- 1Integrative and Experimental Training Science, Institute of Sport Sciences, University of Würzburg, Würzburg, Germany
- 2Department of Sports Science and Movement Pedagogy, Technische Universität Braunschweig, Braunschweig, Germany
- 3Software Engineering Group, Department of Computer Science, University of Würzburg, Würzburg, Germany
- 4Department of Health Sciences, Luleå University of Technology, Luleå, Sweden
- 5Department of Physiology and Pharmacology, Biomedicum C5, Karolinska Institutet, Stockholm, Sweden
Here, we performed a non-systematic analysis of the strength, weaknesses, opportunities, and threats (SWOT) associated with the application of artificial intelligence to sports research, coaching and optimization of athletic performance. The strength of AI with regards to applied sports research, coaching and athletic performance involve the automation of time-consuming tasks, processing and analysis of large amounts of data, and recognition of complex patterns and relationships. However, it is also essential to be aware of the weaknesses associated with the integration of AI into this field. For instance, it is imperative that the data employed to train the AI system be both diverse and complete, in addition to as unbiased as possible with respect to factors such as the gender, level of performance, and experience of an athlete. Other challenges include e.g., limited adaptability to novel situations and the cost and other resources required. Opportunities include the possibility to monitor athletes both long-term and in real-time, the potential discovery of novel indicators of performance, and prediction of risk for future injury. Leveraging these opportunities can transform athletic development and the practice of sports science in general. Threats include over-dependence on technology, less involvement of human expertise, risks with respect to data privacy, breaching of the integrity and manipulation of data, and resistance to adopting such new technology. Understanding and addressing these SWOT factors is essential for maximizing the benefits of AI while mitigating its risks, thereby paving the way for its successful integration into sport science research, coaching, and optimization of athletic performance.
Introduction
Artificial intelligence (AI) is the “theory and development of computer systems able to perform tasks that normally require human intelligence” and “makes it possible for machines to learn from experience, adjust to new inputs, and perform human-like tasks” (1). AI encompasses all forms of classical machine learning and modern artificial neural networks and through the processing of large amounts of available data (2) develop more and more human-like capabilities for decision-making and planning. The various applications of artificial intelligence (AI) are revolutionizing numerous aspects of our society (3–5), including the academic community (5) focusing on applied research relevant to sports.
This community is still in the early stages of utilizing the potential of AI (6) to maintain and improve athletic performance, prevent injuries, optimize training and assist in overall decision-making (7–9). However, as has already been carried out with other novel technologies being applied to the practice of and research on sports (10, 11), an ongoing and comprehensive understanding of the potential strengths, weaknesses, opportunities, and threats (SWOT) of AI in this context is required. To evaluate the application of artificial intelligence (AI) in sports, we executed a SWOT analysis through brief interviews with athletes, coaches, and computer science experts specialized in AI. In addition, we performed a non-systematic review of academic papers, case studies, and reports by actors in the sports industry. The broad perspective on this subject presented here is meant primarily as an aid in strategic planning, risk assessment, and resource allocation in connection with sport science research, coaching, and optimization of athletic performance.
Strengths
Automation of time-consuming tasks
A growing number of repetitive and time-consuming tasks, such as processing, and analysis of medical data, as well as reporting of findings can be performed by AI (12, 13). For example, language-based AI such as ChatGPT (OpenAI, CA, USA) has already demonstrated its potential to assist healthcare professionals in writing medical reports (14), although its applicability with respect to various types of sports writing remains to be established.
Processing and analysis of large amounts of data
AI systems can process large sets of data and perform complex calculations, thereby enabling accurate and efficient analysis of (longitudinal) data on numerous athletes (9). For example, certain types of AI can already analyze the spatiotemporal behavior of soccer players in a manner that allows, among other things, automatic identification of dynamic attack formations, information which might be of value in connection with tactical training (15).
Recognition of patterns and relationships
In connection with its rapid processing and analysis of large amounts of data, AI may reveal patterns, trends, relationships, and other insights not immediately apparent to human observers (16–20).
Weaknesses
Access to comprehensive, up-to-date and high-quality data at all times
When the data employed by AI fails to encompass all relevant aspects of the question being posed (6) and/or is characterized by low quality, flaws, bias, incompleteness, or unreliability, the analyses conducted and the prospective insights derived or conclusions drawn could be fallacious. Particularly worrisome in this context is the fact that the data employed to train AI models are often historical and may not always capture emerging trends, changes in techniques, strategies or rules, or individual differences that can exert a significant impact on the current situation. Similarly, changes in the situation of individual athletes, such as injuries, improvements or deterioration in form, or personal circumstances may not be adequately accounted for. To mitigate this limitation, it is essential to continuously update AI models, as well as to incorporate mechanisms that can identify emerging trends and individual variations.
Unrecognized bias in the data on which the AI system is trained
If the training data are biased (e.g., with respect to race, sex or age), recommendations based on this data may be biased as well, as widely discussed in the medical literature (21). This situation may be exacerbated by the propagation of biases from earlier to later models of AI.
Decision-making based on “black-box” algorithms
Some AI systems, such as artificial neural networks, can be considered “black boxes” which perform data analysis and decision-making that is un- or even counterintuitive to human brains (6). This absence of transparency in AI models can raise concerns among athletes, coaches, and other stakeholders. For instance, when an AI model is initially trained using data regarding elite athletes, but subsequently applied to non-elite or sub-elite athletes, it may introduce biases that could result in erroneous decisions and pose potential risks to athletes which would not be apparent to the humans involved, due to the opaque nature of the decision-making process.
Over-reliance on AI technology
While AI can provide valuable insights, it should not replace human judgment entirely. Athletes and coaches should not abandon their own critical evaluations, but make the final decisions, relying on AI for support. Unfortunately, in this context recent research findings indicate the presence of a tendency towards excessive reliance on AI for decision-making, with an associated potential risk of making the wrong decision (22).
Ethical considerations
Application of AI to sport science raises ethical concerns e.g., regarding the privacy of and consent to use the data collected, which must be addressed. In addition, over-reliance on AI may limit the personal experience and thereby hinder the personal growth of prospective trainers.
Costs and requirements for other resources
Reliable application of AI technology to sport science may require a considerable level of expertise, significant financial investment, specialized infrastructure, and individuals who can accurately interpret the output (6). This could give teams with more resources a considerable advantage.
Potential reduction of human interactions
As AI becomes more prevalent, humans may have to do more and more “cleaning” up and otherwise preparing data for usage by AI. This may necessitate hiring additional personnel or require regular staff members to allocate more of their time to such duties, potentially reducing the time they have available for interaction with their athletes.
Opportunities
Monitoring athletes both long-term and in real-time
AI allows overall individual athletic performance and relevant individual physiological variables to be analyzed both in real-time and long-term. Such feedback enables short- and long-term adjustments (23) in training load and other interventions that can help individualize and optimize both training and competitive performance. Sufficiently comprehensive data, combining video recordings with a wide variety of measurements (24), also promises to predict future performance and aid in early diagnosis of injury, followed by design, monitoring and assessment of appropriate rehabilitation.
Prediction of risk for future injury
On the basis of their analyses of individual athletes, including their health and history of injuries, AI-based systems may aid coaches and medical staff in preventing injuries before they occur (17). In this context, artificial neural networks, decision trees and support vectors are already being used to assess risk for injury in connection with different team sports (17).
Identification of talent
By analyzing large amounts of data concerning the determinants of performance, including physical attributes, and other potential indicators of success for individual athletes, AI can potentially help identify new talent (19, 20).
Development of novel indicators of performance
In addition to simplifying the monitoring of indicators of performance which have been previously difficult to measure, the correct comprehensive analysis and interpretation of data on both individual and groups of athletes by AI could help reveal novel indicators of performance (25).
More automated data- and evidence-based decision-making
The massive amounts of objective data that can be collected and analyzed by AI allow coaches and athletes to more easily make data- and, ideally, evidence-based decisions about training, competition, and coaching strategies concerning, e.g., training intensities, recovery protocols, and tactical adjustments. For instance, training plans for kickboxers generated automatically can be tailored to each individual athlete's current level of performance, period of development, and performance goals, thereby not only reducing the time required for planning, but also providing plans that were more comprehensive and personalized than those designed by an expert coach (26).
Increased automatization of repetitive tasks, helping to improve the coach-athlete relationship
The performance of repetitive and time-consuming tasks by AI could free up more time for personal activities that improve the relationship between a coach and his athletes, which is often regarded as a key factor in the effectiveness of training (27).
More collaboration and data sharing
AI-powered platforms and systems have the potential to facilitate collaboration and other types of interactions between sport scientists, coaches, and athletes, enabling easy sharing of data, insights, and guidelines for best practices.
Threats
Risks to data privacy
The collection, storage and analysis of sensitive data on individual athletes by AI can expose organizations to privacy breaches, cyber-attacks, or other forms of unauthorized access. Thus, robust data protection is required. In addition, there is the possibility that the company managing the AI system may utilize personal data for the purpose of enhancing their models, which may lead to unintentional exposure of this data to third-party users.
Legal and regulatory challenges
The incorporation of AI into sport science raises a number of legal and regulatory concerns, such as ownership of data and copyrights, liability for deleterious consequences of AI-based decisions, and compliance with prevailing regulations concerning data protection. To address such challenges, the European Parliament recently issued a resolution aimed at legislative regulation of AI (28).
Data integrity and manipulation
For a variety of reasons, malicious actors may attempt to manipulate the data employed by AI systems, even introducing false information, actions commonly referred to as adversarial attacks and which can alter the decisions arrived at. One such example would be manipulating the camera of a self-driving car so that it overlooks red traffic lights (29). In a similar manner, sports data could be altered by competitors to cause the opposing team to, for example, adopt suboptimal tactics.
Deleterious social and psychological impact on athletes
Usage of AI in sport science may impair the psychological well-being of athletes, leading, for example, to performance anxiety, pressure to conform to AI-generated recommendations, and less trust in the coach. Communication and psychological aspects of the relationships between athletes, coaches and the support staff may be damaged.
Resistance to adopting AI
As with any new technology, integration of AI into sports science may face resistance (6). There can be many reasons for such resistance and these need to be assessed employing, e.g., the technology acceptance framework (30) to survey coaches, athletes and other stakeholders.
Limitations and future research
One limitation of the present study lies in the fact that, although our conclusions are consistent with those of both other SWOT analyses and non-systematic approaches in the realm of sports science (10, 11), we did not employ a systematic approach to the identification of strengths, weaknesses, opportunities, and threats. Consequently, we cannot guarantee the comprehensiveness of the information provided.
Furthermore, due to the continuous rapid development of artificial intelligence, new strengths, weaknesses, opportunities, and threats may emerge, while some of those discussed here may become less prominent. Future research in this area should aim to assess the application of AI to various aspects of sports science research, coaching, and optimization of athletic performance, utilizing an interdisciplinary approach involving professionals in handling and analyzing data, exercise and training of athletes, and ethical and social issues, among others.
In addition, there is a pressing need to educate sports practitioners in order to ensure that they implement AI properly and optimally, capitalizing on its strengths and opportunities, while mitigating potential threats and weaknesses. In this context, one branch of AI, i.e., Explainable Artificial Intelligence (XAI), is attempting to design machine learning architectures that are more transparent, interpretable, and accountable to human users (so-called “White” or “Glass” as opposed to “Black-Boxes”) (31). In situations where decision-making can exert immediate and long-lasting impacts, such transparency and interpretability are crucial. Understanding the rationale behind analyses performed by AI—including analyses of data on a playeŕs performance, prediction of injury, or optimization of game strategy—enables more informed and ethical usage of this technology, while at the same time promoting trust in its analyses.
Summary
The use of AI in sports science research, coaching and athlete performance faces various strengths, weaknesses, opportunities, and threats, as summarized in Figure 1.
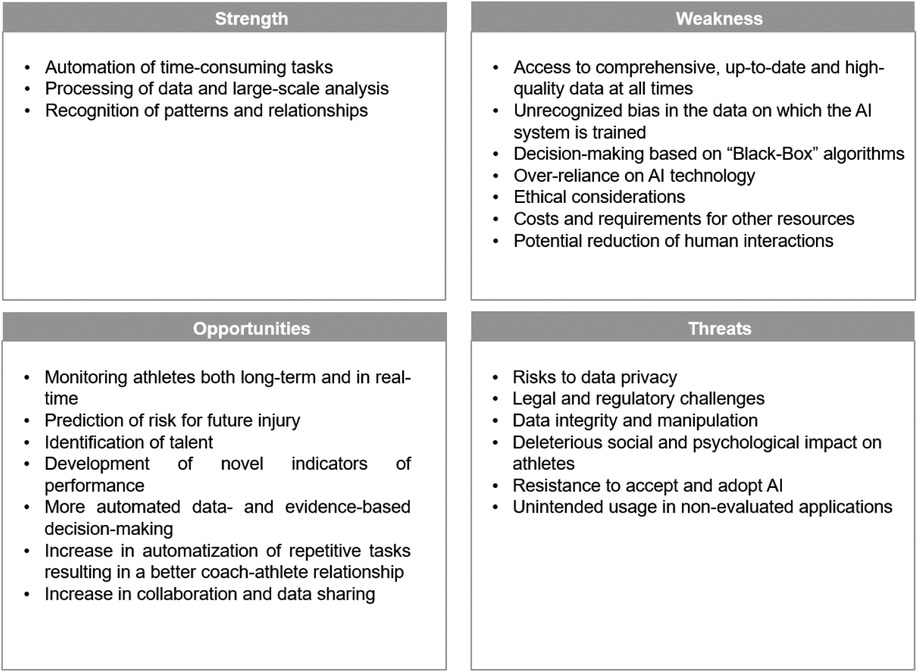
Figure 1. Strengths, weaknesses, opportunities, and threats associated with the use of artificial intelligence in sports science.
AI offers strengths such as automating repetitive and/or time-consuming tasks, performing large-scale analyses, recognizing patterns, predicting future events, and identifying talent. It can help optimize training, prevent injuries, and enhance decision-making. However, there are also weaknesses and threats to consider. The weaknesses include the need for high-quality data, a limited ability to interpret complex sport-specific situations, lack of human intuition, ethical considerations, biases, limited adaptability, costs, and lack of interpretability. Threats include risks to data privacy, legal and regulatory challenges, the integrity and manipulation of data, overreliance on AI, a negative psychological impact on athletes, technological limitations and biases, resistance to change, and overemphasis on quantitative data. It is crucial to address these weaknesses and threats for optimal usage of the strengths and opportunities offered by AI in connection with sports science research, coaching, and the optimization of athletic performance.
Data availability statement
For the original contributions presented in the article, further inquiries can be directed to the corresponding author.
Author contributions
BS: Conceptualization, Writing – original draft, Writing – review & editing. PD: Conceptualization, Writing – original draft, Writing – review & editing. RL: Conceptualization, Formal Analysis, Writing – original draft. HH: Data curation, Writing – original draft.
Funding
The author(s) declare that no financial support was received for the research, authorship, and/or publication of this article.
Conflict of interest
The authors declare that the research was conducted in the absence of any commercial or financial relationships that could be construed as a potential conflict of interest.
The author(s) declared that they were an editorial board member of Frontiers, at the time of submission. This had no impact on the peer review process and the final decision.
Publisher's note
All claims expressed in this article are solely those of the authors and do not necessarily represent those of their affiliated organizations, or those of the publisher, the editors and the reviewers. Any product that may be evaluated in this article, or claim that may be made by its manufacturer, is not guaranteed or endorsed by the publisher.
References
1. Joiner I. Artificial intelligence: AI is nearby. Emerging library technologies. Amsterdam, the Netherlands: Chandos Publishing (2018). 1–22.
2. Parliament E. What is artificial intelligence and how is it used? (2023). https://www.europarl.europa.eu/news/en/headlines/society/20200827STO85804/what-is-artificial-intelligence-and-how-is-it-used
3. Amisha Malik P, Pathania M, Rathaur VK. Overview of artificial intelligence in medicine. J Family Med Prim Care. (2019) 8:2328–31. doi: 10.4103/jfmpc.jfmpc_440_19
4. Malik S, Khan MA, El-Sayed H, Khan J, Ullah O. How do autonomous vehicles decide? Sensors (Basel). (2022) 23. doi: 10.3390/s23010317
5. Miller LE, Bhattacharyya D, Miller VM, Bhattacharyya M. Recent trend in artificial intelligence-assisted biomedical publishing: a quantitative bibliometric analysis. Cureus. (2023) 15:e39224.37337487
6. Hammes F, Hagg A, Asteroth A, Link D. Artificial intelligence in elite sports-A narrative review of success stories and challenges. Front Sports Act Living. (2022) 4:861466. doi: 10.3389/fspor.2022.861466
7. Chase C. The data revolution: cloud computing, artificial intelligence, and machine learning in the future of sports. In: Schmidt SL, editor. 21st century sports: How technologies will change sports in the digital age. Cham: Springer International Publishing (2020). p. 175–89.
8. Schmidt SL. How technologies impact sports in the digital age. In: Schmidt SL, editor. 21st century sports: how technologies will change sports in the digital age. Cham: Springer International Publishing (2020). p. 3–14.
9. Seshadri DR, Thom ML, Harlow ER, Gabbett TJ, Geletka BJ, Hsu JJ, et al. Wearable technology and analytics as a complementary toolkit to optimize workload and to reduce injury burden. Front Sports Act Living. (2020) 2:630576. doi: 10.3389/fspor.2020.630576
10. Sperlich B, Duking P, Holmberg HC. A SWOT analysis of the use and potential misuse of implantable monitoring devices by athletes. Front Physiol. (2017) 8:629. doi: 10.3389/fphys.2017.00629
11. Duking P, Holmberg HC, Sperlich B. The potential usefulness of virtual reality systems for athletes: a short SWOT analysis. Front Physiol. (2018) 9:128. doi: 10.3389/fphys.2018.00128
12. Do HM, Spear LG, Nikpanah M, Mirmomen SM, Machado LB, Toscano AP, et al. Augmented radiologist workflow improves report value and saves time: a potential model for implementation of artificial intelligence. Acad Radiol. (2020) 27:96–105. doi: 10.1016/j.acra.2019.09.014
13. Li X, Tian D, Li W, Hu Y, Dong B, Wang H, et al. Using artificial intelligence to reduce queuing time and improve satisfaction in pediatric outpatient service: a randomized clinical trial. Front Pediatr. (2022) 10:929834. doi: 10.3389/fped.2022.929834
14. Zhou Z. Evaluation of ChatGPT’s capabilities in medical report generation. Cureus. (2023) 15(4):e37589. doi: 10.7759/cureus.37589
15. Goes FR, Brink MS, Elferink-Gemser MT, Kempe M, Lemmink KA. The tactics of successful attacks in professional association football: large-scale spatiotemporal analysis of dynamic subgroups using position tracking data. J Sports Sci. (2021) 39:523–32. doi: 10.1080/02640414.2020.1834689
16. Vermeulen E, Venkata S. (2018). Big data in sport analytics: applications and risks. In: Industrial engineering and operations management (presidencia). Proceedings of the international conference on industrial engineering and operations management. Conferencia llevada a cabo en IEOM society international; Pretoria/Johannesburg, South Africa. Recuperado de https://bit.ly/3ojotk9
17. Claudino JG, Capanema DDO, De Souza TV, Serrão JC, Machado Pereira AC, Nassis GP. Current approaches to the use of artificial intelligence for injury risk assessment and performance prediction in team sports: a systematic review. Sports Med Open. (2019) 5:28. doi: 10.1186/s40798-019-0202-3
18. Sritharan P, Munoz MA, Pivonka P, Bryant AL, Mokhtarzadeh H, Perraton LG. Biomechanical markers of forward hop-landing after ACL-reconstruction: a pattern recognition approach. Ann Biomed Eng. (2022) 50:330–42. doi: 10.1007/s10439-022-02921-4
19. Muazu Musa R, Abdul Majeed APP, Taha Z, Abdullah MR, Husin Musawi Maliki AB, Azura Kosni N. The application of artificial neural network and k-nearest neighbour classification models in the scouting of high-performance archers from a selected fitness and motor skill performance parameters. Sci Sports. (2019) 34:e241–9. doi: 10.1016/j.scispo.2019.02.006
20. Liu J-W, Chen S-H, Chen C-H, Huang T-H. Constructing an artificial intelligence strategy algorithm for the identification of talented rowing athletes. Soft Comput. (2023) 27:1743–50. doi: 10.1007/s00500-021-06050-3
21. Li H, Moon JT, Purkayastha S, Celi LA, Trivedi H, Gichoya JW. Ethics of large language models in medicine and medical research. Lancet Digit Health. (2023) 5:e333–5. doi: 10.1016/S2589-7500(23)00083-3
22. Buçinca Z, Malaya MB, Gajos KZ. To trust or to think: cognitive forcing functions can reduce overreliance on AI in AI-assisted decision-making. Proc ACM Hum Comput Interact. (2021) 5:1–21. doi: 10.1145/3449287
23. Duking P, Achtzehn S, Holmberg HC, Sperlich B. Integrated framework of load monitoring by a combination of smartphone applications, wearables and point-of-care testing provides feedback that allows individual responsive adjustments to activities of daily living. Sensors (Basel). (2018) 18. doi: 10.3390/s18051632
24. Balsalobre-Fernandez C, Tejero-Gonzalez CM, Del Campo-Vecino J, Bavaresco N. The concurrent validity and reliability of a low-cost, high-speed camera-based method for measuring the flight time of vertical jumps. J Strength Cond Res. (2014) 28:528–33. doi: 10.1519/JSC.0b013e318299a52e
25. Phatak AA, Mehta S, Wieland FG, Jamil M, Connor M, Bassek M, et al. Context is key: normalization as a novel approach to sport specific preprocessing of KPI’s for match analysis in soccer. Sci Rep. (2022) 12:1117. doi: 10.1038/s41598-022-05089-y
26. Skerik T, Chrpa L, Faber W, Vallati M. Automated training plan generation for athletes. In: 2018 IEEE international conference on systems, man, and cybernetics (SMC). IEEE (2018). p. 3865–70.
27. Anyadike-Danes K, Donath L, Kiely J. Coaches’ perceptions of factors driving training adaptation: an international survey. Sports Med. (2023):1–8.35687251
28. Parliament E. EU AI act: first regulation on artificial intelligence [Online] (2023). Available at: https://www.europarl.europa.eu/news/en/headlines/society/20230601STO93804/eu-ai-act-first-regulation-on-artificial-intelligence?&at_campaign=20226-Digital&at_medium=Google_Ads&at_platform=Search&at_creation=RSA&at_goal=TR_G&at_advertiser=Webcomm&at_audience=eu%20ai%20legislation&at_topic=Artificial_intelligence_Act&at_location=DE&gclid=CjwKCAjwivemBhBhEiwAJxNWN6MBC6TSCCF-dbkFTGZ4tVTJMpGK3wZz-LEn-k9mvPizheQfyJ1diRoC2UcQAvD_BwE (Accessed 2023).
29. Deng Y, Zheng X, Zhang T, Chen C, Lou G, Kim M. An analysis of adversarial attacks and defenses on autonomous driving models. In: 2020 IEEE international conference on pervasive computing and communications (PerCom). IEEE (2020). p. 1–10.
Keywords: XAI and explainable artificial intelligence, XAI, elite sport, performance, exercise science, SWOT, artifical inteligence
Citation: Sperlich B, Düking P, Leppich R and Holmberg H-C (2023) Strengths, weaknesses, opportunities, and threats associated with the application of artificial intelligence in connection with sport research, coaching, and optimization of athletic performance: a brief SWOT analysis. Front. Sports Act. Living 5:1258562. doi: 10.3389/fspor.2023.1258562
Received: 14 July 2023; Accepted: 29 September 2023;
Published: 18 October 2023.
Edited by:
Korkut Ulucan, Marmara University, TürkiyeReviewed by:
Khairul Hafezad Abdullah, MARA University of Technology, MalaysiaLina E. Lundgren, Halmstad University, Sweden
© 2023 Sperlich, Düking, Leppich and Holmberg. This is an open-access article distributed under the terms of the Creative Commons Attribution License (CC BY). The use, distribution or reproduction in other forums is permitted, provided the original author(s) and the copyright owner(s) are credited and that the original publication in this journal is cited, in accordance with accepted academic practice. No use, distribution or reproduction is permitted which does not comply with these terms.
*Correspondence: Billy Sperlich YmlsbHkuc3BlcmxpY2hAdW5pLXd1ZXJ6YnVyZy5kZQ==