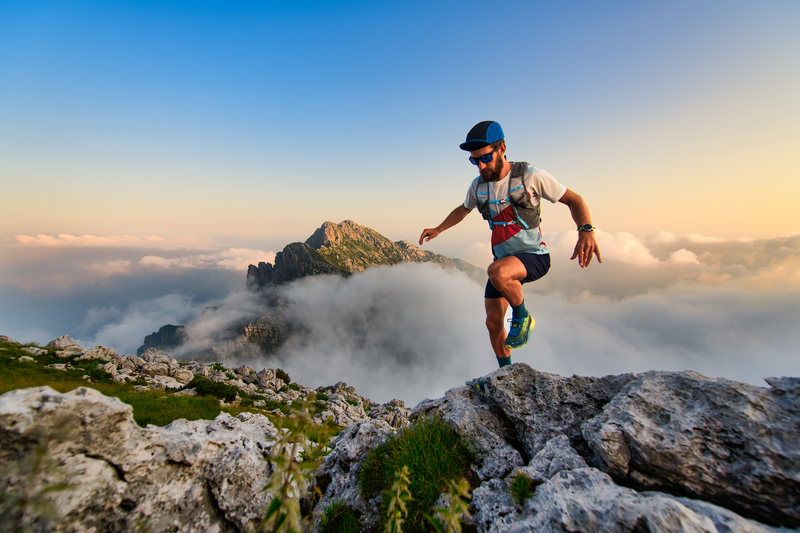
95% of researchers rate our articles as excellent or good
Learn more about the work of our research integrity team to safeguard the quality of each article we publish.
Find out more
ORIGINAL RESEARCH article
Front. Water , 17 December 2024
Sec. Water Resource Management
Volume 6 - 2024 | https://doi.org/10.3389/frwa.2024.1507961
This article is part of the Research Topic Advancement in Hydrological Modeling and Water Resources Management for achieving Sustainable Development Goals (SDGs) View all 16 articles
Introduction: This study aims to assess the efficacy of Quantile mapping (QM) and Delta change (DC) bias correction methods to improve hydrological simulations of the Chiffa basin in northern Algeria. The main issue addressed is the need for corrected climate data to provide reliable hydrological projections in semi-arid climates.
Methods: Hydrological simulations were conducted using the GR2M conceptual rainfall-runoff model, recognized for its robustness in Mediterranean climates. This model was coupled with precipitation simulations from the Rossby Centre regional atmospheric model RCA4 of the Coordinated Regional Climate Downscaling Experiment (Cordex-Africa) forced by two global circulation models (MPI-ESM-LR and CRNM-CM5). Hydrological projections were produced for the future period 20702099 under RCP 4.5 and RCP 8.5 scenarios, comparing raw and bias-corrected data.
Results and discussion: The findings indicate that raw precipitation data are inadequate for reflecting future rainfall trends and simulating future flows. Bias correction methods significantly improved the models performance, with the coefficient of determination (R2) increasing from 0.440.53 to 0.830.97. Additionally, regional climate models project a 5 to 8% decrease in annual flows by the end of the 21st century under RCP 4.5 and RCP 8.5 scenarios. These results highlight the importance of bias correction methods for hydrological impact studies, and we recommend implementing specific adaptation measures, such as improved irrigation efficiency, development of water storage infrastructure, and adoption of drought-resistant agricultural practices. Future research should focus on employing multivariate bias correction methods, utilizing higher-resolution climate data (≤10 km), and implementing ensemble modeling approaches to better characterize uncertainties.
• Coordinated Regional Climate Downscaling Experiment (CORDEX)-Africa domain climate model data are used to assess the hydrological impacts of climate change on the Chiffa basin in northern Algeria.
• Two bias correction methods are used to improve hydrological simulations.
• Bias correction methods highlight important effects on future flow projections.
• The future flow of the study area will likely decrease by the end of the 21st century.
• This study recommends the implementation of adaptation and mitigation measures to ensure the sustainability of water resources.
The climate change assessments and the development of adaptation and mitigation strategies are generally based on the projection of Global Circulation Models (GCMs) (Sorland et al., 2018; IPCC et al., 2019; Parthiban and Amit, 2021). However, the outputs from the GCM simulation have a coarse resolution, which is not adequate for hydrological applications.
Regional Climate Models (RCMs) provide a dynamic downscaling to a finer resolution, making them suitable for hydrological impact studies (Smitha et al., 2018; Shin et al., 2019; Dixit et al., 2021). Some studies have shown that using downscaled GCM data through RCMs (RCM-GCM) can introduce significant uncertainties and biases that can significantly reduce the reliability of the results (Smitha et al., 2018; Mendez et al., 2020; Mesta and Kentel, 2021; Mengistu et al., 2021).
The climate variables that are simulated by various RCM-GCMs generally represent an inadequate distribution of the observed data. The gap that exists between simulation and observation, has been identified as bias or error (Dimri, 2021; Chelkeba, 2021). Therefore, bias correction techniques are applied to enhance the accuracy of RCM-GCM simulations (Pastén-Zapata et al., 2020; Miralha et al., 2021; Tamene and Chala, 2021).
There are several methods of bias correction, depending on different approaches. For example, linear scaling (ML) is a method that corrects the monthly average and maintains the variability of climate model-adjusted data consistent with uncorrected data (Teutschbein and Seibert, 2010; Chathuranika et al., 2022).
The Quantile mapping (QM) method is also a bias correction technique that adjusts the cumulative distribution function of a simulated variable to align with the observed distribution function by using a transfer function (Teutschbein and Seibert, 2012; Willkofer et al., 2018; Soriano et al., 2019; Szabó-Takács et al., 2019). Teutschbein and Seibert (2012), found that all bias correction methods are effective; however, Quantile mapping is more reliable, a finding also supported by several studies (White and Toumi, 2013; Foughali et al., 2015; Maraun, 2016; Emami and Koch, 2018; Tan et al., 2020).
Local intensity scaling (LOCI) is a method that corrects both precipitation intensity and frequency (Schmidli et al., 2006; Willkofer et al., 2018; Satiprasad et al., 2019; Szabó-Takács et al., 2019).
The bias correction can also be done through an approach based on power transformation. This method is used to adjust the rainfall variance statistics. The simulated monthly precipitation is powered by a value “b,” which guarantees that this approach ensures correspondence between the coefficient of variance (CV) of the simulated daily precipitation and the CV of the observed daily precipitation (Rajab et al., 2020; Szabó-Takács et al., 2019).
The Delta change (DC) method is the simplest method. It does not propose a correction for RCM-GCM simulations; instead, it acts as a perturbation method. It generates future climate projections by adjusting the observed climatic series to reflect the differences between the reference and future raw RCM-GCM simulations (Räty et al., 2018; Eekhout and De Vente, 2018; Beyer et al., 2019). This technique was largely used worldwide, especially in the Mediterranean region (Macias et al., 2018; François et al., 2020). In addition, this method was considered the most efficient compared to other methods of bias correction applied at the level of Turkey (Oruc, 2022).
Several studies propose a comparison of bias correction techniques for different regions of the Mediterranean basin. Marcos et al. (2018) assessed the ability of three bias correction methods two linear (mean bias correction (BC), and linear regression (LR)) and a nonlinear (model output statistic analogs (MOS-analog)) to improve the seasonal feature of precipitation simulated by climate models during the period 1981–2100 in the Boadella reservoir (northwestern Mediterranean). The results suggest that the analogous (MOS-analog) approach to model output statistics and the linear regression method generally perform better than other approaches in late autumn and early winter. They demonstrate the possibility of introducing bias correction methods to improve the seasonal prediction of water resources in the climate services system.
Similarly, Martins et al. (2021) have attempted to compare two bias correction methods: linear and Quantile mapping. These methods were applied to a bias-correct RCM-GCM simulation of temperature and precipitation during the reference period 1989–2005 in the Douro Wine Region, Portugal. The results indicate that the linear method corrects only the location (mean) and the scale (standard deviation) of the empirical probability distribution function (EPDF), while the Quantile mapping corrects the complete EPDF. The first method appears to be less computationally demanding, which makes easier to execute in large sets of data.
In Algeria, only a few studies have addressed bias correction when using climate model outputs. Taibi et al. (2021b) demonstrated that the use of Delta change and Quantile mapping methods for the correction of simulated precipitation from the outputs of the Cordex-Africa regional climate models yields a superior analysis of precipitation in the Oran coastal basin in Algeria. Zeroual et al. (2020) also used the Quantile mapping method to identify climate zones based on the Koppen-Geiger classification for all of Algeria by the end of the century.
The presence of biases in the climate simulations resulting from RCM-GCMs is worth correcting before being used in impact studies to allow a better assessment of climate projections. The response of hydrological systems to climate change can be carried out according to the direct use of runoff simulated by the global climate models of the CMIP5 or regional (Alkama et al., 2013; Zheng et al., 2018).
Conceptual Rainfall-Runoff was widely used in hydrological impact studies of climate change, due to the limited data they require (mainly rain and evapotranspiration). This is true, especially for developing countries suffering from data scarcity. This approach is most often used, and it has been the subject of many studies. Those studies aimed at simulating future flows at the scale of a watershed, using the outputs of climatic models of rainfall and temperatures as input data to the hydrological model (Ibrahim et al., 2015; Todorovic and Plavsic, 2016; Giuntoli et al., 2018; Al-Safi et al., 2020; Hadour et al., 2020; Sidibe et al., 2020). In Algeria, the GR2M Hydrological model is widely used given its simplicity but also its efficacy in conveniently reproducing the hydrological functioning of Algerian watersheds (Zeroual et al., 2013; Ouhamdouche et al., 2018).
The Chiffa watershed presents specific hydrological challenges characteristic of Mediterranean semi-arid regions. Its complex topography, ranging from mountainous areas to coastal plains, combined with high temporal and spatial variability of precipitation, makes it particularly sensitive to climate model biases. The basin experiences intense seasonal variations, with dry summers and irregular winter rainfall, often resulting in flash floods. These regional characteristics make the bias correction of climate models particularly important, as uncorrected biases could significantly affect the representation of both extreme events and low-flow periods, which are critical for water resource management in the region. In addition, the size of the Chiffa catchment (316 km2) is smaller than one entire RCM grid cell of CORDEX-Africa (50 km × 50 km = 2,500 km2). This presents a challenge for using climate model simulations, as they are not designed to capture features at spatial scales smaller than their grid resolution (Dakhlaoui and Djebbi, 2021). Applying a bias correction technique could help downscale climate model outputs to align with the study catchment scale (Hakala et al., 2018; Djebbi and Dakhlaoui, 2023).
The aim of this study is to evaluate some bias correction methods for the improvement of hydrological simulations in the Chiffa watershed. Specifically, we investigate how different bias correction methods can better represent the precipitation patterns and hydrological processes of this semi-arid Mediterranean basin. For this purpose, the precipitation from the Rossby Centre regional atmospheric model RCA4 of the Coordinated Regional Climate Downscaling Experiment (Cordex-Africa) forced by two global circulation models (MPI-ESM-LR and CRNM-CM5) was corrected by two bias correction methods, “Quantile mapping (QM)” and “Delta change (DC),” before being used as input data to the GR2M hydrological model to assess the impact of climate change on the hydrological response of the Chiffa basin by 2100, under two Representative Concentration Pathways (RCP): RCP 4.5 and RCP 8.5.
The Chiffa basin is one of the three sub-watersheds of the Mazafran, the most important coastal basin in Algiers. The Chiffa basin is located in northern Algeria between latitudes of 36° 10′ and 36°30’N and longitudes of 2° 30′ and 3° East. It is bounded to the north by Blida, to the west by Hadjout and Djendel, and to the south by Medea (Figure 1). It has an area of approximately 316 km2 at the Amont des Gorges hydrometric station, with a relatively elongated shape. It has an average altitude of 833 m, with a highest point of 1,629 m.
The Chiffa basin presents a complex geological structure (Figure 1) dominated by Triassic and Cretaceous formations in its central part, with Jurassic and lower Cretaceous deposits in the northern section, while Tertiary outcrops are present in the southern area. This geological diversity significantly influences the basin’s hydrogeological behavior, particularly in terms of groundwater storage and surface water-groundwater interactions.
The study area is characterized by a Mediterranean vegetation cover, including various forest formations. These formations are mainly composed of Atlas cedars, Aleppo pines, and Berberis Tuyas (A.P.N.A., 2006). The Chiffa basin belongs to a semi-arid Mediterranean climate with a warm season.
The interannual variability of precipitation in the Chiffa basin is indeed significant (Figure 2). Over the period 1979–2014, annual precipitation varies considerably, ranging from around 400 mm to nearly 1,100 mm, which highlights substantial interannual variability of precipitation. Such variability directly impacts water availability and increases the risk of drought episodes and flood events. On average, the basin receives around 800 mm of annual precipitation, which is characteristic of semi-arid Mediterranean climates. These interannual fluctuations are essential for assessing the basin’s resilience to future climate scenarios and underscore the importance of water resource management strategies adapted to extreme conditions.
The average temperature for the reference period (1979–2014) is around 17°C, characterized by marked seasonal contrasts, with hot summers and mild winters contributing to high evapotranspiration rates during the warm season. Additionally, Northern Africa, including the Chiffa basin, has been identified as one of the most vulnerable “hot spots” to climate change. Projections indicate that the region will experience a significant warming trend, with temperatures expected to rise by 0.5°C–2.5°C by the end of the century under emission scenarios, leading to an increase in hydrological drought (IPCC, 2021).
The hydroclimatic data used in this study correspond to the El Hamdania rain gauge and the Amont des Gorges hydrometric gauging station that were collected from the National Water Resources Agency (ANRH). The temperature data correspond to the climate station of Dar el Beida of the National Office of Meteorology (ONM). All hydro-climatic data are available on a monthly scale during the study period 1979–2014 (Table 1).
The estimation of the potential evapotranspiration (PET) over the historical and projection period is based on the Thornthwaite formula given (Thornthwaite and Mather, 1951) in Equation 1.
Where PET (m) is the mean evapotranspiration of month m (m = 1 to 12) in mm, T(m) is the interannual mean temperature of the month in °C and F (m, ϕ) is the corrective factor for month (m) and latitude, a: 0.016 * I + 0.5, I annual thermal index defined in Equation 2.
Drouiche et al. (2019) showed that the study area has experienced an increase in drought episodes characterized by a reduction in annual rainfall of about 16 to 24% during the period 1973–2001. Regarding the temperature, Bouderbala (2019) observed an increase in temperatures of approximately 6 to 30% in the study area between February and June during the period 1974–2010, highlighting potential consequences on the availability of water resources.
To estimate future flows in the study catchment, the projected monthly precipitation and temperature of the RCM-GCMs RCA4-MPI-ESM-LR and RCA4-CNRM-CM5 of the Africa-Cordex project are used. These two climate models were considered suitable for Algeria compared to other available models (Zeroual et al., 2019). Thus, these models offer simulations of climatic variables at a monthly scale during the period 1981–2100, with a horizontal resolution of about 50 km. Two Representative Concentration Pathways are considered: RCP 4.5 and RCP 8.5, which represent, respectively, an optimistic and a pessimistic greenhouse gas emission scenario.
In this study, the GR2M conceptual model (Perrin et al., 2007) was selected for simulating hydrological processes in the Chiffa watershed after careful consideration of several factors. Although we acknowledge that more complex models with finer temporal resolutions exist, GR2M’s selection was justified by multiple considerations. GR2M’s parsimonious structure, requiring only precipitation and PET as inputs, is particularly advantageous in our study context where data availability is limited. This parsimony, combined with its proven robustness, makes it especially suitable for climate change impact studies where the focus is on long-term hydrological changes rather than short-term events.
For our study focusing on long-term climate change impacts, the monthly resolution proves particularly relevant because it better aligns with the reliability of climate projections, whose uncertainties increase significantly at finer time scales (Lehner et al., 2020). This temporal scale also facilitates the better propagation of uncertainties in climate projections while remaining consistent with water resource management and planning scales.
The model has demonstrated remarkable performance in semi-arid Mediterranean contexts, particularly in northern Algeria. Previous studies have successfully applied GR2M to similar watersheds (Zeroual et al., 2013; Sakaa et al., 2015; Hallouz et al., 2018; Hadour et al., 2020; Pulido-Velazquez et al., 2021; Bouguerra and Mansour, 2023; Mahdaoui et al., 2024), achieving satisfactory results in capturing the dominant hydrological processes at the monthly scale. Although we recognize that the monthly time step may not capture certain short-term events, such as flash floods (Bargaoui et al., 2008; Dakhlaoui et al., 2009), this temporal resolution aligns well with our study’s primary objective of assessing long-term climate change impacts on water resources.
The model structure consists of two main functions (production and routing) organized around two reservoirs. The production function operates through a soil reservoir, while the transfer function is governed by a gravitational water reservoir. The model also accounts for underground water exchange, which is particularly relevant in our semi-arid context where groundwater contributions can be significant. A detailed description of the model structure and equations is available in Perrin et al. (2007).
Model calibration and validation were performed under the R environment using the airGR package (Gader et al., 2020), which provides comprehensive outputs including monthly simulated flow series, numerical performance criteria, graphical outputs, and internal model variables. The calibration process focused on optimizing the model’s two parameters to best represent the watershed’s hydrological behavior, with particular attention to both high and low flow periods to ensure robust performance across different hydrological conditions.
The estimation of the performance of a hydrological model is one of the most important steps in evaluating the quality of the simulation. It requires a comparison between observed and simulated flows. There is a multitude of criteria to evaluate the performance of the hydrological model. Two performance criteria are used in this study: the Nash criterion (Nash and Sutcliffe, 1970) and Pearson’s correlation coefficient.
This criterion compares the mean square deviation of the flux roots to the variance it has given more precise results compared to the other evaluation criteria. This is confirmed by several studies such as Nounangnonhou et al. (2018), Fathi et al. (2019), Ditthakit et al. (2021a) and Orozco et al. (2021). This criterion is based on the sum of the square errors as defined in Equation 3.
Q0 observed flows, Qc simulated flows with the model, : average observed flow, N: number of observations.
Where:
If Nash ≤ 0: the model is no better than the average of the observed flows.
If Nash > 0: the model is better than the average of the observed flows.
If Nash = 1: the model corresponds perfectly to the observed flows.
The linear regression between the calculated and the observed flows, its formulation is as shown in Equation 4.
Where Q0 and QC are, respectively, the observed and simulated flows for i = 1…, N, N the number of inputs and are, respectively, the averages of the observed and simulated flows. The value (r) varies from −1 to 1. If (r) is positive and close to 1, the relationship between the measured flows and the flows calculated by the linear models is increasing, and the scatterplot is concentrated around the regression line, if (r) is negative and close to −1, indicates a perfect negative correlation between the values of observed and predicted flows (Koffi et al., 2011; Charifi, 2018; Ditthakit et al., 2021b).
The Kling-Gupta Efficiency (KGE), developed by Gupta et al. (2009), was designed to enhance the traditional Nash-Sutcliffe Efficiency (NSE) metric. KGE addresses certain limitations of NSE, particularly its sensitivity to data variability; its formulation is as follows in Equation 5:
where represents the observed flows, represents the simulated flows, the average of the observed flows, is the average of the simulated flows, R is the Pearson’s correlation coefficient, is the standard deviation of the observations, and is the standard deviation of the simulations.
With KGE, a value close to 1 indicates a high agreement between observed and simulated values, while values closer to 0 or negative indicate poor performance.
RMSE is calculated from the observation values and then averaged for all the simulations made with the different models. It measures the difference between simulations and observation flows. The formulation is given as shown in Equation 6:
With . is the simulated and observed flow, respectively. For i = 1…N; N is the total number of flow data over the analysis period.
Mostly, climate models have systematic errors in their output. Then, to evaluate the performance of regional climate models, a bias is estimated between simulated and observed rainfall data during the reference period (1981–2010) as shown in Equation 7.
Where, are, respectively, the average observed and simulated rainfall.
The coefficient of determination (R2) represents the proportion of variance in the dependent variable that is predictable from the independent variable(s). In hydrology, it measures how well the simulated flows match the observed flows as shown in Equation 8.
With N being the number of observations, for i = 1…N, represents the observed flows on day i, represents the simulated flows on day i, the average of the observed flows, and the average of the simulated flows.
The R2 values range from 0 to 1, where:
• R2 = 1 indicates a perfect correlation between observed and simulated flows.
• R2 = 0 indicates no correlation.
• Values above 0.5 generally indicate acceptable model performance.
The bias correction of climate data is a crucial step in assessing the impacts of climate change (Tan et al., 2020). In this study, we have carefully selected two widely used bias correction techniques: the Delta change (DC) method and the Quantile mapping (QM) method. This selection was based on their proven effectiveness in Mediterranean and semi-arid regions (Enayati et al., 2021; Taibi et al., 2021b; Dakhlaoui and Djebbi, 2021; Djebbi and Dakhlaoui, 2023), particularly for their distinct but complementary strengths in handling different aspects of climate data correction.
The DC method was selected for its robust approach to adjusting the observed climatic series based on mean monthly changes between reference and future RCM-GCM simulations (Ruelland et al., 2012). This method is particularly advantageous for preserving the temporal structure of observed data, which is important for hydrological impact studies in semi-arid regions. It effectively captures mean shifts in climate variables while maintaining the natural variability patterns present in observations, assuming that the regional bias remains constant over time (Beyer et al., 2019). In addition, the DC technique is recognized for its simplicity of application. The DC method is defined by Equations 9 and 10 (Mendez et al., 2020):
Where (t) and (t) are, respectively, the raw simulated and observed precipitation during the control period, (t) is the raw projected time series, is the bias-corrected projected time series, and μm is the long-term monthly mean.
The QM method was chosen as a complementary approach due to its efficacy in correcting the entire distribution of climate variables by aligning the cumulative distribution functions (CDFs) of observed and simulated data. This method is particularly valuable for correcting extreme values, which are critical in Mediterranean climates like the Chiffa basin, where precipitation patterns show high variability (Motlagh et al., 2022). The QM method is expressed by Equation 11 (Heo et al., 2019):
Where Pm(t) and Ps(t) are, respectively, the corrected and simulated precipitations and Fs and F0−1 are the cumulative distribution function (CDF) of the raw precipitation from the RCM and the inverse CDF of the observed precipitation.
Hydrological modeling performance was evaluated through calibration and validation steps, using multiple evaluation criteria to guarantee a robust assessment of the model’s capabilities. We employed four performance metrics: Nash-Sutcliffe Efficiency (NSE), Pearson’s correlation coefficient (R), Root Mean Square Error (RMSE), and Kling-Gupta Efficiency (KGE), each providing different insights into model performance.
The calibration period (January 1981–December 2000) encompasses various hydrological conditions, including several drought events characteristic of the Mediterranean climate (e.g., in the 1990s). During this period, the model achieved satisfactory performance with an NSE of 77.5% and R of 87.6% (Table 2, Figure 3A). The RMSE of 2.97 mm/month indicates a reasonable level of accuracy in flow prediction, whereas the KGE is 48%.
Figure 3. Hydrological simulation of the rainfall-runoff process using the GR2M model in the Chiffa basin during the calibration and validation periods.
For the validation period (January 2002–December 2012), using the calibrated parameters (X1 = 347.234 mm and X2 = 0.682 mm), the model showed notably high-performance metrics with an NSE of 99% and R of 99.8% (Table 2, Figure 3B). The RMSE improved to 1.01 mm/month, and the KGE increased to 60%.
The hydrological response presented in Figure 4 demonstrates that the model effectively captures the general flow dynamics driven by precipitation inputs, though with varying performance across different hydroclimatic conditions. The model’s performance during both wet and dry periods suggests its capability to simulate the watershed’s hydrological behavior under various climatic conditions.
Figure 4. Result of hydrological simulation rainfall-runoff of the GR2M model on the Chiffa basin during the (A) calibration period (1981–2000), and (B) validation period (2002–2012).
The performance of RCM-GCMs during the reference period (1981–2010) was assessed using multiple statistical metrics: bias, Root Mean Square Error (RMSE), and correlation coefficient (R) between observed and simulated precipitation data (Table 3).
Table 3. Estimated bias between observed and simulated monthly and annual rainfall over the reference period 1981–2010.
On the annual scale, results show that the two raw RCM-GCMs strongly underestimate precipitation. The bias between observations and RCA4-CNRM-CM5 and RCA4-MPI-ESM-LR is, respectively, about −57.03% and −55.92%. This substantial underestimation is further confirmed by high RMSE values of 2102.47 mm and 2061.47 mm, respectively, while the weak correlation coefficients (R = −0.14 and −0.25) indicate poor temporal agreement between simulated and observed precipitation patterns.
The representation of observed and simulated mean monthly precipitation during the control period (1981–2010) shows distinct seasonal patterns. During the wet season (October–May), both models exhibit significant biases, with maximum negative biases occurring in December and January (−71.45% and −66.03%, respectively, for RCA4-CNRM-CM5). These months also show the highest RMSE values (421.91 mm and 394.10 mm, respectively), indicating substantial uncertainty in wet season precipitation simulation. During the dry season (June–September), while relative biases are lower (e.g., −14.5% and −24.5% in June and July for RCA4-CNRM-CM5), the models still struggle to accurately capture the limited rainfall events characteristic of this period, as evidenced by the poor correlation coefficients.
The high bias obtained during the dry season can be explained by the low quantity of precipitation in this season. However, for the wet season, the precipitation simulated by the climate models depends on processes represented by each model. Indeed, some studies have highlighted that climate models generally fail to estimate rainfall correctly during wet periods (Taibi et al., 2019, 2022; Dunning et al., 2018). This is because the resolution of climate models makes them not able to consider more phenomena that occur at a spatial scale smaller than the grid size of climate models, such as convective precipitation and orographic rainfall, which are highly dependent on local relief (Ouatiki et al., 2019; Gu et al., 2022). The location of the Chiffa catchment at the Blidean Atlas downstream, which is characterized by its relief, could be one of the origins of such underestimation.
These significant biases and errors in precipitation simulation could substantially affect hydrological forecasts in the Chiffa basin, particularly affecting seasonal water availability predictions, extreme event forecasting, and long-term water resources planning. This is particularly crucial in the semi-arid context of the Chiffa basin, where accurate precipitation estimates are essential for reliable hydrological modeling. According to the results above, both RCM-GCMs, RCA4-CNRM-CM5, and RCA4-MPI-ESM-LR, cannot reproduce correctly rainfall at the Chiffa basin. A bias correction technique is then necessary to improve the rainfall data simulated by the climate models.
The performance assessment of temperature simulations was conducted using bias, RMSE, and correlation coefficient (R) at both annual and monthly scales (Table 4). At the annual scale, the temperature bias between observations and simulations data of RCA4-CNRM-CM5 and RCA4-MPI-ESM-LR over the reference period (1981–2010) was about −0.8°C and 0.1°C, respectively. The annual RMSE values (52.35°C for RCA4-CNRM-CM5 and 2.21°C for RCA4-MPI-ESM-LR) and correlation coefficients (R = −0.11 and −0.08, respectively) suggest that RCA4-MPI-ESM-LR shows better overall temperature simulation capability.
Table 4. Estimated bias between observed and simulated monthly and annual temperatures over the reference period 1981–2010.
At the monthly scale, results show that generally, temperature variation simulated by the two RCM-GCMs is almost similar.
The RCA4-CNRM-CM5 model shows good performance in winter months, reproducing exactly the monthly temperatures observed in December, January, and February (Bias = 0°C, RMSE <0.5°C). However, it underestimates temperatures during other months, with estimated biases ranging from −0.2°C to −2.2°C. The highest discrepancies are observed in June and October, with RMSE values of 10.48°C and 12.13°C, respectively, suggesting significant model uncertainty during these seasons.
The RCA4-MPI-ESM-LR model demonstrates more consistent performance across seasons. The analysis shows average biases of about 1°C in winter (December–January–February [DJF]), 0.2°C in spring (March–April–May [MAM]), 0.5°C in summer (June–July–August [JJA]), and −0.6°C in autumn (September–October–November [SON]). The RMSE values are lower compared to RCA4-CNRM-CM5, ranging from 0.05°C in July to 5.38°C in June, indicating better overall temperature simulation capability.
The correlation coefficients of both models exhibit weak to moderate correlations with observed temperatures, varying from −0.41 to 0.33, indicating limitations in capturing temperature variability patterns. This performance variability across seasons and models has important implications for hydrological modeling, particularly for processes sensitive to temperatures such as evapotranspiration and snowmelt in the elevated parts of the catchment. While the biases are smaller than precipitation, temperature corrections might still be necessary to ensure reliable hydrological simulations, especially during seasons with larger discrepancies.
The analysis of raw RCM-GCM outputs reveals significant systematic biases in both temperature and precipitation simulations across seasons (Figure 5).
Figure 5. Seasonal analysis of raw RCM-GCM (A) precipitations and (B) temperatures over the reference period (1981–2010).
The precipitation biases are even more substantial (Figure 5A), with both models significantly underestimating rainfall across all seasons. Winter shows the most severe underestimation (up to −60% for RCA4-MPI-ESM-LR), followed by spring (−40% to −50%), while summer and autumn maintain significant negative biases (−30% to −60%).
For temperature, RCA4-CNRM-CM5 shows a consistent cold bias (Figure 5B), particularly pronounced in winter (−1.5°C) and autumn (−1°C), while RCA4-MPI-ESM-LR exhibits a warm bias in spring (+1°C).
These systematic biases underscore the critical need for bias correction in the Chiffa basin context. The substantial precipitation underestimation would lead to unrealistic water availability estimates, and temperature biases would affect evapotranspiration calculations, both important components in semi-arid hydrological modeling. The magnitude of these biases varies seasonally, suggesting that their impact on hydrological responses would be pronounced.
The RCA4-CNRM-CM5 and RCA4-MPI-ESM-LR models provide projected precipitation until 2100 for two representative concentration pathways, RCP 4.5 and RCP 8.5. We propose an analysis of the change coefficient of future precipitation over the period 2070–2099 compared to the reference period 1981–2010, calculated at annual and seasonal scales. To highlight the efficiency of the bias correction methods (Quantile mapping and Delta change), a change coefficient was calculated for raw RCM-GCM and bias-corrected RCM-GCM by the two correction methods.
The raw RCA4-CNRM-CM5 model, at the annual scale, projects a decrease of precipitation of more than 60% under both scenarios (RCP 4.5 and RCP 8.5). However, after correcting the bias, the change coefficient of precipitation indicates a future decrease of precipitation that does not exceed 5 and 27% by 2100, respectively, under RCP 4.5 and RCP 8.5 (Figures 6A,B). The two bias correction methods (Quantile mapping and Delta change) reduced the projected change in precipitation by 33 to 55% compared to raw climate models.
Figure 6. Seasonal and annual projected change in precipitation of raw and bias-corrected (Quantile mapping and Delta change) climate model RCA4-CNRM-CM5 according to (A) RCP 4.5 and (B) RCP 8.5 over the period (2070–2099).
At the seasonal scale, the results show a significant future decrease in precipitation of more than 70% in winter (December–January–February [DJF]), according to RCP 4.5 (Figure 6A) and RCP 8.5 (Figure 6B). For the same season, the results from the rainfall corrected by the two bias correction methods—Quantile mapping and Delta change—indicate a future decrease in rainfall that does not exceed 30%, according to RCP 4.5 (Figure 6A), and 49%, according to RCP 8.5 (Figure 6B). For this season, the bias correction methods have favored a correction of error with a percentage of 40%.
In spring (March–April–May [MAM]), the results of the raw climate model show a decrease in precipitation of 49% under RCP 4.5 (Figure 6A) and 59% under RCP 8.5 (Figure 6B). Whereas, after the correction of this rainfall by the two bias correction methods, the results show an increase of the rainfall that exceeds 16%, according to RCP 4.5(Figure 6A), while the RCP 8.5 scenario shows a decrease of no more than 14% (Figure 6B), yet both methods show a correction performance that exceeds 40%.
For the summer season (June–July–August [JJA]), the raw rainfall over the projection period (2070–2099) indicates a decrease of 20%, according to RCP 4.5 and 45%, according to RCP 8.5, while all the results from the Quantile mapping and Delta change-corrected data show an increase in rainfall of more than 80%, according to RCP 4.5(Figure 6A) and RCP 8.5 (Figure 6B).
In autumn (September–October–December [SON]), bias correction methods reduced the climate predictions simulated by the raw RCM-GCM by 42%. Thus, a decrease of 4% is projected after bias correction for RCP 4.5 (Figure 6A) and 21% according to RCP 8.5 (Figure 6B).
The RCA4-MPI-ESM-LR model, RCP 4.5, shows a great decrease in rainfall, which exceeds 60% at the horizon of 2099 compared to the reference period 1981–2010, for the two RCPs (Figures 7A,B). The results obtained after the correction of the biases indicate a reduction in precipitation of about 20% according to RCP 4.5 (Figure 7A) and 34% according to RCP 8.5 (Figure 7B). These two methods have made it possible to reduce the simulated climatic change by 40% compared with the uncorrected future rainfall.
Figure 7. Seasonal and annual projected change in precipitation of raw and bias-corrected (Quantile mapping and Delta change) climate models. (A) RCA4-MPI-ESM-LR and (B) RCA4-MPI-ESM-LR according to RCP 4.5 and RCP 8.5 over the period (2070–2099).
At the seasonal scale, the projected precipitation with raw RCM-GCM for the period (2070–2099) shows a significant decrease for all seasons, particularly in winter (DJF) which registers a decrease of 61% according to RCP 4.5 (Figure 7A) and 70% according to RCP 8.5 (Figure 7B). After the correction of rainfall by the two bias correction methods (Quantile mapping and Delta change), the projected precipitation shows a decrease of 14% according to RCP 4.5 (Figure 7A) and 31% according to RCP 8.5 (Figure 7B) compared to the reference period. The two bias correction methods have allowed a reduction of 47% of the bias calculated before the correction.
In spring (MAM), the difference between observed and projected raw precipitation over the period (2070–2099) predicts a decrease of the order of 64% according to RCP 4.5 (Figure 7A) and 76% according to RCP 8.5 (Figure 7B). However, after using both bias correction methods, the results indicate a reduction in rainfall of about 20% under the RCP 4.5 scenario (Figure 7A) and 45% under the RCP 8.5 scenario (Figure 7B). Both methods reduced the bias of the RCA4-MPI-ESM-LR model outputs by 31 to 44%.
In summer (JJA), the estimated change in raw precipitation over the projection period shows a decrease of more than 60% under RCP 4.5 and 70% under RCP 8.5. After bias correction, the results show an increase in rainfall of more than 20% under both emission scenarios (Figures 7A,B).
In autumn (SON), the projected raw precipitation over the period (2070–2099) shows a decrease of 57% under the scenario RCP 4.5 (Figure 7A) and 73% under the scenario RCP 8.5 (Figure 7B). After the application of the bias correction methods, the change in precipitation shows a respective decrease of 4 and 37% according to RCP 4.5 and RCP 8.5, which present a respective correction of bias with percentages of 53 and 36%.
The projected potential evapotranspiration over the period 2070–2099 was obtained using the Thornwaite formula forced by the monthly bias-corrected projected temperature by the two RCM-GCMs.
At the annual scale, the projected PET presents an increase of about 10 to 32% according to RCP 4.5 and RCP 8.5 for the two climate models, compared to the reference period for the 2099 horizon (Figures 8A,B).
Figure 8. Seasonal and annual projected change in bias-corrected potential evapotranspiration according to (A) RCA4-CNRM-CM5 and (B) RCA4-MPI-ESM-LR, under RCP 4.5 and RCP 8.5 over the period (2070–2099).
At the seasonal scale, the two RCM-GCM (CNRM-CM5 and MPI-ESM-LR) present future change in PET and show an increase for the four seasons of the year over the 2099 horizon under RCP 4.5 and RCP 8.5 (Figures 8A,B).
The RCA4-CNRM-CM5 model shows an increase of PET during winter (DJF) of about 15 to 65%, respectively, for the RCP 4.5 and RCP 8.5 scenarios (Figure 8A). In spring (MAM), the change in PET exceeds +20% according to the two emission scenarios (Figure 8A), while in summer (JJA), the projected PET shows a little increase of 3% according to RCP 4.5 and 36% according to RCP 8.5 (Figure 8A). In autumn (SON), the RCA4-CNRM-CM5 model projects an increase in PET of about 13% according to RCP 4.5 and 27% according to RCP 8.5 (Figure 8A) by 2099.
The future evolution of PET simulated by the MPI-ESM-LR model in winter (DJF) shows an increase of 11% under RCP 4.5 and 17% under RCP 8.5 (Figure 8B). In spring (MAM), PET estimates a small increase of 9%, according to RCP 4.5, and 23% according to RCP 8.5 (Figure 8B). The summer (JJA) and autumn (SON) seasons are characterized by a high increase in PET by 2099, which varies between 12%, according to RCP 4.5and 38%, according to RCP 8.5 (Figure 8B).
The projected flows of the Chiffa basin were simulated using the GR2M hydrological model calibrated over the reference period 1981–2000 and forced by RCM-GCMs projected corrected precipitation and PET during the period 2070–2099. The results show that the projected change in mean annual and mean seasonal flows is almost identical from the two used raw RCM-GCM (Figures 9A, 10A) because the simulated precipitation and PET are so close. At the annual scale, both RCM-GCM project an increase in the mean annual flow of about 11% over the 2070–2099 projection period under RCP 4.5 and RCP 8.5 emission scenarios (Figures 9A, 10A), while the simulated future raw rainfall is decreasing (see section 3.3.1).
Figure 9. Seasonal and annual projected change in flow at the Chiffa catchment of (A) raw and (B) bias-corrected (Quantile mapping) climate model RCA4-CNRM-CM5 according to RCP 4.5 and RCP 8.5 over the period (2070–2099).
Figure 10. Seasonal and annual projected change in flow at the Chiffa catchment of (A) raw and (B) bias-corrected (Quantile mapping) climate model RCA4-MPI-ESM-LR according to RCP 4.5 and RCP 8.5 over the period 2070–2099.
At the seasonal scale, the two raw RCM-GCM predict an increase in runoff for three seasons—winter, spring, and summer—of about 4, 26, and 37%, respectively, according to RCP 4.5 and RCP 8.5 by 2099 (Figures 9A, 10A). In autumn (SON), the two raw climate models simulate a similar decrease in runoff of about 47% according to the two emission scenarios (Figures 9A, 10A). From these results, it is clear that the future evolution of flows (increasing trend) according to the raw climate model is consistent with the decreasing trend of precipitation projected by these two raw models (see section 3.3.1).
The projected evolution of annual flows simulated based on the bias correction by the Quantile mapping method, which is judged to be more relevant, indicates a decrease of 5 and 10% at the annual scale, respectively, according to the RCP 4.5 and RCP 8.5 emission scenarios by 2099, based on rainfall from the RCA4-CNRM-CM5 model (Figure 9B). At the seasonal scale, these RCM-GCM projects winter (DJF) a decrease of 9 and 13% of the mean seasonal flows, respectively, according to the two emission scenarios, RCP 4.5 and RCP 8.5 (Figure 9B).
In spring (MAM), the model indicates a little increase of 6% according to RCP 4.5 and no modification of the hydrological regime according to RCP 8.5 (Figure 9B). In summer (JJA), a decrease of 3 and 14% of the runoff is projected by the model under RCP 4.5 and RCP 8.5, respectively (Figure 9B). In autumn (SON), the results indicate a significant decrease in runoff of more than 50% under both RCP 4.5 and RCP 8.5 scenarios (Figure 9B), which is principally due to the significant decrease in runoff in October.
Concerning the RCA4-MPI-ESM-LR model at the annual scale, the projected flows at the horizon 2099 show an increase of 11% according to the RCP 4.5, whereas the RCP 8.5 predicts a decrease of 8% (Figure 10B). At the seasonal scale in winter (DJF), the model predicts a small increase of 5% according to RCP 4.5, while RCP 8.5 simulates a decrease of 14% (Figure 10B). In spring (MAM), the model projects an increase in runoff of 25% according to RCP 4.5 over the period 2070–2099; however, this increase is less important according to RCP 8.5 and does not exceed 5% (Figure 10B). In summer (JJA), RCP 4.5 shows an increase in runoff that exceeds 20%, while RCP 8.5 predicts a decrease of 20% (Figure 10B). For the autumn season, the model predicts a significant reduction in runoff of 46 and 69% respectively, according to RCP 4.5 and RCP 8.5 (Figure 10B).
The coefficient of determination (R2) results (Table 5) demonstrate the significant improvement in model performance achieved through bias correction using the Quantile mapping (QM) method. For the raw RCM-CORDEX models, R2 values ranged between 0.44 and 0.53, indicating moderate performance in simulating observed flows. However, after applying the QM bias correction method, the R2 values increased substantially, ranging from 0.83 to 0.97, showing excellent agreement between simulated and observed flows. The most notable improvement was the market for the RCA4-MPI-ESM-LR model under the RCP 4.5 scenario, where R2 increased from 0.45 to 0.97, followed by RCA4-CNRM-CM5 under the RCP 4.5 with an improvement from 0.53 to 0.95. These results demonstrate the effectiveness of the QM bias correction method in enhancing the reliability of flow projections from RCM-CORDEX models.
Table 5. The results of the coefficient of determination (R2) of raw and corrected (QM) flows from RCM-Cordex models.
The performance of the GR2M model on the Chiffa basin showed that it was able to reproduce the hydrological response adequately during both calibration and validation periods. This result was in harmony with several previous studies showing successful application of this model in Algerian catchments such as the Kebir Rhumul basin in Eastern Algeria, where a good simulation of flow by the GR2M with a Nash criterion higher than 0.80 is obtained (Sakaa et al., 2015). Hadour et al. (2020) report results showing the high performance of the GR2M model in the Chellif basin (northern Algeria), with a Nash value of 73.6 and 75.8% estimated, respectively, in the calibration and validation periods. In the Mitidja basin (northern Algeria), Hallouz et al. (2018) showed satisfactory results in the validation of the GR2M with a Nash value higher than 60%. The results obtained in this study and previous ones could confirm GR2M as a suitable model for hydrological application in this region of the world.
A notable finding was the model’s enhanced performance during the validation period compared to the calibration period (Nash values increasing from 77.5 to 99%). This improved performance can be attributed to the more humid hydrological regime during the validation period compared to the calibration period (1981–2000) and improved data quality. This behavior aligns with previous research demonstrating that rainfall-runoff models calibrated under dry conditions often show improved performance during wetter validation periods (Dakhlaoui et al., 2020; Coron et al., 2012). This pattern suggests the model parameters are robust rather than overfitted to specific climatic conditions, enhancing confidence in its application for future projections.
Overall, the RCA4-CNRM-CM5 and RCA4-MPI-ESM-LR models reproduce correctly the seasonality of temperature over the Chiffa basin during the reference period, which was confirmed by several previous studies that found that temperature does not have a significant spatial variability, and climate models are generally able to reproduce it correctly over the study area and over Algeria (Meddi and Meddi, 2009; Taibi et al., 2013; Bessaklia et al., 2018; Drouiche et al., 2019; Taibi et al., 2019).
For the RCA4-CNRM-CM5 model, the two bias correction methods give similar results in terms of future changes in precipitation. Similar results were found in several previous studies that have compared different methods of bias correction (e.g., Obada et al., 2016; Priyanko et al., 2022).
A detailed comparison of the two bias correction methods revealed distinctive patterns in their effectiveness for precipitation adjustment. For the RCA4-CNRM-CM5 model, both Quantile mapping and Delta methods showed comparable performance, with similar precipitation change patterns.
The Quantile mapping method demonstrated significant bias reduction capabilities, particularly in representing the full range of precipitation distribution, which is important for our catchment-scale analysis. This effectiveness was evidenced in other regions, such as Vietnam, where bias reductions of 3 to 45% were achieved in the regional model (RegCM) of the Cordex-SEA project. This project was piloted by five global climate models (CNRM-CM5, MPI-ESM-MR, EC-Earth, CSIRO, and GFDL-ESM2M) of the CMIP5 phase during the period (2046–2065) (Trinh-Tuan et al., 2018). In the Volta basin, where biases were reduced by −9 to 5% for the precipitation simulated by the raw data under the four Cordex–Africa project models of the RCA4 model forced by four global circulation models (MPI-ESM, CNRM-CM5, HadGEM2-ES, and CCLM4) at the level of the Volta basin (West Africa) on the horizon 2080 (Yeboah et al., 2022).
For the RCA4-MPI-ESM-LR model, the Delta method always shows a greater reduction of the change in precipitation, which is confirmed by previous studies such as Taibi et al. (2021b). They compared two bias correction methods (Quantile mapping and Delta method) on the evolution of precipitation simulated by two models (CNRM-CM5 and MPI-ESM-MR) of the Cordex-Africa project at the level of the Oran coastal basin during the projection period (2075–2099). Their results indicate that the Delta method delivered less biased results of −27% and −47% for the RCA4-MPI-ESM-LR model. Miralha et al. (2021) also found that the Delta method outperformed the other bias correction techniques used in this study (Delta, Linear empirical, Quantile mapping, and Quantile Delta mapping), and was applied to bias correct the four CMIP5 project models (CCSM4, CNRM-CM5, IPSL-CM5A-MR, and MPI-ESM-MR) over the period (2046–2065) on the Maumee River in the United States (Giuntoli et al., 2018).
In our study, the Quantile mapping method was particularly relevant given that the Oued Chiffa catchment (315.68 km2) is smaller than one RCM grid cell of Cordex-Africa (~50 km × 50 km = 2,500 km2). This method effectively provided additional statistical downscaling of the RCM output to fit the catchment scale, complementing the dynamical downscaling from the GCM (horizontal grid ~150 km × 150 km) to the RCM grid (~50 km × 50 km), this could explain the important reduction in precipitation change in bias-corrected RCM-GCM compared to raw simulation.
However, we recognize that hydrological modeling in the Chiffa catchment is challenging since RCM-GCM is not designed to represent precipitation features at a spatial scale smaller than their grid (Hakala et al., 2018).
Any bias correction method has limitations. The two bias correction techniques used in this study were univariate ones, which imply temperature and precipitation and have been corrected separately. The technique’s limitation is that they do not take into consideration the intervariable dependence between climate variables. We propose the use, in future studies, more sophisticated techniques, such as multivariate bias correction (Cannon et al., 2020), that take into consideration intervariable dependence.
The results of hydro-climatic projection over the Chiffa catchment confirm results obtained by previous studies with different and/or earlier generations of climate models. For the precipitation, the overall results obtained on the future evolution of precipitation are concordant with previous studies in the Mediterranean region, including the Chiffa catchment (Zanis et al., 2008; Coppola and Giorgi, 2010; Ceglar et al., 2014; Babaousmail et al., 2022). Schilling et al. (2020) reported about a −20% to −40% decrease in projected mean precipitation over most parts of the Mediterranean region by the end of the 21st century, especially in Algeria and Morocco. Indeed, Driouech et al. (2020) showed that the Mediterranean area would experience a significant drying up by the end of the 21st century according to RCP 8.5, particularly over the western part of the MENA region, including Algeria. Their results indicate a reduction in total precipitation amounts ranging from −5% to −20% and exceeding −40% in the west of the Atlas Mountains.
For PET, results in the present study show an important increase in the evaporative demand for all seasons, which is in concordance with other studies already done in the Mediterranean basin. Indeed, Aubé (2016) found a 20% increase in PTE in the Rhone Mediterranean and Corsica basin by 2046–2065. Acharki et al. (2019) also showed that the autumn season is characterized by a high increase in evapotranspiration, which is about 60% over the projection period (2021–2050) in northern Morocco. In Algeria, Hadour et al. (2020) highlighted a 35 mm increase in PET by the end of the 21st century for the four seasons of the year in some basins of northwestern Algeria. Taibi et al. (2021a) found an increase in future PET that exceeds 30%, particularly in summer by 2100 in the Ain Dalia catchment located in eastern Algeria.
We recognize that the projected PET by a temperature-based formula as the Thornthwaite formula used in this study, could overestimate the projected increase in PET compared to a physically based formula such as Penman-Monteith that takes into consideration the change in the major physical variables leading to the evaporative demand (air temperature, vapor pressure, net radiation, and wind speed). The Thornthwaite formula’s primary limitation lies in its sole reliance on temperature, which may not fully capture the complex interactions between climate variables affecting evapotranspiration, particularly in a changing climate context. This simplification could lead to potential biases in extreme seasons; especially during summer when other factors like wind speed and relative humidity play an important role in the evaporative process. However, several studies showed the low sensitivity of hydrological projection to PET formulation, especially for semi-arid regions (Oudin et al., 2005; Dakhlaoui et al., 2020), which is the case of our study catchment. The seasonal analysis of flow reduction indicates a more pronounced decrease, which is consistent with the projected decrease in precipitation. This seasonal pattern of flow reduction has significant implications for water resource management, particularly for irrigation planning and reservoir operation during the dry season.
For flows, the overall results obtained in this study show a decrease in flows by the end of the 21st century in Oued Chiffa. This reduction shows significant seasonal variations, with more pronounced decreases during the wet season (October–May) compared to the dry season. These seasonal patterns have important implications for water resource management, particularly regarding storage requirements. This projected decrease is in agreement with several studies conducted in several regions of the Mediterranean basin. For example, Nerantzaki et al. (2019) found a 24.2% decrease in flows in Greece by 2100. In Italy, the study by Perra et al. (2018) showed a 31% reduction in flows over the period (2041–2070) in the Rio Mannu catchment in southern Sardinia, while in France, on the Hérault River located in the south of the region that flows into the Mediterranean Sea at Agde, models predict a 7% decrease in flows by 2085. This observed impact is frequently recorded in the Mediterranean basin due to the sensitive vulnerability of this region to variations in rainfall and drier, warmer conditions (Coppens et al., 2020).
The hydrological simulation and projection in Oued Chifa were performed over a monthly time step; however, northern Algeria is well known for its torrential pluvial events. These extreme precipitation events are particularly challenging to model as they can generate significant flash floods and represent a substantial portion of the annual water balance in semi-arid regions. Additionally, it is important to consider resilient precipitation events that persist despite overall trends toward drier conditions, as they can also significantly contribute to the hydrological cycle.
Climate change is likely to affect not only the frequency but also the intensity of these events, potentially leading to more severe flooding episodes despite an overall decrease in annual precipitation. We recognize that any future change in precipitation intensity could have a big impact on the hydrological response and affect the proposed hydrological projection.
To better consider these complex interactions in future studies, we recommend implementing multivariate bias correction methods that can preserve the interdependence between different climate variables (such as temperature, precipitation, and humidity). These methods would be particularly relevant for semi-arid regions where the interaction between extreme and resilient precipitation events strongly influences the hydrological response. Furthermore, the use of high-resolution climate models coupled with event-based hydrological modeling could provide more accurate representations of intense precipitation events and their impacts on flash floods, which are important for water resource management and flood risk assessment in the region.
The objective of this study was to assess the impact of climate change on the flows of the Chiffa basin, which necessitates combining a hydrological model with climate model simulations. All the results obtained indicate a decrease in rainfall by 2099 at the scale of our study area.
Initial analysis of raw rainfall simulations from two RCM-GCMs from the Africa-Cordex project (RCA4-MPI-ESM-LR and RCA4-CNRM-CM5) during the reference period (1981–2010) indicates an important bias of 50%. This is primarily due to the models’ limitations in representing regional atmospheric processes and local topographic effects characteristic of the Mediterranean region, with particular difficulties in reproducing extreme precipitation events and interannual variability. They are crucial elements for reliable hydrological modeling. These limitations necessitated the implementation of bias correction techniques to improve the reliability of future projections.
Following an application of bias correction, it is found that the difference between the future simulated rainfall corrected by the two bias correction methods (Quantile mapping and Delta change) by 2099 is significantly reduced biases in rainfall simulations compared to the uncorrected rainfall. This is due to the corrected simulated rainfall achieving a closer alignment with observed precipitation patterns. We observed significant improvements in model performance, with the coefficient of determination (R2) increasing from 0.44–0.53 to 0.83–0.97, demonstrating a better representation of observed flow patterns. This indicates that the two bias correction methods used (Quantile mapping and Delta change) have proven to be effective in adjusting the seasonal and annual mean precipitation simulations (2074–2099) of the RCM (CNRM-CM5 and MPI-ESM-LR) to the observed precipitation values (1981–2010).
However, each correction method presented specific limitations: Quantile mapping occasionally introduced physical inconsistencies in the relationships between variables, while Delta change, although preserving temporal variability, may not fully capture changes in future precipitation distribution patterns. Despite these limitations, both methods substantially enhanced the model outputs’ reliability for future projections.
The projected flows from the bias-corrected RCM-GCM during the period (2070–2099) showed a decrease of 5 and 10%, respectively, for RCP 4.5 and RCP 8.5 compared to the reference period, with more pronounced reductions during the winter and autumn seasons. This difference between scenarios (5% vs. 10%) provides a quantifiable measure of the uncertainty in future climate projections.
The methodological uncertainty was also present in our bias correction approach, though it was significantly reduced through the application of multiple correction methods. The seasonal changes in flow patterns have significant implications for water resource management in the Chiffa basin. The projected decrease in winter and autumn flows could particularly impact agricultural activities, since these seasons are crucial for soil moisture recharge and winter crop irrigation. Urban water supply may experience increased pressure during these periods, potentially requiring adaptation measures such as improved storage capacity or demand management strategies. Furthermore, the decreased flows during these seasons could affect ecosystems, particularly for aquatic habitats and riparian vegetation that rely on seasonal flow patterns. These findings suggest the need for integrated water resource management strategies should consider both human water needs and environmental flow requirements.
Therefore, the results obtained in this study are in full agreement with previous studies, strengthening our confidence in our findings conducted at the scale of the North African and Mediterranean regions. For example, De Girolamo et al. (2022) indicate that climate model projections for the period 2030–2059 predict reductions in the mean annual flow of up to 21 and 39% for the Celone River (Southern Italy) under a Mediterranean climate. Similarly, the study by Madani et al. (2024) in North Africa projects flow reductions ranging from 35 to 43%, with more severe impacts under the RCP 8.5 scenario for the period 2069–2099 in the Oued Abid catchment (Northern Tunisia).
Based on our findings, we recommend several directions for future research and water management: the implementation of multivariate bias correction methods (Cannon et al., 2020) would better preserve inter-variable dependencies, particularly between temperature and precipitation; the utilization of higher-resolution climate data (≤10 km) would improve the representation of local topographic effects; and the application of ensemble modeling approaches using multiple GCMs, RCMs, and hydrological models would better characterize uncertainty ranges and provide more robust projections.
For practical water management, our results suggest the need for specific adaptation strategies, including modified reservoir operation rules, drought-resistant crop selection, and enhanced water conservation measures. These strategies should be designed to address the projected seasonal changes in water availability, especially the more pronounced reductions in winter and autumn flows.
In conclusion, this study strengthens the extensive evidence of climate change impacts on North Africa and the Mediterranean region, underscoring the necessity of robust methodological rigor in climate impact assessments. The demonstrated effectiveness of bias correction methods, coupled with a comprehensive understanding of their limitations, provide valuable insights for future climate impact studies. The alignment of our results with other regional studies strengthens confidence in our projections while acknowledging the continuing challenges of accurately representing local-scale processes.
The integration of more sophisticated modeling approaches, combined with robust uncertainty quantification and practical adaptation strategies, will be crucial for effective water resource management in the face of climate change.
In order to enhance the robustness of future climate impact assessments in the region, we strongly recommend the use of multiple climate models and bias correction methods to capture the complete range of uncertainties. This comprehensive approach, combined with detailed analyses of local conditions and specific adaptation requirements, will support better informed decision-making for sustainable water resource management in the Chiffa basin and similar Mediterranean watersheds.
The data analyzed in this study is subject to the following licenses/restrictions: The National Office of Meteorology (ONM) provides the in-situ data, and the Coordinated Regional Climate Research Study on Africa (CORDEX-Africa) provides the National Agency of Water Resources (ANRH). Projected data from the RCA4-MPI-ESM-LR and RCA4-CNRM-CM5 models. Requests to access these datasets should be directed to https://cordex.org/data-access/.
AM: Writing – original draft. TH: Conceptualization, Methodology, Supervision, Validation, Writing – review & editing. ST: Conceptualization, Methodology, Supervision, Validation, Writing – review & editing. HD: Validation, Writing – review & editing. MM: Supervision, Validation, Writing – review & editing.
The author(s) declare financial support was received for the research, authorship, and/or publication of this article. The author declare that the publication of this paper was supported by ResAllaince Project (101086600 ResAlliance HORIZON-CL6-2022-GOVERNANCE-01), the authors are grateful to the ResAlliance project for funding the article processing charge.
The authors wish to express their gratitude to the National Office of Meteorology (ONM) for providing the climate data as well as the National Agency of Water Resources (ANRH) for the rainfall and hydrometric data on which the analyses of this study are based. Our thanks go to the Regional Climate Downscaling Experiment (CORDEX-Africa). The authors are thankful to the National Research Institute for Rural Engineering, Water and Forestry (INRGREF) for its valuable support of this research.
The authors declare that the research was conducted in the absence of any commercial or financial relationships that could be construed as a potential conflict of interest.
The author(s) declare that no Generative AI was used in the creation of this manuscript.
All claims expressed in this article are solely those of the authors and do not necessarily represent those of their affiliated organizations, or those of the publisher, the editors and the reviewers. Any product that may be evaluated in this article, or claim that may be made by its manufacturer, is not guaranteed or endorsed by the publisher.
A.P.N.A. (2006). Atlas des parcs nationaux algériens (Direction Générale des forêts, Parc national de Théniet El Had. 98) (Atlas of Algerian National Parks (General Directorate of Forests, Theniet El Had National Park). 98).
Acharki, S., Amharref, M., El Halimi, R., and Bernoussi, A. S. (2019). Évaluation par approche statistique de l’impact des changements climatiques sur les ressources en eau: application au périmètre du Gharb (Maroc). J. Water Sci. 32, 291–315. doi: 10.7202/1067310ar
Alkama, R., Marchand, L., Ribes, A., and Decharme, B. (2013). Detection of global runoff changes: results from observations and CMIP5 experiments. Hydrol. Earth Syst. Sci. 17, 2967–2979. doi: 10.5194/hess-17-2967-2013
Al-Safi, H. I. J., Kazemi, H., and Sarukkalige, P. R. (2020). Comparative study of conceptual versus distributed hydrologic modelling to evaluate the impact of climate change on future runoff in unregulated catchments. J. Water Clim. Change 11, 341–366. doi: 10.2166/wcc.2019.180
Aubé, D. (2016). Impacts du changement climatique dans le domaine de l’eau Sur les bassins Rhône-Méditerranée et Corse Bilan actualisé des connaissances (Impacts of climate change in the field of water on the Rhône-Mediterranean and Corsica basins – updated knowledge report). Water & Knowledge, Rhône Mediterranean Corsica Water Agency, 114.
Babaousmail, H., Hou, R., Ayugi, B., Sian, L. K., Ojara, M., Mumo, R., et al. (2022). Future changes in mean and extreme precipitation over the Mediterranean and Sahara regions using Bias-corrected CMIP6 models. Int. J. Climatol. 42, 7280–7297. doi: 10.1002/joc.7644
Bargaoui, Z., Dakhlaoui, H., and Houcine, A. (2008). Modélisation Pluie-Débit et Classification Hydroclimatique. J. Water Sci. 2008, 123–125. doi: 10.7202/018468ar
Bessaklia, H., Ghenim, A. N., Megnounif, A., and Martin-Vide, J. (2018). Spatial variability of concentration and aggressiveness of precipitation in north-east of Algeria. J. Water Land Dev. 36, 3–15. doi: 10.2478/jwld-2018-0001
Beyer, R., Krapp, M., and Manica, A. (2019). A systematic comparison of bias correction methods for paleoclimate simulations. Clim. Past Discuss. 30, 1–23. doi: 10.5194/cp-2019-11
Bouderbala, A. (2019). The impact of climate change on groundwater resources in coastal aquifers: case of the alluvial aquifer of Mitidja in Algeria. Environ. Earth Sci. 78:698. doi: 10.1007/s12665-019-8702-5
Bouguerra, S. A., and Mansour, B. (2023). Rainfall-flow modeling using a global conceptual model: case of the Beni Bahdel watershed (northwest of Algeria). J. Water Manag. Model. 12, 3820–3838.
Cannon, A. J., Piani, C., and Sippel, S. (2020). “Bias correction of climate model output for impact models” in Climate extremes and their implications for impact and risk assessment. eds. J. Sillmann, S. Sippel, and S. Russo (Amsterdam, Netherlands: Elsevier), 77–104.
Ceglar, A., Honzak, L., Žagar, N., Skok, G., Žabkar, R., and Rakovec, J. (2014). Evaluation of precipitation in the ENSEMBLES regional climate models over the complex orography of Slovenia. Int. J. Climatol. 35, 2574–2591. doi: 10.1002/joc.4158
Charifi, S. (2018). Application du modèle hydrologique GR2M Sur les bassins versants de la Soummam et de l’Isser. Conf on implication of a "potato-green bean" mixed crop system on water and light use efficiencies at: WATMED 4-Algiers.
Chathuranika, I. M., Gunathilake, M. B., Azamathulla, H. M., and Rathnayake, U. (2022). Evaluation of future streamflow in the upper part of the Nilwala River basin (Sri Lanka) under climate change. Hydrology. 9:48. doi: 10.3390/hydrology9030048
Chelkeba, B. (2021). Performance assessment of six bias correction methods using observed and RCM data at upper awash basin, Oromia, Ethiopia. J. Water Clim. Change 13, 664–683. doi: 10.2166/wcc.2021.181
Coppens, J., Trolle, D., Jeppesen, E., and Beklioğlu, M. (2020). The impact of climate change on a Mediterranean shallow lake: insights based on catchment and lake modelling. Reg. Environ. Chang. 20:62. doi: 10.1007/s10113-020-01641-6
Coppola, E., and Giorgi, F. (2010). An assessment of temperature and precipitation change projections over Italy from recent global and regional climate model simulations. Int. J. Climatol. 30, 11–32. doi: 10.1002/joc.1867
Coron, L., Andréassian, V., Perrin, C., Lerat, J., Vaze, J., Bourqui, M., et al. (2012). Crash testing hydrological models in contrasted climate conditions: an experiment on 216 Australian catchments. Water Resour. Res. 48, 1–17. doi: 10.1029/2011WR011721
Dakhlaoui, H., Bargaoui, Z., and Bardossy, A. (2009). Comparaison de trois méthodes d'usage de la technique des voisins les plus proches en vue d'amélioration de la performance de l'algorithme SCE-UA appliqué pour le calage du modèle pluie-débit HBV. Hydroinformatics in Hydrology, Hydrogeology and Water Resources, IAHS Publ. 331, 139–153.
Dakhlaoui, H., and Djebbi, K. (2021). Evaluating the impact of rainfall-runoff model structural uncertainty on the hydrological rating of regional climate model simulations. J. Water Clim. Change. doi: 10.2166/wcc.2021.004
Dakhlaoui, H., Hakala, K., and Seibert, J. (2022). “Hydrological impacts of projected climate change on northern Tunisian headwater catchments—an ensemble approach addressing uncertainties” in Climate change in the Mediterranean and middle eastern region. Climate change management. eds. F. W. Leal and E. Manolas (Cham: Springer).
Dakhlaoui, H., Seibert, J., and Hakala, K. (2020). Sensitivity of discharge projections to potential evapotranspiration estimation in northern Tunisia. Reg. Environ. Chang. 20:34. doi: 10.1007/s10113-020-01615-8
De Girolamo, A. M., Barca, E., Leone, M., and Porto, A. L. (2022). Impact of long-term climate change on flow regime in a Mediterranean basin. J. Hydrol. Regn. Chang. 41:101061. doi: 10.1007/s10113-020-01641-6
Dimri, A. P. (2021). Bias correction demonstration in two of the Indian Himalayan river basins. J. Water Clim. Change 12, 1297–1309. doi: 10.2166/wcc.2020.119
Ditthakit, P., Pinthong, S., Salaeh, N., Binnui, F., Khwanchum, L., and Bao Pham, Q. (2021a). Using machine-learning methods for supporting GR2M model in runoff estimation in an ungauged basin. Sci. Rep. 11:19955. doi: 10.1038/s41598-021-99164-5
Ditthakit, P., Pinthong, S., Salaeh, N., Binnui, F., Khwanchum, L., Kuriqi, A., et al. (2021b). Performance evaluation of a two-parameters monthly rainfall-runoff model in the Southern Basin of Thailand. Water. 13:1226. doi: 10.3390/w13091226
Dixit, S., Tayyaba, S., and Jayakumar, K. V. (2021). Spatio-temporal variation and future risk assessment of projected drought events in the Godavari River basin using regional climate models. J. Water Clim. Change 12, 3240–3263. doi: 10.2166/wcc.2021.093
Djebbi, K., and Dakhlaoui, H. (2023). Evaluating regional climate model simulations at Wadi El Abid catchment (northeastern Tunisia) using HBV rainfall-runoff model. Arab. J. Geosci. 16:139. doi: 10.1007/s12517-022-11160-9
Driouech, F., ElRhaz, K., Moufouma-Okia, W., Arjdal, K., and Balhane, S. (2020). Assessing future changes of climate extreme events in the CORDEX-MENA region using regional climate model ALADIN-climate. Earth Syst. Environ. 4, 477–492. doi: 10.1007/s41748-020-00169-3
Drouiche, A., Nezzal, F., and Djema, M. (2019). Variabilité interannuelle des précipitations dans la plaine de la Mitidja en Algérie du Nord (Interannual variability of precipitation in the Mitidja plain in Northern Algeria). J. Water Sci. 32, 165–177. doi: 10.7202/1065205ar
Dunning, C. M., Black, E., and Allan, R. P. (2018). Later wet seasons with more intense rainfall over Africa under future climate change. J. Clim. 31, 9719–9738. doi: 10.1175/JCLI-D-18-0102.1PP
Eekhout, J. P. C., and De Vente, J. (2018). The implications of bias-correction methods and climate model ensembles on soil erosion projections under climate change. Earth Surf. Process. Landf. 44, 1137–1147. doi: 10.1002/esp.4563
Emami, F., and Koch, M. (2018). Evaluation of statistical-downscaling/Bias-correction methods to predict hydrologic responses to climate change in the Zarrine River basin, Iran. Climate. 6:30. doi: 10.3390/cli6020030
Enayati, M., Bozorg-Haddad, O., Bazrafshan, J., Hejabi, S., and Chu, X. (2021). Bias correction capabilities of quantile mapping methods for rainfall and temperature variables. J. Water Clim. Change 12, 401–419. doi: 10.2166/wcc.2020.261
Fathi, M. M., Awadallah, A. G., Abdelbaki, A. M., and Haggag, M. (2019). A new Budyko framework extension using time series SARIMAX model. J. Hydrol. 570, 827–838. doi: 10.1016/j.jhydrol.2019.01.037
Foughali, A., Tramblay, Y., Bargaoui, Z., Carreau, J., and Ruelland, D. (2015). Hydrological modeling in northern Tunisia with regional climate model outputs: performance evaluation and Bias-correction in present climate conditions. Climate. 3, 459–473. doi: 10.3390/cli3030459
François, B., Vrac, M., Cannon, A. J., Robin, Y., and Allard, D. (2020). Multivariate bias corrections of climate simulations: which benefits for which losses? Earth Syst. Dynam. 11, 537–562. doi: 10.5194/esd-11-537-2020
Gader, K., Gara, A., Vanclooster, M., and Slimani, M. (2020). Quantification of predictive uncertainty in climate change context using airGRpackages: case of Medjerda catchment, Tunisia. 3rd Conference of the Arabian Journal of Geosciences (CAJG) In: Webinaire.
Giuntoli, I., Villarini, G., Prudhomme, C., and Hannah, D. M. (2018). Uncertainties in projected runoff over the conterminous United States. Clim. Chang. 150, 149–162. doi: 10.1007/s10584-018-2280-5
Gu, J., Liu, S., Zhou, Z., Chalov, S., and Qi, Z. (2022). Stacking ensemble learning model for monthly rainfall prediction in the Taihu Basin, China. Water. 14:492. doi: 10.3390/w14030492
Gupta, H. V., Kling, H., Yilmaz, K. K., and Martinez, G. F. (2009). Decom-position of the mean squared error and NSE performance criteria: implications for improving hydrological modelling. J. Hydrol. 377, 80–91. doi: 10.1016/j.jhydrol.2009.08.003
Hadour, A., Mahé, G., and Meddi, M. (2020). Watershed based hydrological evolution under climate change effect: an example from North Western Algeria. J. Hydrol. Reg. Stud. 28:100671. doi: 10.1016/j.ejrh.2020.100671
Hakala, K., Addor, N., and Seibert, J. (2018). Hydrological modeling to evaluate climate model simulations and their Bias correction. J. Hydrometeorol. 19, 1321–1337. doi: 10.1175/JHM-D-17-0189.1
Hallouz, F., Karahacane, H., Meddi, M., Mahé, G., Sadi, F., Benchikh, H., et al. (2018). “Impact of climate variability on hydrology of the Western Mitidja watershed, Algeria” in Recent advances in environmental science from the Euro-Mediterranean and surrounding regions. EMCEI. Advances in science, Technology & Innovation. eds. A. Kallel, M. Ksibi, H. Ben Dhia, and N. Khélifi (Cham: Springer), 753–754.
Heo, J. H., Ahn, H., Shin, J. Y., Kjeldsen, T. R., and Jeong, C. (2019). Probability distributions for a quantile mapping technique for a Bias correction of precipitation data: a case study to precipitation data under climate change. Water. 11:1475. doi: 10.3390/w11071475
Ibrahim, B., Karambiri, H., and Polcher, J. (2015). Hydrological impacts of the changes in simulated rainfall fields on Nakanbe Basin in Burkina Faso. Climate. 3, 442–458. doi: 10.3390/cli3030442
IPCC (2019). “Summary for policymakers” in Climate change and land: an IPCC special report on climate change, desertification, land degradation, sustainable land management, food security, and greenhouse gas fluxes in terrestrial ecosystems. eds. V. P. Masson-Delmotte, A. Zhai, S. L. Pirani, C. Connors, S. Péan, and N. Berger, et al. (Geneva: IPCC).
IPCC (2021). “Weather and climate extreme events in a changing climate” in Climate change 2021: The physical science basis. Contribution of working group I to the sixth assessment report of the intergovernmental panel on climate change. eds. V. Masson-Delmotte, P. M. Zhai, and A. Pirani, et al. (Cambridge and New York: Cambridge University Press), 1513–1766.
Koffi, Y. B., Ayral, P. A., Kouassi, A. M., Johannet, A., and Biemi, J. (2011). Modélisation des débits des rivières en région tropicale humide: application des réseaux de neurones et du modèle Gr2m au bandama blanc (côte d’ivoire) (Modeling of rivers flow in tropical humid area: application of neural networks and the model gr2m to the Bandama Blanc River (Côte d’Ivoire)). Rev. Ivoir. Sci. Technol. 17, 151–171. doi: 10.4000/physio-geo.940
Lehner, F., Deser, C., Maher, N., Marotzke, J., Fischer, E. M., Brunner, L., et al. (2020). Partitioning climate projection uncertainty with multiple large ensembles and CMIP5/6. Earth Syst. Dynam. 11:491508. doi: 10.5194/esd-11-491-2020
Macias, D., Garcia-Gorriz, E., Dosio, A., Stips, A., and Keuler, K. (2018). Obtaining the correct sea surface temperature: bias correction of regional climate model data for the Mediterranean Sea. Clim. Dyn. 51, 1095–1117. doi: 10.1007/s00382-016-3049-z
Madani, A. Z., Hermassi, T., Taibi, S., Boudabbous, K., and Mechergui, M. (2024). Climate change impacts on the hydrological behaviour of watershed in northeastern Tunisia (Oued El Abid watershed). GSC Advanc. Res. Rev. 20, 175–186. doi: 10.30574/gscarr.2024.20.3.0350
Mahdaoui, K., Chafiq, T., Asmlal, L., and Tahiri, M. (2024). Assessing hydrological response to future climate change in the Bouregreg watershed, Morocco. Sci. African 23:e02046. doi: 10.1016/j.sciaf.2023.e02046
Maraun, D. (2016). Bias correcting climate change simulations – a critical review. Curr. Clim. Chang. Rep. 2, 211–220. doi: 10.1007/s40641-016-0050-x
Marcos, R., Llasat, M. C., Quintana-Seguí, P., and Turco, M. (2018). Use of bias correction techniques to improve seasonal forecasts for reservoirs – a case study in northwestern Mediterranean. Sci. Total Environ. 610, 64–74. doi: 10.1016/j.scitotenv.2017.08.010
Martins, J., Fraga, H., Fonseca, A., and Santos, J. A. (2021). Climate projections for precipitation and temperature indicators in the Douro wine region: the importance of Bias correction. Agronomy 11:990. doi: 10.3390/agronomy11050990
Meddi, H., and Meddi, M. (2009). Variabilité des précipitations annuelles du Nord-Ouest de l’Algérie (Variability of annual rainfall in North-West Algeria). Sci. Planet. Change / Drought. 20, 57–65. doi: 10.1684/sec.2009.0169
Mendez, M., Maathuis, B., Hein-Griggs, D., and Alvarado-Gamboa, L. F. (2020). Performance evaluation of Bias correction methods for climate change monthly precipitation projections over Costa Rica. Water 12:482. doi: 10.3390/w12020482
Mengistu, A. G., Woldesenbet, T. A., and Dile, Y. T. (2021). Evaluation of the performance of bias-corrected CORDEX regional climate models in reproducing Baro–Akobo basin climate. Theor. Appl. Climatol. 144, 751–767. doi: 10.1007/s00704-021-03552-w
Mesta, B., and Kentel, E. (2021). Superensembles of raw and bias-adjusted regional climate models for Mediterranean region, Turkey. Int. J. Climatol. 42, 2566–2585. doi: 10.1002/joc.7381
Miralha, L., Muenich, R. L., Scavia, D., Wells, K., Steiner, A. L., Kalcic, M., et al. (2021). Bias correction of climate model outputs influences watershed model nutrient load predictions. Sci. Total Environ. 759:143039. doi: 10.1016/j.scitotenv.2020.143039
Motlagh, S. B., Honarbakhsh, A., Azizian, A., et al. (2022). Bias correction performance of global precipitation forecasting systems in Poldokhtar watershed in Iran. Res. Sq. 1–26. doi: 10.21203/rs.3.rs-1644511/v1
Nash, J. E., and Sutcliffe, J. V. (1970). River flow forecasting through conceptual models part I—A discussion of principles. J. Hydrol. 10, 282–290. doi: 10.1016/0022-1694(70)90255-6
Nerantzaki, S. D., Efstathiou, D., Giannakis, G. V., Kritsotakis, M., Grillakis, M. G., Koutroulis, A. G., et al. (2019). Climate change impact on the hydrological budget of a large Mediterranean island. Hydrol. Sci. J. 64, 1190–1203. doi: 10.1080/02626667.2019.1630741
Nounangnonhou, T. C., Fifatin, F. X., Lokonon, B. E., Acakpovi, A., and Sanya, E. A. (2018). Modelling and prediction of ouémé (Benin) river flows by 2040 based on gr2m approach. LARHYSS J. 33, 71–91.
Obada, E., Adéchina, A., Zandagba, J., Biao, E., Amédée, C., and Abel, A. (2016). Comparative study of seven bias correction methods applied to three regional climate models in Mekrou catchment (Benin, West Africa). Int. J. Curr. Eng. Technol. 6, 1831–1840. doi: 10.1016/j.ejrh.2021.100937
Orozco, J. C. C., Aranzana, M. F. G., and Hurtado, S. S. (2021). Hydrological modeling of high jungle area sub-basin, using the GR2M, Temez and Lutz Scholtz models. Congreso Internacional de Innovación y Tendencias en Ingeniería (CONIITI), 1–6. doi: 10.1109/CONIITI53815.2021.9619714
Oruc, S. (2022). Performance of bias corrected monthly CMIP6 climate projections with different reference period data in Turkey. Acta Geophys. 70:777. doi: 10.1007/s11600-022-00731-9
Ouatiki, H., Boudhar, A., Ouhinou, A., Arioua, A., Hssaisoune, M., Bouamri, H., et al. (2019). Trend analysis of rainfall and drought over the Oum Er-Rbia River basin in Morocco during 1970–2010. Arab. J. Geosci. 12:128. doi: 10.1007/s12517-019-4300-9
Oudin, L., Hervieu, F., Michel, C., Perrin, C., Andréassian, V., Anctil, F., et al. (2005). Which potential evapotranspiration input for a lumped rainfall-runoff model? Part 2: towards a simple and efficient potential evapotranspiration model for rainfall-runoff modelling. J. Hydrol. 303, 290–306. doi: 10.1016/j.jhydrol.2004.08.026
Ouhamdouche, S., Bahir, M., Carreira, P. M., and Zouari, K. (2018). Climate change impact on future flows in semi-arid environment, case of Essaouira Basin (Morocco). In: H. Chaminé, M. Barbieri, O. Kisi, M. Chen, and B. Merkel (eds) Advances in Sustainable and Environmental Hydrology, Hydrogeology, Hydrochemistry and Water Resources. Conference of the Arabian Journal of Geosciences, Advances in Science, Technology & Innovation. Springer, Cham
Parthiban, L., and Amit, B. M. (2021). Statistical downscaling using principal component regression for climate change impact assessment at the Cauvery River basin. J. Water Clim. Change 12, 2314–2324. doi: 10.2166/wcc.2021.223
Pastén-Zapata, E., Jones, J. M., Moggridge, H., and Widmann, M. (2020). Evaluation of the performance of euro-CORDEX regional climate models for assessing hydrological climate change impacts in Great Britain: a comparison of different spatial resolutions and quantile mapping bias correction methods. J. Hydrol. 584:124653. doi: 10.1016/j.jhydrol.2020.124653
Perra, E., Piras, M., Deidda, R., Paniconi, C., Mascaro, G., Vivoni, E. R., et al. (2018). Multimodel assessment of climate change-induced hydrologic impacts for a Mediterranean catchment. Hydrol. Earth Syst. Sci. 22, 4125–4143. doi: 10.5194/hess-22-4125-2018
Perrin, C., Claude, M., and Vazken, A. (2007). Modèles hydrologiques du génie rural (GR). Cemagref, UR Hydrosystèmes et Bioprocédés 16.
Priyanko, D., Zhenke, Z., and Hang, R. (2022). Evaluation of four bias correction methods and random forest model for climate change projection in the Mara River basin, East Africa. J. Water Clim. Change 13, 1900–1919. doi: 10.2166/wcc.2022.299
Pulido-Velazquez, D., Collados-Lara, A. J., Pérez-Sánchez, J., Segura-Méndez, F. J., and Senent-Aparicio, J. (2021). Climate change impacts on the streamflow in Spanish basins monitored under near-natural conditions. J. Hydrol. Reg. Stud. 38:100937. doi: 10.1016/j.ejrh.2021.100937
Rajab, H., Shiru, M. S., Shahid, S., Ismail, T., Bin Harun, S., Al-Ansari, N., et al. (2020). Precipitation projection using a CMIP5 GCM ensemble model: a regional investigation of Syria. Eng. Appl. Comput. Fluid Mech. 14, 90–106. doi: 10.1080/19942060.2019.1683076
Räty, O., Räisänen, J., Bosshard, T., and Donnelly, C. (2018). Intercomparison of univariate and joint Bias correction methods in changing climate from a hydrological perspective. Climate. 6:33. doi: 10.3390/cli6020033
Ruelland, D., Ardoin-Bardin, S., Collet, L., and Roucou, P. (2012). Simulating future trends in hydrological regime of a large Sudano-839 Sahelian catchment under climate change. J. Hydrol. 424–425, 207–216. doi: 10.1016/j.jhydrol.2012.01.002
Sakaa, B., Boulghobra, N., Chaffai, H., Hani, A., and Djabri, L. (2015). Application of GR2M for rainfall-runoff modeling in Kébir Rhumel watershed, north east of Algeria. World Appl. Sci. J. 33, 1623–1630. doi: 10.5829/idosi.wasj.2015.33.10.367
Satiprasad, S., Saumava, D., Anirban, D., Debsarkar, A., and Biswajeet, P. (2019). On projected hydrological scenarios under the influence of bias-corrected climatic variables and LULC. Ecol. Indic. 106, 1–17. doi: 10.1016/j.ecolind.2019.105440
Schilling, J., Hertig, E., Tramblay, Y., and Scheffran, J. (2020). Climate change vulnerability, water resources and social implications in North Africa. Reg. Environ. Chang. 20, 1–12. doi: 10.1007/s10113-020-01597-7
Schmidli, J., Frei, C., and Vidale, P. L. (2006). Downscaling from GCM precipitation: a benchmark for dynamical and statistical downscaling methods. Int. J. Climatol. 26, 679–689. doi: 10.1002/joc.1287
Shin, J. Y., Lee, T., Park, T., and Kim, S. (2019). Bias correction of RCM outputs using mixture distributions under multiple extreme weather influences. Theor. Appl. Climatol. 137, 201–216. doi: 10.1007/s00704-018-2585-3
Sidibe, M., Dieppois, B., Eden, J., Mahé, G., Paturel, J. E., Amoussou, E., et al. (2020). Near-term impacts of climate variability and change on hydrological systems in west and Central Africa. Clim. Dyn. 54, 2041–2070. doi: 10.1007/s00382-019-05102-7
Smitha, P. S., Narasimhan, B., Sudheer, K. P., and Annamalai, H. (2018). An improved bias correction method of daily rainfall data using a sliding window technique for climate change impact assessment. J. Hydrol. 556, 100–118. doi: 10.1016/j.jhydrol.2017.11.010
Soriano, E., Mediero, L., and Garijo, C. (2019). Selection of Bias correction methods to assess the impact of climate change on flood frequency curves. Water. 11:2266. doi: 10.3390/w11112266
Sorland, S., Schär, C., Lüthi, D., and Kjellström, E. (2018). Bias patterns and climate change signals in GCM-RCM model chains. Environ. Res. Lett. 13, 1–10. doi: 10.1088/1748-9326/aacc77
Szabó-Takács, B., Farda, A., Skalák, P., and Meitner, J. (2019). Influence of bias correction methods on simulated Köppen−Geiger climate zones in Europe. Climate. 7:18. doi: 10.3390/cli7020018
Taibi, S., Anza, F. Z. H., and Zeroual, S. (2021a). Etude de l’impact des changements climatiques sur la disponibilité des ressources en eau basée sur les simulations du modèle climatique régional RCA4: cas du bassin de Ain DALIA (Algérie). Algerian J. Environ. Sci. Technol. 7, 1860–1869.
Taibi, S., Boegh, E., Blyth, E., Hannah, D. M., Hisdal, H., Kunstmann, H., et al. (2013). “Evolution et régionalisation des précipitations au nord de l’Algérie (1936–2009)” in Climate and land surface changes in hydrology. eds. E. Boegh, E. Blyth, D. M. Hannah, H. Hisdal, H. Kunstmann, and B. Su, et al. (Wallingford: IAHS Publ. 359), 191–197.
Taibi, S., Meddi, M., and Mahe, G. (2019). Seasonal rainfall variability in the southern Mediterranean border: observations, regional model simulations and future climate projections. Atmosfera 32, 39–54. doi: 10.20937/ATM.2019.32.01.04
Taibi, S., Zeroual, A., and Meddi, M. (2022). Effect of autocorrelation on temporal trends in air-temperature in northern Algeria and links with teleconnections patterns. Theor. Appl. Climatol. 147, 959–984. doi: 10.1007/s00704-021-03862-z
Taibi, S., Zeroual, A., and Melhani, N. (2021b). Evaluation de deux méthodes de correction de biais des sorties de modèles climatiques régionaux Cordex-Africa pour la prévision des pluies: cas du bassin côtier oranais. Proc. IAHS. 384, 213–218. doi: 10.5194/piahs-384-213-2021
Tamene, D., and Chala, S. (2021). Assessment of the performance of CORDEX regional climate models in simulating rainfall and air temperature over Southwest Ethiopia. Heliyon. 7:e07791. doi: 10.1016/j.heliyon.2021.e07791
Tan, Y., Guzman, S. M., Dong, Z., and Tan, L. (2020). Selection of effective GCM Bias correction methods and evaluation of hydrological response under future climate scenarios. Climate. 8:108. doi: 10.3390/cli8100108
Teutschbein, C., and Seibert, J. (2010). Regional climate models for hydrological impact studies at the catchment scale: a review of recent modeling strategies. Geography 4, 834–860. doi: 10.1111/j.1749-8198.2010.00357.x
Teutschbein, C., and Seibert, J. (2012). Bias correction of regional climate model simulations for hydrological climate-change impact studies: review and evaluation of different methods. J. Hydrol. 456, 12–29. doi: 10.1016/j.jhydrol.2012.05.052
Thornthwaite, C. W., and Mather, J. R. (1951). The role of evapotranspiration in climate. Arch. Meteorol., Geophys. Bioklimatol., Ser. B 3, 16–39. doi: 10.1007/BF02242588
Todorovic, A., and Plavsic, J. (2016). The role of conceptual hydrologic model calibration in climate change impact on water resources assessment. J. Water Clim. Change 7, 16–28. doi: 10.2166/wcc.2015.086
Trinh-Tuan, L., Matsumoto, J., Tangang, F. T., Juneng, L., Cruz, F., Narisma, G., et al. (2018). Application of quantile mapping bias correction for mid-future precipitation projections over Vietnam. SOLA. 15, 1–6. doi: 10.2151/sola.2019-001
White, R. H., and Toumi, R. (2013). The limitations of bias correcting regional climate model inputs. Geophys. Res. Lett. 40, 2907–2912. doi: 10.1002/grl.50612
Willkofer, F., Schmid, F. J., Komischke, H., Korck, J., Braun, M., and Ludwig, R. (2018). The impact of bias correcting regional climate model results on hydrological indicators for Bavarian catchments. J. Hydrol. Reg. Stud. 19, 25–41. doi: 10.1016/j.ejrh.2018.06.010
Yeboah, K., Akpoti, K., Kabo-Bah, A., Ofosu, E., Siabi, E., Mortey, E., et al. (2022). Assessing climate change projections in the Volta Basin using the CORDEX-Africa climate simulations and statistical bias-correction. Environ. Challenges 6:100439. doi: 10.1016/j.envc.2021.100439
Zanis, P., Kapsomenakis, I., Philandras, C., Douvis, K., Nikolakis, D., Kanellopoulou, E., et al. (2008). Analysis of an ensemble of present day and future regional climate simulations for Greece. Int. J. Climatol. 29, 1614–1633. doi: 10.1002/joc.1809
Zeroual, A., Assani, A. A., Meddi, M., and Alkama, R. (2019). Assessment of climate change in Algeria from 1951 to 2098 using the Köppen–Geiger climate classification scheme. Clim. Dyn. 52, 227–243. doi: 10.1007/s00382-018-4128-0
Zeroual, A., Assani, A. A., Meddi, H., Bouabdelli, S., Zeroual, S., and Alkama, R. (2020). “Assessment of projected precipitations and temperatures change signals over Algeria based on regional climate model: RCA4 simulations” in Water resources in Algeria – part I. The handbook of environmental chemistry. eds. A. M. Negm, A. Bouderbala, H. Chenchouni, and D. Barceló, vol. 97 (Cham: Springer), 135–159.
Zeroual, A., Meddi, M., and Bensaad, S. (2013). “The impact of climate change on river flow in arid and semi-arid rivers in Algeria” in Climate and land surface changes in hydrology. eds. E. Boegh, et al. (Wallingford: International Association of Hydrological Sciences Publ. 359), 105–110.
Keywords: bias correction, climate change impacts, CORDEX-Africa, Delta change, GR2M, hydrological modelling, northern Algeria, Quantile mapping
Citation: Madani AZ, Hermassi T, Taibi S, Dakhlaoui H and Mechergui M (2024) Climate change impacts on the Chiffa basin (northern Algeria) using bias-corrected RCM data. Front. Water. 6:1507961. doi: 10.3389/frwa.2024.1507961
Received: 08 October 2024; Accepted: 27 November 2024;
Published: 17 December 2024.
Edited by:
Vikram Kumar, Planning and Development, Government of Bihar, IndiaReviewed by:
Francesco Viola, University of Cagliari, ItalyCopyright © 2024 Madani, Hermassi, Taibi, Dakhlaoui and Mechergui. This is an open-access article distributed under the terms of the Creative Commons Attribution License (CC BY). The use, distribution or reproduction in other forums is permitted, provided the original author(s) and the copyright owner(s) are credited and that the original publication in this journal is cited, in accordance with accepted academic practice. No use, distribution or reproduction is permitted which does not comply with these terms.
*Correspondence: Taoufik Hermassi, dGFvdWZpay5oZXJtYXNzaUBpbmdyZWYudWNhci50bg==
Disclaimer: All claims expressed in this article are solely those of the authors and do not necessarily represent those of their affiliated organizations, or those of the publisher, the editors and the reviewers. Any product that may be evaluated in this article or claim that may be made by its manufacturer is not guaranteed or endorsed by the publisher.
Research integrity at Frontiers
Learn more about the work of our research integrity team to safeguard the quality of each article we publish.