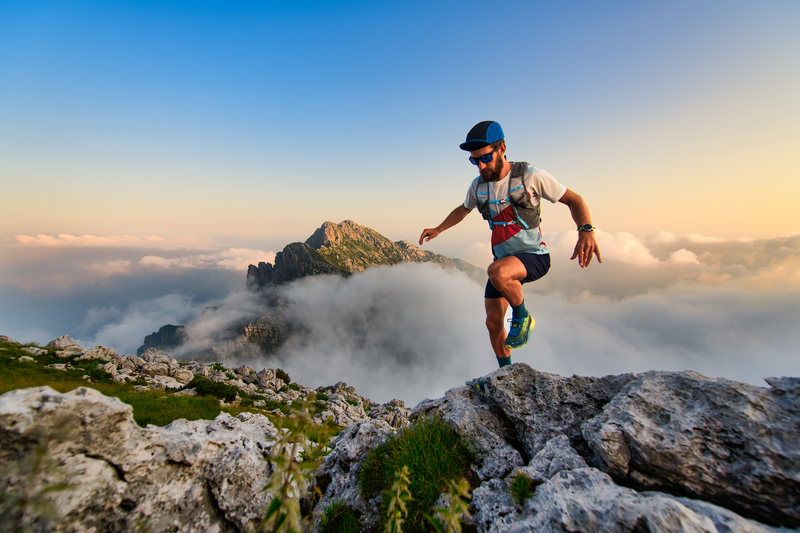
95% of researchers rate our articles as excellent or good
Learn more about the work of our research integrity team to safeguard the quality of each article we publish.
Find out more
ORIGINAL RESEARCH article
Front. Virtual Real. , 11 November 2024
Sec. Virtual Reality and Human Behaviour
Volume 5 - 2024 | https://doi.org/10.3389/frvir.2024.1252351
This article is part of the Research Topic Use of AR/MR/VR in the Context of Occupational Safety and Health View all 7 articles
In virtual reality (VR), we assessed how untrained participants searched for fire sources with the digital twin of a novel augmented reality (AR) device: a firefighter’s helmet equipped with a heat sensor and an integrated display indicating the heat distribution in its field of view. This was compared to the digital twin of a current state-of-the-art device, a handheld thermal imaging camera. The study had three aims: (i) compare the novel device to the current standard, (ii) demonstrate the usefulness of VR for developing AR devices, (iii) investigate visual search in a complex, realistic task free of visual context. Users detected fire sources faster with the thermal camera than with the helmet display. Responses in target-present trials were faster than in target-absent trials for both devices. Fire localization after detection was numerically faster and more accurate, in particular in the horizontal plane, for the helmet display than for the thermal camera. Search was strongly biased to start on the left-hand side of each room, reminiscent of pseudoneglect in scene viewing. Our study exemplifies how VR can be used to study vision in realistic settings, to foster the development of AR devices, and to obtain results relevant to basic science and applications alike.
We used a virtual reality (VR) simulation of smoke-filled rooms to evaluate the usefulness of two different devices for detecting and localizing fire sources: a handheld thermal imaging camera and a novel helmet-mounted heat display. The purpose of this study is threefold: (1) We test whether and how such displays can aid naïve, untrained observers (i.e., non-firefighters) in locating fires, notwithstanding the observation that search in real-world firefighting often has to rely on non-visual information (Lambert et al., 2021). (2) Our study shall exemplify how a realistic VR simulation (in terms of the visual input) can be used to quickly prototype and test a novel augmented reality (AR) device. (3) The obtained data shall provide new insights into visual-search strategies, when search spaces and tasks are complex and realistic, but at the same time unfamiliar to the specific participant. Consequently, we first briefly introduce the role of extended reality (XR; i.e., AR and VR) in search and rescue (Section 1.1), the use of XR in device development (Section 1.2), and the theoretical background on visual search without (Section 1.3) and with (Section 1.4) additional devices as well as on gaze in the real world and VR (Section 1.5). As it will become relevant for interpreting search strategies, we briefly review evidence for so-called pseudoneglect, a tendency to direct attention to the left when starting a new search (Section 1.6).
Firefighting and Urban Search and Rescue (USAR) are fields prominently associated with the potential benefits of XR. VR allows simulating environments that would be too dangerous for training in the real world, while AR devices are particularly useful when sensory information is impoverished through environmental conditions (e.g., low visibility in smoke, little useful auditory information due to noise, restricted tactile information due to protective equipment). While VR is frequently used in the context of firefighting training (e.g., Shi et al., 2021; Wheeler et al., 2021 for a recent review), AR devices are often associated with more general USAR operations (e.g., LaLone et al., 2019; Wang et al., 2018), where low visibility and other sensory impairments are less of an issue. While many tasks in the context of firefighting – e.g., searching for people and/or items under dangerous conditions, time pressure and impoverished sensory input – do come down to variants of search, in many cases, this search is non-visual, as operation happens under zero-visibility (http://www.firefighterrescuesurvey.com/ as cited by Lambert et al., 2021, states a number of 40.2% of victims being found in a zero-visibility environment). However, this does not necessarily imply that providing visual information through AR is useless in these cases – to the contrary, if no visual information is available from the actual environment, the visual modality may be particularly well-suited to provide task-relevant information by technical means. In fact, a current vision for a next-generation integrated AR system for firefighting support, which was developed using VR simulations with firefighters, relies on visual information to provide current information about the floorplan, location and condition of other team members, the environmental conditions, and dangers (Grandi et al., 2021).
It remains an open issue, which sensory channels AR devices should best use to support firefighting. While a definite answer might be highly dependent on the specific task and environmental conditions, in some cases, there is a noteworthy dissociation between signals that firefighters consider most useful, and those that objectively provide the necessary information most effectively and efficiently (Wolf et al., 2019). Along similar lines, using a multimodal (visual and tactile) AR support system was found to be preferred by experienced firefighters over having no such system available – even if it did not improve performance in the particular firefighting scenario tested (Streefkerk et al., 2012). Such dissociations between performance and preference – regardless of sensory modality – highlight that the design of any AR device needs to be validated by controlled experiments. To this end, VR provides a useful tool, as it allows rapid testing of devices under safe, yet realistic conditions – the approach we follow in the present study. The need for objective performance measures does, however, not invalidate the common approach of expert panels and focus groups to design AR support for firefighters (e.g., Kapalo et al., 2018); for the very least these will always be required to ensure the realism of the scenarios considered, especially in VR (e.g., Haskins et al., 2020).
Conceptually related to the aims of the present study, Bailie et al. (2016) used VR (in addition to structured interviews) to develop AR tools for firefighters. These authors stress the realism of their task, combining time pressure, low visibility, auditory noise and an auditory secondary task with a “blind” search task. Interestingly, these authors state that haptic and auditory channels quickly get overloaded, and therefore provide a visual aid (a virtual trace to mark previously searched locations) to facilitate search in (near-)zero visibility. In the present study, we focus on the realism of the visual stimulation, in particular on the physical realism of heat distributions and on visually faithful representations of the devices to be tested. Moreover, we perform a systematic test with a consistent trial structure (a series of independent, identical rooms) to quantify performance in a sample of 24 participants.
While most VR simulations in the context of hazardous situations address the training and support of firefighters or other Search and Rescue professionals, there are also recent approaches to use immersive VR as tool to train laypeople to evacuate buildings or aircrafts effectively and efficiently in case of fire (Feng et al., 2018 for a review). As the focus of the present study is on the efficient deployment of visual information, we also tested naïve, untrained observers to facilitate our initial development of the AR device, although its eventual target group will be firefighting professionals.
VR has become a common tool for the rapid development and testing of novel devices and device variants. This is most evident in the concept of the “digital twin,” a detailed digital copy of a real device, which – originating from military and aerospace applications (Glaessgen and Stargel, 2012) – is nowadays widely applied in manufacturing (Kritzinger et al., 2018 for a review), including the programming of industrial robots (Burghardt et al., 2020). The concept has been extended to a wide range of applications, including virtual versions of smart homes (Gopinath et al., 2019) and even humans, especially in the context of personalized healthcare (Kamel Boulos and Zhang, 2021). VR versions of devices and their user interfaces are also common in the assessment of usability and user experience (e.g., Brade et al., 2017; Hinricher et al., 2023). Using VR to prototype, develop and evaluate devices and their user interfaces extends to wearables, mobile devices and AR. Real mobile devices have also been integrated into VR simulations to test user interaction; for example, to develop and evaluate smartphone applications (Schrom-Feiertag et al., 2021). Lacoche et al. (2022) directly compared an actual AR device with its simulation in VR. In terms of usability and user experience, both the actual and the simulated device yielded comparable results and this was surprisingly robust to parameters like the AR device’s field of view or the environment as such. This shows that VR can be used to test AR devices and allows quick variations of the (simulated) device itself and of the environment it is used in. That is, VR simulations are a potentially useful tool for rapid prototyping and development of AR devices. In the present study, we follow this approach. We construct digital twins of the novel AR device (the helmet display) and the conventional device (the handheld thermal camera) and test them in a controlled, but visually realistic VR scenario on the detection and localization of fire sources.
While professional firefighters will rely on several modalities, and in particular will use tactile information to negotiate smoke-filled environments with zero visibility (Lambert et al., 2021), the present paradigm, locating fire sources, is most closely related to visual search. Visual search is one of the most widely studied paradigms in experimental psychology. Following Treisman and Gelade’s (1980) seminal work on their feature-integration theory (FIT), search tasks are often designed as a presence/absence judgement; that is, participants are asked to respond whether a certain item (target) is in the display or not. While being successful in predicting the search for simple items and their conjunctions as well as asymmetries between present and absent features, FIT does not readily extent to real-world search, as it assumes that items are well-individuated and it does not include a concept of similarity. Alternative models include the saliency map (Koch and Ullman, 1985), which considers maps of differences in features (contrasts) rather than of features as such, and Guided Search (Wolfe et al., 1989), which introduces concepts like feature tuning and explicit top-down guidance. Both models have since evolved to cover a much broader spectrum of tasks and stimuli. Later implementations of the saliency map (Itti et al., 1998; Itti and Koch, 2000) have become a standard reference for the prediction of spatial attention allocation in natural scenes, and the most recent version of Guided Search (GS6.0; Wolfe, 2021) covers advanced mechanisms such as explicit rules for search termination, pre-attentive processing of a natural scene’s gist (e.g., Fei-Fei et al., 2007), and visual working memory. Consequently, models of visual search and attentional guidance nowadays have the means to in principle accommodate complex real-world search scenarios.
When transferring search from simple stimuli and tasks to more and more naturalistic settings, many additional issues arise. These can refer to the target of search itself (e.g., how precisely is its appearance known and how is it presented; Alexander and Zelinsky, 2011; Schmidt and Zelinsky, 2009; Vickery et al., 2005) but also to the target’s context: objects in their correct context are typically found faster (Võ and Henderson, 2009) and search tends to start in regions consistent with typical target occurrence (Eckstein et al., 2006; Neider and Zelinsky, 2006; Torralba et al., 2006). In the present study, we use the firefighting scenario to induce a search task that is complex and from the real world, but nonetheless unfamiliar to the naïve observers. Hence, we can study complex visual search, when contextual (scene) information is unavailable.
In most real-world studies on visual search, “visual” refers to tasks where the signal of interest itself is visual in nature, i.e., largely unmodified as light meets the eye. However, many real-world applications require search in visual displays that are transformed from non-visual input. For example, luggage screening, a prime example for real-world search (Wolfe et al., 2013), uses images obtained by X-ray devices, which differ from naturalistic visual input first and foremost in that all objects in the scene are transparent (cf. Wolfe et al., 2005). The search in medical images is also often used as example for real-world applications of visual search, but there, too, images are obtained through complex transformations of sensory data and therefore break expectations regarding contextual information; consequently, searchers develop or are trained in domain-specific scanning strategies (e.g., Drew et al., 2013), which can reflect and are modulated by expertise (Manning et al., 2006). Even search in complex technical displays, for example, when operating machinery, another typical applied case (e.g., Kuschnereit et al., 2024), necessitates arrangements that are at best reminiscent of real-world scene layouts. While some results transfer from truly visual search to such scenarios, some assumptions, e.g., about contextual cues, are necessarily violated. Conversely, augmented reality (AR) displays often are designed to aid visual search (in a broad sense) in real-world scenarios. Studies relating AR to visual search have often considered technical constraints, such as the limited field of view (Trepkowski et al., 2019), or practical issues, like optimizing search cues to be informative but not distracting (Lu et al., 2012) or with respect to their type and location (Warden et al., 2022). Such studies, however, either need the AR display to be available or consider a question related to AR usage without the AR device as such. In the present study, we use VR to simulate an AR device (the helmet display) and compare it to the current standard method in the same visual-search task (the thermal imaging camera). Thereby, we can make use of the advantages of VR, such as good experimental control, while achieving a realistic impression of the actual AR device and its competitor.
In classical search experiments, performance is often measured in terms of accuracy and reaction times, while gaze shifts are either prevented (“covert search”) or not considered. Many recent paradigms, however, either control for gaze or use gaze-related parameters in addition to reaction times and perceptual judgements (e.g., Becker, 2010). Gaze typically follows attention (Deubel and Schneider, 1996) and can be measured without interfering with the task at hand. Moreover, measuring gaze provides insight into search strategies that would be hard to assess with behavioral measures alone. This includes the spatial order of search (see also section 1.6) and the dissociation between first looking at an item and verifying its identity as target (Malcolm and Henderson, 2010). Since some of these issues only arise for sufficiently complex searches or displays, gaze is particularly well-suited to measure attention and to characterize search in complex and naturalistic settings.
In the real world, gaze – and by inference attention – is largely determined by the task (e.g., Hayhoe and Ballard, 2005; Land, 1992). Even during free exploration, environmental constraints, such as maintaining safe and stable gait, profoundly impact gaze and attention (e.g., 't Hart et al., 2009; Matthis et al., 2018), while at the same time the head is free to move and head and eye movements may serve partially distinct roles ('t Hart and Einhäuser, 2012). Such constraints and differences from the laboratory setting also need consideration for visual search. For example, even for stimuli and tasks that closely resemble the laboratory situation, eye, head and body movements substantially contribute to search (Foulsham et al., 2014), whereas in the lab typically only eye movements are available to scan a limited screen. Several aspects of laboratory data transfer to the real world, such as an interplay between task relevance, fixation probability and object memory (Tatler and Tatler, 2013) or the reduced ability to use negative templates for search compared to positive templates (Kugler et al., 2015). Such studies are clearly distinct from the manifold of applied eye-tracking studies in that they test the extent to which laboratory findings and theories transfer to the real world, with an aim of generalization rather than solving a specific applied question. However, this approach faces a critical trade-off between lab-like experimental control and ecological validity. Here again, VR provides a bridge between the two extremes, by allowing complex stimuli, large environments and realistic tasks, while in principle maintaining full experimental control. The combination with eye tracking has rendered VR an even more promising tool for the study of attention (Clay et al., 2019, for a review). For example, using VR, interactions between visual search for complex realistic objects and memory can be measured and quantified in large environments through which participants can move freely, which would be difficult to realize in a controlled fashion in the real world (Li et al., 2018). Moreover, as one of the pioneering studies using VR in the context of gaze tracking demonstrated, the allocation of gaze towards objects can be linked to broader task-related concepts, such as approach and avoidance behavior, in realistic contexts (Rothkopf et al., 2007). VR setups usually allow for a larger field of view than standard laboratory displays, which – in addition to having the head and the body free to move – might be critical, as peripheral vision contributes substantially to search behavior in natural-scene viewing (Nuthmann and Canas-Bajo, 2022) and real-world tasks (Vater et al., 2022, for a review). At least for the free exploration of indoor spaces, gaze allocation in VR is remarkably similar to real-world behavior (Drewes et al., 2021), provided the VR control does not interfere with gaze (Feder et al., 2022). Together, these results suggest that VR is an ideal test bed to study visual search in realistic scenarios, when task and/or stimulus are of high complexity.
Visual processing in neuro-typical individuals usually has a bias to the left, the so-called pseudoneglect (Bowers and Heilman, 1980). Pseudoneglect is frequently interpreted as bias of attention to the left visual field (Bultitude and Davies, 2006; Nicholls and Roberts, 2002). Other attentional phenomena have similar lateral asymmetries. For example, inhibition of return is more pronounced for cues on the left than for cues on the right, although this asymmetry can largely be attributed to reading direction (Spalek and Hammad, 2005), in contrast to other measures of pseudoneglect (Nicholls and Roberts, 2002). For the case of overt visual search, Zelinsky (1996) described a bias to the upper left for the first search saccade, and in a typical clinical test of actual neglect, target cancellation, which is effectively a search task, the first target canceled is predominantely in the left visual field for neuro-typical participants (Gigliotta et al., 2017). For the case of free scene viewing, an initial tendency to fixate towards the left has also been anecdotally described (e.g., Engmann et al., 2009) before being studied systematically (Nuthmann and Matthias, 2014; Ossandón et al., 2014). For memorization and scene preference tasks, Nuthmann and Matthias (2014) find a robust leftward bias of about one degree for the first 1–2s of scene viewing, which is somewhat larger and more prolonged in the memorization task. Importantly for the present study, Nuthmann and Matthias also found a similar leftward bias for search in natural scenes, which was present even when targets were in the right-hand side of the scene. Ossandón et al. (2014) obtained similar results, though in their study only right-handed individuals showed the leftward bias, while it was largely absent in left-handers. While all these studies show robust effects (at least for right-handers with left-to-right reading direction), they refer to simple displays or to scene viewing, and the biases found are comparably moderate in size. As such, it will be a most interesting issue, whether the leftward bias when encountering a new scene extends to the real world and to virtual reality. While there is no such thing as a true “image onset” in the real-world, encountering a visually new situation, for example, after opening a door, might be comparable in real life.
In the present study we use a visually faithful, high-detail VR reconstruction of a building (Drewes et al., 2021; Feder et al., 2022) to study a real-world visual search task that is aided by display devices. Specifically, we consider the case of detecting and localizing fire sources with the aid of two distinct devices. The first device tested is a thermal imaging camera as it is typically used by firefighters for localizing fire sources. The second device is an AR heat display that is integrated in the firefighter’s helmet (Püschel et al., 2022). Both devices are real-world devices that are reconstructed in detail in our VR simulation. With the aid of either device, participants perform a visual search task as they enter a smoke-filled room and decide whether a fire is present or absent. In the case of a “present” response, they in addition localize the fire by directing a pointing device towards it. We assess how reaction times for the detection task depend on the device, on target presence and on target location, how the localization accuracy depends on the device as well as how gaze direction, head direction, the device direction and the direction of the pointer evolve over time. This follows the threefold aim of this study.
Twenty-four volunteers (11 women, 13 men, age range: 21–49, mean age: 26.9 years (sd: 6.2 years)) from the University of Giessen (JLU) community participated in the study, which was conducted on JLU premises. All participants had normal or corrected-to-normal vision and were naïve to the purpose of the experiment. All but one participant had no experience or training in firefighting. Twenty two were right-handed, 2 left-handed according to self-report. Participants were compensated for their participation at 8 EUR/h or with course credit.
For testing, we used an HTC Vive Pro Eye virtual-reality headset, combined with two Vive handheld controllers. The Vive Pro Eye offers dual OLED displays with a combined resolution of 2,880 × 1,600 pixels, at a screen refresh rate of 90 Hz, and a maximum field of view of 110°. The headset features an integrated Tobii eye tracker, capable of capturing eye movements at a sampling rate of 120 Hz in a tracking range of 110° (accuracy 0.5°–1.1° within 20°). Computations were performed on a Bestware XMG NEO 17 laptop (AMD Ryzen 9 5900HX CPU, 32 GB RAM, Nvidia RTX 3080 Mobile GPU).
The VR environment was modeled in Blender (2.93) and the final firefighting simulation implemented and operated in Unity (2019.4.32f1), using the SteamVR plugin (2.7.3) to handle the tracking of the VR devices and controller inputs, and the SRanipal SDK (1.3.3.0) to access eye-tracking data from Unity. VR and eye tracking were set up in Windows 10 using SteamVR (1.20.4) and SRanipal Runtime (1.3.2.0). Eye tracking was calibrated using SRanipal’s integrated five points calibration procedure.
The VR environment was based on the in-detail replication of an office building at Chemnitz University of Technology used in earlier studies (Figures 1A–G; Drewes et al., 2021; Feder et al., 2022). Rather than using the original layout, doors were placed equidistant to the left-hand side of the corridor at 7.35 m distance between subsequent rooms (Figures 1A, B). The doors led to square rooms that were 7 m × 7 m wide and 2.8 m high. The door was located centrally at one side of the room, and offset by 30.6 cm towards the corridor relative to the inner side of the wall, matching the configuration in the actual building. The starting position for a trial, which was also the center of the virtual cylinder on which targets could appear (see section “fire simulation”), was central to the door, but aligned with the wall (Figures 1C–G).
Figure 1. VR environment. (A, B) Scenes between trials of a block from the participant’s perspective. (A) Block with the helmet display (seen in switched-off state, all LEDs off), (B) block with the thermal imaging camera (seen in the left hand in switched-off state); (C, D) scenes from a training block while searching for the target; unlike in the experimental blocks, the burning cube of wood is visible (C) helmet display, (D) thermal imaging camera; (E, F) scenes from a target-present trial in an experimental block between detection and localization response, blue pointing “beam” is seen while the trigger button is pressed, (E) helmet display, (F) thermal imaging camera. (G) Room configuration as seen from above, triangle: participant initial position, circles: potential target (fire) locations, ɸ azimuthal angle of fire. Fires can be located at heights between 20 cm and 140 cm above the ground. (H) Heat distribution calculated with PyroSim for an example fire source location (angle of −73.64° to the entrance door, height of 60 cm) and a simulation duration of 30 s. Figure modified from the result view in the PyroSim Editor. (I) Definition of azimuthal error (Δɸ).
We used a “point-and-teleport” navigation strategy (Bozgeyikli et al., 2016) to move along the corridor and to enter rooms. In the corridor, the participants could use the controller in their dominant hand to point at any location and teleport there by pressing down the touchpad. In front of each room was a teleportation marker that had to be entered to open the door to that room. Rooms were entered centrally at a fixed location (cyan triangle in Figure 1G) with participants facing straight into the room towards the wall opposing the door. Participants were free to move their head, eyes, arms and body, but remained stationary otherwise.
Heat spread was calculated in PyroSim 2021.3.0901 (Thunderhead Engineering), using the National Institute of Standards and Technology’s Fire Dynamics Simulator (version 6.7.6). A cube with a size of 10 cm × 10 cm × 10 cm was chosen as fire source, emitting heat at a release rate of 540 kW/m2 from all six faces. The heat propagation was simulated for a closed room (7 m × 7 m × 2.8 m, see section “VR environment”), with an initial ambient temperature of 20°C and relative humidity of 40%, at 1013,25 hPa. For 70 different cube positions, the temperature distributions inside the room after 30 s of heat release were computed, with a spatial resolution of 1,000 cells/m³ (Figure 1H).
Fires were placed on a virtual cylinder whose center was the participant’s position when entering the door. For the center of the burning cube, there were 10 different possible azimuthal locations, equally spread out on a semicircle (Figure 1G), and seven different heights (20 cm, 40 cm, 60 cm, 80 cm, 100 cm, 120 cm, and 140 cm above the floor level of the room). In the main experiment, each azimuthal location was used once per participant and device, heights were assigned randomly with replacement, but two subsequent fires never had the same height. For the training trials of each device, 10 fire locations were drawn using the same constraints as in the main experiment.
The helmet display was implemented according to the real prototype (Püschel et al., 2022). It consists of an infrared sensor with a 16 × 4 thermopile array and a field of view of 60° × 16° and 16 LEDs that light up in the colors green, blue, yellow, and red, depending on the measured temperature. Each LED represents one of the 16 horizontal sensor areas, the four vertical zones are combined to form a single display line. In addition, the measuring direction of the infrared sensor is indicated by a laser pointer.
In our experiment, the helmet display was configured to show measured temperatures up to 50°C in green, temperatures between 51°C and 249°C in yellow, and 250°C and above in red. Blue was not used. The helmet display was shown in VR as a screen overlay, positioned 265 px above the center of the image, with each LED being a rectangle (30 px × 30 px) with 15 px spacing between LEDs. Since the infrared sensor of the helmet display measures temperatures in the direction of the central field of view, but the information is displayed in the participants’ upper peripheral field of view, the infrared sensor of the helmet display (virtual implementation and actual prototype) is also equipped with a laser pointer that indicates the direction of measurement, so the participant can learn the offset (Figures 1A,C,E; Supplementary Videos 1, 3, 4).
The controller in the non-dominant hand was simulated as a thermal imaging camera. We chose a Flir E8 as the thermal imaging camera model to achieve the highest possible immersion, since the handles of the Flir thermal imaging camera and the Vive controller are haptically very similar. For better comparability, our thermal imaging camera was implemented to have the same horizontal field of view of 60° as the helmet display. Due to the 4:3 screen ratio of the Flir E8 model, this results in a total field of view of 60° × 45° for our thermal imaging camera (actual Flir E8: 45° × 34°). As a color scheme for the representation of the heat distribution we chose Flir’s ironbow scale, showing the lowest temperature within a trial in a cool and dark color, and the hottest temperature in a bright and warm color, and all other values based on their relative position in the temperature range in between in a continuous color gradient from blue trough purple and pink to orange, yellow, and white (Figures 1B,D,F; Supplementary Videos 2, 5, 6).
Participants performed a combined detection and localization task. In each experimental block, participants subsequently entered a series of 10 rooms. In each room, they first had to decide whether a fire was present (“detection task”). To indicate that they had detected a fire (target-present response), they pressed and held the “trigger” button of the controller placed in their dominant hand (the “pointing device”). This activated a beam emerging from the pointing device, which participants then pointed in the direction of the fire to indicate its location (“localization task”). To indicate completion of the localization task, they released the trigger button, which ended the trial, and observers could return to the corridor, from where they could proceed to the next room for the next trial. If they detected no fire in the room (target-absent response), they pressed one of the side buttons, also ending the trial. For each of the two devices, participants first performed a training block, where the fire was clearly visible (Supplementary Video 1 for the helmet display, Supplementary Video 2 for the thermal imaging camera) and the target was always present. Then two experimental blocks of 10 trials for the same device followed (see Supplementary Videos 3, 4 for the helmet display, Supplementary Videos 5, 6 for the thermal imaging camera), which had 10 target-present and 10 target-absent trials in total. The eye tracker was calibrated at the beginning of each trial using the manufacturer’s procedure, and calibration was validated at the end of each block. The order of device use was counterbalanced across participants, each participant performed first the training for one device, then the experimental blocks for the same device before switching to the other device.
All procedures were reviewed and approved by the applicable local ethics review board (Ethikkommission HSW, Chemnitz University of Technology, case no. V-420-PHKP-WET-Feuerwehr-15012021).
We defined the detection time as the time from entering the room by completing the corresponding teleport operation to the time the response button on the pointing device (either the trigger button for target present or a side button for target absent) was pressed. Only correct trials entered the analysis of detection times. We defined the localization time as the time from the detection response (pressing the trigger button) to the localization response (releasing the trigger button).
For correct target-present trials, two versions of the localization error were computed at the time of button release. The distance of the center of the fire to the beam emerging from the pointing device (i.e., the closest point on the beam to the fire’s center) was defined as the 3D localization error. In addition, we computed the intersection of the beam with the virtual cylinder of potential fire locations; the angular difference between the azimuth (on the cylinder) of this intersection and the azimuth of the fire’s center was defined as the azimuthal localization error (Figure 1I).
In addition to the direction of the pointing device, we also assessed the direction of gaze, the direction of the head and – for trials with the thermal imaging camera – the direction of the thermal imaging camera. As we tracked data from both eyes, for the gaze direction, we assumed a “cyclopian eye” at the center between the two eyes. Gaze is referenced to world coordinates by combining the “eye-in-head” data from the eye-tracker with the head orientation. We report the direction of the simulated laser that is emitted from the top of the simulated helmet (where in the real system the thermal sensor is located) and refer to this measure as “head-aligned laser.” Except for a deviation in vertical direction (150 mm to the center of the headset), this is identical to the usual definition of head direction (and definitions are identical in the horizontal plane). For comparison, we also report the “head-aligned laser” direction for trials with the thermal imaging camera (where the laser is not present). For the thermal imaging camera, we define its direction as that of the invisible beam perpendicular to its lens at the front of the virtual device. When computing deviations in 3D or azimuthal direction for these three “effectors” (gaze, head-aligned laser, thermal imaging camera) we use the same definitions as for the localization error in gaze of the pointing device.
Since – to foreshadow the results – performance in the detection task was practically at ceiling (3 errors in 960 trials), no statistical analysis of detection performance was conducted.
Detection times were analyzed by means of a 2 × 2 repeated-measures analysis of variance (rmANOVA) with factors device (two levels: helmet display, thermal imaging camera) and target presence (two levels: present, absent).
Since the localization task only applies to target-present trials, the effects of device on localization time and on the two localizations errors were assessed with paired t-tests.
The dependence of detection time on azimuthal location of the fire in target-present trials, was analyzed with a 2 × 10 rmANOVA with factors device (as above) and position (the azimuthal fire locations as discrete, nominal levels), and follow-up t-tests were conducted to compare corresponding locations on the left and right of the midline. To avoid empty cells, this analysis was restricted to those 21 participants who performed the detection task in all target-present trials correctly.
Gaze time courses were analyzed for all participants for the first 10 s after trial onset. This was restricted to target-absent trials to avoid confounds by the target localization. The time-courses were truncated at the time of the target-absent response, reducing the number of available data towards the end of the 10-s period, but avoiding any confounds from the response as such and the subsequent leaving of the room. Since the sampling of the device is not entirely uniform in time, gaze data of each trial was interpolated at 100 Hz using linear interpolation where needed. Missing data, e.g., due to blinks, were not interpolated, but retained as missing data. Data were then first averaged across trials to obtain one mean curve per participant, then across participants. This analysis was conducted separately for the two devices. To identify the timepoints at which gaze differed significantly from the straight ahead, a t-test was conducted at each time point. To adjust for alpha-error inflation as consequence of the multiple tests, we adjusted the alpha level to an expected false discovery rate (FDR) of 5% using the procedure introduced by Benjamini and Hochberg (1995). The adjusted alpha level is reported along with the uncorrected p-values.
To qualitatively assess the time course of all “effectors” (gaze, head-aligned laser, pointing device, thermal imaging camera) relative to the fire detection in correct target-present trials, we used the same interpolation as for the gaze data and aligned data either to the detection or the localization response. All trials were truncated at the time of the localization response to avoid confounds from the effector use when leaving the room and proceeding to the next. Otherwise, the treatment was identical to the gaze data in the aforementioned gaze analysis at trial onset.
Across the total of all 24 participants, there were only three trials (of 960), in which a participant gave an incorrect response to the detection task. All three errors were misses (target absent reported, while a fire was present), one for the thermal camera, two for the helmet display, all committed by different participants. In all cases, participants immediately uttered that they made an error, such that it is likely that the response was mainly a motor slip or response button mix up rather than an actual perceptual miss.
For the time to decide whether a fire was present in the room (detection task), correct responses were faster for the thermal imaging camera than for the helmet display (F(1,23) = 16.0, p < .001, Figure 2A). As expected in a visual search task, target-present trials were faster than target-absent trials (F(1,23) = 10.6, p = .004). There was no interaction between the factors device and target presence (F(1,23) = 0.63, p = .435); that is, we have no evidence that the benefit of the thermal camera would be modulated by whether a target was present or not. In target-present trials, the time from detection to localization had a trend to be shorter for the helmet display than for the thermal imaging camera (t(23) = 2.01, p = .056, Figure 2B). Together, this indicates that the thermal imaging camera has advantages for detecting fire, but reporting the fire’s location after detection tends to be faster when wearing the helmet display than when using the thermal imaging camera.
Figure 2. Reaction times. (A) Detection task. (B) Localization task. Errorbars denote standard error of the mean (s.e.m.). Hatched: helmet display, solid gray: thermal camera. Note the different scales in (A, B).
Besides analyzing the speed of detection and localization, we asked whether the accuracy with which a fire was located differed between the devices. We measured the distance between the beam originating from the pointer and midpoint of the fire at the time the trigger button of the pointer was released. For this 3D localization error, we found a trend to a benefit for the helmet display (t(23) = 2.06, p = .051): the error was 9.8 cm ± 4.7 cm (mean ± s.e.m.) smaller for the helmet display than for the thermal imaging camera (Figure 3A). For training, when the fire was visible, this difference was only 1.9 cm ± 0.8 cm and the errors themselves were also substantially smaller (black lines in Figure 3A) and on the order of the size of the burning cube (10 cm edge length). Hence, a putative motor difference, which, e.g., could result from simultaneously operating two handheld devices, could not explain the observed difference for the main experiment.
Figure 3. Localization error. (A) 3D error. (B) Azimuthal error. (C) Vertical component of 3D error. (A–C): Mean and s.e.m. for correct target-present trials; horizontal lines denote training error. Hatched: helmet display, solid gray: thermal camera. (D) Distribution of azimuthal error (x-axis) and vertical error (y-axis) for the two devices. Representation smoothed with a 5 deg (azimuth) x 30 cm (height) sliding average. Note that panels (A–C) denote absolute error, (D) includes the direction (sign) of the error.
Since the tested configuration of the helmet display had only one horizontal line, users needed to integrate visual information over different head positions to obtain an estimate of the vertical location of the fire. It could therefore be expected that the benefit of the helmet display would play out particularly in the horizontal dimension. To test this, we computed the angular (azimuthal) error (Δϕ) in fire localization for the projection perpendicular to the floor plane. We found this error to differ between the devices, with a benefit for the helmet display (t(23) = 2.51, p = .019): the error was 2.1° ± 0.83° smaller for the helmet display than for the thermal imaging camera (Figure 3B). This corresponds to a reduction of the horizontal error by 12.8 cm ± 5.1 cm. Again, this could not be explained by a putative motor difference, as the difference in training was a mere 0.7° ± 0.5° and the training error itself (black lines in Figure 3B) in the order of the angular size of the burning cube. In contrast, considering the absolute value of the vertical component of the 3D error, no difference was found between the devices (t(23) = 0.128, p = .899; Figure 3C). The larger error in the azimuthal direction for the thermal camera is also evident in a 2D representation of the errors that preserves the error’s sign: while on average both devices are rather accurate on the vertical midline, the precision is higher (the spread lower) for the helmet display (Figure 3D, top) than for the thermal imaging camera (Figure 3D, bottom). In sum, there is a localization advantage for the helmet display over the thermal imaging camera, particularly for the horizontal direction, which is not explained by motor differences in pointing when operating the devices.
Participants entered each room at a predefined location, standing centrally in the door and looking forward. Hence, we asked whether the detection time depended on the azimuthal location of the target. Since each azimuthal location was probed only once per device, we excluded those participants from this analysis who had one erroneous trial. For the remaining 21, we found a main effect of location (F(9,20) = 4.59, p < .001). Unsurprisingly, we confirmed the benefit of the thermal camera (F(1,20) = 57.6, p < .001), which we had already observed in the location-averaged data. Importantly, we found no evidence for an interaction between the two factors (F(9,20) = 0.33, p = .963), suggesting that the benefit is location-independent. Qualitatively, we observed that the detection was fastest in the center, and tended to drop to either side (Figure 4). More prominently, we observed that detection was slower on the right-hand side of the room than on the left-hand side. To quantify this, – due to absence of an interaction - we first aggregated data over both devices at each location and for each observer and then compared the detection times between the corresponding left-side and right-side locations. For the outer locations, we found differences between the sides (73.6°: t(20) = 3.02, p = .007; 57.3°: t(20) = 2.77, p = .012; 40.9°: t(20) = 2.54, p = .020), but not for the inner locations (24.6°: t(20) = 0.04, p = .970; 8.2°: t(20) = 0.50, p = .622). Hence, for eccentric locations, targets were detected faster on the left-hand side than on the corresponding right-hand side.
To assess whether the benefit for the left side reflected a general preference to start search on this side, we analyzed how the gaze direction changed over the course of the first 10 s of all target-absent trials in all participants. Since there were time points for which the gaze direction differed at an uncorrected 5% alpha level (though none of those remained significant at an expected FDR of 5%), we treated the two devices separately for this analysis. For the helmet display, we found an initial deviation of gaze direction to the left (Figure 5A). The deviation peaked at 21° at 1.42 s after trial onset and was significantly different from the straight ahead (p < .006, the adjusted alpha level at an expected FDR of 5%) from 0.17 to 1.46 s. After the initial leftward bias, a rebound to the right followed reaching a maximum of 14°. This rebound, however, was not significantly different from the straight ahead at the adjusted alpha level. Gaze for the thermal imaging camera had a similar time course (Figure 5B). It deviated significantly (p < 0.020) to the left from the straight ahead from 0.09 s after trial onset to 1.79 s, with a peak at 25.5° 1.11 s after trial onset. For the thermal imaging camera, the rebound to the right was significant at the adjusted alpha level from 3.45 to 5.76 s, peaking at 24.1° after 5.11 s before leveling off in the center. Since in the context of scene viewing it has been suggested that the initial leftward bias depends on handedness (Ossandón et al., 2014), and as the device and pointer was swapped for left-handed individuals, we plot the data of our two left-handed participants for comparison. Both qualitatively show a similar gaze behavior to the average: an initial deviation to the left followed by a rebound to the right for both devices (Figures 5C, D). In sum, for both devices, we see an initial orienting to the left, which is followed (inevitably) by some rebound to the right, and this pattern is not limited to the right-handed individuals.
Figure 5. Time course of gaze direction over a trial. (A) Helmet display: mean and s.e.m. over all participants; (B) Thermal imaging camera: mean and s.e.m. over all participants. Black bars in (A, B) indicate significant difference from 0° at an expected FDR of 5%; 0° corresponds to straight ahead when entering the room. (C) Mean over trials for the two left-handed participants, helmet display. (D) Mean over trials for the two left-handed participants, thermal imaging camera. Note the difference in scale between top and bottom panels. Gaps in trace indicate no available data for the respective time point (Note that data get sparser towards the end of the trial as only a few trials last 10 s).
To obtain an additional qualitative understanding for the dynamics of device use, we aligned the data from all correct target-present trials to the time of the localization response (Figures 6A–D). For the helmet display, the deviation of the head direction from the target (Figure 6A, magenta) levels off at the minimum distance about 2.5 s to 3 s before the localization is indicated. During this period, gaze direction (Figure 6A, black) and the direction of the pointing device (Figure 6A, red) continue towards the target until less than a second before the localization response. When using the thermal imaging camera (Figure 6B), the deviation from the target levels off at about 1.5 s before the response for all four “effectors” (gaze, head, thermal camera and response pointer). For the head, this is later than in case of the helmet display, while for gaze and response pointer it is earlier. We also note that in 3D-space the thermal camera (Figure 6B, blue) was not pointed directly towards the target even at the time of the response, as its deviation remained substantially larger than those of the response pointer, head and gaze. This is understandable, as the thermal imaging camera has an extended display, in particular in the vertical direction, such that no major adjustment of the height to the fire position is needed to solve the localization task. When considering only the azimuthal deviation (Figures 6C, D), time courses were comparable to the 3D deviation. However, the azimuthal deviation of the thermal imaging camera at the time of the localization response was smaller than the error made with the response pointer (Figure 6D). This suggests that the thermal imaging camera horizontally is directed in the direction of the fire and the coordinate transform between the two hands induces some additional deviation in the azimuthal direction. To test whether localization could be already nearly complete at the time of the detection response, we additionally aligned the same data to the detection response (Figures 6E–H). For the case of the helmet display, the alignment of the head (and thus the display) with the target neared completion about 1 s prior to the detection response, whereas gaze and in particular the response device continued to be readjusted towards the target while the detection response had already been given (Figure 6E). When using the thermal imaging camera, all effectors were close to the minimal distance at the time of the detection response (Figure 6F). The time course of the azimuthal deviation relative to the detection response (Figures 6G, H) was similar to the 3D case, but it is noteworthy that the azimuthal alignment of the camera was completed already slightly prior to the detection response (Figure 6H, blue). Although this analysis remained deliberately qualitative, it revealed remarkable difference in the device use; most importantly, the adjustment of the thermal imaging camera is largely limited to the horizontal (azimuthal) direction, presumably indicating a benefit of its wide vertical range, which renders a precise vertical adjustment unnecessary, potentially aiding quicker detection as compared to the helmet display.
Figure 6. Time courses of deviations from fire in target-present trials. (A–D) Time courses relative to time point of the localization response; (E–H) time courses relative to time point of the detection response. In all panels, mean and s.e.m. over participants are depicted for the deviation of the gaze vector from the target (black), the deviation of the response pointer (red), and the deviation of the head-aligned laser (magenta). For the trials with thermal camera, the deviation of its direction from the target is depicted in addition (blue). (A, B, E, F) depict the three-dimensional distance, (C, D, G, H) depict the azimuthal deviation. Note that data are truncated at the localization response for each trial, such that the number of trials entering the analysis decreases for t > 0 s in panels (E–H), rendering the representations somewhat more noisy in the late stages of these plots.
In the present study, we demonstrated how a VR setting that has a high visual fidelity, can be used to evaluate two different visual displays, including an augmented reality helmet, for their use in a complex visual-search task. VR plays out a particular strength in that it allows for scenarios whose real-life version would be too hazardous for untrained participants. On a conceptual level, the data highlight the need to distinguish between detection (Is a fire present?) and localization (Where is the fire?), for which the devices show contrary results to each other. Beyond the specific evaluation task, the data provide insight into overall search behavior, such as the substantial leftward bias when starting search, which results in targets on the right being detected later than targets on the left. Using gaze tracking and assessing the relative time course of all sensing devices and effectors provides insight as to how the interplay between looking and acting unfolds over time.
The observation that gaze upon entering a room initially is biased to the left is consistent with search for simple items (Zelinsky, 1996), free viewing of scenes (Engmann et al., 2009; Nuthmann and Matthias, 2014; Ossandón et al., 2014) and search in naturalistic scenes (Nuthmann and Matthias, 2014). It should be noted, however, that the effects found in the present study are about an order of magnitude larger than those typically observed in scene viewing. For example, the biases in the studies of Nuthmann and Matthias as well as of Ossandón and colleagues are on the order of 2°, whereas we observe an effect of more than 20°, although the time courses are roughly comparable. However, in our paradigm, observers were free to move their head and the bulk of orienting towards potential target locations indeed used head rather than eye movements. While there is no scene onset, which has a profound effect on reorienting towards the image center in natural scene viewing ('t Hart et al., 2009), the opening of the door starts a new trial and thereby corresponds to a scene onset. It could be argued that such onsets are specific to VR, but events like entering a room, opening a door, switching on the light, etc., also do exist in real life and arguably constitute “natural” onsets to which an attentional reorienting takes place. It should be noted that in our paradigm, participants approach the rooms always from the same side of the corridor and the door’s opening direction is animated identically in all cases. While it cannot fully be excluded that this contributes to spatial biases when entering a new room, we consider it unlikely that this explains the full effect. First, there is no strategic benefit of starting search at one side or the other, as all rooms are independent from each other. Second, as a consequence of the point-and-teleport control, participants “enter” the room centrally without any animation of the door being visible at trial onset. The observed pseudoneglect also exemplifies how gaze tracking reveals spatial search strategies and patterns. From a practical point of view, knowledge about such strategies is useful as guidelines may either respect them when they are beneficial or neutral to performance or take active countermeasures if they constitute a bias harmful to task completion.
When searching a natural scene, there is a crucial influence of context, which constrains the search area at which a target is likely to be found (Eckstein et al., 2006; Neider and Zelinsky, 2006; Torralba et al., 2006). By the smoke simulation used in our present study, in contrast, contextual information is largely eliminated, which corresponds to the actual challenge faced by firefighters. Nonetheless, owing to the training block, participants are likely aware of the range of possible target locations and constrained to the room in front of them. While this approach is owed to the application scenario, the absence of scene context is not unusual for other cases of real-life visual search, either. For example, in luggage screening (Wolfe et al., 2005, 2013), there also is a confined search space (the luggage item), but the context is – if anything – very unlike most everyday visual experience, in particular due to lack of opacity that would usually aid foreground-background separation and scene segmentation. Similarly, in 3D image search, as for example, in medical contexts (Drew et al., 2013), observers also lack information from familiar scene layout. Understanding search strategies in such scenarios and relating them to issues from fundamental research on visual search, is crucial to prevent biases that might be decremental to performance in real life. In the context of the application studied in the present study, it is in fact critical that firefighters learn to deal with the lack of contextual information in smoke-filled environments and to rely on their equipment instead. Here, AR solutions – like the helmet display – hold a great promise, provided their advantages and disadvantages are carefully evaluated in realistic settings.
Several earlier studies have used VR to study visual search, with a particular focus on scene layout and memory. While visual search in a 3D VR environment is in general similar to searching a 2D scene with similar content, memory – in particular learnt associations between space and search targets – is more relevant in 3D than in 2D (Li et al., 2016). Memory-related search benefits in VR arise from quickly learning the general spatial layout of the 3D environment (Li et al., 2018), and these results seem in line with real-world search (Draschkow and Võ, 2016). However, similar VR experiments suggest that the acquisition of the spatial layout may incur costs for the initial search in a novel 3D environment that only pay off when repeatedly searching the same environment (Beitner et al., 2021). These and similar experiments in VR are of highest relevance to probe the transfer from theories, models and empirical findings established in scene search on screen to the real world. However, these approaches are different from ours in several respects. First, our VR aims at a high degree of visual realism, using parts of an actual building and high-detail simulations of actual devices along with a physics-based simulation of fire. Second, we focus on visual search that is aided by a device, a mode that is different from the visual search with the naked eye, but arguably of high importance to a variety of applications, including the present one (searching for fire sources) and the aforementioned examples of luggage screening and medical imaging. As a consequence, memory and scene layout are irrelevant (all rooms are identical), which is in sharp contrast to everyday search, but in line with the situation firefighters are faced with in smoky settings, where spatial scene layout is nearly impossible to discern visually.
A key result for the applied question asked in this study is the separation between localization and detection performance. The fact that the current standard system (the thermal imaging camera) outperforms the first instantiation of the novel system (the helmet camera) with respect to detection is not surprising, but in fact reassuring that the simulation is sufficiently realistic as to not setting up a strawman. The fact that the benefit reverses for localization, both in terms of speed and accuracy, already indicates potential benefits of the novel system. Its localization benefit may arise from the fact that no change of coordinate frames is needed, unlike the transformation from the hand carrying the thermal imaging camera to the hand carrying the pointer. Interestingly, a difference between (direct) localization, i.e., pointing towards a target, and target detection has already been described for a more abstract, but still three-dimensional, setting (Liu et al., 2003). Importantly, direct localization, as was also used here, did not interfere with the detection task, suggesting distinct processes for detection and localization, argued by Liu and colleagues to map to the ventral and dorsal visual pathways (Mishkin and Ungerleider, 1982), respectively. It seems conceivable that this division of labor will also aid the localization for the helmet display as compared to the thermal imaging camera, as only one device needs to be pointed at the target.
The localization benefit of the helmet display is particularly evident in the azimuthal direction. This is not surprising, as the helmet display for any given moment provides only information about the horizontal position of the heat source, while its vertical location needs to be inferred by the change of the display as consequence of the head movement. It will be an interesting issue for further development whether additional rows will improve localization and potentially detection further. From an applied point of view, it is also important to highlight that the use of the two devices is not mutually exclusive. The spatial information for the thermal imaging camera is decoupled from the looking direction; it therefore allows scanning behavior while maintaining gaze elsewhere. During firefighting the current task can vary over time and between individuals, where for some cases aspects of detection may be more relevant, for others precise localization. In addition, irrespective of search performance, the helmet display has the advantage for the user to have both hands free. The helmet display may also be used in different modes, for example, as a surround view warning device compared to the localization device as used herein. Testing the interplay between different devices for various fire-fighting aspects is also readily achievable in our VR setting. Moreover, there is potential for optimization of the display, for example, with respect to resolution, to color, to size, to the gain relative to head movements (which in principle could deviate from one to, for example, cover the full 360° within the users’ visual field), to position in the visual field and so forth. Here, the advantages of VR for quick prototyping becomes particularly evident. While building prototypes with diverse settings would be cumbersome, and it would be hard to cover continuous ranges for these parameters, in VR this requires a mere change of parameters or a few lines of additional code. On a conceptual level, our study combines multiple use cases for extended reality (XR) in vision science and visualization: the application case concerns an actual AR device, the VR allows for lab-like experimental control in a visual search task, and the VR allows a highly realistic simulation of the envisioned application domain. It thereby exemplifies how VR can bridge gaps between fundamental and applied research as well as between experimental control and ecological validity, in particular for research questions where thorough real-life testing is impossible, unethical or prohibitively expensive.
In the spirit of digital twins (see section 1.2), we employ a visually realistic VR environment, a visually faithful simulation of fire and smoke, and a visually faithful simulation of the AR device to be evaluated. Importantly, we also use a visually faithful simulation of the current state-of-the-art device for the specific operation (the thermal imaging camera); that is, for our comparison we are not setting up a strawman, but make this baseline a challenging one. Consequently, we did not expect that the first version of the helmet display would outperform the thermal imaging camera (even though it does with respect to localization accuracy and precision; Figure 3), nor did we attempt any claims of the impact of the seen benefit on the actual operation. Instead, we provide a setting in which further versions of the device can be quickly prototyped and tested (and are currently being tested) – in naïve participants as well as in firefighters at every level of expertise and training - prior to running expensive, and potentially dangerous, real-world tests.
Several earlier studies have discussed the use of VR in the context of search-and-rescue operations. Common research directions include - for example - optimizing spatial navigation strategies (Shi et al., 2021), investigating workload in technology-supported operations (Dell’Agnola et al., 2020) or assessing - and eventually facilitating - human-robot interaction in dangerous scenarios (Atkinson and Clark, 2014). Based on a comprehensive review, Wheeler et al. (2021) argue that VR holds the promise to provide sufficient ecological validity for training firefighters for their real-world task – provided a number of guidelines are met. Our present approach is complementary to these earlier studies. Our main focus has been on evaluating the first instantiation of an AR device – the helmet display – in a sufficiently realistic VR environment. While the use of AR for search-and-rescue has been proposed earlier, it has mostly remained a vision for the future (e.g., LaLone et al., 2019), in particular as a rigorous evaluation for search-and-rescue operations poses a challenge (e.g., Wang et al., 2018). It should also be noted that these studies refer to USAR under normal visibility, while in firefighting visibility can be low or zero. Low visibility may provide an advantage to using the visual channel in the device as it is not occupied otherwise (cf. e.g., Bailie et al., 2016), even if tactile or multimodal information might appear preferable to firefighters (Wolf et al., 2019; Streefkerk et al., 2012).
The main aim of the present study was an initial evaluation of the helmet display as a device providing visual information to further optimize its design to provide relevant visual information effectively and efficiently. Realism and fidelity therefore focused on the visual domain and the physical simulation of the heat distribution, which are the key variables of interest. Consequently, the task was entirely visual, while in real-world firefighting situations, firefighters may rely heavily on tactile and auditory information for some of their tasks. It is evident that after an initial optimization in naïve observers, the device has to be evaluated with firefighters of different levels of expertise as well as in scenarios that go beyond the visual aspects. This may include aspects like time pressure, varying visibility, threats, auditory noise, secondary tasks, etc. Importantly, all of these aspects can be readily integrated in a VR setting. Another issue may arise from the simulation of movement in VR. In a real firefighting situation, this will often involve careful progress with tactile guidance, which is quite the opposite to the point-and-teleport strategy used herein. We chose point-and-teleport mostly for convenience given the trial-wise structure of the task (one room after another with no relation between them). An extension of the visual setting to a continuous scenario guided by real-world demands would be straightforward. Although fully realistic movements are challenging, hybrid strategies of simulated translational movements combined with real rotational movements (Feder et al., 2022) provide a good compromise as they are extendable to more complex in-place bodily movements, such as ducking, and to crawling.
While it is evident that the detection and localization of fire sources with devices augmenting vision – either the handheld camera or the novel helmet display – constitutes only one specific aspect of the broad range of tasks firefighting encompasses, our study demonstrates how VR can be used to prototype and evaluate AR displays for this application. On an abstract level, this exemplifies the usefulness of VR simulations and digital twins for rapid AR development. The study also highlights the fact that visual search is not always done with the naked eye, but in practice often requires the interplay of searcher, environment and technological device, which are ideally studied in conjunction. Our VR simulation has a high visual fidelity and high physical fidelity with respect to the heat distribution. This ensures realism along the dimensions of relevance – that is, providing visual information about a physically realistic heat distribution to the user. The setting is readily extendable to include further aspects of the real firefighting scenario and ready for use by actual firefighting professionals. Besides these practical aspects, we used the scenario as a complex search task, which, in comparison to typical visual search in the real world, deprived participants of contextual information. Interestingly, this allowed us to uncover that the pseudoneglect, an effect of high theoretical relevance, is substantially more pronounced under such conditions than – for example – in natural scene viewing. In turn, knowledge about such spatial search strategies, patterns or biases like the pseudoneglect can be of practical relevance, for example, when planning search or devising guidelines for search.
The raw data supporting the conclusions of this article will be made available by the authors, without undue reservation.
All study procedures were reviewed and approved by the applicable local ethics review board (Ethikkommission HSW, Chemnitz University of Technology, case no. V-420-PHKP-WET-Feuerwehr-15012021). The studies were conducted in accordance with the local legislation and institutional requirements. The participants provided their written informed consent to participate in this study.
SF: Conceptualization, Data curation, Formal Analysis, Investigation, Methodology, Software, Visualization, Writing–original draft. AP: Conceptualization, Writing–review and editing. MŞ: Data curation, Investigation, Writing–review and editing. SO: Conceptualization, Funding acquisition, Supervision, Writing–review and editing. AB: Conceptualization, Funding acquisition, Supervision, Writing–original draft. WE: Conceptualization, Data curation, Formal Analysis, Funding acquisition, Project administration, Supervision, Visualization, Writing–original draft.
The author(s) declare that financial support was received for the research, authorship, and/or publication of this article. The work was funded in part by the Deutsche Forschungsgemeinschaft (DFG, German Research Foundation) project ID 416228727 – SFB 1410, projects A03, A04 and C01.
We are grateful to Jutta Billino and her group at JLU Gießen for providing logistic support including their lab space for running the experiments. We thank Thunderhead Engineering for providing us with a time-limited academic PyroSim license free of charge for the purpose of this study. The manuscript is available as a preprint in PsyArXiv (https://doi.org/10.31234/osf.io/ymhvt).
The authors declare that the research was conducted in the absence of any commercial or financial relationships that could be construed as a potential conflict of interest.
All claims expressed in this article are solely those of the authors and do not necessarily represent those of their affiliated organizations, or those of the publisher, the editors and the reviewers. Any product that may be evaluated in this article, or claim that may be made by its manufacturer, is not guaranteed or endorsed by the publisher.
The Supplementary Material for this article can be found online at: https://www.frontiersin.org/articles/10.3389/frvir.2024.1252351/full#supplementary-material
SUPPLEMENTARY VIDEO 1 | Training trial with the helmet display. A high-resolution version is available at https://osf.io/mrzdh.
SUPPLEMENTARY VIDEO 2 | Training trial with the thermal imaging camera. A high-resolution version is available at https://osf.io/wmta3.
SUPPLEMENTARY VIDEO 3 | Target-present trial with the helmet display. A high-resolution version is available at https://osf.io/gaqu9.
SUPPLEMENTARY VIDEO 4 | Target-absent trial with the helmet display. A high-resolution version is available at https://osf.io/dgu4a.
SUPPLEMENTARY VIDEO 5 | Target-present trial with the thermal imaging camera. A high-resolution version is available at https://osf.io/qe8xd.
SUPPLEMENTARY VIDEO 6 | Target-absent trial with the thermal imaging camera. A high-resolution version is available at https://osf.io/98nkp.
Alexander, R. G., and Zelinsky, G. J. (2011). Visual similarity effects in categorical search. J. Vis. 11 (8), 9. doi:10.1167/11.8.9
Atkinson, D. J., and Clark, M. H. (2014). “Methodology for study of human-robot social interaction in dangerous situations,” in Proc. Sec. Intern. Conf. Human-agent Interact. (ACM), 371–376. doi:10.1145/2658861.2658871
Bailie, T., Martin, J., Aman, Z., Brill, R., and Herman, A. (2016). “Implementing user-centered methods and virtual reality to rapidly prototype augmented reality tools for firefighters,” in Found. Augment. Cogn.: Neuroergonom. Operational Neurosci.: 10th International Conference, AC 2016, Proc., Part II (Springer International Publishing), 135–144. doi:10.1007/978-3-319-39952-2_14
Becker, S. I. (2010). The role of target–distractor relationships in guiding attention and the eyes in visual search. J. Exp. Psychol. Gen 139 (2), 247–265. doi:10.1037/a0018808
Beitner, J., Helbing, J., Draschkow, D., and Võ, M.L.-H. (2021). Get your guidance going: investigating the activation of spatial priors for efficient search in virtual reality. Brain Sci. 11 (1), 44. doi:10.3390/brainsci11010044
Benjamini, Y., and Hochberg, Y. (1995). Controlling the false discovery rate: a practical and powerful approach to multiple testing. J. R. Stat. Soc. B (Meth.) 57 (1), 289–300. doi:10.1111/j.2517-6161.1995.tb02031.x
Bowers, D., and Heilman, K. M. (1980). Pseudoneglect: effects of hemispace on a tactile line bisection task. Neuropsychologia 18 (4-5), 491–498. doi:10.1016/0028-3932(80)90151-7
Bozgeyikli, E., Raij, A., Katkoori, S., and Dubey, R. (2016). “Point & teleport locomotion technique for virtual reality,” in Proc. 2016 Ann. Symp. Comp. Hum. Interact. Play, 205–216. doi:10.1145/2967934.2968105
Brade, J., Lorenz, M., Busch, M., Hammer, N., Tscheligi, M., and Klimant, P. (2017). Being there again–Presence in real and virtual environments and its relation to usability and user experience using a mobile navigation task. Int. J. Hum. Comput. Stud. 101, 76–87. doi:10.1016/j.ijhcs.2017.01.004
Bultitude, J. H., and Davies, A. M. A. (2006). Putting attention on the line: investigating the activation–orientation hypothesis of pseudoneglect. Neuropsychologia 44 (10), 1849–1858. doi:10.1016/j.neuropsychologia.2006.03.001
Burghardt, A., Szybicki, D., Gierlak, P., Kurc, K., Pietruś, P., and Cygan, R. (2020). Programming of industrial robots using virtual reality and digital twins. Appl. Sci. 10 (2), 486. doi:10.3390/app10020486
Clay, V., König, P., and Koenig, S. (2019). Eye tracking in virtual reality. J. Eye Mov. Res. 12 (1), 3. doi:10.16910/jemr.12.1.3
Dell’Agnola, F., Momeni, N., Arza, A., and Atienza, D. (2020). “Cognitive workload monitoring in virtual reality based rescue missions with drones,” in Virtual, Augment. Mixed Real. Design and Interact. 12th Intern. Conf., VAMR 2020 Proc. Part I (Cham: Springer), 397–409. doi:10.1007/978-3-030-49695-1_26
Deubel, H., and Schneider, W. X. (1996). Saccade target selection and object recognition: evidence for a common attentional mechanism. Vis. Res. 36 (12), 1827–1837. doi:10.1016/0042-6989(95)00294-4
Draschkow, D., and Võ, M.L.-H. (2016). Of “what” and “where” in a natural search task: active object handling supports object location memory beyond the object’s identity. Atten. Percept. Psychophys. 78, 1574–1584. doi:10.3758/s13414-016-1111-x
Drew, T., Võ, M.L.-H., Olwal, A., Jacobson, F., Seltzer, S. E., and Wolfe, J. M. (2013). Scanners and drillers: characterizing expert visual search through volumetric images. J. Vis. 13 (10), 3. doi:10.1167/13.10.3
Drewes, J., Feder, S., and Einhäuser, W. (2021). Gaze during locomotion in virtual reality and the real world. Front. Neurosci. 15, 656913. doi:10.3389/fnins.2021.656913
Eckstein, M. P., Drescher, B. A., and Shimozaki, S. S. (2006). Attentional cues in real scenes, saccadic targeting, and Bayesian priors. Psych. Sci. 17 (11), 973–980. doi:10.1111/j.1467-9280.2006.01815.x
Engmann, S., ‘t Hart, B. M., Sieren, T., Onat, S., König, P., and Einhäuser, W. (2009). Saliency on a natural scene background: effects of color and luminance contrast add linearly. Atten. Percep. Psychophys. 71 (6), 1337–1352. doi:10.3758/APP.71.6.1337
Feder, S., Bendixen, A., and Einhäuser, W. (2022). “A hybrid control strategy for capturing cognitive processes in virtual reality (VR) in a natural and efficient way,” in 2022 IEEE 9th Intern. Conf. Comput. Intell. Virtual Environm. Measurem. Sys. and Appl. – CIVEMSA (IEEE), 1–6. doi:10.1109/CIVEMSA53371.2022.9853646
Fei-Fei, L., Iyer, A., Koch, C., and Perona, P. (2007). What do we perceive in a glance of a real-world scene? J. Vis. 7 (1), 10. doi:10.1167/7.1.10
Feng, Z., González, V. A., Amor, R., Lovreglio, R., and Cabrera-Guerrero, G. (2018). Immersive virtual reality serious games for evacuation training and research: a systematic literature review. Comput. Educ. 127, 252–266. doi:10.1016/j.compedu.2018.09.002
Foulsham, T., Chapman, C., Nasiopoulos, E., and Kingstone, A. (2014). Top-down and bottom-up aspects of active search in a real-world environment. Can. J. Exp. Psychol. 68 (1), 8–19. doi:10.1037/cep0000004
Gigliotta, O., Malkinson, T. S., Miglino, O., and Bartolomeo, P. (2017). Pseudoneglect in visual search: behavioral evidence and connectional constraints in simulated neural circuitry. Eneuro 4 (6), ENEURO 0154–217. doi:10.1523/ENEURO.0154-17.2017
Glaessgen, E. H., and Stargel, D. S. (2012). “The digital twin paradigm for future NASA and US Air Force vehicles,” in 53rd AIAA/ASME/ASCE/AHS/ASC Struct. Struct. Dynam. Mat. Conf. 0. doi:10.2514/6.2012-1818
Gopinath, V., Srija, A., and Sravanthi, C. N. (2019). Re-design of smart homes with digital twins. J. Phys. Conf. Ser. 1228, 012031. doi:10.1088/1742-6596/1228/1/012031
Grandi, J. G., Cao, Z., Ogren, M., and Kopper, R. (2021). “Design and simulation of next-generation augmented reality user interfaces in virtual reality,” in 2021 IEEE Conf. Virtual Real. 3D User Interface (IEEE), 23–29. doi:10.1109/VRW52623.2021.00011
Haskins, J., Zhu, B., Gainer, S., Huse, W., Eadara, S., Boyd, B., et al. (2020). “Exploring VR training for first responders,” in IEEE Conf. Virtual Real. 3D User Interface (IEEE), 57–62. doi:10.1109/VRW50115.2020.00018
Hayhoe, M., and Ballard, D. (2005). Eye movements in natural behavior. Trends Cogn. Sci. 9 (4), 188–194. doi:10.1016/j.tics.2005.02.009
Hinricher, N., König, S., Schröer, C., and Backhaus, C. (2023). Effects of virtual reality and test environment on user experience, usability, and mental workload in the evaluation of a blood pressure monitor. Front. Virtual Real 4, 1151190. doi:10.3389/frvir.2023.1151190
Itti, L., and Koch, C. (2000). A saliency-based search mechanism for overt and covert shifts of visual attention. Vis. Res. 40 (10-12), 1489–1506. doi:10.1016/S0042-6989(99)00163-7
Itti, L., Koch, C., and Niebur, E. (1998). A model of saliency-based visual attention for rapid scene analysis. IEEE Trans. Patt. Anal. Mach. Intell. 20 (11), 1254–1259. doi:10.1109/34.730558
Kamel Boulos, M. N., and Zhang, P. (2021). Digital twins: from personalised medicine to precision public health. J. Pers. Med. 11 (8), 745. doi:10.3390/jpm11080745
Kapalo, K. A., Bockelman, P., and LaViola Jr, J. J. (2018). “’Sizing up’ emerging technology for firefighting: augmented reality for incident assessment,” in Proc. Hum. Fact. Ergon. Soc. Ann. Meet (Los Angeles: SAGE), 1464–1468. doi:10.1177/1541931218621332
Koch, C., and Ullman, S. (1985). Shifts in selective visual attention: towards the underlying neural circuitry. Hum. Neurobiol. 4 (4), 219–227.
Kritzinger, W., Karner, M., Traar, G., Henjes, J., and Sihn, W. (2018). Digital Twin in manufacturing: a categorical literature review and classification. IFAC-PapersOnLine 51 (11), 1016–1022. doi:10.1016/j.ifacol.2018.08.474
Kugler, G., 't Hart, B. M., Kohlbecher, S., Einhäuser, W., and Schneider, E. (2015). Gaze in visual search is guided more efficiently by positive cues than by negative cues. PloS One 10 (12), e0145910. doi:10.1371/journal.pone.0145910
Kuschnereit, A., Bendixen, A., Mandl, D., and Einhäuser, W. (2024). “Using eye tracking to aid the design of human machine interfaces (HMIs) in industrial applications,” in Proc. 1st intern. Conf. Hybrid soc. Editors B. Meyer, O. Kanoun, and U. Thomas (Springer), 1.
Lacoche, J., Villain, E., and Foulonneau, A. (2022). Evaluating usability and user experience of AR applications in VR simulation. Front. Virtual Real 3, 881318. doi:10.3389/frvir.2022.881318
LaLone, N. J., Alharthi, S. A., and Toups Dugas, P. O. (2019). “A vision of augmented reality for urban search and rescue,” in Proc. Halfw. Future Symp. 14. doi:10.1145/3363384.3363466
Lambert, K., Merci, B., Gryspeert, C., and Jekovec, N. (2021). Search & rescue operations during interior firefighting: a study into crawling speeds. Fire Saf. J. 121, 103269. doi:10.1016/j.firesaf.2020.103269
Land, M. F. (1992). Predictable eye-head coordination during driving. Nature 359, 318–320. doi:10.1038/359318a0
Li, C.-L., Aivar, M. P., Kit, D. M., Tong, M. H., and Hayhoe, M. M. (2016). Memory and visual search in naturalistic 2D and 3D environments. J. Vis. 16 (8), 9. doi:10.1167/16.8.9
Li, C.-L., Aivar, M. P., Tong, M. H., and Hayhoe, M. M. (2018). Memory shapes visual search strategies in large-scale environments. Sci. Rep. 8, 4324. doi:10.1038/s41598-018-22731-w
Liu, G., Healey, C. G., and Enns, J. T. (2003). Target detection and localization in visual search: a dual systems perspective. Percept. Psychophys. 65 (5), 678–694. doi:10.3758/BF03194806
Lu, W., Duh, B. L. H., and Feiner, S. (2012). “Subtle cueing for visual search in augmented reality,” in 2012 IEEE Intern. Symp. Mixed Augm. Reality – ISMAR (IEEE), 161–166. doi:10.1109/ISMAR.2012.6402553
Malcolm, G. L., and Henderson, J. M. (2010). Combining top-down processes to guide eye movements during real-world scene search. J. Vis. 10 (2), 1–11. doi:10.1167/10.2.4
Manning, D., Ethell, S., Donovan, T., and Crawford, T. (2006). How do radiologists do it? The influence of experience and training on searching for chest nodules. Radiography 12 (2), 134–142. doi:10.1016/j.radi.2005.02.003
Matthis, J. S., Yates, J. L., and Hayhoe, M. M. (2018). Gaze and the control of foot placement when walking in natural terrain. Curr. Biol. 28 (8), 1224–1233.e5. doi:10.1016/j.cub.2018.03.008
Mishkin, M., and Ungerleider, L. G. (1982). Contribution of striate inputs to the visuospatial functions of parieto-preoccipital cortex in monkeys. Behav. Brain Res. 6 (1), 57–77. doi:10.1016/0166-4328(82)90081-X
Neider, M. B., and Zelinsky, G. J. (2006). Scene context guides eye movements during visual search. Vis. Res. 46 (5), 614–621. doi:10.1016/j.visres.2005.08.025
Nicholls, M. E., and Roberts, G. R. (2002). Can free-viewing perceptual asymmetries be explained by scanning, pre-motor or attentional biases? Cortex 38 (2), 113–136. doi:10.1016/s0010-9452(08)70645-2
Nuthmann, A., and Canas-Bajo, T. (2022). Visual search in naturalistic scenes from foveal to peripheral vision: a comparison between dynamic and static displays. J. Vis. 22 (1), 10. doi:10.1167/jov.22.1.10
Nuthmann, A., and Matthias, E. (2014). Time course of pseudoneglect in scene viewing. Cortex 52, 113–119. doi:10.1016/j.cortex.2013.11.007
Ossandón, J. P., Onat, S., and König, P. (2014). Spatial biases in viewing behavior. J. Vis. 14 (2), 20. doi:10.1167/14.2.20
Püschel, A., Kilian, W., and Odenwald, S. (2022). “Heat on sight-display thermal radiation in the peripheral field of view,” in Adv. Mech. Design engin. Manufact. IV: proc. Intern. Joint conf. Mech. Design engin. Adv. Manufact, JCM 2022 (Cham: Springer), 1483–1494. doi:10.1007/978-3-031-15928-2_129
Rothkopf, C. A., Ballard, D. H., and Hayhoe, M. M. (2007). Task and context determine where you look. J. Vis. 7 7 (14), 16. doi:10.1167/7.14.16
Schmidt, J., and Zelinsky, G. J. (2009). Short article: search guidance is proportional to the categorical specificity of a target cue. Q. J. Exp. Psychol. (Hove). 62 (10), 1904–1914. doi:10.1080/17470210902853530
Schrom-Feiertag, H., Regal, G., Puthenkalam, J., and Suette, S. (2021). “Immersive experience prototyping: using mixed reality to integrate real devices in virtual simulated contexts to prototype experiences with mobile apps,” in 2021 IEEE Int. Symp. Mixed Augm. Reality Adj. (IEEE), 75–81. doi:10.1109/ISMAR-Adjunct54149.2021.00025
Shi, Y., Kang, J., Xia, P., Tyagi, O., Mehta, R. K., and Du, J. (2021). Spatial knowledge and firefighters’ wayfinding performance: a virtual reality search and rescue experiment. Saf. Sci. 139, 105231. doi:10.1016/j.ssci.2021.105231
Spalek, T. M., and Hammad, S. (2005). The left-to-right bias in inhibition of return is due to the direction of reading. Psychol. Sci. 16 (1), 15–18. doi:10.1111/j.0956-7976.2005.00774.x
Streefkerk, J. W., Vos, W., and Smets, N. (2012). “Evaluating a multimodal interface for firefighting rescue tasks,” in Proc. Hum. Fact. Ergon. Soc. Ann. Meet (Los Angeles: SAGE), 277–281. doi:10.1177/1071181312561054
Tatler, B. W., and Tatler, S. L. (2013). The influence of instructions on object memory in a real-world setting. J. Vis. 13 (2), 5. doi:10.1167/13.2.5
Torralba, A., Oliva, A., Castelhano, M. S., and Henderson, J. M. (2006). Contextual guidance of eye movements and attention in real-world scenes: the role of global features in object search. Psychol. Rev. 113 (4), 766–786. doi:10.1037/0033-295x.113.4.766
Treisman, A. M., and Gelade, G. (1980). A feature-integration theory of attention. Cogn. Psychol. 12 (1), 97–136. doi:10.1016/0010-0285(80)90005-5
Trepkowski, C., Eibich, D., Maiero, J., Marquardt, A., Kruijff, E., and Feiner, S. (2019). “The effect of narrow field of view and information density on visual search performance in augmented reality,” in 2019 IEEE Conf. Virtual Real. 3D User Interface (IEEE), 575–584. doi:10.1109/VR.2019.8798312
't Hart, B. M., and Einhäuser, W. (2012). Mind the step: complementary effects of an implicit task on eye and head movements in real-life gaze allocation. Exp. Brain Res. 223 (2), 233–249. doi:10.1007/s00221-012-3254-x
't Hart, B. M., Vockeroth, J., Schumann, F., Bartl, K., Schneider, E., König, P., et al. (2009). Gaze allocation in natural stimuli: comparing free exploration to head-fixed viewing conditions. Vis. Cogn. 17 (6-7), 1132–1158. doi:10.1080/13506280902812304
Vater, C., Wolfe, B., and Rosenholtz, R. (2022). Peripheral vision in real-world tasks: a systematic review. Psychon. Bull. Rev. 29, 1531–1557. doi:10.3758/s13423-022-02117-w
Vickery, T. J., King, L. W., and Jiang, Y. (2005). Setting up the target template in visual search. J. Vis. 5 (1), 8. doi:10.1167/5.1.8
Võ, M.L.-H., and Henderson, J. M. (2009). Does gravity matter? Effects of semantic and syntactic inconsistencies on the allocation of attention during scene perception. J. Vis. 9 (3), 24. doi:10.1167/9.3.24
Wang, R., Lu, H., Xiao, J., Li, Y., and Qiu, Q. (2018). “The design of an augmented reality system for urban search and rescue,” in 2018 IEEE Intern. Conf. Intell. Safety Robot (IEEE), 267–272. doi:10.1109/IISR.2018.8535823
Warden, A. C., Wickens, C. D., Mifsud, D., Ourada, S., Clegg, B. A., and Ortega, F. R. (2022). “Visual search in augmented reality: effect of target cue type and location,” in Proc. Hum. Fact. Ergon. Soc. Ann. Meet (Los Angeles: SAGE), 373–377. doi:10.1177/1071181322661260
Wheeler, S. G., Engelbrecht, H., and Hoermann, S. (2021). Human factors research in immersive virtual reality firefighter training: a systematic review. Front. Virtual Real. 2, 671664. doi:10.3389/frvir.2021.671664
Wolf, F., Soni, P., Kuber, R., Pawluk, D., and Turnage, B. (2019). “Addressing the situational impairments encountered by firefighters through the design of alerts,” in Proc. 16th Intern. Web for All Conf (New York: ACM), 22. doi:10.1145/3315002.3317556
Wolfe, J. M. (2021). Guided Search 6.0: an updated model of visual search. Psychon. Bull. Rev. 28 (4), 1060–1092. doi:10.3758/s13423-020-01859-9
Wolfe, J. M., Brunelli, D. N., Rubinstein, J., and Horowitz, T. S. (2013). Prevalence effects in newly trained airport checkpoint screeners: trained observers miss rare targets, too. J. Vis. 13 (3), 33. doi:10.1167/13.3.33
Wolfe, J. M., Cave, K. R., and Franzel, S. L. (1989). Guided search: an alternative to the feature integration model for visual search. J. Exp. Psychol. Hum. Percept. Perform. 15 (3), 419–433. doi:10.1037/0096-1523.15.3.419
Wolfe, J. M., Horowitz, T. S., and Kenner, N. M. (2005). Rare items often missed in visual searches. Nature 435, 439–440. doi:10.1038/435439a
Keywords: visual search, virtual reality, augmented reality, pseudoneglect, firefighting, digital twin
Citation: Feder S, Püschel A, Şimşek M, Odenwald S, Bendixen A and Einhäuser W (2024) Visual search for hazardous items: using virtual reality (VR) in laypersons to test wearable displays for firefighters. Front. Virtual Real. 5:1252351. doi: 10.3389/frvir.2024.1252351
Received: 03 July 2023; Accepted: 16 October 2024;
Published: 11 November 2024.
Edited by:
Diego Vilela Monteiro, ESIEA University, FranceReviewed by:
Georg Regal, Austrian Institute of Technology (AIT), AustriaCopyright © 2024 Feder, Püschel, Şimşek, Odenwald, Bendixen and Einhäuser. This is an open-access article distributed under the terms of the Creative Commons Attribution License (CC BY). The use, distribution or reproduction in other forums is permitted, provided the original author(s) and the copyright owner(s) are credited and that the original publication in this journal is cited, in accordance with accepted academic practice. No use, distribution or reproduction is permitted which does not comply with these terms.
*Correspondence: Sascha Feder, c2FzY2hhLmZlZGVyQHBoeXNpay50dS1jaGVtbml0ei5kZQ==
Disclaimer: All claims expressed in this article are solely those of the authors and do not necessarily represent those of their affiliated organizations, or those of the publisher, the editors and the reviewers. Any product that may be evaluated in this article or claim that may be made by its manufacturer is not guaranteed or endorsed by the publisher.
Research integrity at Frontiers
Learn more about the work of our research integrity team to safeguard the quality of each article we publish.