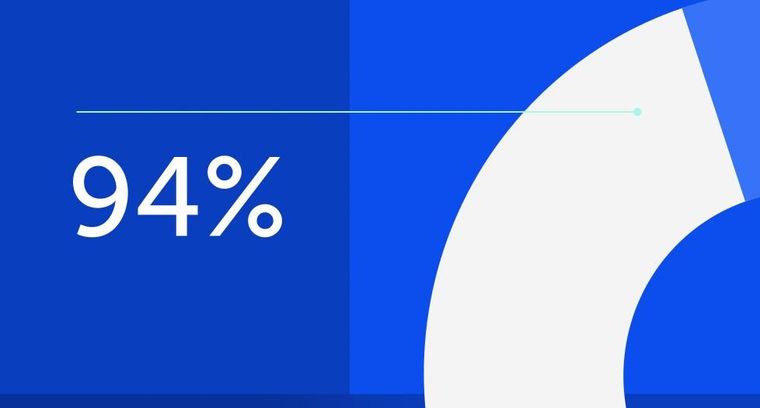
94% of researchers rate our articles as excellent or good
Learn more about the work of our research integrity team to safeguard the quality of each article we publish.
Find out more
ORIGINAL RESEARCH article
Front. Virtual Real., 10 January 2024
Sec. Virtual Reality and Human Behaviour
Volume 4 - 2023 | https://doi.org/10.3389/frvir.2023.1308133
This article is part of the Research TopicVirtual Reality for Diversity, Equity, and InclusionView all 4 articles
While gender differences have been studied in both real and virtual worlds separately, few studies have focused on multitasking in hybrid environments. This study investigated the gender factor in multitasking within a mixed reality environment. Thirty-six participants completed eight experimental conditions to assess their workload, task priority, and hand usage. Two distinct tasks were employed in the experiment: a block-matching task for the physical world, where participants located and matched English letters with their corresponding positions on a wooden board, and the N-back task for the virtual world. Four conditions focused on digital-physical monotasking, while the rest involved mixed-reality multitasking. The results reveal that perceived mental demand is a significant factor. Males prioritized virtual tasks, whereas females prioritized both tasks equally. Understanding the factors influencing gender-based performance differences can enhance the design of practical mixed reality applications, addressing equity and quality concerns. This study suggests that cognitive load plays a vital role in determining how genders perform when juggling multiple tasks, both physical and virtual.
We all find ourselves multitasking, whether at work or at home, to accomplish tasks efficiently. However, questions arise about the impact of multitasking on our mental workload and whether it differs based on gender (Grassini and Laumann, 2020). As our world becomes increasingly digital, with immersive technologies permeating various fields, there is a growing need for research on multitasking in digital-physical hybrid or mixed-reality environments. Mixed reality (MR) is defined as an environment that enables users to interact with a digital representation of real-world information, offering the advantages of both physical (real) and digital (virtual) worlds (North et al., 2021). While existing literature does not provide a universally accepted definition of MR, it is generally described as encompassing everything between two extremes: a fully realistic environment and a fully virtual one (Speicher et al., 2019). In essence, MR merges real-world elements with computer-generated constructs (Farshid et al., 2018). MR is often referred to as a type of hybrid system that incorporates both physical and virtual elements, terms like “digital-physical hybrid” and “mixed reality” are used interchangeably (Tang et al., 2020; Jeong et al., 2022).
Multitasking plays a vital role in our lives, as humans are expected to efficiently manage various activities simultaneously (Kaufman-Scarborough and Lindquist, 1999). In the past, individuals had limited access to technologies such as computers and phones. However, today’s technologies are no longer confined to a single location, allowing for multitasking in work contexts that were previously impossible. Due to the rapid advancements in technologies, human factors engineers play a crucial role in expanding the research field in parallel with the growth of these technologies to better understand the relationship between humans and such systems. Understanding how identity factors, such as gender, significantly affect individuals allows for potential adjustments in mixed reality settings. In the context of gender differences, numerous genuine arguments and debates exist regarding the distinctions, or lack thereof, between females and males (Halpern, 2000). These gender differences manifest in various aspects, including physical strengths, communication styles, and memory capacity. According to Sweller’s cognitive load theory, a high mental workload necessitates extra resource allocation for processing more information, and the presence of a large amount of information can increase our cognitive workload (Sweller, 1988). Given that mixed reality is a non-traditional environment, the objective is to explore the interaction of gender within this distinctive context.
In this current study, we selected four variables to assess gender differences within the context of our experimental setting: (1) workload, (2) accuracy, (3) task priority, and (4) hand usage. Workload is a crucial factor in any work activity (Widiastuti et al., 2020), and it is closely linked to task performance and workload (Hancock and Matthews, 2018). Understanding multitasking priorities is vital because it provides insights into which each gender prioritizes, whether in the real or virtual world. Since participants use their hands in both real and virtual tasks, and there is an interference effect when performing both cognitive and motor tasks simultaneously (Baddeley and Della Sala, 1996; Lindenberger et al., 2000), the hand use variable is essential for MR designers to consider. A common stereotype suggests that females are better at multitasking than males (Ingalhalikar et al., 2013; Szameitat et al., 2015; Hirnstein et al., 2018; Lui et al., 2020). Despite this popular belief, there is relatively little empirical evidence to determine whether gender differs in their everyday multitasking ability, and studies have shown inconsistent findings (Hirnstein et al., 2018). Additionally, a perceived gender difference in multitasking, in general, exists (Ren et al., 2009). This raises the question of whether gender differences are evident in a mixed-reality multitasking scenario. This study contributes to understanding whether gender differences play a role in the performance of hybrid world multitasking. Hence, we hypothesize that gender differences exist in mixed reality applications.
Previous literature has revealed a significant relationship between gender and mental workload (Hancock, 1988; Hancock et al., 1992), and gender differences have been observed in brain structures (Hines, 2011; Joel, 2012). Maccoby and Jacklin (1978) concluded that gender differences exist in cognitive abilities, including mathematical, spatial, and verbal skills. Therefore, we hypothesize the presence of gender differences in subjective workload during mixed-reality multitasking. Accuracy is a crucial performance measure (Walther and Moore, 2005), and gender has been linked to self-assessment accuracy (González-Betancor et al., 2019). Hence, we hypothesize that gender influences performance accuracy in mixed-reality multitasking. Lastly, participants use their hands in these experiments, and research has shown that the use of dominant or non-dominant hands can influence task performance (Vines et al., 2008; Noguchi et al., 2009; Schweiger et al., 2021) due to differences in motor abilities between genders (Čular et al., 2010).
While gender differences in multitasking have been studied exclusively (Ren et al., 2009; Mäntylä, 2013; Crews and Russ, 2020; Lui et al., 2020), limited research has investigated these differences in the context of mixed reality multitasking. Therefore, this study aims to establish a baseline for comparing multitasking performance between different genders in a mixed reality environment, as opposed to previous studies that focused on comparisons within either the real or virtual world. It also seeks to determine whether the well-known negative impact of multitasking on performance (Lascau et al., 2019) remains consistent in the case of multitasking compared to monotasking. To address these objectives, we have formulated three research questions (RQ) for this study:
RQ1. Does gender significantly affect human workload and performance in the physical (real) world, digital (virtual) world, and multitasking between both?
RQ2. Does gender significantly affect task priority in mixed-reality multitasking?
RQ3. Is there a gender difference in agreement between self-reported and observed hand use in these environments?
Numerous studies have investigated gender differences in VR applications (Hancock et al., 1992; Bayro et al., 2022). Zhao et al. (2017) conducted market research to gauge gender-specific mental workload in online shopping involving AR and VR technologies. Their findings revealed that males experienced significantly higher workloads than females. Chang (2020) delved into the effect of gender and other factors in a virtual reality driving simulation. The experimental results, supported by statistical analysis, demonstrated that gender played a significant role in task performance, with males outperforming females. Charkhandaz Yeganeh et al. (2019) conducted a study to explore gender effects on driving performance and mental workload using a virtual simulator. While they found no significant differences between males and females, females exhibited slightly weaker performance and perceived higher mental workloads compared to males.
Enochsson et al. (2004) conducted a study involving medical students using simulated laparoscopy and virtual reality simulators to compare right-handed males and females. Their research revealed that the performance of right-handed males was correlated with their visuospatial ability, which was not the case for females. While no significant differences were identified, it was apparent that task quality was best when executed by the dominant hand in right-handed males (Elneel et al., 2008). Peters and Durding (1979) conducted a study comparing right-handers and left-handers in a motor task involving finger tapping, with performance measured by speed and regularity. Their research revealed statistical differences in performance between the two groups. In our virtual-world task, participants were asked to tap on a virtual cube whenever they needed to respond to stimuli, potentially affecting their physical demands.
In another context, researchers have explored gender differences in the physical world. For example, a study conducted a spatial memory assessment based on gender by using a card-placing task (Baizan et al., 2019). The findings indicated that males outperformed females in this task. Rodríguez-López et al. (2021) conducted research investigating gender disparities in mental workload and burnout among fashion retailing workers, uncovering gender differences in these aspects. Similarly, Saylik et al. (2018) conducted a study similar to our virtual task experiment. They tasked participants with matching the color of a virtual cube with its previous appearance or one further back in time. In Saylik et al. (2018), both males and females were presented with a set of compounded stimuli consisting of two-colored shapes. Their performance was assessed based on task accuracy, revealing that males achieved higher accuracy rates than females. This led to the conclusion that cognitive functions exhibit sensitivity to gender differences.
The variable of handedness was identified as a factor affecting task performance. Misra et al. (1984) observed a statistical difference in reaction times between the right and left hands in both males and females, with males exhibiting faster responses overall. Furthermore, it was noted that the right hands responded more rapidly than the left hands, largely due to the right hands responded more rapidly than the left hands, largely due to the right-handed dominance among the participants in the study. In a study by Ruff and Parker (1993), two motor tasks, including a finger-tapping test, were implemented. They identified statistical differences in performance between genders, with women demonstrating notably slower performance.
Mixed reality aims to blend real and virtual environments, creating an environment where real and virtual content interact and coexist in real time (Bekele et al., 2018; Park et al., 2021). Lui et al. (2020) examined gender differences in multitasking on cognitive skills and found that males excelled in concurrent multitasking, while females were better at task switching. Hirsch et al. (2019) conducted a study testing gender performance in sequential and simultaneous multitasking with 96 subjects, measuring reaction time and accuracy. Surprisingly, their findings showed no significant difference. Most of the research in the literature on gender differences in multitasking has focused on either physical or virtual tasks. Hence, there is a gap in our understanding of gender roles in digital-physical multitasking.
The task priority variable is considered in mixed reality multitasking conditions, where participants had to prioritize one of the world tasks over the other. Choi et al. (2012) conducted a study to investigate whether gender stereotypes apply to virtual worlds. The findings showed that females tend to be more active in virtual worlds than males. According to McDonnell et al. (2021), most of the world’s population is right-handed, with only a minority of approximately 10.6% being left-handed. While this represents about 40 million left-handed individuals in the United States, left-handedness remains underrepresented in motor control research. Analyzing the hand-use variable in MR multitasking contributes to addressing this research limitation more effectively. Sokołowska (2021) conducted a modeling study of handedness to recognize dominant and non-dominant hands in both real and virtual conditions. The study found a statistically significant difference between dominant and non-dominant hands in both environments.
Despite the considerable interest in researching gender comparisons of multitasking, several studies have reported inconsistent findings. Surprisingly, gender-related differences in multitasking have received relatively less attention in research (Mäntylä, 2013; Strayer et al., 2013). Most studies have focused on gender differences in multitasking within daily life tasks or work-related contexts. However, considering the growing prevalence of AR/VR technologies, which have become some of the most critical emerging technologies today, it is imperative to investigate this issue more comprehensively. In much of the previous research, users’ performance in real-world first was typically compared to their performance in virtual environments to draw conclusions about which world yielded significant findings (Brade et al., 2017; Coutrot et al., 2019; Guerreiro et al., 2020; Hsu et al., 2021; Joyner et al., 2021). However, we found very limited research that directly addressed our specific area of interest: investigating gender differences in mixed reality environments. Consequently, conducting this study helps address this underexplored research area. Deloitte, a prominent consulting company, predicted that by 2025, over 14 million workers in the United States would be using smart glasses (Rogers, 2018). This projection highlights the fact that workers will increasingly perform both physical and digital tasks simultaneously while using these glasses. Given the growing potential of this technology, it is essential to understand its interaction with human users.
A power analysis was conducted using R studio to determine the sample size. Multiple effect sizes trials were conducted with a power of 80% and 90% respectively. An effect size of 0.5 and an alpha of 0.05 indicated that at least 28 participants were required to achieve a power of 0.8. The other trials yielded larger sample sizes which were out of our ability to collect with respect to budget and time. A total of 36 subjects, aged 19 to 43 (M = 23.9, SD = 4.22), participated in the experiment. We recruited an equal number of males and females, all with normal or corrected-to-normal vision and no hearing impairments. Participants reported varying levels of experience with AR/VR devices. Most had experience with mobile AR/VR technologies compared to wearable AR/VR devices. Only two participants were left-handed, while the rest were right-handed. They were compensated $15 in cash for their time. Ethical approval was obtained from the University of Illinois at Chicago’s Institutional Review Board (IRB# 2020-0466), and informed consent was obtained as necessary.
To assess gender differences in the physical world, we employed a block-matching task using educational materials (Getianlai Toys), consisting of English letter blocks and a board for pairing. This task was selected for its simplicity and capacity to yield data for our dependent variables. Participants were instructed to place pre-assigned blocks on the board within the 90-s time limit. Accuracy was measured to evaluate their performance, with the task imposing a visual search for English letters, thus influencing mental workload. For digital world assessment, we developed a virtual N-back task application compatible with the Microsoft HoloLens 2 device. We opted for this dynamic measure as individual differences in working memory can affect presence in virtual environments (Rawlinson et al., 2012). The N-back task is a well-known tool for assessing working memory capacity, involving recalling colors of a virtual cube displayed through the mixed reality headset and manipulated using hand gestures. The N parameter represents the number of steps requested to return to memory (Kirchner, 1958; Chen et al., 2008). The application of the N-back test used in our experiment was matching the colors of a cube that appears virtually in front of the participant through the mixed reality headset by using their hands. Two N parameters were used: N = 1 and N = 2. These tasks were chosen as they require both manual and cognitive processes, bridging the physical and virtual worlds. Figure 1 shows an illustration of the virtual N-back task. Participant performance was recorded using a video camera, and the headset was worn while seated for the virtual task. The experimental setup is depicted in Figure 2.
FIGURE 1. Illustration of the virtual N-back task showing 1-back and 2-back tasks. A virtual application of the N-back test with multiple trials of showing different colored virtual cubes in a specific time frame, where 1-back or 2-back represents the required response.
FIGURE 2. Experimental setup. (A) A scene from the experimenter’s point of view showing one participant wearing HoloLens and interacting with a virtual cube that is shown to the experimenter on a laptop screen, (B) A scene from the participant’s point of view while interacting with a virtual cube and pairing English letters to a wooden board simultaneously: ➀ Microsoft Hololens 2, ➁ real-world task material, ➂ virtual-world material.
We designed the experiment with eight conditions, as outlined in Table 1. These conditions were created by combining two difficulty levels: easy and hard. In the physical world task, the difficulty levels were determined by the order in which English letters were assigned to participants. Three different orders were used: forward-bottom-top (FBT), backward-top-bottom (BTB), and backward-bottom-top (BBT). FBT involved placing letters from N to Z and then from A to M, BTB from M to A and then Z to N, and BBT from Z to N and then M to A. These three orders constituted the hard level. The easy level in the physical task involved no specific order for placing the English letters. For the virtual world task, we employed two levels of the N-back test. N = 1 represented the easy level, while N = 2 represented the hard level. The order of the conditions was randomized to prevent experimenter bias. This study explores gender differences in performance in both real and virtual worlds, using “gender” as the independent variable (IV) to represent biological sex differences with two levels: males and females.
Participants were selected based on an eligibility survey to ensure the absence of hearing or visual impairments. Eligible participants completed a consent form and a demographic survey. Prior to the actual experiment, participants were given an introduction about the nature of the experiment and the different tasks, followed by the completion of two practice conditions. In the physical world task practice, participants paired numbers on a board within 30 s. For the virtual-world task practice, they tried the N-back test on a PC, experiencing both N = 1 and N = 2 levels. Participants interacted with virtual cubes and watched a demo video illustrating the virtual-world task. After each practice condition, participants completed a NASA-TLX survey. Each subject performed all eight conditions in a random order after practicing both physical and virtual conditions to get familiar with the experimental tasks. After each condition, they filled out a post-task survey, including a NASA-TLX survey and questions about hands used and task priority during multitasking. Participants were instructed to stop if the 90-s timer elapsed. Upon completing all conditions, they filled out a payment form. The total experiment duration averaged about 90 min per single participant. The whole experiment campaign lasted for about 3 months. Figure 3 shows the experiment’s flow.
FIGURE 3. The flow of the experiment. The procedure of the experiment is shown in a flow diagram. At first, participants signed a consent form and filled out a demographic survey which took about 10 min. Second, they practiced the experiment for about 5 min and then they filled a pairwise comparison survey for another 5 min. After practicing, they experimented on eight different conditions given in a random order for about 35 min, and they filled out the NASA-TLX survey after each condition which took a total time of about 30 min. In the end, they were paid for their time in cash. The total time taken per participant was about 90 min.
The NASA-TLX is a multidimensional tool used to assess perceived workload across six dimensions: mental demand, physical demand, temporal demand, performance, effort, and frustration. These dimensions collectively reflect the workload experienced during most tasks, defined as the physical and mental effort required (Hart and Staveland, 1988). Participants rate each dimension on a scale from 0 to 100. We calculated the weighted rating by first determining the count of each dimension from the pairwise comparison survey and then obtaining the sum product of each participant’s ratings with the count results.
We measured Total completion time (TCT) to determine the time each participant took to complete each condition. Each condition had a 90-s time limit, and participants were asked to stop if they reached this limit. Therefore, TCT served as a dependent variable only for single real-world tasks. In the other six conditions involving virtual-world tasks, a built-in timer of 90 s automatically stopped the test. The experimenter recorded each participant’s TCT in an Excel sheet during the experiment.
We assessed participant accuracy using two methods. For real-world tasks, we calculated accuracy by dividing the total number of correctly placed English letters on the wooden board by 26 (the total number of the English alphabet) and then multiplying the result by 100 to obtain a percentage. The experimenter recorded each participant’s total number of correctly placed letters in an Excel sheet. For virtual-world tasks, accuracy was determined by dividing the total number of correct answers by the total instances, and the results were multiplied by 100 to obtain a percentage. The number of correct and incorrect answers was displayed on a virtual screen through HoloLens. In the four multitasking conditions, where each condition involved both real-world and virtual-world tasks, we calculated the accuracy of each world task separately using the respective accuracy equations. We then averaged these two accuracies per condition to obtain the final accuracy of each multitasking condition. This approach ensured that differences in complexity between the multitasking conditions were considered.
Task priority is a qualitative measure used in this study to determine each participant’s priority in the four multitasking conditions. Participants were asked in the post-task survey which world task they prioritized during each multitasking session, and the frequency of their answers was analyzed using the Chi-square statistical test. These conditions were prioritized due to their dual-task nature, while the rest focused on single tasks. This measure aims to understand the participants’ perspectives regarding the importance of RW-VW tasks.
Two types of data were collected for this variable: (1) self-reported hand use from participants in post-task surveys and (2) observed hand use through visual inspection by the experimenter. Participants could select their right hand, left hand, or both hands. These findings are valuable for MR application designers, as many tools cater to right-handed users (Jung ad Jung, 2009). Additionally, studying the agreement or discrepancy between self-reported and observed hand use is essential for understanding the relationship between behavior and habit (da Cunha et al., 2019; Dale et al., 2010; Diefenbacher et al., 2019).
Eight conditions were conducted for each participant, resulting in a total of 288 datasets (8 conditions
To analyze these dependent variables, considering gender as an independent variable in this study, we employed the Mann-Whitney nonparametric test, which serves as a nonparametric alternative to the t-test (MacFarland and Yates, 2018). This test assessed the statistical significance of each variable and provided corresponding p-values. Effect sizes were calculated to gauge the extent to which gender influenced each dependent measure. Effect size estimates were interpreted using the correlation coefficient r, computed as r = |z|/√n, where n represents the total number of observations on which z is based (Tomczak and Tomczak, 2014). Since our independent variable had only two levels, no post hoc tests were conducted. Box plots were generated to visualize the distribution of quantitative values. For the task priority variable, the chi-square test was employed after verifying its assumptions. Additionally, a statistical correlation analysis was conducted on the hand usage variable to explore the relationship between self-reported and observed hand usage by both males and females across all conditions. All analyses were carried out using R Studio, with a significance level set at α = 0.05.
The results of this experiment were analyzed across the three divisions: single RW task, single VW task, and multitasking between both. In each division, gender was treated as an independent variable, and the reported p-values considered both difficulty levels (easy and hard). Employing the Mann-Whitney test, we observed a significant difference in gender for single RW tasks, specifically in the dimension of mental demand. Gender accounted for 6.32% of the variance in mental demand, resulting in a p-value of 0.03. On average, males reported a mental demand of 23.9%, while females reported 36.5% (See Figure 4A). However, the NASA-TLX subscales for physical demand (p = 0.07), temporal demand (p = 0.08), effort (p = 0.26), and performance (p = −0.06), as well as frustration (p = 0.06), showed no significant differences. The weighted rating measure was found to be statistically significant, yielding a p-value of 0.02, with an effect size of 8.27%. On average, males reported a calculated weighted rating of 32.3%, whereas females reported 44% (See Figure 4B). Surprisingly, no significant difference was found for the single virtual-world task. Mental demand had a p-value of 0.68, while physical demand had a p-value of 0.33. The p-values for temporal demand, effort, performance, and frustration were 0.5, 0.96, 0.79, and 0.64, respectively. Regarding multitasking between the two worlds, we found the NASA-TLX results were consistent with performing a single RW task. Significant differences were observed solely in the mental demand dimension, showing a p-value of 0.001 and an effect size of 0.077. This indicates that gender contributed to 7.73% of the variance in mental demand results during multitasking. On average, males reported a mental demand of 34.9%, while females reported 50.7% (See Figure 4C). However, the weighted rating here was not significant. The higher mental demand values were found in females compared to males, with an average MD of 23.9% for males, and 36.5% for females in the RW task setting, 34.9% for males, and 50.7% for females in the multitasking setting. The higher significance level of mental demand in multitasking compared to performing only a single RW task could be attributed to gender differences in primary cognitive abilities (Lauer et al., 2019).
FIGURE 4. Significant dependent measures of NASA-TLX. Three boxplots showing gender (male and female) on the x-axis and the percentages of the significant NASA-TLX subscales on the y-axis. (A) mental demand percentages data for single RW tasks experiment, (B) weighted rating data for single RW tasks experiment, (C) mental demand percentages data of multitasking experiment (ns: p > .05; *: p < .05; **: p < .01; ***: p < .001; ****: p < .0001).
When comparing the means for each task division, we found that males had the highest average score for the temporal demand of a single real-world task, while females scored highest in performance. The smallest difference in standard deviations for males and females was observed in the frustration dimension, with values of 21.27 and 17.16, respectively. In the case of a single virtual task, males achieved the highest average score in the performance subscale (48.74%), while females had the highest score in the physical demand subscale (49.91%). The smallest score differences were observed in the mental demand dimension for both males and females. Regarding the multitasking conditions, males had their highest average score (74.47%) in the effort subscale, while females had their highest average in the physical demand subscale (74.51%). Both males and females showed minimal score differences in the effort subscale, with values of 20.35 and 21.26, respectively. In terms of NASA-TLX subscales associations, we found the highest correlation between physical demand and effort for all the divisions–single RW task, single VW task, and multitasking–with correlation coefficients of 0.83, 0.71, and 0.68, respectively. You can refer to Figure 5 for the correlation matrices for each division.
FIGURE 5. Correlation matrix of NASA-TLX subscales. Three correlation plots, where the x-axis represents the six NASA-TLX subscales, and the y-axis represents correlation values ranging from −1 to 1. The circles inside the plot represent the correlation between every two subscales. Thus, the bigger and darker the circle, the larger the correlation. The numbers inside each circle represent the correlation coefficient value. (A) correlation of a single RW task, (B) correlation of a single VW task, and (C) correlation of MR multitasking.
The average completion time for males was 81.08 s, while females completed the tasks in an average of 75.92 s. This means that males took, on average, 5.16 s longer than females. However, the Mann-Whitney test conducted on the TCT results did not reveal a significant difference between genders, with a p-value of 0.08. This lack of significance may be attributed to the relatively small difference in task completion times between males and females.
The average accuracies for males were as follows: 90.69% for single RW tasks, 50% for single VW tasks, and 38.23% for MR multitasking. Notably, males performed best in RW tasks. In contrast, females achieved the following accuracies: 92.84%, 65.30%, and 41.12%, respectively. Visual observation of these percentages indicates that females outperformed males in all experimental conditions. The accuracy results were calculated based on each experimental condition overall for all participants. For single RW tasks, the average accuracy was 98.83% for the easy level and 84.72% for the hard level. In the case of single VW tasks, the average accuracy was 74.05% for the easy level and 41.26% for the hard level. Regarding RW-VW multitasking conditions, the average accuracy was as follows: 53.83% for easy RW-easy VW, 36.84% for easy RW-hard VW, 40.18% for hard RW-easy VW, and 27.87% for hard RW-hard VW. Upon testing these results with the Mann-Whitney test, we found no significant difference in any of the three experimental settings, as indicated by the resulting p-values of 0.45, 0.13, and 0.53, respectively.
The concept of task priority was exclusively considered for the multitasking conditions. Participants could choose one of three options: prioritizing the real-world task, the virtual-world task, or both tasks. In total, there were 144 datasets for the multitasking conditions (4 multitasking conditions
FIGURE 6. Selected priority of multitasking between RW-VW. A bar plot of each priority response selection frequency in the four multitasking conditions. The y-axis represents the frequencies of each selection by each gender, while the x-axis represents the gender (male and female). The x-axis is grouped based on each priority selection: either prioritizing real-world tasks, virtual-world tasks, or both world tasks equally.
All p-values from the correlation analysis of multitasking by males and females were less than 0.05 for self-reported and observed hand use. In the case of males, there was a moderate positive correlation (correlation coefficient = 0.67) between self-reported and observed hand use in the real-world part of multitasking. However, in the virtual world part, the correlation coefficient was 0.76, indicating a strong positive relationship. For females, both the real-world and virtual-world parts showed strong positive relationships, with correlation coefficients of 0.74 and 0.73, respectively. In summary, no significant differences were found between males and females or between the RW and VW tasks. Figure 7 illustrates the frequency distribution of self-reported and observed hand use for males and females, categorized by task type. It’s evident that most participants used their right hand when performing the virtual task, while a mixed hand was used for the real-world task.
FIGURE 7. Dominant hand used in multitasking. A bar plot of the frequencies of the hands used in the four multitasking conditions. Two types of frequencies were collected: observed dominant hand and self-reported dominant hand. The y-axis represents the frequencies of each hand selection, while the x-axis represents the gender (male and female). The x-axis is grouped based on each hand selection: right hand, left hand, or both hands. (A) RW part of the experiment, (B) VW part of the experiment.
This research aimed to determine the impact of gender differences on single RW tasks, single VW tasks, and multitasking between both, using workload, accuracy, task priority, and hand use as dependent measures. These variables were chosen to evaluate MR performance in males and females. Previous literature has shown that workload and accuracy can be useful parameters for assessing task performance under time pressure (Wang et al., 2016). Additionally, participants’ hand movements were considered a form of motor workload, which can affect performance (Silva, 2014). Gender differences have also been observed in finger-tapping tests, suggesting varied motor responses (Ruff and Parker, 1993). Regarding RQ1, we found significance only in mental demand for (1) performing a single RW task and (2) multitasking between RW and VW. Although the mental demand effect was found, as per participants’ self-rating, it aligns with evidence suggesting that biological sex can influence memory function (Loprinzi and Frith, 2018). Significant mental workload could have implications for the design of such environments, including task allocation, training, personalization, and more. This knowledge contributes to shaping future mixed reality experiences based on individual characteristics such as sex. Despite the general notion that males have faster reaction times and lower TCT, likely due to gender-based differences in motor responses (Nikam and Gadkari, 2015; Der et al., 2006), we found that females outperformed males with an average TCT of 75.92 s. Nevertheless, the TCT difference between males and females was found to be statistically insignificant. In examining accuracies during multitasking between real and virtual worlds, we observed that females exhibited a slight advantage over males, despite the non-significant results. This aligns with the stereotype that females excel at multitasking (Buser and Petet, 2011; Rippon, 2019), supporting this notion despite its statistical insignificance.
To address RQ2, we found a significant gender difference in task prioritization; males favored virtual tasks, while females equally prioritized both worlds’ tasks. Most participants self-reported as right-handed, but our analysis revealed varied hand usage for real-world tasks, inconsistent with their demographic information. In contrast, for virtual tasks, almost all participants used their right hands, aligning with their perceived dominant hand. Consequently, we found no significant gender difference in the correlation between self-reported and observed hand use (addressing RQ3). These findings may be influenced by previous research demonstrating cognitive differences between right and left-handed individuals (Cherbuin and Brinkman, 2006; Al-Hashel et al., 2016), as well as the potential impact of sex hormones on cognitive performance (Vidal et al., 2006).
We explored gender differences in subjective and objective measurements, including NASA-TLX, TCT, accuracy, task priority, and hands used. This study takes a step toward understanding gender disparities in multitasking within digital-physical hybrid environments and offers practical insights for MR designers considering gender-related factors in multitasking with MR technology. The tasks utilized in the study were designed to provide illustrative examples for practical applications. For instance, the block-matching task is applicable in operational settings that require visual-spatial skills, such as quality control in automotive manufacturing. In this context, workers use a block-matching task to examine and confirm the accurate positioning of specific components on vehicles. On the other hand, the virtual N-back task is advantageous for training workers to enhance their working memory, attention, and capacity to track multiple stimuli. Participants found multitasking between the two worlds more challenging than expected, likely due to its novelty and some participants’ lack of experience with AR/VR devices. Although this study focused on temporally overlapping task processing, the results provide valuable insights into human engagement with new technologies like mixed reality. For instance, MR designers could consider users’ dominant hands based on gender, and future work contexts may assign mixed-world tasks to female workers and virtual-world tasks to male workers, as suggested by the priority variable results. Gender criteria should be taken into account in job selection and work content (Abbasi et al., 2022). This paper opens the door to further research exploring the relationship between genders and MR multitasking; to provide a well-established technological setting based on gender.
It’s important to note some limitations of this study. First, our participant pool primarily consisted of university students aged 19 to 26, potentially limiting the generalizability of results. Second, we could only assess the TCT variable for single real-world tasks due to the automated timer for the virtual task. Third, we did not include a feedback question in the survey, which could have improved the procedure for future subjects. Lastly, while we used difficulty levels (easy and hard) to form different condition combinations, future research should investigate how more advanced multitasking experiments between real and virtual worlds impact gender performance.
The raw data supporting the conclusion of this article will be made available by the authors, without undue reservation.
The studies involving humans were approved by the University of Illinois at Chicago’s Institutional Review Board (IRB# 2020-0466). The studies were conducted in accordance with the local legislation and institutional requirements. The participants provided their written informed consent to participate in this study.
SA: Conceptualization, Data curation, Formal Analysis, Funding acquisition, Investigation, Methodology, Writing–original draft, Writing–review and editing. HJ: Conceptualization, Funding acquisition, Investigation, Methodology, Supervision, Writing–review and editing.
The author(s) declare financial support was received for the research, authorship, and/or publication of this article. This research was supported by the College of Engineering at the University of Illinois at Chicago and the first author’s Saudi Arabian Cultural Mission (SACM) fellowship.
The authors would like to thank Bryan Havens and Yalda Ghasemi for providing initial insights to collect the data and Edison Larco for developing the interface.
The authors declare that the research was conducted in the absence of any commercial or financial relationships that could be construed as a potential conflict of interest.
All claims expressed in this article are solely those of the authors and do not necessarily represent those of their affiliated organizations, or those of the publisher, the editors and the reviewers. Any product that may be evaluated in this article, or claim that may be made by its manufacturer, is not guaranteed or endorsed by the publisher.
Abbasi, A. M., Darvishi, E., Rodrigues, M. A., and Sayehmiri, K. (2022). Gender differences in cognitive performance and psychophysiological responses during Noise Exposure and different workloads. Appl. Acoust. 189, 108602. doi:10.1016/j.apacoust.2021.108602
Al-Hashel, J. Y., Ahmed, S. F., Al-Mutairi, H., Hassan, S., Al-Awadhi, N., and Al-Saraji, M. (2016). Association of cognitive abilities and brain lateralization among primary school children in Kuwait. Neurosci. J. 2016, 1–5. doi:10.1155/2016/6740267
Baddeley, A. D., and Della Sala, S. (1996). Working memory and executive control. Philosophical Trans. R. Soc. Lond. Ser. B Biol. Sci. 351 (1346), 1397–1403. doi:10.1098/rstb.1996.0123
Bayro, A., Dai, T., and Jeong, H. (2022). “Does gender influence presence in virtual environments? An analysis of open-source igroup presence questionnaire data,” in 2022 IEEE 3rd International Conference on Human-Machine Systems (ICHMS) (IEEE), 1–6. doi:10.1109/ICHMS56717.2022.9980589
Bekele, M. K., Pierdicca, R., Frontoni, E., Malinverni, E. S., and Gain, J. (2018). A survey of augmented, virtual, and mixed reality for Cultural Heritage. J. Comput. Cult. Herit. 11 (2), 1–36. doi:10.1145/3145534
Brade, J., Lorenz, M., Busch, M., Hammer, N., Tscheligi, M., and Klimant, P. (2017). Being there again – presence in real and virtual environments and its relation to usability and user experience using a mobile navigation task. Int. J. Human-Computer Stud. 101, 76–87. doi:10.1016/j.ijhcs.2017.01.004
Buser, T., and Peter, N. (2011). Multitasking: productivity effects and gender differences. SSRN Electron. J. doi:10.2139/ssrn.1766552
Chang, W. T. (2020). The effects of age, gender, and control device in a virtual reality driving simulation. Symmetry 12 (6), 995. doi:10.3390/sym12060995
Charkhandaz Yeganeh, R., Ebrahimi, H., Alimohammadi, I., and Khalilzadeh Ranjbar, G. (2019). Survey of gender effect on driving performance and mental workload of young drivers using a driving simulator. Iran. Occup. Health 16 (1), 47–59.
Chen, Y.-N., Mitra, S., and Schlaghecken, F. (2008). Sub-processes of working memory in the N-back task: an investigation using ERPs. Clin. Neurophysiol. 119 (7), 1546–1559. doi:10.1016/j.clinph.2008.03.003
Cherbuin, N., and Brinkman, C. (2006). Hemispheric interactions are different in left-handed individuals. Neuropsychology 20 (6), 700–707. doi:10.1037/0894-4105.20.6.700
Choi, G., Chung, H., and Kim, Y. (2012). Are stereotypes relative to gender usage applicable to virtual worlds? Int. J. Human-Computer Interact. 28 (6), 399–405. doi:10.1080/10447318.2011.601973
Coutrot, A., Schmidt, S., Coutrot, L., Pittman, J., Hong, L., Wiener, J. M., et al. (2019). Virtual navigation tested on a mobile app is predictive of real-world wayfinding navigation performance. PLOS ONE 14 (3), 02132722–e213312. doi:10.1371/journal.pone.0213272
Crews, D. E., and Russ, M. J. (2020). The impact of individual differences on multitasking ability. Int. J. Prod. Perform. Manag. 69 (6), 1301–1319. doi:10.1108/ijppm-04-2019-0191
Čular, D., Miletić, Đ., and Miletić, A. (2010). Influence of dominant and non-dominant body side on specific performance in taekwondo. Kinesiology 42 (2), 184–193.
da Cunha, D. T., de Rosso, V. V., Pereira, M. B., and Stedefeldt, E. (2019). The differences between observed and self-reported food safety practices: a study with food handlers using structural equation modeling. Food Res. Int. 125, 108637. doi:10.1016/j.foodres.2019.108637
Dale, A. M., Strickland, J., Gardner, B., Symanzik, J., and Evanoff, B. A. (2010). Assessing agreement of self-reported and observed physical exposures of the upper extremity. Int. J. Occup. Environ. Health 16 (1), 1–10. doi:10.1179/oeh.2010.16.1.1
Der, G., and Deary, I. J. (2006). Age and sex differences in reaction time in adulthood: results from the United Kingdom health and lifestyle survey. Psychology and Aging 21 (1), 62–73. doi:10.1037/0882-7974.21.1.62
Diefenbacher, S., Pfattheicher, S., and Keller, J. (2019). On the role of habit in self-reported and observed hand hygiene behavior. Appl. Psychol. Health Well-Being 12 (1), 125–143. doi:10.1111/aphw.12176
Elneel, F. H., Carter, F., Tang, B., and Cuschieri, A. (2008). Extent of innate dexterity and ambidexterity across handedness and gender: implications for training in laparoscopic surgery. Surg. Endosc. 22 (1), 31–37. doi:10.1007/s00464-007-9533-0
Enochsson, L., Isaksson, B., Tour, R., Kjellin, A., Hedman, L., Wredmark, T., et al. (2004). Visuospatial skills and computer game experience influence the performance of virtual endoscopy. J. Gastrointest. Surg. 8 (7), 874–880. doi:10.1016/j.gassur.2004.06.015
Farshid, M., Paschen, J., Eriksson, T., and Kietzmann, J. (2018). Go boldly!: explore augmented reality (AR), virtual reality (VR), and mixed reality (MR) for business. Bus. Horizons 61 (5), 657–663. doi:10.1016/j.bushor.2018.05.009
González-Betancor, S. M., Bolívar-Cruz, A., and Verano-Tacoronte, D. (2019). Self-assessment accuracy in higher education: the influence of gender and performance of University Students. Act. Learn. High. Educ. 20 (2), 101–114. doi:10.1177/1469787417735604
Grassini, S., and Laumann, K. (2020). Are modern head-mounted displays sexist? A systematic review on gender differences in HMD-mediated virtual reality. Front. Psychol. 11, 1604. doi:10.3389/fpsyg.2020.01604
Guerreiro, J., Sato, D., Ahmetovic, D., Ohn-Bar, E., Kitani, K. M., and Asakawa, C. (2020). Virtual navigation for blind people: transferring route knowledge to the real-world. Int. J. Human-Computer Stud. 135, 102369–102412. doi:10.1016/j.ijhcs.2019.102369
Halpern, D. F. (2000). Sex differences in cognitive abilities. Third edition. Lawrence Erlbaum Associates, Inc.
Hancock, P. A. (1988). The effect of gender and time of day upon the subjective estimate of mental workload during the performance of a simple task. Adv. Psychol., 239–250. doi:10.1016/s0166-4115(08)62390-0
Hancock, P. A., and Matthews, G. (2018). Workload and performance: associations, insensitivities, and dissociations. Hum. Factors J. Hum. Factors Ergonomics Soc. 61 (3), 374–392. doi:10.1177/0018720818809590
Hancock, P. A., Vercruyssen, M., and Rodenburg, G. J. (1992). The effect of gender and time-of-day on time perception and mental workload. Curr. Psychol. 11 (3), 203–225. doi:10.1007/bf02686841
Hart, S. G., and Staveland, L. E. (1988). Development of NASA-TLX (task load index): results of empirical and theoretical research. Adv. Psychol., 139–183. doi:10.1016/s0166-4115(08)62386-9
Hines, M. (2011). Gender development and the human brain. Annu. Rev. Neurosci. 34 (1), 69–88. doi:10.1146/annurev-neuro-061010-113654
Hirnstein, M., Larøi, F., and Laloyaux, J. (2018). No sex difference in an everyday multitasking paradigm. Psychol. Res. 83 (2), 286–296. doi:10.1007/s00426-018-1045-0
Hirsch, P., Koch, I., and Karbach, J. (2019). Putting a stereotype to the test: the case of gender differences in multitasking costs in task-switching and dual-task situations. PLOS ONE 14 (8), 02201500–e220216. doi:10.1371/journal.pone.0220150
Hsu, C.-H., Chung, C.-H., Venkatakrishnan, R., Venkatakrlshnan, R., Wang, Y.-S., and Babu, S. V. (2021). “Comparative evaluation of digital writing and art in real and immersive virtual environments,” in 2021 IEEE Virtual Reality and 3D User Interfaces (VR), 635–642. doi:10.1109/vr50410.2021.00089
Ingalhalikar, M., Smith, A., Parker, D., Satterthwaite, T. D., Elliott, M. A., Ruparel, K., et al. (2013). Sex differences in the structural connectome of the human brain. Proc. Natl. Acad. Sci. 111 (2), 823–828. doi:10.1073/pnas.1316909110
Jeong, H., Bayro, A., Umesh, S. P., Mamgain, K., and Lee, M. (2022). Social media users’ perceptions of a wearable mixed reality headset during the COVID-19 pandemic: aspect-based sentiment analysis. JMIR Serious Games 10 (3), e36850. doi:10.2196/36850
Joel, D. (2012). Genetic-gonadal-genitals sex (3G-sex) and the misconception of brain and gender, or, why 3G-males and 3G-females have intersex brain and intersex gender. Biol. Sex Differ. 3 (1), 27. doi:10.1186/2042-6410-3-27
Joyner, J. S., Vaughn-Cooke, M., and Benz, H. L. (2021). Comparison of dexterous task performance in virtual reality and real-world environments. Front. Virtual Real. 2, 64–81. doi:10.3389/frvir.2021.599274
Jung, H. S., and Jung, H.-S. (2009). Hand dominance and hand use behaviour reported in a survey of 2437 Koreans. Ergonomics 52 (11), 1362–1371. doi:10.1080/00140130903067805
Kaufman-Scarborough, C., and Lindquist, J. D. (1999). Time management and polychronicity. J. Manag. Psychol. 14 (3/4), 288–312. doi:10.1108/02683949910263819
Kirchner, W. K. (1958). Age differences in short-term retention of rapidly changing information. J. Exp. Psychol. 55 (4), 352–358. doi:10.1037/h0043688
Lascau, L., Gould, S. J., Cox, A. L., Karmannaya, E., and Brumby, D. P. (2019). “Monotasking or multitasking,” in Proceedings of the 2019 CHI Conference on Human Factors in Computing Systems. doi:10.1145/3290605.3300649
Lauer, J. E., Yhang, E., and Lourenco, S. F. (2019). The development of gender differences in spatial reasoning: a meta-analytic review. Psychol. Bull. 145 (6), 537–565. doi:10.1037/bul0000191
Lindenberger, U., Marsiske, M., and Baltes, P. B. (2000). Memorizing while walking: increase in dual-task costs from young adulthood to old age. Psychol. Aging 15 (3), 417–436. doi:10.1037/0882-7974.15.3.417
Loprinzi, P., and Frith, E. (2018). The role of sex in memory function: considerations and recommendations in the context of exercise. J. Clin. Med. 7 (6), 132. doi:10.3390/jcm7060132
Lui, K. F. H., Yip, K. H. M., and Wong, A. C.-N. (2020). Gender differences in multitasking experience and performance. Q. J. Exp. Psychol. 74 (2), 344–362. doi:10.1177/1747021820960707
Maccoby, E. E., and Jacklin, C. N. (1978). The psychology of sex differences, 2. Stanford University Press.
MacFarland, T. W., and Yates, J. M. (2018). Introduction to nonparametric statistics for the Biological Sciences using R. Springer International Publishing.
Mäntylä, T. (2013). Gender differences in multitasking reflect spatial ability. Psychol. Sci. 24 (4), 514–520. doi:10.1177/0956797612459660
McDonnell, J., Murray, N. P., Ahn, S., Clemens, S., Everhart, E., and Mizelle, J. C. (2021). Examination and comparison of theta band connectivity in left- and right-hand dominant individuals throughout a motor skill acquisition. Symmetry 13 (4), 728. doi:10.3390/sym13040728
Misra, N., Mahajan, K. K., and Maini, B. K. (1984). Comparative study of visual and auditory reaction time of hands and feet in males and females. Ind. J. Physiol. P'larmac 29, 213–218.
Nikam, L. H., and Gadkari, J. V. (2015). Effect of age, gender and body mass index on visual and auditory reaction times in Indian population. Indian j.physiol. Pharmacol. 5, 124–127.
Noguchi, T., Demura, S., Nagasawa, Y., and Uchiyama, M. (2009). Influence of measurement order by dominant and nondominant hands on performance of a pursuit-rotor task. Percept. Mot. Ski. 108 (3), 905–914. doi:10.2466/pms.108.3.905-914
North, S., North, M., Garofalo, D., and Parajapati, D. (2021). The effects of mixed reality immersion on users’ performance and perception of multitasking while performing concurrent real world tasks. J. Comput. Sci. Coll. 36 (8), 73–88. doi:10.5555/3470135.3470142
Park, K. B., Choi, S. H., Lee, J. Y., Ghasemi, Y., Mohammed, M., and Jeong, H. (2021). Hands-free human–robot interaction using multimodal gestures and deep learning in wearable mixed reality. IEEE Access 9, 55448–55464. doi:10.1109/access.2021.3071364
Peters, M., and Durding, B. (1979). Left-handers and right-handers compared on a motor task. J. Mot. Behav. 11 (2), 103–111. doi:10.1080/00222895.1979.10735178
Rawlinson, T. G., Lu, S., and Coleman, P. (2012). Individual differences in working memory capacity and presence in virtual environments. Adv. Brain Inspired Cognitive Syst., 22–30. doi:10.1007/978-3-642-31561-9_3
Ren, D., Zhou, H., and Fu, X. (2009). “A deeper look at gender difference in multitasking: gender-specific mechanism of cognitive control,” in 2009 Fifth International Conference on Natural Computation, 13–17. doi:10.1109/icnc.2009.542
Rippon, G. (2019). Do women and men have different brains? New Sci. 241 (3219), 28–31. doi:10.1016/s0262-4079(19)30376-8
Rodríguez-López, A. M., Rubio-Valdehita, S., and Díaz-Ramiro, E. M. (2021). Influence of the COVID-19 pandemic on Mental Workload and burnout of fashion retailing workers in Spain. Int. J. Environ. Res. Public Health 18 (3), 983. doi:10.3390/ijerph18030983
Rogers, S. (2018). What is mixed reality and what does it mean for enterprise? Forbes. from https://www.forbes.com/sites/solrogers/2018/12/04/what-is-mixed-reality-and-what-does-it-mean-for-enterprise/?sh=497b4fa15df9 (Accessed July 17, 2022).
Ruff, R. M., and Parker, S. B. (1993). Gender- and age-specific changes in motor speed and eye-hand coordination in adults: normative values for the finger tapping and grooved pegboard tests. Percept. Mot. Ski. 76 (3), 1219–1230. doi:10.2466/pms.1993.76.3c.1219
Saylik, R., Raman, E., and Szameitat, A. J. (2018). Sex differences in emotion recognition and working memory tasks. Front. Psychol. 9, 1072. doi:10.3389/fpsyg.2018.01072
Schweiger, D., Stone, R., and Genschel, U. (2021). Nondominant Hand Computer Mouse training and the bilateral transfer effect to the dominant hand. Sci. Rep. 11 (1), 4211. doi:10.1038/s41598-021-83770-4
Silva, F. P. (2014). Mental workload, task demand and driving performance: what relation? Procedia - Soc. Behav. Sci. 162, 310–319. doi:10.1016/j.sbspro.2014.12.212
Sokołowska, B. (2021). A novel perspective for examining and comparing real and virtual test tasks performed by the dominant and non-dominant hand in healthy adults. Symmetry 13 (10), 1810. doi:10.3390/sym13101810
Speicher, M., Hall, B. D., and Nebeling, M. (2019). “What is mixed reality?,” in Proceedings of the 2019 CHI Conference on Human Factors in Computing Systems. doi:10.1145/3290605.3300767
Strayer, D. L., Medeiros-Ward, N., and Watson, J. M. (2013). Gender invariance in multitasking. Psychol. Sci. 24 (5), 809–810. doi:10.1177/0956797612465199
Szameitat, A. J., Hamaida, Y., Tulley, R. S., Saylik, R., and Otermans, P. C. (2015). “Women are better than men”–public beliefs on gender differences and other aspects in multitasking. PLOS ONE 10 (10), 01403711–e140426. doi:10.1371/journal.pone.0140371
Tang, Y. M., Au, K. M., Lau, H. C., Ho, G. T., and Wu, C. H. (2020). Evaluating the effectiveness of learning design with mixed reality (MR) in Higher Education. Virtual Real. 24 (4), 797–807. doi:10.1007/s10055-020-00427-9
Tomczak, M., and Tomczak, E. (2014). The need to report effect size estimates revisited. An overview of some recommended measures of effect size. Trends Sport Sci. 1 (21), 19–25.
Vidal, A., Puig, O., Boget, T., Salamero, M., and Salamero, M. (2006). Gender differences in cognitive functions and influence of sex hormones. Actas Esp. Psiquiatr. 34 (6), 408–415.
Vines, B. W., Nair, D., and Schlaug, G. (2008). Modulating activity in the motor cortex affects performance for the two hands differently depending upon which hemisphere is stimulated. Eur. J. Neurosci. 28 (8), 1667–1673. doi:10.1111/j.1460-9568.2008.06459.x
Walther, B. A., and Moore, J. L. (2005). The concepts of bias, precision and accuracy, and their use in testing the performance of species richness estimators, with a literature review of estimator performance. Ecography 28 (6), 815–829. doi:10.1111/j.2005.0906-7590.04112.x
Wang, L., He, X., and Chen, Y. (2016). Quantitative relationship model between workload and time pressure under different flight Operation Tasks. Int. J. Industrial Ergonomics 54, 93–102. doi:10.1016/j.ergon.2016.05.008
Widiastuti, R., Nurhayati, E., Wardani, D. P., and Sutanta, E. (2020). Workload measurement of batik workers at UKM batik jumputan Yogyakarta using Rula and NASA-TLX. J. Phys. Conf. Ser. 1456 (1), 012032. doi:10.1088/1742-6596/1456/1/012032
Keywords: gender differences, multitasking, monotasking, digital-physical, hybrid, user experience, workload
Citation: Abbas S and Jeong H (2024) Unveiling gender differences: a mixed reality multitasking exploration. Front. Virtual Real. 4:1308133. doi: 10.3389/frvir.2023.1308133
Received: 05 October 2023; Accepted: 12 December 2023;
Published: 10 January 2024.
Edited by:
Yi (Joy) Li, Kennesaw State University, United StatesReviewed by:
Meredith Carroll, Florida Institute of Technology, United StatesCopyright © 2024 Abbas and Jeong. This is an open-access article distributed under the terms of the Creative Commons Attribution License (CC BY). The use, distribution or reproduction in other forums is permitted, provided the original author(s) and the copyright owner(s) are credited and that the original publication in this journal is cited, in accordance with accepted academic practice. No use, distribution or reproduction is permitted which does not comply with these terms.
*Correspondence: Heejin Jeong, aGVlamluLmplb25nQGFzdS5lZHU=
Disclaimer: All claims expressed in this article are solely those of the authors and do not necessarily represent those of their affiliated organizations, or those of the publisher, the editors and the reviewers. Any product that may be evaluated in this article or claim that may be made by its manufacturer is not guaranteed or endorsed by the publisher.
Research integrity at Frontiers
Learn more about the work of our research integrity team to safeguard the quality of each article we publish.