org/10.5061/dryad.4j0zpc8m8.
Author contributions
PL: Conceptualization, Data curation, Software, Writing – original draft, Writing – review & editing. JS: Data curation, Investigation, Validation, Writing – review & editing. SM: Validation, Writing – review & editing. CS: Validation, Writing – review & editing. DK: Supervision, Validation, Writing – review & editing. MD: Supervision, Validation, Writing – review & editing. KM: Conceptualization, Supervision, Writing – review & editing. VE: Conceptualization, Supervision, Validation, Writing – review & editing.
Funding
The author(s) declare that no financial support was received for the research, authorship, and/or publication of this article.
Acknowledgments
We want to acknowledge all the staff from the Wadsworth Center Mycobacteriology Laboratory for the MTB culturing, DNA isolation and susceptibility testing as well as the Wadsworth Center Advanced Genomic Technologies Cluster for library preparations and whole-genome sequencing.
Conflict of interest
The authors declare that the research was conducted in the absence of any commercial or financial relationships that could be construed as a potential conflict of interest.
Publisher's note
All claims expressed in this article are solely those of the authors and do not necessarily represent those of their affiliated organizations, or those of the publisher, the editors and the reviewers. Any product that may be evaluated in this article, or claim that may be made by its manufacturer, is not guaranteed or endorsed by the publisher.
Supplementary material
The Supplementary Material for this article can be found online at: https://www.frontiersin.org/articles/10.3389/ftubr.2024.1441923/full#supplementary-material
References
1. CDCGlobal. Controlling the Global TB Epidemic. Centers for Disease Control and Prevention (2024). Available at: https://www.cdc.gov/globalhivtb/who-we-are/about-us/globaltb/globaltb.html (accessed March 13, 2024).
2. Rageade F, Picot N, Blanc-Michaud A, Chatellier S, Mirande C, Fortin E, et al. Performance of solid and liquid culture media for the detection of Mycobacterium tuberculosis in clinical materials: meta-analysis of recent studies. Eur J Clin Microbiol Infect Dis. (2014) 33:867–70. doi: 10.1007/s10096-014-2105-z
3. Asmar S, Drancourt M. Rapid culture-based diagnosis of pulmonary tuberculosis in developed and developing countries. Front Microbiol. (2015) 6:1184. doi: 10.3389/fmicb.2015.01184
4. Santos JA, Leite A, Soares P, Duarte R, Nunes C. Delayed diagnosis of active pulmonary tuberculosis—potential risk factors for patient and healthcare delays in Portugal. BMC Publ Health. (2021) 21:2178. doi: 10.1186/s12889-021-12245-y
5. Borrell S, Trauner A, Brites D, Rigouts L, Loiseau C, Coscolla M, et al. Reference set of Mycobacterium tuberculosis clinical strains: a tool for research and product development. PLoS ONE. (2019) 14:e0214088. doi: 10.1371/journal.pone.0214088
6. Anthony RM, Tagliani E, Nikolayevskyy V, de Zwaan R, Mulder A, Kamst M, et al. Experiences from 4 years of organization of an external quality assessment for mycobacterium tuberculosis whole-genome sequencing in the European Union/European Economic area. Microbiol Spectr. (2023) 11:e0224422. doi: 10.1128/spectrum.02244-22
7. Shea J, Halse TA, Lapierre P, Shudt M, Kohlerschmidt D, Van Roey P, et al. Comprehensive whole-genome sequencing and reporting of drug resistance profiles on clinical cases of Mycobacterium tuberculosis in New York State. J Clin Microbiol. (2017) 55:1871–82. doi: 10.1128/JCM.00298-17
8. WHO. Catalogue of Mutations in Mycobacterium tuberculosis Complex and Their Association With Drug Resistance. (2023). Available at: https://www.who.int/publications/i/item/9789240028173 (accessed August 2, 2024).
9. Woods GL, Brown-Elliott BA, Conville PS, Desmond EP, Hall GS, Lin G, et al. Susceptibility Testing of Mycobacteria, Nocardiae, and Other Aerobic Actinomycetes. 2nd Edn. Wayne, PA: Clinical and Laboratory Standards Institute (2011). Available at: http://www.ncbi.nlm.nih.gov/books/NBK544374/ (accessed May 31, 2024).
10. NYSDOH NGS. Molecular Guidance Update. (2023). Available at: https://www.wadsworth.org/sites/default/files/WebDoc/ID_WGS_NGS_Molecular_Guidance_update_032223.pdf (accessed April 22, 2024).
Keywords: tuberculosis, Mycobacterium tuberculosis, whole genome sequence (WGS), bioinformatics, clinical validation, antimicrobial susceptibility, antibiotic resistance
Citation: Lapierre P, Shea J, Murphy SG, Smith C, Kohlerschmidt D, Dickinson M, Musser KA and Escuyer V (2024) iValiD-TB: a fully characterized Mycobacterium tuberculosis dataset for antimicrobial resistance bioinformatics workflow validations. Front. Tuberc. 2:1441923. doi: 10.3389/ftubr.2024.1441923
Received: 31 May 2024; Accepted: 13 August 2024;
Published: 13 September 2024.
Edited by:
Karen Marie Dobos, Colorado State University, United StatesReviewed by:
Octavio Rivero-Lezcano, Complejo Asistencial Universitario de León (CHLeon), SpainRichard Anthony, National Institute for Public Health and the Environment, Netherlands
Copyright © 2024 Lapierre, Shea, Murphy, Smith, Kohlerschmidt, Dickinson, Musser and Escuyer. This is an open-access article distributed under the terms of the Creative Commons Attribution License (CC BY). The use, distribution or reproduction in other forums is permitted, provided the original author(s) and the copyright owner(s) are credited and that the original publication in this journal is cited, in accordance with accepted academic practice. No use, distribution or reproduction is permitted which does not comply with these terms.
*Correspondence: Pascal Lapierre, cGFzY2FsLmxhcGllcnJlQGhlYWx0aC5ueS5nb3Y=
†ORCID: Pascal Lapierre orcid.org/0000-0003-1238-0955