- 1Research Institute for the Environment and Livelihoods, Charles Darwin University, Darwin, NT, Australia
- 2Fenner School of Environment and Society, Australian National University, Canberra, ACT, Australia
- 3Faculty of Agriculture, Universitas Nusa Cendana, Kupang, Indonesia
Woody vegetation restoration projects are an important feature of landscape function in Indonesian karst savannas. Understanding the relationship between available moisture and vegetation condition can assist with the planning and implementation of revegetation efforts. Working at vegetation restoration sites in East Nusa Tenggara, Indonesia, we applied a windowed cross-correlation method to mean values of NDVI to examine the lag between moisture input and NDVI response for both rainfall and soil moisture between 1999 and 2018. To test for increasing or decreasing trends in NDVI and rainfall time series, we undertook Mann–Kendall trend analyses. We identified increasing trends in Landsat 7 NDVI at two of four restoration sites, with annual increases in NDVI of 2.7 and 3.74 × 10−4 respectively. We found that rainfall dependent sites had significant Pearson’s correlations with NDVI ranging from 0.52 to 0.71, while NDVI was not correlated with rainfall at shallow groundwater sites. There was a clear negative effect of the very dry period on all sites, and this was less pronounced at shallow groundwater sites. Wet years resulted in a positive response to NDVI across all sites, while the response was lower in very wet years with annual rainfall above 1,200 mm. We found that between 2 and 4 months of antecedent rainfall gave the highest correlation with NDVI, while for soil moisture the closest relationship was found with no lag and 1 month lag. Through this study, we demonstrated the applicability of using NDVI, rainfall, and soil moisture trend analyses to identify groundwater-dependent vegetation patches and monitor the effectiveness of vegetation restoration.
1 Introduction
Karst aquifers in savanna environments provide essential water supplies to support human populations in Asia, Africa, Australia, and Central America (Klaas, 2008; Schwinning, 2008; Ward et al., 2013; Rossatto et al., 2014; Goldscheider et al., 2020). These environments are potentially under threat from a combination of water stress and landcover change (Sankaran, 2019). In these highly seasonal landscapes, the spatial and temporal patterns of tree water uptake remain poorly understood, yet enhanced knowledge of their dynamics is important to sustainably manage the groundwater systems (Asbjornsen et al., 2011; Banks et al., 2011; Acharya et al., 2018). In karst savannas, vegetation water use is often dependent on the moisture available in the epikarst (i.e., the zone of weathered bedrock beneath the soil layer) (Schwinning, 2010; Nie et al., 2012; Jones, 2013; Rossatto et al., 2014). Relative to a non-karst environment where infiltration to the tree root zone occurs gradually after soil saturation, groundwater recharge in karst ecosystems occurs rapidly following intense rainfall events due to the presence of large fissures or conduits (Wilcox et al., 2006; Schwinning, 2008; Sarrazin et al., 2018). The epikarst is also a key subsurface zone for groundwater recharge (Schwinning, 2008).
The presence, type, and density of vegetation in recharge zones may affect fluxes of soil moisture, epikarst storage, and, ultimately, groundwater availability (Cardella Dammeyer et al., 2016). In tropical savannas, the dominant savanna tree and shrub species have evolved to transpire perennially (Eaasmi et al., 2009), potentially influencing deep soil water and groundwater stores, particularly during the dry season when shallow moisture sources are depleted. By exploring the dynamics between vegetation productivity and moisture availability, we can better predict the resilience of tropical karst savanna ecosystems, including moisture stores, to changes in future environmental conditions. Relevant changes include the likely prolonged dry seasons, changes in the frequency and intensity of recharge events, continued influence of the El Niño Southern Oscillation (ENSO) (Brown et al., 2013), and changes in groundwater abstraction rates. Here, we investigate these dynamics using satellite image-derived normalised difference vegetation index (NDVI) surfaces and moisture trend analyses to provide preliminary insights into the response of tropical karst savanna vegetation to available moisture.
NDVI is a proxy for productivity (Aguilar et al., 2012), as it indicates photosynthetic activity by measuring chlorophyll reflectance (Glenn et al., 2010). The seasonal and interannual response of vegetation conditions to moisture availability is commonly studied using NDVI trend analyses. By determining the relationship between vegetation productivity and moisture availability, we can predict how vegetation conditions may be affected by changing moisture regimes under future climate conditions (Chamaillé-Jammes and Fritz, 2009). Previous studies have established that NDVI responds to antecedent rainfall with a range of lag times in the order of 1 week (Shinoda, 1995; Kong et al., 2020) and 1 month to 2 months (e.g., Chamaille-Jammes et al., 2006; Fu and Burgher, 2015; Souza et al., 2016). The variations in lag times are attributed to site-specific factors such as topography, soil types (Chamaille-Jammes et al., 2006), infiltration rates, and density of vegetation (Kong et al., 2020). Previous trend analyses have shown that rainfall anomalies (i.e., departures from long-term means) may have a more significant influence on NDVI than seasonal variability (Chen et al., 2020) and that the degree of influence varies with vegetation structure (Anchang et al., 2019; Brandt et al., 2019).
The degree to which vegetation productivity is influenced by moisture relative to other environmental drivers varies spatially and temporally (Lehmann et al., 2014; Beringer et al., 2015), and vegetation communities have degrees of dependence on groundwater, subject to phenological and location characteristics (e.g., Cook and O'Grady, 2006; Lamontagne et al., 2005). Tropical savanna vegetation communities are dominated by C4 grasses, interspersed with predominantly C3 evergreen trees (Monk, 1997; Myers et al., 1997; Murphy and Bowman, 2012; Rossatto et al., 2014). During the dry season, trees and shrubs use deep soil moisture or groundwater to varying degrees as a function of plant root density and the water use strategies of individual species (Cook and O'Grady, 2006; Lamontagne et al., 2005; Monk, 1997; Murphy and Bowman, 2012; Rossatto et al., 2014; Schwinning, 2008; Ward et al., 2013). The degree of vegetation groundwater dependence is indicated by how closely NDVI is coupled with rainfall (i.e., where NDVI is poorly correlated to rainfall, vegetation is considered to be groundwater dependent) (Páscoa et al., 2020).
Soil moisture provides a better representation of plant-available water than rainfall, and this parameter co-varies with NDVI at a range of lag times (Ahmed et al., 2017; Tian et al., 2019). Microwave remote sensing provides the capability for direct observation of surface soil moisture condition by analysing the microwave radiation emitted or reflected by the soil, and several microwave satellite missions have been deployed to provide continuous global scale soil moisture estimation (Ford et al., 2014; Tian et al., 2021). Root-zone soil moisture, which is an important indicator of plant water availability, is widely obtained through the use of model simulations. In addition, modelled estimates of root-zone soil moisture from satellite retrievals have proved to have generally good agreement with ground data (Ford et al., 2014; Tian et al., 2019) and are, therefore, a valid indicator of plant-available water.
There is a demand for knowledge of plant-available water and the associated vegetation productivity response in karst savanna landscapes of Indonesia. This demand stems from concerns of land managers about the links between soil and water resources, reduced tree cover, and biodiversity and livelihoods (Pellokila et al., 2014). Government and non-government programs have sought to improve landscape function in priority areas through regreening and reforestation. This work has focussed on sites that are particularly prone to erosion (Monk, 1997; Scott et al., 2005; Wallace et al., 2005; BPDAS, 2011; Mulyoutami et al., 2016); it has been done without data about plant water use. Climate predictions indicate that the southernmost islands of Indonesia (e.g., East Nusa Tenggara province) will be subjected to increased durations of periods without rainfall and higher rainfall during the early wet season (Brown et al., 2013). ENSO will continue to be the main driver of interannual variability in rainfall across the region, with potentially greater rainfall extremes in a future warmer climate (Brown et al., 2013). Against this background, we selected a site in the karst landscapes of Sumba (East Nusa Tenggara), which was a focal area for land restoration programs from 2009 to 2018. It is important to understand both the potential for success of these programs in the context of moisture availability, as well as any potential influences of increased tree cover on hydrology, including water availability in springs for human use. Therefore, the aim of this research was to provide insights into the response of vegetation to available moisture through analyses of NDVI, rainfall, and soil moisture trends.
Our analysis aimed to characterise the interannual (1999–2018) NDVI trends at 10 patches of karst savanna vegetation and determine the influence of available moisture on these trends to inform land restoration projects. The 10 patches of savanna vegetation include three sites with shallow groundwater access and seven sites with deep (>20 m) groundwater. The seven sites with deep groundwater access are classified as: 1) sites with no intervention (n = 3) and; 2) sites with restoration intervention (n = 4). We hypothesised that: 1) at the landscape scale, NDVI is correlated with soil moisture and rainfall; 2) NDVI at the three sites where groundwater is readily available is less sensitive to rainfall; 3) interannual variability of rainfall and rainfall anomalies are important drivers of NDVI; and 4) positive trends in interannual NDVI can be detected at the four restoration sites irrespective of moisture trends.
2 Materials and methods
2.1 Study period
The NDVI response of vegetation was observed through analysis of satellite imagery between 1999 and 2018, while rainfall records from 1974 to 2018 were used to determine long-term rainfall characteristics.
2.2 Study area
The study site is in the Haharu district, East Sumba, East Nusa Tenggara, Indonesia. It is an area of 796 km2 within the tropical savanna climate zone of East Indonesia (centred around 9°28′S, 119°58′E). This study area is underlain by the Kaliangga formation, an area of uplifted quaternary reef limestone with some karst formation (Effendi and Apandi, 1981).
Mean annual rainfall is 862 mm (coefficient of variation 28%) (Badan Meterologi Klimatologi dan Geofisika BMKG, 2020), most of which falls over the wet season between November and May Figure 1). Mean annual temperatures are 17.4°C (minimum) to 28.5°C (maximum) (Badan Meterologi Klimatologi dan Geofisika BMKG, 2020). The main weather systems influencing the study area are the northwest monsoon from November to May and the dry southeast monsoon from June to September (Aldrian and Dwi Susanto, 2003). ENSO and the Indian Ocean Dipole are known to contribute to interannual rainfall anomalies in the study area, with the strongest influence of both climate drivers occurring during the dry season (Supari et al., 2018; Kurniadi et al., 2021). Sumba island is among the driest islands in Indonesia (As-syakur et al., 2013), and an understanding of inter-seasonal moisture dynamics is a focus for this reason.
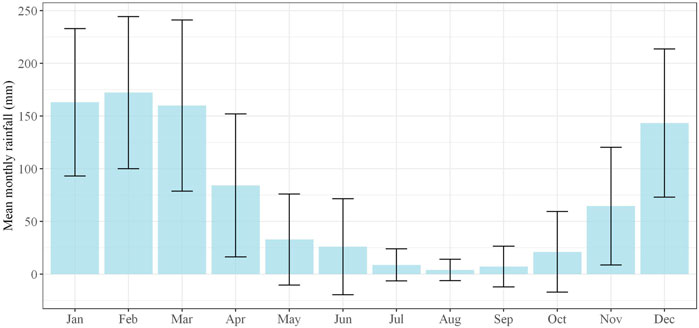
Figure 1. Long-term monthly rainfall in Waingapu, East Sumba, from 1974 to 2018. Source: Badan Meterologi Klimatologi dan Geofisika (BMKG), (2020). Error bars indicate one standard deviation.
The proportion of tree cover has fluctuated in Sumba during the past 25,000 years in response to water stress, with abrupt changes particularly apparent during periods of dry season aridity (Dubois et al., 2014). In addition, anthropogenic landscape influences during recent times, including the use of fire and the clearing of trees for domestic and agricultural needs, may have enhanced this landscape change (Monk, 1997). In selected locations in Sumba, however, tree-clearing trends have been reversed in the last two decades as a result of restoration efforts (Pellokila et al., 2014).
Soils in the Haharu district are shallow, stony, and calcareous, with relatively low to moderate clay content and associated low water holding capacity. Some areas of heavier clay soils retain moisture for longer periods and can become waterlogged in particularly wet periods (Monk, 1997). The soil texture at each of the 10 selected vegetation patches was sandy clay.
2.3 Vegetation and vegetation patch selection
Savanna grasslands are a dominant vegetation type in the study area, having been subject to minimal development for a mix of land uses, including household-scale agroforestry, vegetable gardening, and livestock grazing (Mulyoutami et al., 2016; Seran Mau et al., 2017). Generally, the vegetation in the study area comprises a dense understory of perennial grasses: alang-alang (Imperata cylindrica), kangaroo grass (Themeda triandra), and black speargrass (Heteropogon contortus) interspersed with both evergreen and deciduous trees (Monk, 1997). Shallow groundwater sites support evergreen trees, including local mango (Buchanania arborescens), mahogany (Swietenia macrophylla), teak (Tectona grandis), tamarind (Tamarindus indica) and figs (Ficus species), and the semi-deciduous lac tree (Schleichera oleosa). Away from shallow groundwater, scattered tree species include the evergreen Chinese apple (Ziziphus mauritiana), cassia (Senna siamea), and deciduous gmelina (Gmelina arborea). At deep groundwater sites with restoration interventions, species that have been cultivated include teak, injuwatu (Pleiogynium timoriense), mahogany, cashew (Anacardium occidentale), and gmelina (Monk, 1997; Pellokila et al., 2014).
The ten 1 ha vegetation patches were selected within the district according to their depth to groundwater and land management status. Three sites are located next to groundwater discharge points, which support evergreen vegetation that has been actively conserved, maintained, or restored, while the seven other sites are in areas where groundwater is unlikely to be available to trees (i.e., water table between 21 m and 47 m below ground level). The seven sites with deep groundwater were further classified as deep groundwater sites with no intervention (n = 3) and deep groundwater sites with managed vegetation restoration activities (n = 4) (Figure 2; Table 1).
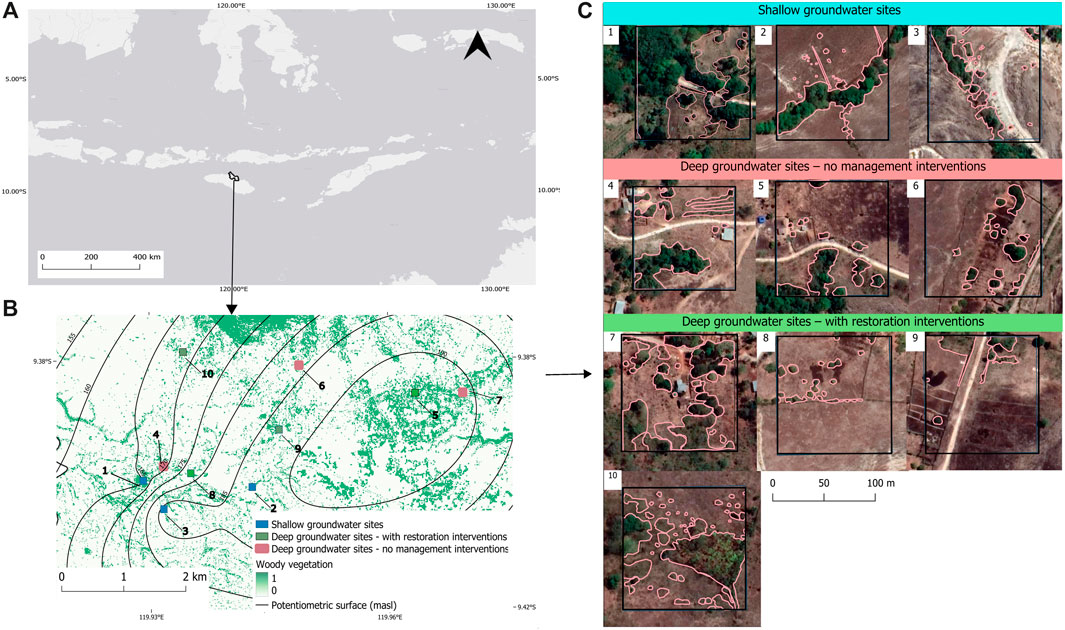
Figure 2. (A) Location of the study area in the East Sumba regency of East Nusa Tenggara, Indonesia; (B) detailed study area indicating the location of vegetation patches, with site type code by colour; the potentiometric surface (5 m depth contours); woody and non-woody vegetation cover; (C) delineation of woody canopy cover at each site on Google satellite imagery (Map data ©2015 Google).
To determine the proportion of woody and non-woody vegetation in the study area and in 1-ha vegetation patches, we completed a supervised classification using field-verified training points of 15 landcover classes on a composite image. The composite image was created from median pixel values of cloud-free multispectral Sentinel images between 01/01/2018 and 01/07/2018 at 10 m resolution in Google Earth Engine (GEE) (Coleman et al., 2020). These dates were selected to correspond with a period of field verification of landcover. We then reclassified the 15 land cover classes to a binary woody and non-woody grid, where woody pixels were defined as those dominated by C3 tree and shrub species, and non-woody pixels were dominated by C4 grasses, surface water, or bare ground (Supplementary Table S1). Woody cover fraction for each 1 ha vegetation patch polygon was determined using the Grid Statistics for Polygons module in Saga GIS 7.2.0 (Conrad et al., 2015), and the performance of this classification was checked against a manual delineation of woody canopy cover. Canopy cover was delineated manually by outlining canopies and summing canopy polygon areas in QGIS 3.10.6 (QGIS Development Team, 2020) using high-resolution Google satellite imagery (Google and Maxar Technologies, 2018), and samples of canopy areas were ground-truthed at each site.
2.4 Rainfall data
Rainfall time series data from the proximal Bureau of Meteorology, 2021 (BMKG) rainfall station in Waingapu (51 km away in a straight line from the field site) were used to establish long-term regional rainfall trends. The time period for this long-term rainfall data baseline was from January 1974 to December 2014. Rainfall data from the Climate Hazards Group Infrared Rainfall with Station (CHIRPS) daily 0.05° × 0.05° gridded time series product were accessed through GEE for comparison with daily local manual gauge data collected between July 2015 and December 2017 and proximal BMKG gauge data (Figure 3). With the daily local gauge data as a reference dataset, Spearman’s rank-based correlations were calculated for the CHIRPS and BMKG data to determine the most accurate dataset to represent rainfall in the study area (Supplementary Table S2).
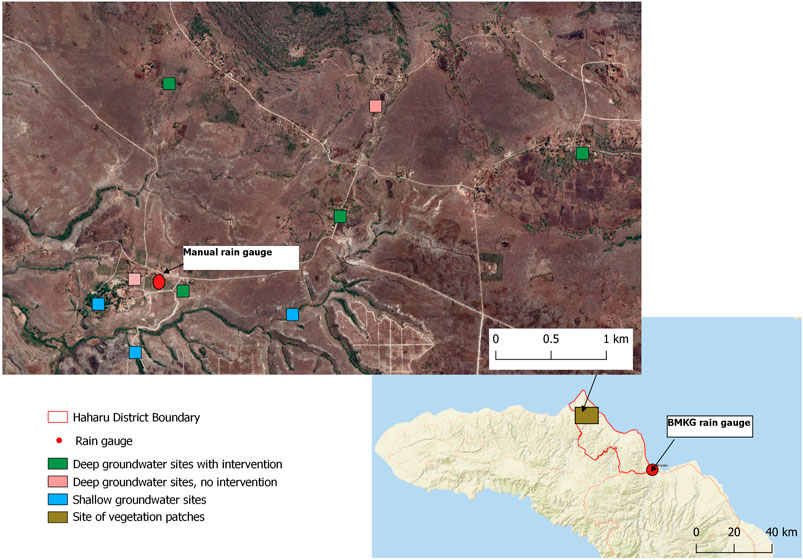
Figure 3. Location of rain gauges relative to the study area. The local daily manual gauge is indicated close to the vegetation patches. The BMKG rain gauge is shown just outside the Haharu district boundary.
We defined the wet season as commencing on 1 November and concluding on 30 May, and rainfall events outside of the wet season were classified as dry season rain. Commencement of the wet season is based on the definition by Peel et al. (2007) that a wet season month receives >60 mm on average. Water years were defined as the periods from 1 November of a given year to 30 October of the following year and were then classified as very dry to very wet to assess the influence of interannual rainfall anomalies on NDVI (Table 2).
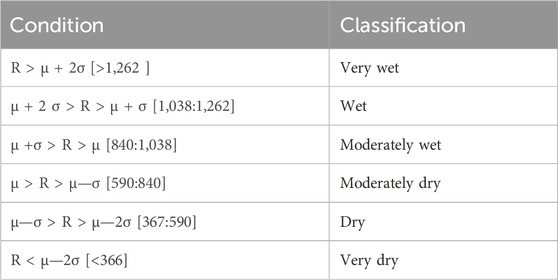
Table 2. Wet season classification criteria. Water years are classified according to deviation from the mean. R = annual rainfall, μ = long-term mean annual rainfall, and σ = standard deviation. Rainfall amounts are shown in mm.
Historical Troup Southern Oscillation Index (SOI) data were obtained from the Bureau of Meteorology, Australia (Bureau of Meteorology (BOM), 2021), to examine the influence of ENSO on rainfall anomalies and moisture availability. While the classification of El Niño and La Niña events varies according to meteorological institution (Van Oldenborgh et al., 2021), for simplicity, we defined El Niño (La Niña) years as those with annual mean SOI below −7 (above +7) (Supplementary Figure S1).
2.5 Soil moisture and groundwater estimate
The recent Soil Moisture Active Passive (SMAP) (Entekhabi et al., 2010) product has demonstrated superior quality in measuring surface soil moisture condition compared with other operational products (Chen et al., 2014b; Cui et al., 2018; Kumar et al., 2018). However, soil moisture retrieved from SMAP only represents the soil moisture content in up to the top 5 cm of the soil, and it is incomplete in space and time. Data assimilation, which is the process of optimally combining model simulations with independent observations to improve the accuracy of model estimation (Entekhabi et al., 2010), is the most widely used approach to obtain soil moisture estimates. In this study, we derived values for surface soil moisture and root zone soil moisture from the gridded 0.1° × 0.1° soil moisture time from the Satellite-Guided Root-zone moisture Analysis and Forecasting System (S-GRAFS) (Tian et al., 2023).
To characterise seasonal access to moisture for each patch, we estimated the depth to groundwater and distance to drainage lines. For depth to groundwater, the potentiometric surface across the study area was generated in Saga GIS 7.2.0 (Conrad et al., 2015). Groundwater elevation contours were interpolated on a 30 m × 30 m digital elevation surface from known mean depth to groundwater at six points (community-managed wells and springs) within a 5 km × 5 km area using multilevel B- Spline Interpolation (Supplementary Figure S2).
2.6 NDVI data
Most published analyses of vegetation responses to rainfall in savanna landscapes, such as MODIS NDVI, Global Inventory Modelling and Mapping Studies (GIMMS) NDVI and MODIS leaf area index (LAI) time series data, are applied at a coarse spatial resolution (>250 m), which has the advantage of long historical records and high frequency. However, analyses at this coarse scale do not have the ability to detect small, localised fluxes in vegetation productivity driven by fine-scale hydrological or landcover changes. In addition, measurements of NDVI at lower resolution tend to underestimate the proportion of photosynthetically active vegetation due to aggregation with surrounding less vegetated patches (Munyati and Mboweni, 2013). Thus, in this study, we extracted data from MODIS imagery for district-scale analyses and from Landsat 7 imagery for patch-scale analyses. We also trialled Sentinel 2 data, but as the data are only available from 2015 onwards, we found that the dataset was insufficient for time series analysis.
NDVI data were derived from the 30 m Landsat (LANDSAT/LE07/C01/T1_SR, n = 360) and 500 m MODIS (MOD13A1.061, n = 434) image collections in GEE for the period 1999 to 2018. For Landsat data, the cloud and cloud shadow QA bands were used to eliminate pixels containing cloud and red band saturation. Landsat 7 images post 31/05/2003 had some missing data due to failure of the scan line corrector, amounting to 22% data loss per scene (Loveland and Dwyer, 2012). However, this does not greatly impact NDVI time series analysis (Zhu, 2017). It is also preferable to derive NDVI consistently from Landsat 7 rather than gap-fill with data derived from Landsat 5 retrievals, as the red reflectance values differ between the two sensors (Teillet et al., 2001; Sulla-Menashe et al., 2016), and a change in orbit resulted in inconsistencies in values (Roy et al., 2016). For MODIS, the MOD13A1.061 image collection available in GEE is atmospherically corrected and masked for water, clouds, and cloud shadow. The study period was set as 1999 to 2018 to provide sufficient observations for trend analyses and to coincide with water and soil sampling for related studies between 2014 and 2018.
2.7 Statistical analyses
Statistical tests were conducted with R version 4.0.3 (R Core Team, 2020) using the “Kendall” (v2.21; McLeod, 2022), “modifiedmk” (v1.6; Patakamuri, 2021), and “zyp” packages (Brounaugh et al., 2013). A link to the full reproducible code is provided in the data availability statement. To investigate the temporal correlation between monthly NDVI and moisture parameters (rainfall and soil moisture), a windowed cross-correlation (WCC) was applied. In the WCC method, a temporal window is selected to examine the changes in correlation between two time series (Boker et al., 2002), and this enabled us to determine the period that NDVI lags behind available moisture. The correlations between 1-month to 12-month antecedent rainfall and NDVI were calculated for each vegetation patch, while a linear regression model was used between total annual rainfall and mean annual NDVI to analyse the sensitivity of each vegetation patch to changes in available moisture (adapted from Liu et al., 2017; Aguilar et al., 2012; Chamaillé-Jammes and Fritz, 2009). For soil moisture, pixel-wise correlations with NDVI were determined at the scale of the soil moisture grid (0.1° × 0.1°) for the period 2015 to 2018. Results calculated on water years are referred to hereafter as “annual” values. Because the NDVI and rainfall data were not normally distributed, a Spearman rank-based correlation test was used to identify sites where mean annual NDVI had the strongest relationship with annual rainfall.
To test for increasing or decreasing trends in monthly NDVI and rainfall time series, non-parametric Mann–Kendall (MK) trend analyses were applied. MK was chosen because it is an appropriate method for detecting trends in time series data irrespective of outliers, and it is commonly used in hydrological studies (e.g., Hamed, 2008). The null and alternative hypotheses for the MK were: H0: data are independent and randomly ordered; and Ha: there is a monotonic trend. The MK test statistic (ZMK) was calculated using the block bootstraped Mann–Kendall trend test (Hippel and McLeod, 1994). The block bootstrapping method accounts for autocorrelation in the time series (Önöz and Bayazit, 2012). We present the MK test statistic results, along with the 2-sided p-values, where α = 0.05, with 95% confidence intervals for the bootstrap statistics. Where significant trends were identified, the overall annual change (ΔNDVI y−1) in the time series was calculated as m × 12, where m is the slope of the Theil Sen regression line for the monthly NDVI time series (adapted from Sen, 1968; Venter et al., 2020).
Minimum, maximum, mean, and amplitude NDVI values were determined for the whole 1999:2018 Landsat time series and for subsets of the time series based on classes of wet seasons (from very dry to very wet; see Section 2.3). Mean minimum and maximum NDVI values indicate the extremes of photosynthetic activity for each vegetation patch, while seasonal amplitudes provide insights into the split of vegetation patches between evergreen woody vegetation and deciduous shrubs and grasses (Forkel et al., 2013).
The Pearson’s correlations between NDVI and soil moisture gridded time series at two depths (surface and root zone) were calculated for all pixels in the Haharu district polygon for the period between 2015 and 2018. Linear regression was undertaken to determine the sensitivity of the soil moisture to the NDVI correlation of the fraction of woody cover. All vegetation patches are located within the same 0.1° × 0.1° soil moisture pixel, so an inter-site comparison of soil moisture was not possible.
The process for selection and preparation of datasets and methods of data analysis are summarised in Figure 4.
3 Results
3.1 Rainfall trends
Monthly rainfall data in the period between May 2015 and June 2018 from both the proximal BMKG gauge and the CHIRPS gridded dataset were closely correlated with local rainfall measurements (rho = 0.843, p-value <0.01 and rho = 0.838, p < 0.01, respectively, Supplementary Table S2). We selected the BMKG gauge data for use in further analyses due to the slightly higher correlation result. We found that the five driest years between 1974 and 2018, with rainfall anomalies ranging between −2.08 and −1.14, all coincided with El Niño events, and conversely, the four wettest years, with rainfall anomalies between +1.5 and +3.3, coincided with La Niña events (Supplementary Figure S1). There were no statistically significant trends in monthly rainfall using MK tests in the period between 1999 and 2018 (ZMK −0.03, p-value 0.43).
3.2 NDVI patterns and trends at the district scale
Woody landcover accounts for 32% of the district, while non-woody accounts for 68%. Dense woody areas with an NDVI >0.5 are largely confined to riparian corridors, steeper hillslopes, and groundwater discharge areas. NDVI values range from −0.53 to 0.83, and the district mean value is 0.36. Woody cover has an NDVI range of approximately 0.6–0.8, while grass dominated landcover is <0.5 (Figure 5). Negative NDVI values correspond to ephemeral water bodies in the district. As a general rule, NDVI was highest between February and April (late wet season) and lowest between September and November (late dry season). At the district scale using the MK test, we did not find significant long-term trends in the mean monthly MODIS (n = 434) or Landsat 7 (n = 356) time series data from 1999 to 2018.
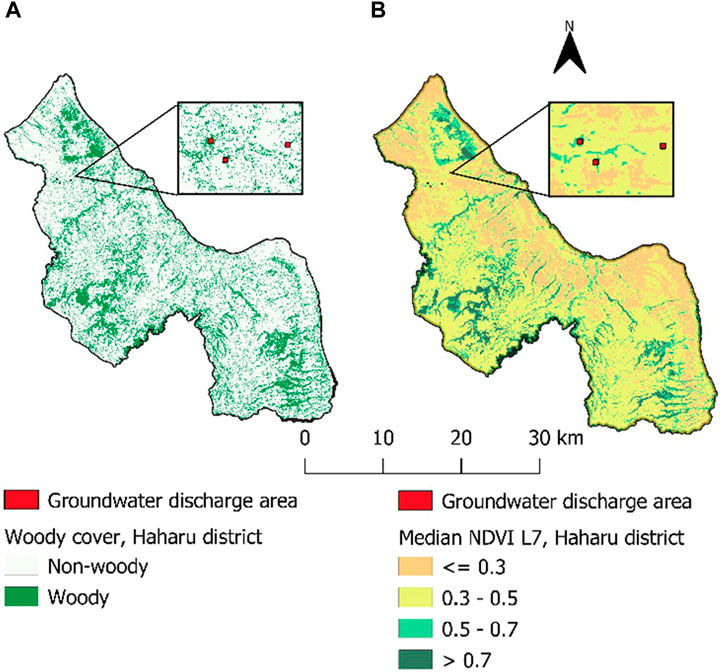
Figure 5. (A) Binary woody and non-woody classification derived from 15 land cover classes. (B) Median interannual NDVI in the Haharu district derived from Landsat 7 imagery.
3.3 Response of monthly NDVI and soil moisture to rainfall
Using the WCC method with windows of 1 month for the period between 1999 and 2018, a lag time of 4 months between rainfall and the Landsat 7 NDVI monthly time series gave the highest correlation (r = 0.71, p = <0.001), with similar yet lower values for rainfall lags of 2 months, 3 months, 5 months, and 6 months (Figure 6A). To compare WCC results with soil moisture data, which is only available for the period between 2015 and 2018, we also computed WCC for this subset of the time series. In the subset of the time series, we found that a lag of 2 months gave the highest correlation, followed by 1 month (Figure 6B). We attribute this difference to the three consecutive moderately dry years from 2015 to 2017, where response to rainfall would have been more rapid (Ahmed et al., 2017).
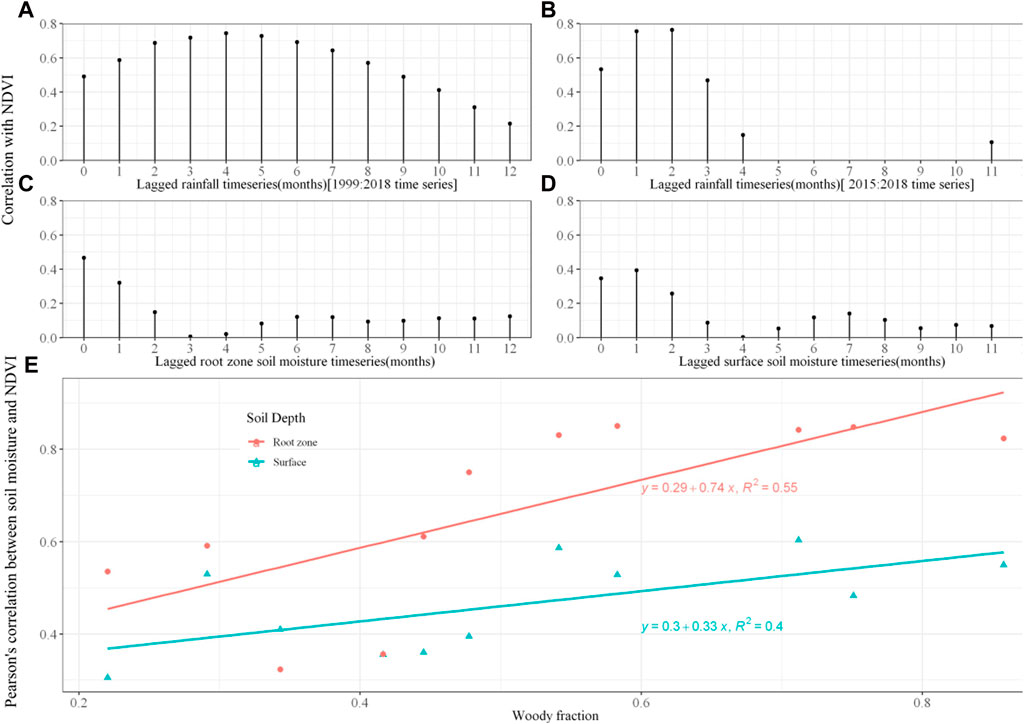
Figure 6. (A) Cross-correlation values for monthly rainfall vs monthly Landsat 7 derived NDVI between 1999 and 2018, (B) rainfall vs Landsat 7 derived NDVI between 2015 and 2018, (C) root-zone soil moisture vs NDVI, (D) surface soil moisture vs NDVI, and (E) Pixel-wise correlation between annual soil moisture and NDVI at the root zone and at the surface as a factor of woody cover fraction.
The cross-correlation between NDVI and soil moisture differed by soil depth. We found that the overall correlation between surface soil moisture (0–5 cm depth) and NDVI for all valid pixels within the district polygon was highest with a 1-month lag (0.40, p < 0.001) followed by no lag (0.35, p < 0.001) (Figure 6D). For soil moisture in the root zone (0–1 m depth), the highest correlation was found with no lag (0.47, p < 0.001), followed by a 1-month lag (0.32, p < 0.001) (Figure 6C). For pixels within the district where the fraction of woody cover was >0.5, monthly NDVI was highly correlated with root-zone soil moisture (between 0.82 and 0.85), while surface soil moisture was not as well correlated (between 0.48 and 0.6). The fraction of woody cover had a stronger influence on the NDVI soil moisture relationship in the root zone than in the shallow soil zone (Figure 6E).
3.4 NDVI trends at the patch scale
We did not find a consistent relationship between site type and NDVI minimum, maximum, mean, or amplitude values, nor did we find a consistent influence of the fraction of woody cover on these results (Supplementary Table S3). A significant decreasing trend in annual NDVI amplitudes was found at a deep groundwater site with no intervention (site 4; ZMK −0.56, p-value 0.001) and an increasing trend at a deep groundwater site with restoration intervention (site 8; ZMK 0.439, p-value 0.010). This suggests a potential change in the woody and grasscover fractions or the effect of anomalous wet periods on grass productivity at these two sites (Chamaille-Jammes et al., 2006; Ma et al., 2013; Kahiu and Hanan, 2018). No trend in NDVI amplitude was found at the remaining sites, suggesting no significant change over the study period in the woody and grasscover fractions at these sites. For mean monthly NDVI values, we found significant positive trends at a shallow groundwater site (site 1; ZMK 0.318, p-value <0.001, Δ 3.74 × 10−4 y−1) and at a deep groundwater site with restoration intervention (site 9; ZMK 0.238, p-value <0.001, Δ 2.7 × 10−4 y−1). Two additional restoration sites (sites 7 and 8) had positive trends; however, we could not conclude that these were significant trends as p-values were ≥0.5 (Supplementary Table S4).
Using WCC, we established that a lag time of 2 months between rainfall and the Landsat 7 NDVI monthly time series gave the highest correlation for most sites, ranging between 0.49 (site 4) and 0.76 (site 8) (Table 3). Whilst the NDVI at two of the shallow groundwater sites had low correlations with rainfall at 0 month, 1 month, and 2 months, and groundwater sites with restoration interventions were the most sensitive of all sites with lagged rainfall, we found that the correlation could not be predicted by site type alone, as site 2 (a shallow groundwater site) had higher correlations with lagged rainfall than deep groundwater sites.
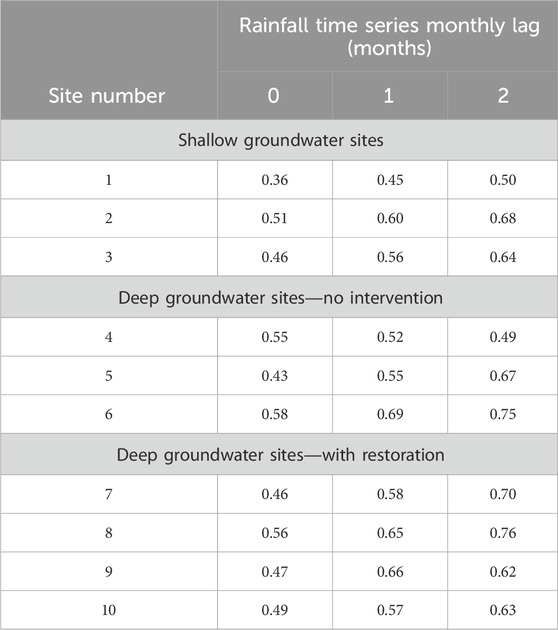
Table 3. Pearson’s correlation (r) of monthly NDVI by lagged monthly rainfall time series (0 month, 1 month, and 2 month lags).
There was a significant relationship between mean annual NDVI and total annual rainfall at four of the seven deep groundwater sites (Spearman rank coefficients between 0.47 and 0.57) but no significant relationship for any of the shallow groundwater sites (Spearman rank coefficients between −0.17 and 0.16) (Table 4; Figure 7). These relationships were not well represented by linear models, as the data were not normally distributed (Supplementary Table S5).
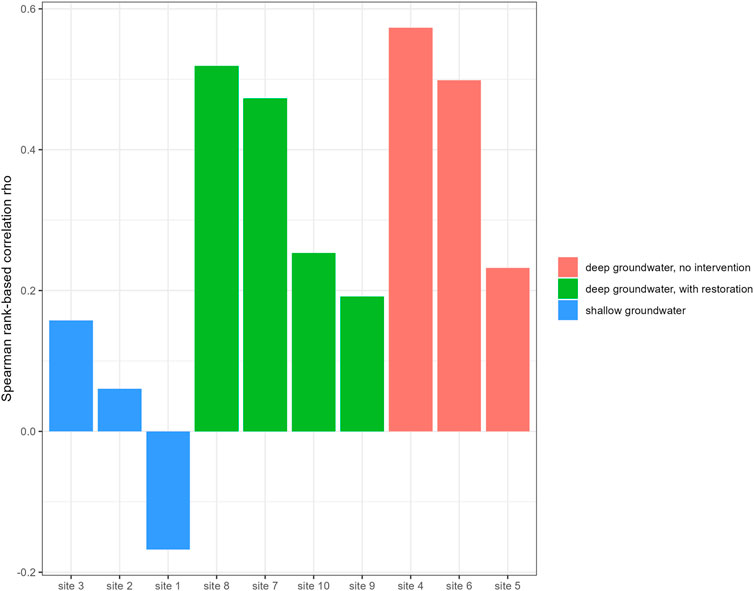
Figure 7. Spearman’s rank-based correlation of annual NDVI vs. annual rainfall for each site. Bars are grouped by site type.
3.5 Response of NDVI to anomalies of wet season rainfall
Most years in the study period had moderate to wetter than long-term mean rainfall. We found differences in the mean, median, and interquartile ranges of NDVI by site type in response to annual rainfall classes. Mean and median NDVI were highest for shallow groundwater sites across the whole period and all wet season classes. The range of NDVI values for shallow groundwater sites was significantly higher than other site types in the moderately dry and very dry periods. For the deep groundwater sites, both with and without restoration intervention, there was a significant difference in NDVI in the wet period relative to the whole study period, indicating a positive influence of additional rainfall on NDVI at these sites. For all site types, there was a statistically significant difference between median values in the very dry period relative to the very wet period. Mean and median values in the very wet period were lower than wet for all site types (Figure 8A–C), which can be explained by a decrease in NDVI in the late wet season in the very wet period (Figures 8D–F). We identified a threshold volume of annual rainfall in the order of 1,200 mm (between one and two standard deviations above mean rainfall), beyond which any additional rainfall had a neutral or negative effect on NDVI.
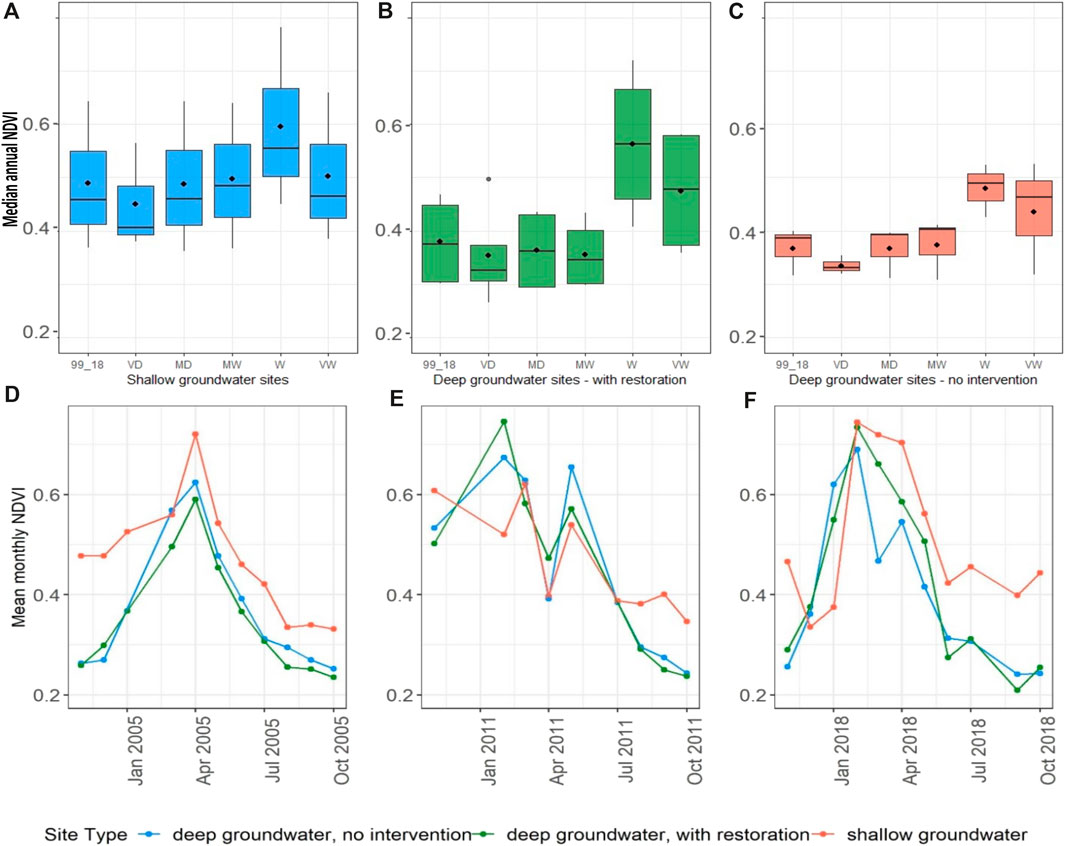
Figure 8. Distribution of median annual NDVI values by wet season classification: very dry (VD), moderately dry (MD), moderately wet (MW), wet (W), and very wet (VW) years and site type: (A) Shallow groundwater sites, (B) Deep groundwater sites—with restoration, and (C) Deep groundwater sites—no intervention. Boxes span the interquartile range, lines denote the median, and circles denote the mean and mean monthly NDVI by site type in a (D) VD, (E) VW, and (F) W year.
4 Discussion
4.1 Response of NDVI reliably linked to rainfall and soil moisture
Our results show that in tropical karst savanna areas subject to high rainfall seasonality, the NDVI correlation to rainfall can reliably be linked to the presence of subsurface moisture. Specifically, where subsurface moisture is depleted, the correlation between NDVI and rainfall will be high and vice versa. In line with our first hypothesis, we found that root-zone soil moisture was correlated with NDVI in the corresponding (lag0) and preceding month (lag1). Shallow soil moisture was also correlated with NDVI, although not as strongly. These findings agree with previous studies such as Ahmed et al. (2017), who used the 0.5° global monthly soil moisture data and GIMMS NDVI3g to identify correlations between NDVI and soil moisture in the Sahel region of Africa. The study found that the highest correlation between the two variables was between lag0 and lag1 and that south of the study region, where mean rainfall is higher, longer lag times gave the highest correlations. Ahmed et al. (2017) also identified that during a wetter phase, the lag time that gave the highest correlation was greater compared with a more normal phase of rainfall. We were unable to test this, given the short soil moisture time series, and we note this as a limitation. Our data showed that the strength of the correlation between soil moisture and NDVI increased in line with the woody cover fraction. The correlation between woody cover, soil moisture, and NDVI has previously been reported, for example, by Tian et al. (2019), who demonstrated an NDVI–surface soil moisture correlation in grasslands and an NDVI–deep soil moisture correlation in sites with higher woody fractions (shrub and forest sites). Our finding of a rapid response of NDVI to soil moisture corresponds with the results of cross-correlation analyses of soil moisture and the 1-km GIMMS NDVI3g in Australia from 1996 to 2005 (Chen et al., 2014b). It is important to note that in our study, soil moisture had a reasonable correlation with NDVI, particularly where woody fractions are higher. However, soils on the study sites are shallow (approximately 30 cm deep), mostly with limited water storage capacity. Therefore, during the early to mid-dry season, the soil moisture index may represent moisture in the epikarst, the zone between soil and bedrock in karst environments (Querejeta et al., 2007; Schwinning, 2008). This zone is rapidly recharged and more resistant to evaporation than the soil zone (Swaffer et al., 2014). Carbonate bedrock can provide accessible sources of water for plants with sufficiently deep roots during dry periods. However, the available water content is dependent on the characteristics of the rock, such as its primary porosity (Estrada-Medina et al., 2013; Nardini et al., 2021). The roots of some of the dominant tree species in our study sites have been observed to grow into deep fractures in the bedrock to access moisture (Meha, 2019; personal communication, 4 February).
4.2 Groundwater access reduces sensitivity to rainfall inputs
While soil moisture provides the most accurate representation of plant-available moisture, antecedent rainfall also provides an indication of moisture availability. At the patch scale in our study, NDVI was most strongly correlated with 2 months to 6 months of antecedent rainfall (lag2 to lag6); however, there was a clear decoupling between NDVI and antecedent rainfall at the three sites with shallow groundwater access, in accordance with our second hypothesis. We attribute this result to the fact that tree growth at these sites was much less dependent on rainfall inputs (Ma et al., 2013). Furthermore, we found that NDVI was consistently higher at sites with shallow groundwater access, which is comparable to findings by O'Grady et al. (2011) that LAI increased with groundwater access (NDVI is a function of LAI; Carlson and Ripley, 1997). This is explained by the concept of ecological optimality, where ecosystems evolve to equilibrate with available moisture (Schymanski et al., 2009). While vegetation productivity is water-limited in tropical savanna systems (Eamus et al., 2000a; Eamus et al., 2000a), the vegetation patches at our sites with groundwater access were less susceptible to short-term rainfall anomalies, as their hydraulic architecture likely evolved with available groundwater (Eamus et al., 2016). These sites, while less vulnerable to short-term dry periods, may be influenced by more severe dry phases or longer-term changes in rainfall patterns (Ma et al., 2013), which are likely to determine the volume of recharge and discharge to and from the groundwater store. Analysis of the relationship between NDVI and rainfall identified that the response of plant productivity to rainfall amounts was not uniform across vegetation patches. However, additional rainfall had a greater positive effect on sites with no groundwater availability to trees. This corresponds with the finding that rainfall pulses drive savanna vegetation greening responses at savanna sites in northern Australia; however, there are limited seasonal and interannual changes in enhanced vegetation index or LAI at sites with access to deep soil water or groundwater (Ma et al., 2013).
4.3 EL Niño events reduce vegetation productivity
It is valuable to relate the reported rainfall anomalies in the study region to ENSO events as a useful predictor of the impacts of ENSO on rainfall and vegetation productivity in the future. Climate studies have found that El Niño events generally occur every 2–5 years and that La Niña events are usually at least 7 years apart but are variably spaced (Wolter and Timlin, 2011). Previous studies, including Kurniadi et al. (2021) and Supari et al. (2018), reported a more significant influence of ENSO on rainfall during the dry season than the wet season in Indonesia. Consistent with these studies, we established that La Niña events resulted in higher rainfall totals and greater proportions of annual rainfall falling in the dry season. We also found that El Niño years resulted in lower than mean rainfall in the study area. More importantly, our results show that the vegetation productivity of this karst tropical savanna ecosystem was significantly impacted by strong El Niño events but recovered when followed by wetter periods resulting from La Niña conditions. Meanwhile, it was previously known that NDVI decreases in association with intense El Niño events (Erasmi et al., 2009; Arjasakusuma et al., 2018). Our study provides a detailed analysis of this relationship, specifically in the tropical karst savanna environment. Our finding supports our third hypothesis that interannual rainfall variability and anomalies are important drivers of NDVI in tropical karst savannas. Protracted dry periods may lead to a significant reduction in vegetation productivity and a long recovery period at both groundwater-dependent sites (due to a reduction in groundwater recharge) and at rainfall-dependent sites due to the absence of soil moisture (Chiloane et al., 2022). The wettest periods in the time series, the 2000 and 2011 La Niña years, did not contribute to significant changes in productivity. Annual rainfall thresholds for NDVI responses, such as the 1,200 mm that we identified, have been reported in related studies, such as Ahmed et al. (2017), Nicholson and Farrar (1994), Al-Bakri and Suleiman (2004), and Fu and Burgher (2015). A threshold of 1,100 mm per year or 200 mm per month across a range of vegetation types, including grassland/shrubland, was established by Nicholson et al. (1990), who studied the GIMMS NDVI3g response to rainfall in the Sahel and East Africa from 1982 to 1985. Nicholson and Farrar (1994) reported a threshold amount of approximately 500 mm per year or 50–100 mm per month on sandy soils in the semi-arid savanna of Botswana, illustrating a large variation in NDVI response of savanna vegetation due to site effects including latitude, mean rainfall trends, and soil type. Our study investigated the NDVI response to moisture at sites with consistent soil type, latitude, and annual rainfall as it was focussed on a small district scale. The study also observed the variation in responses in relation to access to groundwater and vegetation management activities.
In karst savannas, it has been found that maintenance of groundwater levels, and thus maintenance of vegetation productivity of groundwater-dependent sites, is dependent on successive wet periods (Cardella Dammeyer et al., 2016). Longer time series that include a greater number of ENSO events resulting in rainfall anomalies would provide a greater ability to predict vegetation response to climate conditions. While not considered in this study, other climate drivers, including the Indian Ocean Dipole (IOD), can influence dry season rainfall between June and November in Indonesia (Kurniadi et al., 2021). However, ENSO remains the main driver of interannual variability in the western Pacific monsoon rainfall (Kurniadi et al., 2021).
4.4 Effect of vegetation restoration on long-term NDVI trends
While we found that there was no increasing or declining long-term trend in NDVI at the district scale, our results at the patch scale identified two sites with significantly increasing interannual NDVI trends (an increase of 3.74 × 10−4 NDVI yr−1 and 2.7 × 10−4 NDVI yr−1 at sites one and nine respectively). Both sites are subject to woody vegetation conservation and restoration (Pellokila et al., 2014). We attribute the increasing NDVI trends to the restoration activities that commenced in 2013 at both sites. We did not identify positive trends at the other sites with restoration activities, which could be related to lower intensity of tree planting, less seasonal intercropping with cash crops, or less effective tree management approaches and/or mitigating factors such as damage from fire and livestock (Pellokila et al., 2014, V. Sabathini, personal communication, 07 July 2021). It would be valuable to repeat the analysis at additional restoration sites within the study region to establish whether positive trends could be detected above the background regional greening trend.
Studies on long-term background NDVI trends in the region have provided varying results. Between 2000 and 2010 in Australia, a decline in both rainfall and NDVI was identified across 90% of the continent using 1 km resolution 10-day SPOT-derived NDVI surfaces (Liu et al., 2017). The study by Liu et al. (2017) examined the effect of a severely dry period on vegetation in Australia and, therefore, did not explore the effect of the 2011 La Niña event. In spanning a period that included the 2011 wet event (1982–2011), Chen et al. (2014a) found that the majority of the Asia–Australia region had no significant trend in monthly 0.25° resolution NDVI derived from the GIMMS dataset, with smaller areas showing an annual growth rate of 6.22 × 10−4. A slight increase of 5 × 105 yr−1 was reported for the Great Mekong Subregion in the period 1982 to 2013 by Han and Song (2022), while in Indonesia, de Jong et al. (2011) reported a decreasing trend from 1981 to 2006 by using a linear model of NDVI residuals, also derived from GIMMS NDVI3g.
Beyond identifying overall NDVI trends in the region, Wu et al. (2013) attempted to distinguish between background greening and revegetation efforts and suggested that an annual increase in MODIS NDVI of 6.0 × 10−4 between the years 2000 and 2010 in the Beijing–Tianjin Sand Source Region may have been due to an effective ecological restoration program. However, this increasing trend was not found to be significant. Wu et al. (2013) also acknowledged that it is difficult to separate the effects of climate change and human activity on changes in NDVI in their study. More recently, an annual increase in NDVI of 1.7 × 10−3 from 1999 to 2015 was identified in China using multiple linear regressions to investigate the effects of climate change and ecological restoration on NDVI (Song et al., 2022). This study also reported a relative contribution of 75% from afforestation to NDVI increases in most areas. Using residual trend analysis, Liang et al. (2023) monitored MODIS and GIMMS NDVI increases resulting from planting efforts in ecological reserves in China between 1982 and 2018 and identified annual growth rates up to 5.3 × 10−3. This large range of annual increases in NDVI in the Asia–Australia region from a minimum of 2.7 × 10−4 in our study to a maximum of 1.7 × 10−3 reported in China suggests that the choice of imagery, spatial and temporal scales, and statistical methods affects NDVI results. The variation in these methods likely influences the ability to accurately identify the response of vegetation to changes in moisture availability, especially in the karst savanna environment where trees are scattered.
4.5 Limitations of the study
Although we were able to clearly differentiate the NDVI response of groundwater-dependent vegetation patches from those reliant on rainfall, as well as detect the effect of managed vegetation restoration efforts at two sites, this was a small-scale study over an area of less than 800 km2. There remain some uncertainties about the relative contributions of climate and human intervention to changes in NDVI at each site, and it would be valuable to apply methods such as those in Liang et al. (2023) and Song et al. (2022) to assess the key driving factors in NDVI increases. In addition, our findings would be strengthened with an investigation of the correlation between soil moisture and rainfall in the study area over the full length of the study period, as well as changes in temporal dynamics between soil moisture and NDVI during extreme wet and dry years. In addition to the limitations of a short time series of soil moisture data, the coarse resolution of these data precluded an analysis of soil moisture trends at the vegetation patch scale. To further strengthen conclusions relating to the groundwater dependency of selected vegetation patches, future research should include analysis of higher-resolution soil moisture data.
5 Conclusion
Our study provides foundational insights into the relationship between available moisture and vegetation productivity in tropical karst savannas. We established that the NDVI-rainfall relationship in a tropical karst savanna region is a reliable predictor of shallow groundwater access or groundwater-dependent ecosystems. We also found that the fine spatial resolution of Landsat NDVI data is necessary to identify trends in NDVI at the patch scale. Through the use of MK trend analyses of Landsat 7 NDVI data, we identified a generally increasing trend at some, but not all, sites with managed restoration of woody vegetation. We also showed that interannual rainfall variability and rainfall anomalies related to ENSO are important drivers of NDVI, such that a deviation from the usual ENSO patterns may result in major changes to vegetation productivity.
Data availability statement
The datasets presented in this study can be found in online repositories. The names of the repository/repositories and accession number(s) can be found below: DOI: 10.5281/zenodo.13131831.
Author contributions
PG: conceptualization, data curation, formal analysis, investigation, methodology, project administration, software, visualization, writing–original draft. ST: conceptualization, methodology, resources, writing–review and editing. CD: conceptualization, methodology, project administration, supervision, visualization, writing–review and editing. PW: conceptualization, methodology, resources, supervision, writing–review and editing. NR: conceptualization, methodology, resources, writing–review and editing. AE: methodology, supervision, writing–review and editing.
Funding
The author(s) declare that financial support was received for the research, authorship, and/or publication of this article. This study was conducted as a chapter within a PhD thesis (not yet submitted at the time of publication).
Acknowledgments
We thank all staff of KOPPESDA, Waingapu Indonesia, for their assistance with the study in locating data sources and their support with field work; Ventia Sabathini (Wahana Visi Indonesia) for background information on revegetation works in the study area; Gianluca Scortechini (Fenner School of Environment and Society, Australian National University) for his assistance in generating JavaScript code for extracting data from Google Earth Engine; Prof. Lindsay Hutley (Research Institute for Environment and Livelihoods, Charles Darwin University) for early conceptual guidance; the Indonesian Ministry of Research and Technology for a permit for this research; Jakobis Messakh (Universitas Nusa Cendana) for thesis co-supervision and guidance in East Nusa Tenggara, Sara Beavis (Australian National University) for thesis supervision in Australia and, importantly, members of the Napu, Wunga, and Rambangaru villages in the Haharu district, East Sumba, for hosting and supporting us during fieldwork, sharing ideas, and allowing this research to be conducted.
Conflict of interest
The authors declare that the research was conducted in the absence of any commercial or financial relationships that could be construed as a potential conflict of interest.
Publisher’s note
All claims expressed in this article are solely those of the authors and do not necessarily represent those of their affiliated organizations, or those of the publisher, the editors and the reviewers. Any product that may be evaluated in this article, or claim that may be made by its manufacturer, is not guaranteed or endorsed by the publisher.
Supplementary material
The Supplementary Material for this article can be found online at: https://www.frontiersin.org/articles/10.3389/frsen.2024.1280712/full#supplementary-material
References
Acharya, B. S., Kharel, G., Zou, C. B., Wilcox, B. P., and Halihan, T. (2018). Woody plant encroachment impacts on groundwater recharge: a review. Water 10, 1466. doi:10.3390/w10101466
Aguilar, C., Zinnert, J. C., Polo, M. J., and Young, D. R. (2012). NDVI as an indicator for changes in water availability to woody vegetation. Ecol. Indic. 23, 290–300. doi:10.1016/j.ecolind.2012.04.008
Ahmed, M., Else, B., Eklundh, L., Ardo, J., and Seaquist, J. (2017). Dynamic response of NDVI to soil moisture variations during different hydrological regimes in the Sahel Region. Int. J. Remote Sens. 38, 5408–5429. doi:10.1080/01431161.2017.1339920
al-Bakri, J. T., and Suleiman, A. S. (2004). NDVI response to rainfall in different ecological zones in Jordan. Int. J. Remote Sens. 25, 3897–3912. doi:10.1080/01431160310001654428
Aldrian, E., and Dwi Susanto, R. (2003). Identification of three dominant rainfall regions within Indonesia and their relationship to sea surface temperature. Int. J. Climatol. 23, 1435–1452. doi:10.1002/joc.950
Anchang, J. Y., Prihodko, L., Kaptué, A. T., Ross, C. W., Ji, W., Kumar, S. S., et al. (2019). Trends in woody and herbaceous vegetation in the savannas of west Africa. Remote Sens. 11, 576. doi:10.3390/rs11050576
Arjasakusuma, S., Yamaguchi, Y., Hirano, Y., and Zhou, X. (2018). ENSO- and rainfall-sensitive vegetation regions in Indonesia as identified from multi-sensor remote sensing data. ISPRS Int. J. geo-information 7, 103. doi:10.3390/ijgi7030103
Asbjornsen, H., Goldsmith, G. R., Alvarado-Barrientos, M. S., Rebel, K., van Osch, F. P., Rietkerk, M., et al. (2011). Ecohydrological advances and applications in plant–water relations research: a review. J. Plant Ecol. 4, 3–22. doi:10.1093/jpe/rtr005
As-Syakur, A. R., Tanaka, T., Osawa, T., and Mahendra, M. S. (2013). Indonesian rainfall variability observation using TRMM multi-satellite data. Int. J. Remote Sens. 34, 7723–7738. doi:10.1080/01431161.2013.826837
BADAN METEROLOGI KLIMATOLOGI DAN GEOFISIKA (BMKG) (2020). BMKG data online. Available at: https://dataonline.bmkg.go.id/home (Accessed August 01, 2023).
Banks, E. W., Brunner, P., and Simmons, C. T. (2011). Vegetation controls on variably saturated processes between surface water and groundwater and their impact on the state of connection. Water Resour. Res. 47. doi:10.1029/2011wr010544
Beringer, J., Hutley, L. B., Abramson, D., Arndt, S. K., Briggs, P., Bristow, M., et al. (2015). Fire in Australian savannas: from leaf to landscape. Glob. change Biol. 21, 62–81. doi:10.1111/gcb.12686
BPDAS (2011). Mewujudkan bumi NTT hijau: potret kinerja rehabilitasi hutan and lahan di Provinsi Nusa Tenggara Timur, Kupang, Balai Pengelolaan Daerah Aliran Sungai Benain Noelmina.
Brandt, M., Hiernaux, P., Rasmussen, K., Tucker, C. J., Wigneron, J.-P., Diouf, A. A., et al. (2019). Changes in rainfall distribution promote woody foliage production in the Sahel. Commun. Biol. 2, 133. doi:10.1038/s42003-019-0383-9
Brown, J. R., Colman, R. A., Moise, A. F., and Smith, I. N. 2013. The western Pacific monsoon in CMIP5 models: model evaluation and projections. J. Geophys. Res. Atmos., 118, 12,475, doi:10.1002/2013jd020290
BUREAU OF METEOROLOGY (BOM) (2021). Southern oscillation index (SOI) since 1876, monthly data. bom.gov.au: Bureau of Meteorology.
Cardella Dammeyer, H., Schwinning, S., Schwartz, B. F., and Moore, G. W. (2016). Effects of juniper removal and rainfall variation on tree transpiration in a semi-arid karst: evidence of complex water storage dynamics. Hydrol. Process. 30, 4568–4581. doi:10.1002/hyp.10938
Carlson, T. N., and Ripley, D. A. (1997). On the relation between NDVI, fractional vegetation cover, and leaf area index. Remote Sens. Environ. 62, 241–252. doi:10.1016/s0034-4257(97)00104-1
Chamaillé-Jammes, S., and Fritz, H. (2009). Precipitation-NDVI relationships in eastern and southern African savannas vary along a precipitation gradient. Int. J. Remote Sens. 30, 3409–3422. doi:10.1080/01431160802562206
Chamaille-Jammes, S., Fritz, H., and Murindagomo, F. (2006). Spatial patterns of the NDVI–rainfall relationship at the seasonal and interannual time scales in an African savanna. Int. J. remote Sens. 27, 5185–5200. doi:10.1080/01431160600702392
Chen, B., Xu, G., Coops, N. C., Ciais, P., Innes, J. L., Wang, G., et al. (2014a). Changes in vegetation photosynthetic activity trends across the Asia–Pacific region over the last three decades. Remote Sens. Environ. 144, 28–41. doi:10.1016/j.rse.2013.12.018
Chen, T., de Jeu, R. A. M., Liu, Y. Y., van der Werf, G. R., and Dolman, A. J. (2014b). Using satellite based soil moisture to quantify the water driven variability in NDVI: a case study over mainland Australia. Remote Sens. Environ. 140, 330–338. doi:10.1016/j.rse.2013.08.022
Chen, Z., Wang, W., and Fu, J. (2020). Vegetation response to precipitation anomalies under different climatic and biogeographical conditions in China. Sci. Rep. 10, 830. doi:10.1038/s41598-020-57910-1
Chiloane, C., Dube, T., and Shoko, C. (2022). Impacts of groundwater and climate variability on terrestrial groundwater dependent ecosystems: a review of geospatial assessment approaches and challenges and possible future research directions. Geocarto Int. 37, 6755–6779. doi:10.1080/10106049.2021.1948108
Coleman, R. W., Stavros, N., Yadav, V., and Parazoo, N. (2020). A simplified framework for high-resolution urban vegetation classification with optical imagery in the Los Angeles megacity. Remote Sens. 12, 2399. doi:10.3390/rs12152399
Conrad, O., Bechtel, B., Bock, M., Dietrich, H., Fischer, E., Gerlitz, L., et al. (2015). System for automated geoscientific analyses (SAGA) v. 2.1.4. Geosci. Model. Dev. 8, 1991–2007. doi:10.5194/gmd-8-1991-2015
Cook, P. G., and O'Grady, A. P. (2006). Determining soil and ground water use of vegetation from heat pulse, water potential and stable isotope data. Oecologia 148, 97–107. doi:10.1007/s00442-005-0353-4
Cui, C., Xu, J., Zeng, J., Chen, K.-S., Bai, X., Lu, H., et al. 2018. Soil moisture mapping from satellites: an intercomparison of SMAP, SMOS, FY3B, AMSR2, and ESA CCI over two dense network regions at different spatial scales. Remote Sens., 10, 33, doi:10.3390/rs10010033
de Jong, R., de Bruin, S., de Wit, A., Schaepman, M. E., and Dent, D. L. (2011). Analysis of monotonic greening and browning trends from global NDVI time-series. Remote Sens. Environ. 115, 692–702. doi:10.1016/j.rse.2010.10.011
Dubois, N., Oppo, D. W., Galy, V. V., Mohtadi, M., van der Kaars, S., Tierney, J. E., et al. (2014). Indonesian vegetation response to changes in rainfall seasonality over the past 25,000 years. Nat. Geosci. 7, 513–517. doi:10.1038/ngeo2182
Eamus, D., Huete, A., and Yu, Q. (2016). “Water relations, hydraulic architecture and transpiration by plants,” in Vegetation dynamics: a synthesis of plant ecophysiology, remote sensing and modelling. Editors A. HUETE, D. EAMUS, and Q. YU (Cambridge: Cambridge University Press).
Eamus, D., O'Grady, A. P., and Hutley, L. (2000a). Dry season conditions determine wet season water use in the wet-tropical savannas of northern Australia. Tree Physiol. 20, 1219–1226. doi:10.1093/treephys/20.18.1219
Effendi, A. C., and Apandi, T. (1981). Geological map of Sumba quadrangle. Indonesia. doi:10.1016/0264-8172(94)90055-8
Entekhabi, D., Njoku, E. G., O'Neill, P. E., Kellogg, K. H., Crow, W. T., Edelstein, W. N., et al. (2010). The soil moisture active passive (SMAP) mission. Proc. IEEE 98, 704–716. doi:10.1109/jproc.2010.2043918
Erasmi, S., Propastin, P., Kappas, M., and Panferov, O. (2009). Spatial patterns of NDVI variation over Indonesia and their relationship to ENSO warm events during the period 1982–2006. J. Clim. 22, 6612–6623. doi:10.1175/2009jcli2460.1
Estrada-Medina, H., Santiago, L. S., Graham, R. C., Allen, M. F., and Jiménez-Osornio, J. J. (2013). Source water, phenology and growth of two tropical dry forest tree species growing on shallow karst soils. Trees 27, 1297–1307. doi:10.1007/s00468-013-0878-9
Ford, T. W., Harris, E., and Quiring, S. M. (2014). Estimating root zone soil moisture using near-surface observations from SMOS. Hydrol. Earth Syst. Sci. 18, 139–154. doi:10.5194/hess-18-139-2014
Forkel, M., Carvalhais, N., Verbesselt, J., Mahecha, M. D., Neigh, C. S. R., and Reichstein, M. 2013. Trend change detection in NDVI time series: effects of inter-annual variability and methodology. Remote Sens., 5, 2113–2144. doi:10.3390/rs5052113
Fu, B., and Burgher, I. (2015). Riparian vegetation NDVI dynamics and its relationship with climate, surface water and groundwater. J. Arid Environ. 113, 59–68. doi:10.1016/j.jaridenv.2014.09.010
Glenn, E. P., Nagler, P. L., and Huete, A. R. (2010). Vegetation index methods for estimating evapotranspiration by remote sensing. Surv. Geophys. 31, 531–555. doi:10.1007/s10712-010-9102-2
Goldscheider, N., Chen, Z., Auler, A. S., Bakalowicz, M., Broda, S., Drew, D., et al. (2020). Global distribution of carbonate rocks and karst water resources. Hydrogeology J. 28, 1661–1677. doi:10.1007/s10040-020-02139-5
GOOGLE and MAXAR TECHNOLOGIES (2018). Google satellite imagery. Available at: https://earth.google.com/(Accessed August 01, 2023).
Hamed, K. H. (2008). Trend detection in hydrologic data: the Mann–Kendall trend test under the scaling hypothesis. J. Hydrology 349, 350–363. doi:10.1016/j.jhydrol.2007.11.009
Han, Z., and Song, W. (2022). Interannual trends of vegetation and responses to climate change and human activities in the Great Mekong Subregion. Glob. Ecol. Conservation 38, e02215. doi:10.1016/j.gecco.2022.e02215
Hippel, K. W., and Mcleod, A. I. (1994). Time series modelling of water resources and environmental systems. Cambridge: Elsevier.
Jones, W. K. (2013). Physical structure of the epikarst. Acta Carsologica 42, 311–314. doi:10.3986/ac.v42i2-3.672
Kahiu, M. N., and Hanan, N. P. (2018). Estimation of woody and herbaceous leaf area index in sub-saharan Africa using MODIS data. J. Geophys. Res. Biogeosciences 123, 3–17. doi:10.1002/2017jg004105
Klaas, D. (2008). Indigenous water management: sustainable water conservation strategies in karstic dominated area in rote island, NTT province, Indonesia. Masters of engineering science (research). Monash Univ.
Kong, D., Miao, C., Wu, J., Zheng, H., and Wu, S. (2020). Time lag of vegetation growth on the Loess Plateau in response to climate factors: estimation, distribution, and influence. Sci. Total Environ. 744, 140726. doi:10.1016/j.scitotenv.2020.140726
Kumar, S. V., Dirmeyer, P. A., Peters-Lidard, C. D., Bindlish, R., and Bolten, J. (2018). Information theoretic evaluation of satellite soil moisture retrievals. Remote Sens. Environ. 204, 392–400. doi:10.1016/j.rse.2017.10.016
Kurniadi, A., Weller, E., Min, S. K., and Seong, M. G. (2021). Independent ENSO and IOD impacts on rainfall extremes over Indonesia. Int. J. Climatol. 41, 3640–3656. doi:10.1002/joc.7040
Lamontagne, S., Cook, P. G., O'Grady, A., and Eamus, D. (2005). Groundwater use by vegetation in a tropical savanna riparian zone (Daly River, Australia). J. Hydrology 310, 280–293. doi:10.1016/j.jhydrol.2005.01.009
Lehmann, C. E. R., Anderson, T. M., Sankaran, M., Higgins, S. I., Archibald, S., Hoffmann, W. A., et al. (2014). Savanna vegetation-fire-climate relationships differ among continents. Sci. Am. Assoc. Adv. Sci. 343, 548–552. doi:10.1126/science.1247355
Liang, W., Quan, Q., Wu, B., and Mo, S. (2023). Response of vegetation dynamics in the three-north region of China to climate and human activities from 1982 to 2018. Sustainability 15, 3073. doi:10.3390/su15043073
Liu, N., Harper, R. J., Dell, B., Liu, S., and Yu, Z. (2017). Vegetation dynamics and rainfall sensitivity for different vegetation types of the Australian continent in the dry period 2002–2010. Ecohydrology 10, e1811. doi:10.1002/eco.1811
Loveland, T. R., and Dwyer, J. L. (2012). Landsat: building a strong future. Remote Sens. Environ. 122, 22–29. doi:10.1016/j.rse.2011.09.022
Ma, X., Huete, A., Yu, Q., Coupe, N. R., Davies, K., Broich, M., et al. (2013). Spatial patterns and temporal dynamics in savanna vegetation phenology across the North Australian Tropical Transect. Remote Sens. Environ. 139, 97–115. doi:10.1016/j.rse.2013.07.030
Mulyoutami, E., Sabastian, G., and Roshetko, J. (2016). in Gendered knowledge and perception in managing grassland areas in East Sumba, Indonesia. Editor W. A. C. I. S. A. R. PROGRAM (Indonesia: Bogor).
Munyati, C., and Mboweni, G. (2013). Variation in NDVI values with change in spatial resolution for semi-arid savanna vegetation: a case study in northwestern South Africa. Int. J. Remote Sens. 34, 2253–2267. doi:10.1080/01431161.2012.743692
Murphy, B. P., and Bowman, D. M. J. S. (2012). What controls the distribution of tropical forest and savanna? Ecol. Lett. 15, 748–758. doi:10.1111/j.1461-0248.2012.01771.x
Myers, B. A., Duff, G. A., Eamus, D., Fordyce, I. R., O'Grady, A., and Williams, R. J. (1997). Seasonal variation in water relations of trees of differing leaf phenology in a wet-dry tropical savanna near Darwin, northern Australia. Aust. J. Bot. 45, 225–240. doi:10.1071/bt96015
Nardini, A., Petruzzellis, F., Marusig, D., Tomasella, M., Natale, S., Altobelli, A., et al. (2021). Water ‘on the rocks’: a summer drink for thirsty trees? New Phytol. 229, 199–212. doi:10.1111/nph.16859
Nicholson, S. E., Davenport, M. L., and Malo, A. R. (1990). A comparison of the vegetation response to rainfall in the Sahel and East Africa, using normalized difference vegetation index from NOAA AVHRR. Clim. change 17, 209–241. doi:10.1007/bf00138369
Nicholson, S. E., and Farrar, T. J. (1994). The influence of soil type on the relationships between NDVI, rainfall, and soil moisture in semiarid Botswana. I. NDVI response to rainfall. Remote Sens. Environ. 50, 107–120. doi:10.1016/0034-4257(94)90038-8
Nie, Y.-P., Chen, H.-S., Wang, K.-L., and Yang, J. (2012). Water source utilization by woody plants growing on dolomite outcrops and nearby soils during dry seasons in karst region of Southwest China. J. Hydrology 420-421, 264–274. doi:10.1016/j.jhydrol.2011.12.011
O'Grady, A. P., Carter, J. L., and Bruce, J. (2011). Can we predict groundwater discharge from terrestrial ecosystems using existing eco-hydrological concepts? Hydrol. Earth Syst. Sci. 15, 3731–3739. doi:10.5194/hess-15-3731-2011
Önöz, B., and Bayazit, M. (2012). Block bootstrap for Mann–Kendall trend test of serially dependent data. Hydrol. Process. 26, 3552–3560. doi:10.1002/hyp.8438
Páscoa, P., Gouveia, C. M., and Kurz-Besson, C. 2020. A simple method to identify potential groundwater-dependent vegetation using NDVI MODIS. Forests, 11, 147, doi:10.3390/f11020147
Patakamuri, S. K. 2021. Modifiedmk: modified versions of Mann kendall and Spearman's rho trend tests. 1.6 ed.
Peel, M. C., Finlayson, B. L., and Mcmahon, T. A. (2007). Updated world map of the Köppen-Geiger climate classification. Hydrol. Earth Syst. Sci. 11, 1633–1644. doi:10.5194/hess-11-1633-2007
Pellokila, M. R., Riwu Kaho, L. M., Nalle, A. N., Nur, M. S. M., Riwu Kaho, P. L. B., and Mella, W. I. I. (2014). “Evaluation plan for scaling up FMNR program in mainland Sumba,” in Universitas Nusa Cendana W. V. INDONESIA
QGIS DEVELOPMENT TEAM (2020). QGIS geographic information system. Open Source Geospatial Found. Proj. Available at: http://qgis.osgeo.org (Accessed June 15, 2020).
Querejeta, J. I., Estrada-Medina, H., Allen, M. F., and Jiménez-Osornio, J. J. (2007). Water source partitioning among trees growing on shallow karst soils in a seasonally dry tropical climate. Oecologia 152, 26–36. doi:10.1007/s00442-006-0629-3
R CORE TEAM (2020). R: a Language and environment for statistical computing. Vienna, Austria: R Foundation for Statistical Computing.
Rossatto, D. R., Silva, L. C. R., Sternberg, L. S. L., and Franco, A. C. (2014). Do woody and herbaceous species compete for soil water across topographic gradients? Evidence for niche partitioning in a Neotropical savanna. South Afr. J. Bot. 91, 14–18. doi:10.1016/j.sajb.2013.11.011
Roy, D. P., Kovalskyy, V., Zhang, H. K., Vermote, E. F., Yan, L., Kumar, S. S., et al. (2016). Characterization of Landsat-7 to Landsat-8 reflective wavelength and normalized difference vegetation index continuity. Remote Sens. Environ. 185, 57–70. doi:10.1016/j.rse.2015.12.024
Sankaran, M. (2019). Droughts and the ecological future of tropical savanna vegetation. J. Ecol. 107, 1531–1549. doi:10.1111/1365-2745.13195
Sarrazin, F., Hartmann, A., Pianosi, F., Rosolem, R., and Wagener, T. (2018). V2Karst V1.1: a parsimonious large-scale integrated vegetation–recharge model to simulate the impact of climate and land cover change in karst regions. Geosci. Model. Dev. 11, 4933–4964. doi:10.5194/gmd-11-4933-2018
Schwinning, S. (2008). The water relations of two evergreen tree species in a karst savanna. Oecologia 158, 373–383. doi:10.1007/s00442-008-1147-2
Schwinning, S. (2010). The ecohydrology of roots in rocks. Ecohydrology 3, 238–245. doi:10.1002/eco.134
Schymanski, S. J., Sivapalan, M., Roderick, M. L., Hutley, L. B., and Beringer, J. (2009). An optimality-based model of the dynamic feedbacks between natural vegetation and the water balance. Water Resour. Res. 45, W01412–n/a. doi:10.1029/2008wr006841
Scott, D. F., Bruijnzeel, L. A., and Mackensen, J. (2005). “The hydrological and soil impacts of forestation in the tropics,” in Forests, water and people in the humid tropics. Editors M. BONELL, and L. A. BRUIJNZEEL (Cambridge: Cambridge University Press).
Sen, P. K. (1968). Estimates of the regression coefficient based on kendall’s tau. J. Am. Stat. Assoc. 63, 1379. doi:10.2307/2285891
Seran Mau, Y. I., Ir, K. P. B., and Riwu Kaho, N. (2017). “Peta Partisipatif: perencanaan tata fungsi lahan sebagai panduan pembangunan desa,” in Waingapu, Sumba, Nusa Tenggara timur, Indonesia: wahana Visi Indonesia W. V. INDONESIA
Shinoda, M. (1995). Seasonal phase lag between rainfall and vegetation activity in tropical Africa as revealed by NOAA satellite data. Int. J. Climatol. 15, 639–656. doi:10.1002/joc.3370150605
Song, W., Feng, Y., and Wang, Z. (2022). Ecological restoration programs dominate vegetation greening in China. Sci. Total Environ. 848, 157729. doi:10.1016/j.scitotenv.2022.157729
Souza, R., Feng, X., Antonino, A., Montenegro, S., Souza, E., and Porporato, A. (2016). Vegetation response to rainfall seasonality and interannual variability in tropical dry forests. Hydrol. Process. 30, 3583–3595. doi:10.1002/hyp.10953
Sulla-Menashe, D., Friedl, M. A., and Woodcock, C. E. (2016). Sources of bias and variability in long-term Landsat time series over Canadian boreal forests. Remote Sens. Environ. 177, 206–219. doi:10.1016/j.rse.2016.02.041
Supari, H., Tangang, F., Salimun, E., Aldrian, E., Sopaheluwakan, A., and Juneng, L. (2018). ENSO modulation of seasonal rainfall and extremes in Indonesia. Clim. Dyn. 51, 2559–2580.
Swaffer, B. A., Holland, K. L., Doody, T. M., Li, C., and Hutson, J. (2014). Water use strategies of two co-occurring tree species in a semi-arid karst environment. Hydrol. Process. 28, 2003–2017. doi:10.1002/hyp.9739
Teillet, P. M., Barker, J. L., Markham, B. L., Irish, R. R., Fedosejevs, G., and Storey, J. C. (2001). Radiometric cross-calibration of the Landsat-7 ETM+ and Landsat-5 TM sensors based on tandem data sets. Remote Sens. Environ. 78, 39–54. doi:10.1016/s0034-4257(01)00248-6
Tian, S., Renzullo, L. J., Pipunic, R. C., Lerat, J., Sharples, W., and Donnelly, C. (2021). Satellite soil moisture data assimilation for improved operational continental water balance prediction. Hydrol. Earth Syst. Sci. 25, 4567–4584. doi:10.5194/hess-25-4567-2021
Tian, S., Renzullo, L., and Cai, D. (2023). Satellite-driven 10km global root-zone soil moisture analysis for drought monitoring. Zenodo. doi:10.5281/zenodo.7553986
Tian, S., Renzullo, L., van Dijk, A., Tregoning, P., and Walker, J. (2019). Global joint assimilation of GRACE and SMOS for improved estimation of root-zone soil moisture and vegetation response. Hydrology Earth Syst. Sci. 23, 1067–1081. doi:10.5194/hess-23-1067-2019
van Oldenborgh, G. J., Hendon, H., Stockdale, T., L’Heureux, M., de Perez, E. C., Singh, R., et al. (2021). Defining El Niño indices in a warming climate. Environ. Res. Lett. 16, 044003. doi:10.1088/1748-9326/abe9ed
Venter, Z. S., Scott, S. L., Desmet, P. G., and Hoffman, M. T. (2020). Application of Landsat-derived vegetation trends over South Africa: potential for monitoring land degradation and restoration. Ecol. Indic. 113, 106206. doi:10.1016/j.ecolind.2020.106206
Wallace, J. S., Young, A., and Ong, C. K. (2005). “The potential of agrofroestry for sutainable land and water management,” in Forest, water and people in the humid tropics. Editors M. BONELL, and L. A. BRUIJNZEEL (United Kingdom: Cambridge University Press).
Ward, D., Wiegand, K., and Getzin, S. (2013). Walter's two-layer hypothesis revisited: back to the roots. Oecologia 172, 617–630. doi:10.1007/s00442-012-2538-y
Wilcox, B. P., Owens, M. K., Dugas, W. A., Ueckert, D. N., and Hart, C. R. (2006). Shrubs, streamflow, and the paradox of scale. Hydrol. Process. 20, 3245–3259. doi:10.1002/hyp.6330
Wolter, K., and Timlin, M. S. (2011). El Niño/Southern Oscillation behaviour since 1871 as diagnosed in an extended multivariate ENSO index (MEI.ext). Int. J. Climatol. 31, 1074–1087. doi:10.1002/joc.2336
Wu, Z., Wu, J., Liu, J., He, B., Lei, T., and Wang, Q. (2013). Increasing terrestrial vegetation activity of ecological restoration program in the Beijing–Tianjin Sand Source Region of China. Ecol. Eng. 52, 37–50. doi:10.1016/j.ecoleng.2012.12.040
Keywords: NDVI, groundwater dependence, karst savanna, woody restoration, Indonesia
Citation: Godwin P, Tian S, Duvert C, Wurm P, Riwu Kaho N and Edwards A (2024) Detecting groundwater dependence and woody vegetation restoration with NDVI and moisture trend analyses in an Indonesian karst savanna. Front. Remote Sens. 5:1280712. doi: 10.3389/frsen.2024.1280712
Received: 21 August 2023; Accepted: 26 June 2024;
Published: 23 August 2024.
Edited by:
Wietske Bijker, University of Twente, NetherlandsCopyright © 2024 Godwin, Tian, Duvert, Wurm, Riwu Kaho and Edwards. This is an open-access article distributed under the terms of the Creative Commons Attribution License (CC BY). The use, distribution or reproduction in other forums is permitted, provided the original author(s) and the copyright owner(s) are credited and that the original publication in this journal is cited, in accordance with accepted academic practice. No use, distribution or reproduction is permitted which does not comply with these terms.
*Correspondence: Penelope Godwin, penelope.godwin@cdu.edu.au