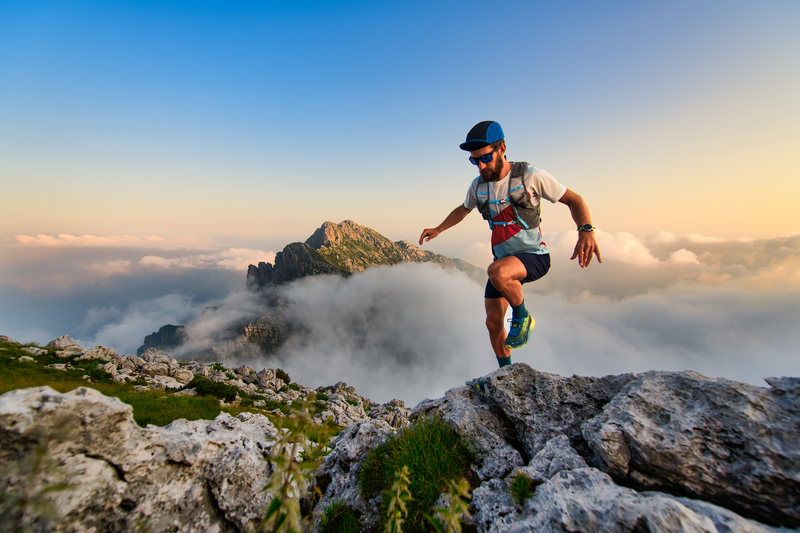
95% of researchers rate our articles as excellent or good
Learn more about the work of our research integrity team to safeguard the quality of each article we publish.
Find out more
ORIGINAL RESEARCH article
Front. Virtual Real. , 07 November 2023
Sec. Virtual Reality and Human Behaviour
Volume 4 - 2023 | https://doi.org/10.3389/frvir.2023.1210303
Introduction: Incorporating an additional limb that synchronizes with multiple body parts enables the user to achieve high task accuracy and smooth movement. In this case, the visual appearance of the wearable robotic limb contributes to the sense of embodiment. Additionally, the user’s motor function changes as a result of this embodiment. However, it remains unclear how users perceive the attribution of the wearable robotic limb within the context of multiple body parts (perceptual attribution), and the impact of visual similarity in this context remains unknown.
Methods: This study investigated the perceptual attribution of a virtual robotic limb by examining proprioceptive drift and the bias of visual similarity under the conditions of single body part (synchronizing with hand or foot motion only) and multiple body parts (synchronizing with average motion of hand and foot). Participants in the conducted experiment engaged in a point-to-point task using a virtual robotic limb that synchronizes with their hand and foot motions simultaneously. Furthermore, the visual appearance of the end-effector was altered to explore the influence of visual similarity.
Results: The experiment revealed that only the participants’ proprioception of their foot aligned with the virtual robotic limb, while the frequency of error correction during the point-to-point task did not change across conditions. Conversely, subjective illusions of embodiment occurred for both the hand and foot. In this case, the visual appearance of the robotic limbs contributed to the correlations between hand and foot proprioceptive drift and subjective embodiment illusion, respectively.
Discussion: These results suggest that proprioception is specifically attributed to the foot through motion synchronization, whereas subjective perceptions are attributed to both the hand and foot.
The primary objective of the implementation of wearable robotic limbs is the realization of human prosthetics (Schiefer et al., 2015; Marasco et al., 2018) and augmentation (Llorens Bonilla et al., 2012; Parietti and Asada, 2014). Human prosthetics aim to restore the function of existing limbs or body parts, while human augmentation aims to extend or enhance motor function and capabilities by adding limbs or body parts that are not naturally present in the body. Wearable robotic limbs designed for human augmentation overcome the inherent physical and spatial limitations of the human body and have evolved alongside advances in human augmentation technology (Prattichizzo et al., 2021). These limbs interact with the user through a motion synchronization system for the user’s body parts (Kojima et al., 2017; Sasaki et al., 2017). When users embody such wearable robotic limbs as part of their own bodies (Gallagher, 2000), they experience improved task performance (Llorens Bonilla et al., 2012; Sasaki et al., 2017). In this process, users develop a sense of manipulation over the wearable robotic limbs (sense of agency). In addition, wearable robotic limbs that synchronize the dynamics of users’ movements enable a sense of integration with the user’s body (sense of body ownership). Motor synchronization and haptic feedback are well-known methods to induce the sense of embodiment (Kalckert and Ehrsson, 2014; Kokkinara et al., 2015). In particular, the congruence between visual and motor information is a crucial factor in eliciting a sense of agency and body ownership (Farrer et al., 2008). These subjective sensations also arise in relation to virtual objects (Sanchez-Vives et al., 2010). Virtual reality (VR) provides the environment to manipulate the alignment between the users’ visual and motor information. Therefore, virtual robotic limbs have been employed as a reference to investigate the embodiment of wearable robotic limbs (Takizawa et al., 2019; Sakurada et al., 2022).
When users manipulate wearable robotic limbs that are synchronized with their motions, this induces changes in their motor functions (Dingwell et al., 2002). As users adapt to the new visuomotor feedback generated by controlling the wearable robotic limbs, they update their motor function (Mazzoni and Krakauer, 2006; Kasuga et al., 2015). These changes indicate that the users are adapting their motor models to the robotic limbs. In this process, the trajectory of the users’ body parts serves as an objective measure of their movement changes. Therefore, the trajectory serves as a reference for evaluating user motor function during the manipulation of wearable robotic limbs (Kasuga et al., 2015; Hagiwara et al., 2020).
During the embodiment process facilitated by motion synchronization with wearable robotic limbs, users perceive these limbs as integral parts of their bodies (Kalckert and Ehrsson, 2014; Abdi et al., 2015). This perception enables intuitive interaction between the users and the wearable robotic limbs (Sasaki et al., 2017; Khazoom et al., 2020; Kieliba et al., 2021). These limbs are embodied in the users’ upper or lower limbs, i.e., hands and feet. The user’s perception of these wearable robotic limbs is attributed to specific body parts. Wearable robotic limbs can enhance interaction by synchronizing with multiple body parts using a weighted average method (Hagiwara et al., 2021; Sakurada et al., 2022). Furthermore, an additional limb synchronized with two user limbs enables individuals to achieve high task accuracy and smooth movement (Hagiwara et al., 2020; Fribourg et al., 2021). The motion synchronization method provides the user with new visuomotor feedback that differs from natural body manipulation, leading to the embodiment of wearable robotic limbs through this novel feedback. Previous studies on the motion synchronization of robotic limbs have revealed embodiment through the synchronization of movements of individual body parts (Sasaki et al., 2017; Saraiji et al., 2018; Kieliba et al., 2021). On the other hand, while the contribution of motion synchronization for wearable robotic limbs by multiple body parts of a user to the improvement of these manipulations has been shown (Sakurada et al., 2022), the embodiment of each body part has not been evaluated separately. In particular, understanding to which body part users attribute their perception of the wearable robotic limbs (perceptual attribution) under synchronization with multiple body parts is crucial for learning manipulation and designing sensory feedback. Previous studies have suggested using head motion (Iwasaki and Iwata, 2018; Oh et al., 2020; Sakurada et al., 2022) and other entities’ motion (Hagiwara et al., 2021) as reference body parts for the weighted average method. However, the perceptual attribution of the weighted average of the hand and foot has not been thoroughly investigated. Investigating the perceptual attribution of the hand and foot provides new insights regarding the embodiment of wearable robotic limbs. Therefore, by using the weighted average of the hand and foot, exploring the perceptual attribution of the wearable robotic limbs clarifies the body re-mapping process in relation to motion synchronization.
The rubber hand illusion is a typical example of embodiment (Botvinick and Cohen, 1998). Several studies have investigated the emergence of a sense of agency and body ownership based on it (Preston, 2013; Kalckert and Ehrsson, 2014; Krom et al., 2019). These studies have identified key explanatory factors for embodiment, including visuomotor and visuohaptic feedback (Kalckert and Ehrsson, 2014), visual appearance similarity (Krom et al., 2019), and distance from the fake hand and body (Preston, 2013). The coincidence of the user’s visual and motor information plays a significant role in triggering embodiment. In the context of virtual body embodiment, this coincidence refers to the spatial synchronization ratio relative to the user (Kokkinara et al., 2015; Fribourg et al., 2021). Kokkinara et al., 2015 suggested that mapping double or quadruple movements of the user onto a virtual body influences the sense of body ownership. Fribourg et al. proposed a method for mapping onto a virtual body using a weighted average of motion of the user and other entities (Fribourg et al., 2021). Their study suggested that lower weights on the user’s motion result in a mismatch between visual and motor information, consequently reducing the sense of agency.
Visual appearance similarity is another important factor in embodiment. This affects the sense of agency and body ownership toward a fake or virtual hand (Argelaguet et al., 2016; Lin and Jörg, 2016; Krom et al., 2019). demonstrated that a virtual body with a robotic appearance, while retaining the anatomical structure of a human body, elicited a proprioceptive drift (Krom et al., 2019). Lorraine et al. and Ferran et al. found that even with motion synchronization, users did not experience a sense of body ownership for virtual objects such as spheres and blocks (Argelaguet et al., 2016; Lin and Jörg, 2016).
Wearable robotic limbs designed for human augmentation incorporate body remapping to facilitate user interaction (Abdi et al., 2015; Sasaki et al., 2017; Saraiji et al., 2018; Kieliba et al., 2021; Umezawa et al., 2022). These studies have proposed novel interaction systems that extend the capabilities of the user’s body. Sasaki et al. developed MetaLimbs, wearable robotic limbs that remap to the foot for user interaction (Sasaki et al., 2017). Saraji et al. evaluated the embodiment through a search task using MetaLimbs (Saraiji et al., 2018). Abdi et al., 2015 evaluated an application that manipulated a third virtual hand utilizing the user’s foot as an input modality for a surgical robotic arm. They investigated task performance and subjective embodiment illusions when a virtual hand interacted with the user’s natural hands. Kieliba et al., 2021 developed a third thumb on the user’s hand that mapped to the foot thumb. They observed improved task performance and a sense of embodiment in the third thumb after 5 days of daily use and training. In addition, Umezawa et al. developed a robotic finger that operates independently of the user’s natural body parts (Umezawa et al., 2022). They demonstrated that humans could experience embodiment with such independent robotic limbs. Consequently, these wearable robotic limbs provide augmented embodiment by presenting new visuomotor information to the user. By manipulating these limbs, users learn and update their natural motor functions based on visuomotor feedback (Hagiwara et al., 2019; Kieliba et al., 2021). Kasuga et al., 2015 investigated the adaptation of the user’s motor model to mirror manipulation of an object with inverted coordinate positions relative to the body midline. They evaluated changes in participants’ error correction during a simple point-to-point task. The frequency of error correction indicates adjustments in motor function when people manipulate objects using their body parts.
The aforementioned studies focused on the perceptual attribution of proprioception and subjective perception during motion synchronization with a single body part. However, the perceptual attribution during motion synchronization with multiple body parts has not been adequately investigated. Additional limbs improve the user’s task performance during motion synchronization with multiple body parts (Iwasaki and Iwata, 2018; Hagiwara et al., 2020). In particular, the hand and foot are common targets for mapping wearable robotic limbs, each possessing its own distinct motor function (Pakkanen and Raisamo, 2004). Understanding the connection between motor function and perceptual mapping is crucial for designing effective manipulation systems for these wearable robotic limbs. Such an understanding contributes to the embodiment process, which takes into account the interaction between the user and the wearable robotic limbs. In addition, the impact of visual appearance similarity on perceptual attribution remains unclear. Therefore, it is necessary to further investigate perceptual attribution based on visual information while considering the body remapping of the hand and foot.
The purpose of this study was to examine the perceptual attribution of a wearable robotic limb when users manipulate it with both their hands and feet in a virtual environment. Specifically, we aimed to determine whether users perceived the virtual robotic limb as a hand or a foot during this manipulation. Furthermore, we investigated the effect of the visual similarity of the virtual robotic limb on perceptual attribution. By doing so, we intended to gain insight into the user’s embodiment process of the virtual robotic limb as a body part and to understand the role of visual information in this process.
To investigate the perceptual attribution to hand and foot, we utilized a virtual robotic limb (Figure 1A) that was synchronized with hand and foot movements simultaneously. Manipulation conditions included a single body part (hand or foot only) and multiple body parts (average motion of hand and foot) (Figure 1B). In addition, we altered the visual appearance of the virtual robotic limb to investigate the effect of visual similarity bias on natural body parts. The visual appearance conditions were human avatar, humanoid, and manipulator appearances (Figure 1C). We set these visual appearance similarities based on human anatomical construction and typical robotic limb end effector shape. We measured the proprioceptive drift, reaction time, trajectory, error correction frequency, and subjective embodiment illusions (sense of agency, sense of body ownership, and subjective perceptual attribution) per motor task (Figure 2). According to previous studies, the user’s proprioceptive drift during motion synchronization between a virtual hand and a single body part can be attributed to a specific body part. Therefore, the perceptual attribution for multiple body parts is represented by both proprioceptive drift and subjective perceptual attributions. Furthermore, the reaction time and trajectory in the point-to-point task encoded a temporal and spatial amount of motion for each body part. This motion information serves as visuomotor feedback that enhances embodiment. Additionally, the user’s error correction frequency indicated adaptation to the manipulation strategy when synchronizing the virtual robotic limb with the motion of the body parts. Based on these indices, we formulated research questions (RQs) to investigate perceptual attribution. The RQs are as follows.
• RQ1: Does the proprioceptive drift toward the virtual robotic limb, synchronized with multiple body parts, align with the subjective perceptual attribution?
• RQ2: Does the amount of motion of multiple body parts determine the attribution of proprioception and subjective perception?
• RQ3: Does the visual appearance similarity bias of the virtual robotic limb to a natural body part affect the perceptual attribution?
• RQ4: Does the manipulation of multiple body parts and the visual similarity bias affect the user’s error correction frequency?
FIGURE 1. Experimental conditions. Participants wore a head-mounted display and controller. Each tracker was fixed to the hand, foot, and pelvis (A). Manipulation conditions (B). Visual appearance conditions (C). Human avatar appearance conditions were baseline for all conditions (D). Created with Unity Editor®. Unity is a trademark or registered trademark of Unity Technologies.
FIGURE 2. Correspondence between each research question and measurement index. The sentences in each cell are the meanings of each index in the investigation of the RQs.
A total of 20 men and four women (mean age: 24.250 en2.625 (SD) years, maximum age: 32 years, minimum age: 21 years) (G*Power 3.1.9.7; effect size: .25, α error: .05, power: .690, within-subjects factors: 8) volunteered to participate in this study. They had normal vision and physical abilities and provided written informed consent before the experiment. The participants were engineering students and researchers who had regular exposure to VR systems and virtual robotic limbs. The study was conducted according to an experimental protocol approved by the Research Ethics Committee of the Faculty of Science and Technology, Keio University.
We used the Unity Engine to create the experimental visuomotor feedback, which was presented through a head-mounted display (HTC VIVE Pro, 1440 441600 pixels per eye, 110° diagonal, refresh rate of 90 Hz) (Figure 1A). The participants wore three motion trackers on their right hand, right foot, and pelvis (VIVE Tracker 2018; precision: less than 2 mm, accuracy: less than 7.5 mm, sampling rate: better than 60 Hz, delay: less than 44 msec). The foot tracker was securely fixed on top of the shoe to track foot motion, while the hand and pelvis trackers were attached to bands wrapped around the hand and pelvis, respectively. Participants also held a controller (VIVE Controller 2018; spatial resolution: within 1 mm; sampling rate: better than 60 Hz; delay: less than 44 msec) to proceed with the experiment and complete the task. The coordinates of the HMD, trackers, and controller, measured by the base station (SteamVR Base Station 2.0; range: 7 m, field of view: 150° × 110° diagonal) were sent to the Unity Engine via the Steam VR plug-in1. Two base stations were positioned diagonally to prevent body occlusion (distance: 2.8 m, height: 2.5 m) and ensure uninterrupted tracking. The design of the virtual robotic limb was based on Sasaki et al. (Sasaki et al., 2017). The end effector was solved using the Cyclic Coordinate Descent IK (CCD IK) function of the Unity Engine’s FinalIK plug-in2. Each of the five joints leading up to the end effector had three degrees of freedom. We modified the appearance of the end effector according to each visual condition.
We set the origin based on the center position of the human avatar and the height of the ground. In the single body part condition, the end effector of the virtual robotic limb followed the coordinates of the hand or foot (Figure 1B). The end effector had an offset from the hand and foot, positioned at mid-height based on a seated position (i.e., lower than the natural hand and higher than the natural foot). In the multiple body parts condition, the end effector followed the average coordinates of the hand and foot. In addition, we employed the appearance of the human avatar as a baseline in virtual space (Figure 1C). For the human avatar appearance, the participants completed each task with the human avatar. For the humanoid appearance, the end effector visually resembled the natural hand in terms of human anatomical construction. Therefore, in this case, the visual appearance was biased toward the natural hand. For the manipulator appearance, the end effector bore no visual resemblance to either the hand or the foot based on human anatomical structure. The experimental conditions encompassed a total of eight conditions, excluding the multiple body parts for the human avatar appearance, derived from the combination of manipulation and visual appearance conditions (Figure 1D).
In the point-to-point task, the home target was positioned +30 mm across and +30 mm forward from the mid-height between the hand and foot in the seated position. According to the work of Kasuga et al., 2015, the reaching target was set to be 12 mm in diameter and appeared +100 mm above, +100 mm across, and +100 mm forward of the home target. Upon contact between the tip of the pole at the center of the human avatar or virtual robotic limb and the home target, the home target disappeared and a reaching target appeared (Figure 3). When the participant touched the red pole to the reaching target, accompanied by a 1-s electronic tone, the reaching target disappeared and the home target reappeared. For the reaching task, we used reaching targets with a diameter of 15 mm. These reaching targets appeared at +100 1030 mm in height, +100 mm across, and +100 mm in front of the participant’s hand or foot in the seated position (Shibuya et al., 2017) (Figure 4). Each target was randomly highlighted in green during the task.
FIGURE 3. Point-to-point task. Participants touched each target in the virtual space with a red pole attached to the human avatar or virtual robotic limb. The position of the target corresponded to real space. Created with Unity Editor®. Unity is a trademark or registered trademark of Unity Technologies.
FIGURE 4. Reaching task to measure the proprioceptive drift. Each task for hand (A) and foot (B) was completed on targets appearing at different positions. The drift for each condition was the height difference (C) between the highlighted target position Pht and the final position Pe of the hand position Pi(i:0,1,… , e). Created with Unity Editor®. Unity is a trademark or registered trademark of Unity Technologies.
The participants completed the entire task while maintaining a seated position. At the beginning of the experiment, participants trained and completed the pre-reaching task (Figures 4A, B) with one trial for each reaching target (Figure 5). In the reaching task, participants reached a highlighted target location with their hand or foot. Throughout the task, participants did not have any visual information about their actual bodies in the virtual space. The final position (Figure 4C) of each reaching movement corresponded to the position of the hand tracker when the participants pressed the controller pad. After an electronic tone, another target was newly highlighted in random order. Participants returned their hand to the seated position before reaching for the new highlighted target. When the virtual robotic limb was synchronized with the hand and foot motions simultaneously, participants randomly completed the tasks with each hand and foot. After the pre-reaching task, we calibrated the human avatar’s head, pelvis, right hand, left hand, and right foot against the head-mounted display, waist tracker, right-hand tracker, controller, and right foot tracker, respectively. Subsequently, the root of the virtual robotic limb followed behind the pelvis of the human avatar. Participants underwent training with 10 trials of the point-to-point task and one trial of the post-reaching task for each target after the calibration process. The participants used the electronic tone and the disappearance of objects to identify the timing of task completion during this training phase. We re-calibrated the human avatar before the actual point-to-point and post-reaching tasks. Subsequently, participants completed 50 trials of the actual point-to-point task and one trial of the post-reaching task for each target. In the point-to-point task (Figure 3), participants repeated the trial between the home and reaching targets using the red pole. In the multiple body parts condition, participants moved their hand and foot simultaneously. Finally, participants answered each questionnaire (Table 1) presented in the virtual space. There was approximately a 1-min interval between each experimental condition, and the order of the conditions was randomized.
FIGURE 5. Experimental procedure. Participants completed the sequence from the pre-reaching task to the questionnaire for each condition.
We defined the reaction time in one trial of the point-to-point task as the time from reaching 10% of peak velocity to completion. The trajectory during the reaction time was the sum of the absolute values of the 3D vector. We then analyzed the average reaction time and the average trajectory of all trials for one condition. The calculation of the error correction followed the work of Kasuga et al., 2015 (Figure 6A). The visual position Pr of the tip of the virtual robotic limb was projected onto the plane PLh–t, which was perpendicular to the plane PLh–a–t connecting the human avatar’s head point Pa, home target point Ph, and reaching target point Pt, and connecting Ph and Pt (Figure 6B). The visual angle error was then given by the angle θe between the vectors formed by Ph, Pt, and Pr. Error-increasing segments were identified when the visual angular error at peak velocity doubled or more between trials. The frequency of unique error correction was defined as the occurrence of a segment (error correction segment) with a cumulative error of 5% or less, pooled across trials in all conditions. The height difference between the reaching target point Pht and the final reaching point Pe highlighted in the reaching task was the drift value for each measurement. The actual proprioceptive drift was calculated as the average difference in reaching targets between the two tasks (post-reaching task minus pre-reaching task). In addition, the direction of proprioceptive drift for the hand and foot was reversed according to the up-down position of the end effector (i.e., the direction for the proprioceptive drift of the hand was negative, while that of the foot was positive). We tested the normality of each indicator with the Shapiro-Wilk test, and we assessed significant differences using the Friedman test for multi-group comparisons (p < .05). For two-group comparisons and multi-group post-tests, we used the Wilcoxon signed-rank test with Bonferroni correction to adjust for p-values. Nonparametric uncorrelated tests (G*Power 3.1.9.7; effect size: .25, α error: .05, power: .338) for proprioceptive drift and questionnaire score were conducted using Spearman’s rank correlation coefficient (p < .05). The Huber loss function employed to calculate the linear regression model was as follows:
FIGURE 6. Definition of the user’s unique error correction. The error-increasing segment (A) with 2D motion based on a previous study (Kasuga et al., 2015) is the segment where the visual angle error
where r and η are the residual error and the threshold (η = 1.350) of the outliers, respectively. We calculated the coefficient and intercept that minimized the sum of the mean squares of this Huber loss function. This approach allowed us to consider all data points, even outliers, in the regression analysis. We did not exclude outliers from the analysis, nor did we exclude data from participants who fell into the outlier category in other analyses.
The proprioceptive drifts of the hand were significantly lower in the humanoid (Singleh: Z = −3.714, d = −.758, p = .002) and manipulator (Singlem: Z = −2.886, d = −.589, p = .041) appearance conditions compared to those of the human avatar appearance when manipulating the virtual robotic limb with the hand (Figure 7A). Thus, the proprioception of the hand drifted specifically toward the virtual robotic limb in both appearances. In addition, the proprioceptive drifts of the hand were significantly lower for the humanoid appearance than for the multiple body parts when manipulating the virtual robotic limb with the hand, respectively (Multih: Z = −3.629, d = −.741, p = .003, Multim: Z = −3.343, d = −.682, p = .009). Conversely, the proprioceptive drifts of the foot were significantly higher when manipulating the virtual robotic limb with the foot compared to those of the human avatar appearance (Singleh: Z = 4.257, d = .869, p < .001, Singlem: Z = 4.000, d = .817, p < .001, Multih: Z = 4.143, d = .846, p < .001, Multim: Z = 3.686, d = .725, p = .002) (Figure 7B). Thus, the proprioception of the foot drifted toward the virtual robotic limb in all conditions. Furthermore, there was no significant correlation between the proprioceptive drifts of the hand and the proprioceptive drifts of the foot in the humanoid appearance during hand and foot manipulation (p = .671, r = −.145, R2 = −.122) (Figure 8A). These proprioceptive drifts were significantly correlated with manipulator appearance (p < .001, r = .465, R2 = .147) (Figure 8B).
FIGURE 7. Proprioceptive drift. Boxplots show the proprioceptive drift of hand (A) and foot (B) for each manipulation condition and visual appearance condition (a: human avatar, h: humanoid, m: manipulator) (*: p < 05, **: p < 001). Created with Unity Editor®. Unity is a trademark or registered trademark of Unity Technologies.
FIGURE 8. Correlations of the proprioceptive drift in hand and foot motions. (A) and (B) display the correlation between the proprioceptive drift of the hand and the proprioceptive drift of the foot, highlighting the differences between the visual appearance conditions. These plots illustrate the drift distance as scatter points, the Huber regression line in black, and the confidence interval depicted as a gray area. The correlation coefficient (r) and the coefficient of determination (R2) are provided. The drift directions for the hand and foot are illustrated in (C). Created with Unity Editor®. Unity is a trademark or registered trademark of Unity Technologies.
The average reaction times in the human avatar appearance condition manipulated by a hand were significantly shorter compared to almost all other conditions
FIGURE 9. Reaction time and trajectories of the point-to-point task. (A) shows the average reaction times for all conditions (a: avatar, h: humanoid, m: manipulator). The average trajectories of the virtual robotic limb for hand and foot motions (B) are compared with those of the human avatar condition. The boxplots for single body motions (C), (D) and multiple body part motions (E), and (F) show the differences in hand and foot scores for each manipulation and end effector appearance condition (*: p < 05, **: p < 001).
In both the human avatar and virtual robotic limb conditions, the visual angular errors reflected the error correction of participants in each condition. When the errors persisted for 5 frames, the pooled error-increasing segments across all conditions were found to be less than 5% (Figure 10A). The number of unique error correction segments was not significantly different across conditions (Figure 10B).
FIGURE 10. Unique error correction for each condition. Bar plots (A) show the frequency of each number of error accumulations in the error-increasing segment. Boxplots (B) show the number of error correction segments for the manipulation and visual appearance conditions (a: avatar, h: humanoid, m: manipulator).
We have summarized the statistical values of the questionnaire analysis in the Supplementary Table S1. Q1, Q2, Q3, Q7, and Q8 showed significant differences for both virtual robotic limb appearances (Figures 11A, B, and Supplementary Table S1). Q1 scores were significantly higher in the hand condition compared to the foot and multiple body parts conditions. Q2 and Q7 scores were significantly higher in the hand condition compared to the foot and multiple body parts conditions. In addition, these scores were significantly lower in the foot condition than in the multiple body parts condition. Q3 scores were significantly higher in the hand condition than in the conditions of the foot and multiple body parts. Furthermore, these scores were significantly higher in the foot condition than in the multiple body parts condition. Q8 scores were significantly lower in the hand condition than in the foot and multiple body parts conditions in the manipulator appearance condition, and Q6 scores were significantly higher in the hand condition than in the foot condition. Furthermore, Figures 11C, D show differences in the subjective sense of agency and body ownership between the human avatar appearance and the multiple body parts condition. Q2 and Q7 scores for the hand in the human avatar appearance were significantly higher compared to the virtual robotic limb in the multiple body parts condition. These scores for the foot in the human avatar appearance were significantly lower compared to the virtual robotic limb in the multiple body parts condition. Q3 and Q8 scores for the hand of the human avatar appearance were significantly lower than in the virtual robotic limb of the multiple body parts condition. These scores for the foot in the human avatar were significantly higher than those of the virtual robotic limb in the multiple body parts condition. Q4 scores for the human avatar hand appearance were significantly lower than those in the multiple body parts condition of the manipulator appearance. Q6 scores for the human avatar hand appearance were significantly higher than those for the multiple body parts condition. Q9 scores for the human avatar’s hand appearance were significantly lower than those for the multiple body parts condition.
FIGURE 11. Questionnaire scores. Boxplots for humanoid (A) and manipulator (B) appearances show comparisons between manipulation conditions. Questionnaire scores in the multiple body parts condition are shown in the boxplots in comparison with the human avatar (a: human avatar) score of hand (C) and foot (D) (*: p <.05,**: p <.001) for both appearances (h: humanoid, m: manipulator).
Q2 and Q3 scores in the multiple body parts condition were significantly correlated with those in the manipulator appearance condition (p = .035, r = .433, R2 = .277) (Figure 12B). Q7 and Q8 scores in the multiple body parts condition were significantly correlated with those in the humanoid condition (p = .017, r = .482, R2 = .218) (Figure 12C) and the manipulator appearance condition (p < .001, r = .838, R2 = .691) (Figure 12D). There was no significant correlation between the questionnaire scores for each body part (hand: Q2, Q7, foot: Q3, Q8) and each proprioceptive drift in the multiple body parts condition (Table 2).
FIGURE 12. Correlation between the sense of agency and body ownership in multiple body parts condition. The correlation plots of humanoid appearance (A, C) and manipulator appearance (B, D) for each body part show the questionnaire scores (scatters), Huber-regression (black line), confidence interval (gray area), and number of participants (color bar), respectively (r: correlation coefficient, R2 : coefficient of determination).
Each measurement index in this study showed a pattern of perceptual attribution in each condition (Figure 13). However, the results of proprioceptive drift and subjective perceptual attribution did not support the congruence with perceptual attribution in the first research question (RQ1: Does the proprioceptive drift toward the virtual robotic limb, synchronized with multiple body parts, align with the subjective perceptual attribution?). In the multiple body parts condition, a significant proprioceptive drift was observed in the participants’ foot compared to the human avatar appearance (Figure 7). This suggests that the participants perceived the virtual robotic limb as their foot during the occurrence of proprioceptive drift. In previous studies, the proprioceptive drift occurred toward the body part that was subjectively attributed (Kalckert and Ehrsson, 2017; Krom et al., 2019). The questionnaire revealed a contrasting pattern of perceptual attribution compared to proprioceptive drift. The questionnaire scores for both body parts were significantly higher in the multiple body parts condition than in the human avatar appearance (Figure 11C, D). This suggests that a strong sense of agency and body ownership was experienced for both body parts in the multiple body parts condition compared to the baseline. In addition, there was no observed correlation between proprioceptive drift and questionnaire scores for each body part in this study (Table 2). Previous studies have reported a discrepancy between the proprioceptive drift and subjective embodiment illusion when a false hand had a spatial-temporal distortion relative to the natural hand (Holle et al., 2011; Abdulkarim and Ehrsson, 2016). Furthermore, a meta-analysis of the correlation between subjective embodiment illusion and proprioceptive drift in the rubber hand illusion showed that each index captures different aspects of the rubber hand illusion (Tosi et al., 2023). These studies suggested that proprioceptive drift and subjective embodiment involve independent, parallel processes. Our results showed that the subjective embodiment illusion of each body part and the proprioceptive drift were incongruent even under conditions in which the wearable robotic limb was synchronized with multiple body parts. Therefore, these results indicate that the processing of perceptual attribution during proprioceptive drift and subjective perceptual attribution differ when operating multiple body parts. This finding contributes to our understanding of the embodiment process of wearable robotic limbs in the context of human augmentation. Moreover, we expect this finding to contribute to the embodiment of novel wearable robotic limbs using motion synchronization for multiple body parts.
The multiple body parts condition consistently showed equal or better reaction times than the other conditions in each appearance condition (Figure 9A). In addition, the foot trajectories were consistently longer than those of the hand (Figures 9B–F). These results highlight the distinct amounts of motion between the hand and the foot, with the foot exhibiting a longer trajectory. The lower spatial task accuracy of the foot compared to the hand (Pakkanen and Raisamo, 2004) suggests an extra foot path in the point-to-point task. Thus, there is a bias in the visuomotor feedback from the amounts of motion of the hand and foot in the multiple body parts condition. This bias likely contributes to the independent effects observed in the processes of proprioceptive drift and subjective perceptual attribution. In this study, the proprioception of the foot, which had a longer trajectory than the hand, drifted to the virtual robotic limb. This result suggests that the motion amounts of each body part influence the proprioceptive drift, supporting the findings of RQ2 (Do the motion amounts of multiple body parts determine the attribution of proprioception and subjective perception?). The subjective perceptual attribution was not determined by the amount of motion in each body part. Previous studies have discussed the effects of visuomotor feedback on proprioception and subjective embodiment illusions in the context of a single body part (Maravita et al., 2003; Kokkinara et al., 2015; Bourdin et al., 2019). In the present work, we investigated the relationship between visuomotor feedback and perceptual attribution for a virtual robotic limb mapped to multiple body parts. These results highlight the gap between users’ subjective perception and proprioception in body augmentation using wearable robotic limbs under motion synchronization with multiple body parts.
The proprioceptive drift in the multiple body parts condition showed a positive correlation between both body parts in manipulator appearance (Figure 8). In this study, the vertical position of the hand and foot was reversed based on the end effector (Figure 8C). This positive correlation indicates an increased proprioceptive drift toward a specific body part. Furthermore, the subjective sense of agency and body ownership for each body part also exhibited significant positive correlations between both body parts in the manipulator appearance condition (Figures 12B, D). The questionnaire indicated an equivalent sense of agency and body ownership for both body parts in the manipulator appearance condition. This correlation also revealed an inter-participant bias in the questionnaire scores. In other words, these correlation results showed a between-participant bias in perceptual attributions. Thus, these findings support the influence of visual similarity on perceptual attribution, as addressed in RQ3 (Does the visual appearance similarity bias of the virtual robotic limb to a natural body part affect the perceptual attribution?). The effect of visual similarity on perceptual attribution can be attributed to multisensory integration in embodiment. Previous studies have shown that proprioceptive drift and subjective embodiment illusion are represented by multisensory integration (Argelaguet et al., 2016; Lin and Jörg, 2016; Shibuya et al., 2017; Krom et al., 2019). Furthermore, humans independently process multisensory information during the occurrence of proprioceptive drift and subjective embodiment illusions (Holle et al., 2011; Abdulkarim and Ehrsson, 2016). In our manipulator appearance, there were no fingers that exhibited any human anatomical structural similarity between the hand and foot. This anatomical body structure is related to the effects of visual similarity on the embodiment process (Argelaguet et al., 2016; Lin and Jörg, 2016). Therefore, some participants may have perceived the virtual robotic limb as simply a body part rather than specifically as a hand or foot. These results suggest the contribution of visual similarity to the integration of a wearable robotic limb, synchronized with multiple body parts, into the user’s body.
We calculated the error correction segment based on the participants’ viewpoints (Figure 6) to investigate RQ4 (Does the manipulation of multiple body parts and the visual similarity bias affect the user’s error correction frequency?). These segments were defined as instances where five or more consecutive visual angular errors occurred (Figure 10A). However, we did not find any significant differences in the error correction segments across all conditions (Figure 10B). This result suggests that the time required for motor model adaptation differs from the occurrence of proprioceptive drift. In a previous study (Kasuga et al., 2015), the learning task for new visuomotor feedback was performed over several days with 100 trials each. In contrast, the embodiment illusion for a fake hand occurred through visuomotor stimuli during 23 s (Kalckert and Ehrsson, 2017). This result weakly supports the notion that proprioceptive drift occurs before the motor model, which is responsible for the participants’ error corrections, is adopted. Thus, our study suggests that the occurrence of embodiment and changes in the frequency of error correction for the virtual robotic limb involve distinct sequences and factors. Finally, the future direction of our study is to investigate the relationship between embodiment and motor function in more detail. This future research will help clarify how users integrate wearable robotic limbs into their bodies.
The participants in our study had the flexibility to adjust the amount of motion of their hand and foot to manipulate the virtual robotic limb according to their preferences. This allowed for individual differences in the trajectories of each body part during active movement. However, a systematic analysis by restricting the amount of motion of each body part could be conducted in future studies to further explore this aspect.
It is important to note that in the human avatar and humanoid conditions, the visual appearance of the virtual robotic limb was based on the understanding of the natural body structure from previous studies (Argelaguet et al., 2016; Lin and Jörg, 2016; Krom et al., 2019). However, we did not customize the parameters for each participant, which may introduce individual differences in the experimental results. The human avatar synchronized with the participant’s hand and foot served as the baseline for perceptual attribution in our study. Therefore, our results are based on comparisons with the avatar synchronization condition rather than comparisons with a general motor asynchrony condition.
We defined the visual angular error with respect to a movable plane based on the participants’ viewpoints, which projected the pole at the tip of the virtual robotic limb rather than a fixed plane as in Kasuga et al., 2015. This approach aimed to minimize motion biases in the visual angular error, as excessive participant motion during the task was not observed.
Our discussion did not extensively explore the detailed mapping areas between the participants’ bodies and the virtual robotic limb. The objective indices in our study focused on the motions of the back of the hand and the top of the foot. Additionally, the subjective evaluations did not specifically address the detailed mapping area or the sense of additional limbs.
In a previous study, researchers investigated how hand and foot representations changed in the brain by measuring brain activity using functional magnetic resonance imaging (fMRI) (Kieliba et al., 2021). Exploring updates in brain activity with virtual robotic limbs manipulated by multiple body parts is an intriguing area for future research.
In this study, we aimed to investigate the perceptual attribution of a virtual robotic limb manipulated by multiple body parts (hand and foot) and the impact of visual similarity on this attribution. To address our research questions (RQ1, RQ2, RQ3, RQ4), we measured proprioceptive drift, reaction time, trajectory, error correction frequency, and subjective embodiment illusion (sense of agency, sense of body ownership, and subjective perceptual attribution) using a point-to-point task. The task was performed with the virtual robotic limb, and we manipulated its visual appearance to examine the effect of visual similarity.
Regarding RQ1, we did not find congruence between proprioceptive drift and subjective perceptual attribution. While we observed proprioceptive drift of the participants’ foot toward the virtual robotic limb during manipulation with multiple body parts, subjective perceptual attribution occurred for both the hand and the foot. Therefore, the proprioceptive drift toward the virtual robotic limb during motion synchronization with multiple body parts was not congruent with subjective perceptual attribution. For RQ2, we found support for the effect of the amount of motion of each body part on proprioceptive drift. However, subjective perceptual attribution did not show a significant effect on the amount of motion in each body part. Regarding RQ3, manipulator appearance increased the correlations between proprioceptive drift and subjective embodiment illusions for the hand and foot, respectively. These correlations indicated a between-participant bias in perceptual attribution. Our manipulator appearance lacked visual similarity based on human anatomical structure, suggesting that some participants perceived the virtual robotic limb as simply a body part rather than a hand or foot. Thus, these results supported the effect of visual appearance similarity on perceptual attribution. For RQ4, we did not observe a change in error correction frequency across all experimental conditions. This result suggests that the time required for perceptual attribution may be different from that required for motor function adaptation.
In conclusion, our study revealed a lack of congruence between proprioceptive drift and subjective perceptual attribution in manipulation using multiple body parts. This finding suggests independent parallel processes of proprioceptive drift attribution and subjective perceptual attribution. We anticipate that our findings will contribute to the design and development of operation methods for wearable robotic limbs with multiple body parts and enhance our understanding of how we perceive them.
The raw data supporting the conclusion of this article will be made available by the authors, without undue reservation.
The studies involving humans were approved by The research ethics committee at the Faculty of Science and Technology, Keio University. The studies were conducted in accordance with the local legislation and institutional requirements. The participants provided their written informed consent to participate in this study.
KS, MK, and MS conceived and designed the experiments. KS collected and analyzed the data. KS, RK, FN, MK, and MS contributed to the preparation of the manuscript. All images, drawings, and photographs were obtained or created by KS. All authors contributed to the article and approved the submitted version.
This study was supported by JST ERATO, Grant Number JPMJER1701, by JST SPRING, Grant Number JPMJSP2123, and by JSPS KAKENHI, Grant Number JP22KK0158.
The authors declare that the study was conducted in the absence of any commercial or financial relationships that could be construed as a potential conflict of interest.
All claims expressed in this article are solely those of the authors and do not necessarily represent those of their affiliated organizations, or those of the publisher, the editors and the reviewers. Any product that may be evaluated in this article, or claim that may be made by its manufacturer, is not guaranteed or endorsed by the publisher.
The Supplementary Material for this article can be found online at: https://www.frontiersin.org/articles/10.3389/frvir.2023.1210303/full#supplementary-material
1https://store.steampowered.com/app/250820/SteamVR
2http://www.root-motion.com/final-ik.html
Abdi, E., Burdet, E., Bouri, M., and Bleuler, H. (2015). Control of a supernumerary robotic hand by foot: an experimental study in virtual reality. PloS one 10, e0134501. doi:10.1371/journal.pone.0134501
Abdulkarim, Z., and Ehrsson, H. H. (2016). No causal link between changes in hand position sense and feeling of limb ownership in the rubber hand illusion. Atten. Percept. Psychophys. 78, 707–720. doi:10.3758/s13414-015-1016-0
Argelaguet, F., Hoyet, L., Trico, M., and Lecuyer, A. (2016). “The role of interaction in virtual embodiment: effects of the virtual hand representation,” in 2016 IEEE virtual reality (VR), 3–10. doi:10.1109/VR.2016.7504682
Botvinick, M., and Cohen, J. (1998). Rubber hands ‘feel’touch that eyes see. Nature 391, 756. doi:10.1038/35784
Bourdin, P., Martini, M., and Sanchez-Vives, M. V. (2019). Altered visual feedback from an embodied avatar unconsciously influences movement amplitude and muscle activity. Sci. Rep. 9, 19747. doi:10.1038/s41598-019-56034-5
Dingwell, J. B., Mah, C. D., and Mussa-Ivaldi, F. A. (2002). Manipulating objects with internal degrees of freedom: evidence for model-based control. J. Neurophysiology 88, 222–235. doi:10.1152/jn.2002.88.1.222
Farrer, C., Bouchereau, M., Jeannerod, M., and Franck, N. (2008). Effect of distorted visual feedback on the sense of agency. Behav. Neurol. 19, 53–57. doi:10.1155/2008/425267
Fribourg, R., Ogawa, N., Hoyet, L., Argelaguet, F., Narumi, T., Hirose, M., et al. (2021). Virtual co-embodiment: evaluation of the sense of agency while sharing the control of a virtual body among two individuals. IEEE Trans. Vis. Comput. Graph. 27, 4023–4038. doi:10.1109/TVCG.2020.2999197
Gallagher, S. (2000). Philosophical conceptions of the self: implications for cognitive science. Trends Cognitive Sci. 4, 14–21. doi:10.1016/S1364-6613(99)01417-5
Hagiwara, T., Ganesh, G., Sugimoto, M., Inami, M., and Kitazaki, M. (2020). Individuals prioritize the reach straightness and hand jerk of a shared avatar over their own. iScience 23, 101732. doi:10.1016/j.isci.2020.101732
Hagiwara, T., Katagiri, T., Yukawa, H., Ogura, I., Tanada, R., Nishimura, T., et al. (2021). “Collaborative avatar platform for collective human expertise,” in SIGGRAPH asia 2021 emerging technologies (New York, NY, USA: Association for Computing Machinery). SA ’21 Emerging Technologies. doi:10.1145/3476122.3484841
Hagiwara, T., Sugimoto, M., Inami, M., and Kitazaki, M. (2019). “Shared body by action integration of two persons: body ownership, sense of agency and task performance,” in 2019 IEEE conference on virtual reality and 3D user interfaces (VR), 954–955. doi:10.1109/VR.2019.8798222
Holle, H., McLatchie, N., Maurer, S., and Ward, J. (2011). Proprioceptive drift without illusions of ownership for rotated hands in the “rubber hand illusion” paradigm. Cogn. Neurosci. 2, 171–178. doi:10.1080/17588928.2011.603828
Iwasaki, Y., and Iwata, H. (2018). “A face vector - the point instruction-type interface for manipulation of an extended body in dual-task situations,” in 2018 IEEE international conference on cyborg and bionic systems (CBS), 662–666. doi:10.1109/CBS.2018.8612275
Kalckert, A., and Ehrsson, H. H. (2014). The moving rubber hand illusion revisited: comparing movements and visuotactile stimulation to induce illusory ownership. Conscious. Cognition 26, 117–132. doi:10.1016/j.concog.2014.02.003
Kalckert, A., and Ehrsson, H. H. (2017). The onset time of the ownership sensation in the moving rubber hand illusion. Front. Psychol. 8, 344. doi:10.3389/fpsyg.2017.00344
Kasuga, S., Kurata, M., Liu, M., and Ushiba, J. (2015). Alteration of a motor learning rule under mirror-reversal transformation does not depend on the amplitude of visual error. Neurosci. Res. 94, 62–69. doi:10.1016/j.neures.2014.12.010
Khazoom, C., Caillouette, P., Girard, A., and Plante, J.-S. (2020). A supernumerary robotic leg powered by magnetorheological actuators to assist human locomotion. IEEE Robotics Automation Lett. 5, 5143–5150. doi:10.1109/LRA.2020.3005629
Kieliba, P., Clode, D., Maimon-Mor, R. O., and Makin, T. R. (2021). Robotic hand augmentation drives changes in neural body representation. Sci. Robotics 6, eabd7935. doi:10.1126/scirobotics.abd7935
Kojima, A., Yamazoe, H., Chung, M. G., and Lee, J.-H. (2017). “Control of wearable robot arm with hybrid actuation system,” in 2017 IEEE/SICE international symposium on system integration (SII), 1022–1027. doi:10.1109/SII.2017.8279357
Kokkinara, E., Slater, M., and López-Moliner, J. (2015). The effects of visuomotor calibration to the perceived space and body, through embodiment in immersive virtual reality. ACM Trans. Appl. Percept. 13, 1–22. doi:10.1145/2818998
Krom, B. N., Catoire, M., Toet, A., van Dijk, R. J. E., and van Erp, J. B. (2019). Effects of likeness and synchronicity on the ownership illusion over a moving virtual robotic arm and hand. In 2019 IEEE World Haptics Conf. (WHC). 49–54. doi:10.1109/WHC.2019.8816112
Lin, L., and Jörg, S. (2016). “Need a hand? How appearance affects the virtual hand illusion,” in Proceedings of the ACM symposium on applied perception (New York, NY, USA: Association for Computing Machinery), 69–76. SAP ’16. doi:10.1145/2931002.2931006
Llorens Bonilla, B., Parietti, F., and Asada, H. H. (2012). “Demonstration-based control of supernumerary robotic limbs,” in 2012 IEEE/RSJ international conference on intelligent robots and systems, 3936–3942. doi:10.1109/IROS.2012.6386055
Marasco, P. D., Hebert, J. S., Sensinger, J. W., Shell, C. E., Schofield, J. S., Thumser, Z. C., et al. (2018). Illusory movement perception improves motor control for prosthetic hands. Sci. Transl. Med. 10, eaao6990. doi:10.1126/scitranslmed.aao6990
Maravita, A., Spence, C., and Driver, J. (2003). Multisensory integration and the body schema: close to hand and within reach. Curr. Biol. 13, R531–R539. doi:10.1016/S0960-9822(03)00449-4
Mazzoni, P., and Krakauer, J. W. (2006). An implicit plan overrides an explicit strategy during visuomotor adaptation. J. Neurosci. 26, 3642–3645. doi:10.1523/JNEUROSCI.5317-05.2006
Oh, J., Ando, K., Iizuka, S., Guinot, L., Kato, F., and Iwata, H. (2020). “3d head pointer: a manipulation method that enables the spatial localization for a wearable robot arm by head bobbing,” in 2020 23rd international symposium on measurement and control in robotics (ISMCR), 1–6. doi:10.1109/ISMCR51255.2020.9263775
Pakkanen, T., and Raisamo, R. (2004). “Appropriateness of foot interaction for non-accurate spatial tasks,” in CHI ’04 extended abstracts on human factors in computing systems (New York, NY, USA: Association for Computing Machinery), 1123–1126. CHI EA ’04. doi:10.1145/985921.986004
Parietti, F., and Asada, H. H. (2014). “Supernumerary robotic limbs for aircraft fuselage assembly: body stabilization and guidance by bracing,” in 2014 IEEE international conference on robotics and automation (ICRA), 1176–1183. doi:10.1109/ICRA.2014.6907002
Prattichizzo, D., Pozzi, M., Baldi, T. L., Malvezzi, M., Hussain, I., Rossi, S., et al. (2021). Human augmentation by wearable supernumerary robotic limbs: review and perspectives. Prog. Biomed. Eng. 3, 042005. doi:10.1088/2516-1091/ac2294
Preston, C. (2013). The role of distance from the body and distance from the real hand in ownership and disownership during the rubber hand illusion. Acta Psychol. 142, 177–183. doi:10.1016/j.actpsy.2012.12.005
Sakurada, K., Kondo, R., Nakamura, F., Fukuoka, M., Kitazaki, M., and Sugimoto, M. (2022). “The reference frame of robotic limbs contributes to the sense of embodiment and motor control process,” in Augmented humans 2022 (New York, NY, USA: Association for Computing Machinery), 104–115. AHs 2022. doi:10.1145/3519391.3522754
Sanchez-Vives, M. V., Spanlang, B., Frisoli, A., Bergamasco, M., and Slater, M. (2010). Virtual hand illusion induced by visuomotor correlations. PloS one 5, e10381. doi:10.1371/journal.pone.0010381
Saraiji, M. Y., Sasaki, T., Kunze, K., Minamizawa, K., and Inami, M. (2018). “Metaarms: body remapping using feet-controlled artificial arms,” in Proceedings of the 31st annual ACM symposium on user interface software and technology (New York, NY, USA: Association for Computing Machinery), 65–74. UIST ’18. doi:10.1145/3242587.3242665
Sasaki, T., Saraiji, M. Y., Fernando, C. L., Minamizawa, K., and Inami, M. (2017). “Metalimbs: multiple arms interaction metamorphism,” in ACM SIGGRAPH 2017 emerging technologies (New York, NY, USA: Association for Computing Machinery). SIGGRAPH ’17. doi:10.1145/3084822.3084837
Schiefer, M., Tan, D., Sidek, S. M., and Tyler, D. J. (2015). Sensory feedback by peripheral nerve stimulation improves task performance in individuals with upper limb loss using a myoelectric prosthesis. J. Neural Eng. 13, 016001. doi:10.1088/1741-2560/13/1/016001
Shibuya, S., Unenaka, S., and Ohki, Y. (2017). Body ownership and agency: task-dependent effects of the virtual hand illusion on proprioceptive drift. Exp brain Res 235, 121–134. doi:10.1007/s00221-016-4777-3
Takizawa, R., Verhulst, A., Seaborn, K., Fukuoka, M., Hiyama, A., Kitazaki, M., et al. (2019). “Exploring perspective dependency in a shared body with virtual supernumerary robotic arms,” in 2019 IEEE international conference on artificial intelligence and virtual reality (AIVR), 25–257. doi:10.1109/AIVR46125.2019.00014
Tosi, G., Mentesana, B., and Romano, D. (2023). The correlation between proprioceptive drift and subjective embodiment during the rubber hand illusion: a meta-analytic approach. Q. J. Exp. Psychol. 0, 17470218231156849. doi:10.1177/17470218231156849
Keywords: virtual reality, embodiment, perceptual attribution, wearable robotic limbs, proprioceptive drift, sense of agency, sense of body ownership, error correction frequency
Citation: Sakurada K, Kondo R, Nakamura F, Kitazaki M and Sugimoto M (2023) Investigating the perceptual attribution of a virtual robotic limb synchronizing with hand and foot simultaneously. Front. Virtual Real. 4:1210303. doi: 10.3389/frvir.2023.1210303
Received: 22 April 2023; Accepted: 31 August 2023;
Published: 07 November 2023.
Edited by:
Shogo Okamoto, Tokyo Metropolitan University, JapanReviewed by:
Yuki Ban, The University of Tokyo, JapanCopyright © 2023 Sakurada, Kondo, Nakamura, Kitazaki and Sugimoto. This is an open-access article distributed under the terms of the Creative Commons Attribution License (CC BY). The use, distribution or reproduction in other forums is permitted, provided the original author(s) and the copyright owner(s) are credited and that the original publication in this journal is cited, in accordance with accepted academic practice. No use, distribution or reproduction is permitted which does not comply with these terms.
*Correspondence: Kuniharu Sakurada, a2guc2FrdXJhZGFAaW1sYWIuaWNzLmtlaW8uYWMuanA=
Disclaimer: All claims expressed in this article are solely those of the authors and do not necessarily represent those of their affiliated organizations, or those of the publisher, the editors and the reviewers. Any product that may be evaluated in this article or claim that may be made by its manufacturer is not guaranteed or endorsed by the publisher.
Research integrity at Frontiers
Learn more about the work of our research integrity team to safeguard the quality of each article we publish.