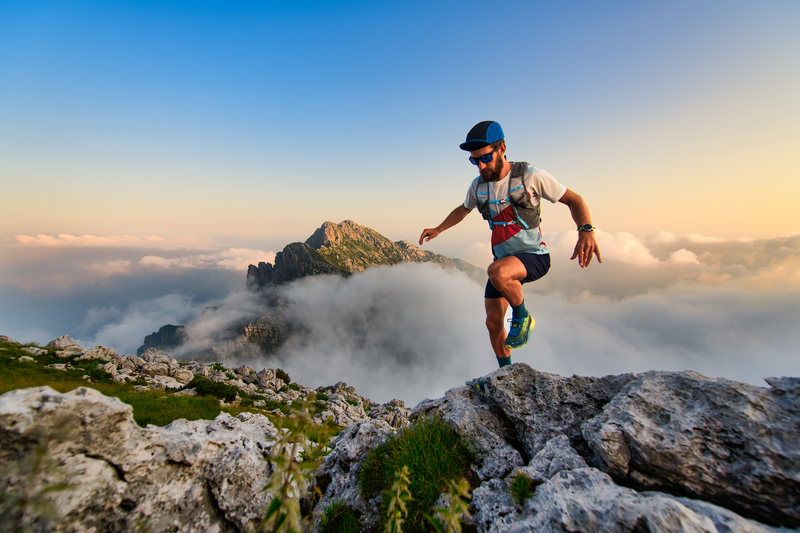
94% of researchers rate our articles as excellent or good
Learn more about the work of our research integrity team to safeguard the quality of each article we publish.
Find out more
ORIGINAL RESEARCH article
Front. Microbiomes , 18 February 2025
Sec. Host and Microbe Associations
Volume 4 - 2025 | https://doi.org/10.3389/frmbi.2025.1539923
Enteric microbiota plays a crucial role in the health and productivity of poultry, including influences on nutrient absorption, immune function, and pathogen resistance. In this study, we conducted a genome-wide association study (GWAS) to identify host genetic variants associated with selected bacterial genera found in chickens. We used high-density 600K SNP Affymetrix DNA arrays for genotyping, alongside 16S rRNA gene sequencing to profile caecal microbiota from the same individual chickens. A commercial broiler line (Cobb400, n = 300) and an indigenous (Kadaknath, n = 300) chicken breed from India were investigated, allowing for a comprehensive cross-ecotype analysis. Our analysis identified several host-genetic markers and candidate genes associated with the presence and abundance of specific bacterial genera with known pathogenic or commensal roles, and with specific caecal Enterotypes. Whole-genome sequencing data were then used to further investigate candidate regions around significantly associated variants from the high-density DNA array. Of note, we found markers nearby the genes coding for classical complement activation component C1q, ephrin receptors, and other immunity and inflammatory responses as well as genes coding for products associated with vitamin and co-factor metabolism. The results underscore the impact that host genetics has on the regulation of the gut microbiota and highlights potential pathways through which host genetic variation influences host-bacterial crosstalk and potentially modulates microbial community structure. These findings contribute to the growing understanding of the genetic basis of host-microbiota interactions and offer new avenues for improving poultry health and productivity through selective breeding strategies targeting the microbiome.
Chickens (Gallus gallus domesticus) are a cornerstone of global agriculture, serving as one of the most widely consumed sources of animal protein produced with a smaller environmental footprint over other livestock species (OECD et al., 2024). As global demand for poultry products continues to rise, maintaining the health and productivity of chickens has become increasingly vital for food security and economic stability. A key factor in ensuring the well-being of poultry is the gut microbiome, particularly the caecal microbiota, which plays a crucial role in several physiological processes that impact the overall health and productivity of the host (Sergeant et al., 2014). The chicken caeca harbour a diverse and dynamic community of microorganisms composed of trillions of bacteria, archaea, fungi, and viruses, all existing in a delicate equilibrium. The caecal microbiota is integral to digestion and absorption of nutrients, modulation of the immune responses, and therefore protection against pathogens (Pandit et al., 2018).
In chickens, the caecal microbiota is primarily involved in the fermentation of complex carbohydrates that escape digestion in the upper gastrointestinal tract. Through this fermentation process, the microbiota produces short-chain fatty acids (SCFAs) such as acetate, propionate, and butyrate, which are absorbed by the host and serve as significant sources of energy (Gilroy et al., 2021). In addition to SCFAs, the microbiota synthesises essential vitamins, including vitamin K and certain B vitamins, which are crucial for multiple metabolic functions (Sergeant et al., 2014). These microbial activities not only enhance the nutritional value of the diet but also contribute to overall health and growth performance, making the caecal microbiome a critical factor in poultry production (Dittoe et al., 2022).
Beyond its nutritional contributions, the caecal microbiota plays a pivotal role in protecting the host from infectious organisms. The microbial community acts as a barrier by competing with pathogens for nutrients and attachment sites, producing antimicrobial compounds, and modulating the host’s immune responses (Awad et al., 2009). However, the caeca are also known reservoirs for zoonotic pathogens such as Campylobacter, pathogenic Escherichia coli, Salmonella, and Helicobacter (Kaakoush et al., 2014). These pathogens can persist in the caecal environment and be transmitted to humans through the consumption or handling of contaminated poultry products, posing significant public health risks (Berry and Wells, 2016). The caecal microbiota is also a potential reservoir of antimicrobial resistance genes (ARGs) that can be transferred to pathogenic bacteria, exacerbating the challenge of controlling infections in animals and humans (Hedman et al., 2020).
The composition of the caecal microbiota is highly dynamic and is influenced by a multitude of factors, including geographic location, environmental conditions, diet and feed additives, age, and farming practices (Kers et al., 2018). Among these, diet is a particularly important determinant of microbial composition. The composition and quality of feed, including the presence of prebiotics, probiotics, other dietary supplements, and toxins, can significantly alter the microbial ecosystem within the caeca (Li et al., 2020). In addition to environmental and dietary factors, host genetics also play a critical role in shaping enteric microbial populations. Recent studies have highlighted the significant impact of host genetic makeup on the composition and function of gut microbiota (Benson et al., 2010). Genetic variations in the host can influence several aspects of microbiome structure, including the abundance of specific microbial taxa, the overall diversity of the microbial community, and the functional capabilities of the microbiota (Kurilshikov et al., 2017). These findings suggest that the microbiome is, to some extent, heritable and that selective breeding could be a potential strategy for optimising gut health in poultry and thereby overall health, welfare and productivity. Moreover, the relationship between host genetics and the gut microbiota is bidirectional. Not only do host genetics influence the microbiome, but the microbiome also affects the expression of host genes, particularly those involved in immune responses and metabolic processes (Kers et al., 2018). This intricate host-microbiome crosstalk highlights the complexity of the gut ecosystem and underscores the importance of considering both genetic and environmental factors in the management of poultry health. Understanding the genetic basis of these interactions will be valuable when developing targeted interventions that can enhance the health, productivity, and disease resistance of chickens.
In this study, we aimed to identify host genetic variation associated with selected caecal bacterial genera that are known to play either pathogenic, beneficial or commensal roles in the gut microbiome of chickens. Leveraging a comprehensive approach, we used host genotype data obtained through a genome-wide high-density DNA array, alongside microbiota data derived from 16S rRNA gene sequencing, both collected from the same individual chickens (commercial broilers, Cobb400 and indigenous chickens, Kadaknath from India). Our previous study that compared microbiome composition in two indigenous Indian breeds (Kadaknath and Aseel) and two commercial broiler lines (Cobb400 and Ross 308) demonstrated comparatively greater effects of location than breed/variety in shaping the chicken caecal microbiome (Pandit et al., 2018). Nevertheless, in closer examination of variation in microbiome structure between the commercial broilers and indigenous breeds, several key biomarkers associated with chicken varieties were identified, indicating further scope for quantitative genetic analysis. Moreover, the microbiome dataset used in this work was previously described in our other work, which explored the concept of caecal Enterotypes - a distinct state of microbiota structure within the caeca (Hay et al., 2023). Originally proposed in 2011, the concept of enterotypes arose from the finding that human gut microbiomes could be assigned to one of a small number of stable states (enterotypes) based on the relative proportions of dominant bacterial taxa (Arumugam et al., 2011). The concept was later applied to other animal and bird species including chickens (Moeller et al., 2012; Wang et al., 2014; Mach et al., 2015; Ramayo-Caldas et al., 2016; Yuan et al., 2020). Enterotypes are shaped over time by dietary intake and demonstrate resilience to temporary fluctuations in diet (Arumugam et al., 2011). In line with other studies, our previous study observed the presence of 3 distinct Enterotypes differing by the ratio of Firmicutes to Bacteroides with a decreasing ratio from Enterotype 1 to 3 (Hay et al., 2023). In the current study, we extend this concept by using Enterotype as one of the phenotypes for genome wide association studies (GWAS), thereby linking specific host genetic variants to the shaping of specific microbial community structures. Additionally, we analysed host whole-genome sequencing (WGS) data at high coverage (40 X) to delve deeper into candidate genomic loci that show strong associations with the caecal microbiota or Enterotypes in the GWAS. By focusing on the genetic determinants of caecal microbial composition, this study aims to provide new insights into the complex interactions between the host and the gut microbiome that will advance understanding of how host genetic variation influences the microbiome and exploration of the potential for selective breeding strategies that leverage these insights to improve the health and productivity of poultry.
The work was conducted with permission from the Ethical Review Panel of Anand Agricultural University (AAU) (now Kamdhenu University) and the Clinical Research Ethical Review Board (CRERB) of the Royal Veterinary College under the reference URN 2014 1280. Participating farmers were informed of the objectives of the study and written consent was obtained.
Two chicken lines, Cobb400 and Kadaknath, were selected for the study due to their distinct genetic backgrounds and production traits. Cobb400, a widely used commercial broiler in the study region, has been intensively selected for rapid growth and feed efficiency. In contrast, Kadaknath, an indigenous Indian slow growing black-boned breed, is known for disease resilience (Ramasamy et al., 2010). Samples from 600 chickens were collected from the farms/backyard production systems in Gujarat, a western state of India. The sampled chickens included 300 commercial Cobb400 broiler and 300 indigenous Kadaknath chickens. The birds were sampled from farms rearing both lines together (n = 30, 5 birds sampled of each line per farm), broiler-only (n = 15, 10 birds sampled per farm) or Kadaknath-only farms (n = 15, 10 birds sampled per farm), The birds were sacrificed between 35 and 42 days of age and blood was collected onto NucleoSave cards (Macherey-Nagel, Germany) for genomic DNA extraction. Caecal pouches were surgically removed post-mortem to collect the caecal content in Qiagen RNAprotect Bacteria reagent (1:1 ratio) for microbiome analysis. At each farm/site, detailed metadata related to location and farming/management practices was also collected to control for environmental variation in the genetic studies. The detailed description of the experimental design, including the location of sites and the metadata for each site is outlined in detail in our previous studies (Hinsu et al., 2018; Hay et al., 2023).
Total DNA was extracted from caecal content using a Qiagen DNeasy Stool Mini Kit (Qiagen, Germany) following the manufacturer’s instructions with minor modifications (Pandit et al., 2018). Briefly, 200 µl of sample was mixed with 1 ml InhibitEX buffer and incubated at 80°C for 10 minutes. The mixture was centrifuged and 600 µl of supernatant was processed as per the protocol. Around 12.5 ng DNA was used to amplify V3-V4 regions of the 16S rRNA gene using 341F and 785R primers and prepare libraries as mentioned in the Illumina 16S library preparation guide (Illumina Inc., USA). The libraries were sequenced on the Illumina MiSeq platform using 300x2 paired-end chemistry. A total of nine sequencing runs were set to accommodate all the samples.
Chicken’s genomic DNA was extracted from NucleoSave cards using the GenSolve DNA kit (GenTegra, USA) as per the manufacturer’s instructions. Genomic DNA from all 600 samples was submitted to Edinburgh Genomics to genotype using the Axiom Genome-wide Chicken Array (ThermoFisher Scientific, USA). The Axiom Genome-wide Chicken Array contains around 620,000 SNP markers (Kranis et al., 2013).
A subset of 31 chicken DNA samples (Cobb400 = 13, Kadaknath = 18) were selected based on specific genera abundance such as Campylobacter and Escherichia.Shigella to represent chickens in the extremes of the phenotypes for whole genome sequencing. Libraries were prepared using Illumina TruSeq DNA Nano kits (Illumina Inc., USA) and sequenced on the Illumina HiSeq platform using 2x150 bp chemistry to generate average 40x genome coverage per sample. The library preparation and sequencing were outsourced to Edinburgh Genomics (UK).
The 16S rRNA gene sequencing data was analysed using the DADA2 package (Callahan et al., 2016). Briefly, the data was quality filtered, denoised, paired reads were merged, and chimera-checked to generate the final Amplicon Sequence Variants (ASV) table using the default parameters as given in the DADA2 tutorial (https://benjjneb.github.io/dada2/tutorial.html). In line with DADA2 recommendations, samples from each flow cell were processed individually and then merged at a later stage before generating the ASV table. ASVs were assigned taxonomy using SILVA v132 and phylogeny was generated within QIIME2 (Quast et al., 2013; Bokulich et al., 2018; Bolyen et al., 2019). An additional step of clustering sequences with 99% similarity was performed to reduce the number of ASVs for taxonomic classification within QIIME2. The ASV table, phylogenetic tree, taxonomy information and metadata were merged to generate a phyloseq object which was then used for other downstream analysis (McMurdie and Holmes, 2013). The samples were rarefied to 10,000 reads and samples with less than 10,000 reads were removed from the analysis. The ASV table was agglomerated at genus level to obtain genera level abundance.
From the detected genera, Bacillus, Campylobacter, Cloacibacillus, Eisenbergiella, Enterococcus, Escherichia.Shigella, Helicobacter, Lactobacillus, Parasutterella and Sutterella were selected as phenotypes for GWAS. The choice of genera was made based on their involvement and role in chicken caecal microbiota (Oakley et al., 2014; Hiippala et al., 2016; Lim et al., 2017; Clavijo and Florez, 2018; Zhang et al., 2021; Derqaoui et al., 2022; Chen et al., 2023; Ge et al., 2023). For instance, Campylobacter and Helicobacter are common zoonotic pathogens from chickens, while Bacillus and Lactobacillus are key commensals/beneficial microbes for chicken.
Genera detected in 30%-60% of samples (Bacillus, Campylobacter, Cloacibacillus, Enterococcus, Escherichia-Shigella and Sutterella) were analysed as binary traits (present/absent), while those detected in >60% of samples (Eisenbergiella, Helicobacter, Lactobacillus and Parasutterella) were analysed as continuous traits in subsequent genetic analysis. The relative abundance of genera analysed as continuous traits was normalised by rank-based inverse normal transformation using the GenABEL package v1.8-0 in R (Aulchenko et al., 2007).
Additionally, the classified Enterotypes from our previous study were also used as phenotypes for GWAS (Hay et al., 2023). Enterotypes refer to the distinct clusters of enteric microbiota based on the relative abundance of different bacterial species. Enterotypes were classified by clustering the AIT distance matrix of genus-level abundance using partition around medoids (PAM) clustering algorithm. Please refer (Hay et al., 2023) for the detailed methodology of Enterotype classification.
PLINK v1.9 (https://www.cog-genomics.org/plink/) was used for quality control of the genotypic data: minor allele frequency greater than 5%, sample call rate greater than 90% and a Hardy-Weinberg equilibrium p-value less than 10-6 (flags: –maf 0.005 –geno 0.1 –hwe 0.000001) (Purcell et al., 2007). The filtered variants were used to generate genomic relationship matrix which was then used to perform Principal Component Analysis and plotted using R. SNP positions of the markers were remapped to the galGal6 (GRCg6a) chicken genome assembly using the LiftOver remapping tool (https://genome.ucsc.edu/cgi-bin/hgLiftOver). Updated SNP positions on galGal6 were used for all downstream analysis.
Additional information for each farm/site was collected in the form of detailed questionnaire. The questionnaire included detailed information covering the farm description, farm location, surrounding geographical features, farming practices and other factors potentially affecting microbiota like other animals/chickens on farms. More details are available from our previous publication (Hay et al., 2023). Multiple correspondence analyses (MCA) of bird and environmental farm variables were performed to identify key components explaining the phenotypic variance in recorded metadata using FactoMineR package in R (Lê et al., 2008). The first four MCA axes, which explained more than 70% of variation, were taken as a covariates for SNP-based heritability estimates and genome-wide association study (GWAS) analyses.
QC filtered variants were used to estimate SNP-based heritability for each selected genera and Enterotypes individually using the genomic relatedness based restricted maximum-likelihood method (GREML) implemented within GCTA v.1.94.beta (Yang et al., 2011). The genomic relationship matrix among individuals (GRM) was produced using ‘–make-grm’ flag as
in which gij represents the estimated genetic relationship between chicken i and j; xiv and xjv are the counts of the reference alleles in chicken i and chicken j, representatively; is the frequency of the reference allele in the population; and N is the total number of SNPs. SNP-based heritability, i.e. the proportion of total phenotypic variance attributed to genetic variation, was quantified using restricted maximum likelihood analysis (‘–reml’) function in a model that included the first four MCA axes and breed as covariates to account for potential environmental effects.
GWAS analyses were performed using the Genome-Wide Efficient Mixed Model Association (GEMMA) algorithm v0.98 (Zhou and Stephens, 2012) for each selected genera and Enterotype as a separate trait. GEMMA employs a linear mixed model (LMM) framework that accounts for population structure and relatedness by incorporating a genomic relationship matrix as a polygenic random effect. This approach helps control for confounding due to cryptic relatedness and population stratification, thereby reducing false-positive associations. For each GWAS, chicken breed and the first four MCA components of environmental farm categorical variables were fitted as covariates to account for potential environmental influences on microbial composition. The Wald test, implemented within GEMMA, was used to assess the significance of SNP associations. To control for multiple testing, a Bonferroni correction was applied, setting the genome-wide significance threshold at 1.19 × 10-7 (0.05/418,665) and a suggestive genome-wide, counting for one false discovery per genome scan, threshold of 2.39 × 10-6 (1/418,665). The GWAS results were visualised using Manhattan plots and quantile-quantile (Q-Q) plots produced using the rMVP R package (Yin et al., 2021).
The 50 kb upstream and downstream regions of the significantly associated SNPs from GWAS results were considered as the candidate region for further analysis. The threshold for genomic regions was determined based on the values derived from the LD decay in both breeds (see Results section). All the genes located in the candidate regions were identified using the BiomaRt R package using Galgal6a database from Ensembl v106 annotations (Durinck et al., 2009). The extracted gene list was used as the input to perform enrichment analysis of KEGG pathways using Clusterprofiler R package (Yu et al., 2012). The results were corrected using Benjamini-Hochberg correction with significance threshold set at P< 0.1 to account for the smaller number of genes.
The quality of the sequencing reads was assessed with FastQC v0.11.9 software (https://www.bioinformatics.babraham.ac.uk/projects/fastqc) and QC was performed with Trimmomatic v0.39 (Bolger et al., 2014). QC-pass data was mapped to the galGal6 (GRCg6a) chicken genome assembly using the Burrows-Wheeler Alignment tool (BWA v0.7.10) (Li and Durbin, 2009) and variant calling was performed following the best practice for the Genome Analysis Toolkit with GATK v4.1.6 (McKenna et al., 2010). The vcf file was further filtered according to hard-filter thresholds suggested within the GATK pipeline, and only bi-allelic SNPs were used for downstream analysis. Linkage disequilibrium (LD) decay from the WGS data was estimated by calculating r2 and plotted for each population using PopLDdecay with default parameters (Zhang et al., 2019).
All the variants within the candidate regions based on the GWAS results were extracted from the WGS data using bcftools v1.9 (Danecek et al., 2021). The extracted variants were annotated using VEPv110 with chicken GRC6a assembly from Ensembl genes 106 (McLaren et al., 2016). Further, the variants with a predicted ‘HIGH or ‘MODERATE’ impact were filtered for further interrogation (Vaser et al., 2016). These HIGH or MODERATE impact variants included all variants with deleterious effects predicted by SIFT. The variants were further removed if variant only had a single genotype, or less than 3 samples were observed for any genotype. The filtered variants were associated with respective phenotype/trait using linear model in R (lm() function) and p-values adjusted using Benjamini-Hochberg (BH). The phenotype/trait was taken as dependent variable, and individual genetic variants were tested as fixed effects while adjusting for the effects of covariates (the first four MCA components of environmental farm categorical variables and breed). The BH-adjusted p-value threshold of 0.05 was considered as threshold for significance of associations. The LD between all pairs of these tested variants were calculated using GWLD package in R (Zhang et al., 2023).
The study included 600 chickens: 300 Cobb400 broilers, bred for meat production and 300 Kadaknath, a black-boned indigenous Indian breed, farmed for meat and eggs. All chickens were sampled from Gujarat, the Western-most state of India. While the two varieties have different growth rates, birds were sampled at the same age range of 35-42 days to avoid age bias. Data from both chicken breeds were analysed together to identify genetic variation associated with microbiome structure and genera abundance across chicken breeds.
Each caecal microbiome was studied by sequencing the V3-V4 region of the 16S rRNA gene across nine separate Illumina sequencing runs. The data was analysed using the DADA2 pipeline, resulting in 9217 Amplicon Sequence Variants (ASVs) from 600 samples. After rarefying at 10,000 reads and removing samples failing QC in host genotype data, 6326 ASVs remained from 559 chicken samples which included 292 Cobb400 and 267 Kadaknath chickens. Agglomerated abundance per genus was considered as phenotypes for performing GWAS, which showed Bacteroides and Faecalibacterium as the dominant genera (Supplementary Figure S1). Ten genera were selected for their beneficial/commensal or pathogenic/zoonotic roles and analysed as binary or continuous traits in GWAS, depending on their prevalence across the samples (Table 1). Additionally, microbiome community state, or “Enterotype” was also used as a phenotype. In our previous study using the same set of samples, three distinct Enterotypes were defined in the caecal microbial community (Hay et al., 2023). In line with other studies, these Enterotypes differed by the ratio of Firmicutes to Bacteroides with a decreasing ratio from Enterotype 1 to 3. As Enterotypes are stable-states of community, they can provide a better measure for studying host-microbiome crosstalk compared to individual taxa abundance/prevalence. Details regarding the microbiome structure and diversity, differences between Cobb400 and Kadaknath, as well as the classification of Enterotypes, have been thoroughly covered in our previous publications (Pandit et al., 2018; Hay et al., 2023).
Table 1. Genome-wide heritability estimated for colonisation by selected bacterial genera and caecal enterotypes using GCTA-GREML.
All 600 chicken samples were genotyped with the chicken 600K single-nucleotide polymorphism (SNP) Affymetrix array (Kranis et al., 2013). After quality control with PLINK v1.9, 418,665 high-quality SNPs from 559 samples remained for further analysis. As expected, multidimensional scaling analysis (MSA), based on the SNP data, revealed population structure and showed a clear separation between the two chicken breeds (Supplementary Figure S2) (Psifidi et al., 2018).
Genetic heritability (h2) was estimated separately for each selected genus and Enterotype. Heritability for the selected genera ranged from 0 to 0.33, with 6 of the 10 genera exhibiting non-zero estimates (likelihood ratio test, P < 0.05) (Table 1). Enterococcus (h2 = 0.33) and Helicobacter (h2 = 0.27) were the genera with the highest heritability estimates from binary and continuous phenotypes, respectively. Similarly to previous studies, species of the zoonotic genus Campylobacter showed significant genetic heritability (h2 = 0.20, P < 0.01) (Psifidi et al., 2016b; Banos et al., 2020; Psifidi et al., 2021), while very low genetic heritability was observed for Escherichia.Shigella (h2 = 0.03, P = 0.21). Interestingly, for the most prevalent genus Lactobacillus (present in 92% of the samples) the heritability was close to zero. For the Enterotypes, a low genetic heritability was estimated (h2 = 0.13, P < 0.3).
Genotypes and phenotypes were compared from 559 birds using a linear mixed model with GEMMA v0.9.8 (Zhou and Stephens, 2012). The GWAS studies identified 7 significant (p < 1.19 × 10-7) and 28 suggestive significant (p < 2.39 × 10-6) genome-wide level associations between SNPs and the presence of specific gut microbial genera (Table 2; Supplementary Figures S3–S12). Genome-wide significant associations were identified for Campylobacter (GGC4: ~39.2Mb, GGC8:~26.9Mb, GGC10:~2.5Mb, GGC25:~2.6Mb), Sutterella (GGC1:~180.3Mb) and Parasutterella (GGC1:~90.6Mb) abundance on Gallus gallus Chromosomes (GGC) 1, 4, 8, 10 and 25 (Table 2). The most significant association (p = 6.28x10-13) was observed between Campylobacter abundance and a SNP located at 26,982,059 bp on GGC8, which was close to HOOK1 (Hook Microtubule Tethering Protein 1) gene. Overall, the greatest number of associations were observed for Campylobacter and Cloacibacillus (9 SNPs, each), followed by Parasutterella (5 SNPs) and Enterococcus (4 SNPs). There were no significant associations with genera Bacillus and Lactobacillus, the two most common commensals, which is in accordance with the zero heritability estimated for them. Moreover, we also identified five suggestive genome-wide associations between SNPs and caecal Enterotypes, four of which were closely located on GGC5 (Table 2; Figure 1). Two sets of associations were observed on GGC5 separated by approximately 74 kb distance. Interestingly, the candidate regions for Enterotypes included genes coding three chains of complement component 1q (i.e.C1qA, C1qB and C1qC).
Table 2. Significant SNPs identified by GWAS and candidate genes associated with colonisation by the selected bacterial genera and caecal enterotypes.
Figure 1. Manhattan plot (left) and QQplot (right) depicting -log10(p-value) from the GWAS results with caecal Enterotypes as trait.
50 kb upstream/downstream windows around the significant SNPs were considered as candidate regions to search for genes and genetic variants of interest for each of the phenotypes under investigation. The selection of 50 kb windows was based on Linkage Disequilibrium (LD) analysis as described below. Combined, all the candidate regions contained a limited number of protein-coding genes (n = 82) and non-coding RNAs (n = 33) (Supplementary Table S1). Consistent with the number of identified associations, most protein-coding genes were observed within the candidate regions for Campylobacter abundance (n = 25) followed by Cloacibacillus abundance (n = 15) and Enterotype (n = 9).
Enrichment analysis of the gene lists in the candidate regions revealed enrichment for 14 KEGG pathways (Benjamini-Hochberg (BH) adjusted p < 0.05) (Figure 2). The most significantly enriched KEGG pathway was efferocytosis (BH adjusted p = 0.0009), related to the Enterotype phenotype. Other pathways with enrichment were part of signal transduction, amino acid metabolism and metabolism of cofactors and vitamins. Specifically, thiamine metabolism (BH adjusted p = 0.035) for Enterotype; apelin signalling pathway (BH adjusted p = 0.044), tyrosine metabolism (BH adjusted p = 0.018), tryptophan metabolism (BH adjusted p = 0.018), valine, leucine and isoleucine degradation (BH adjusted p = 0.018), nicotinate and nicotinamide metabolism (BH adjusted p = 0.018), and retinol metabolism (BH adjusted p = 0.018) for Helicobacter abundance; beta-alanine metabolism (BH adjusted p = 0.0096) and pantothenate and CoA biosynthesis (BH adjusted p = 0.0096) for Escherichia.Shigella abundance; ErbB signalling pathway (BH adjusted p = 0.105) for Eisenbergiella abundance; lysine degradation (BH adjusted p = 0.079) for Cloacibacillus abundance; and alanine, aspartate and glutamate metabolism (BH adjusted p = 0.006) for Parasutterella abundance.
Figure 2. Dot plot showing enriched KEGG pathways from over enrichment analysis. The size of dots corresponds to the number of genes observed for the respective category and the dots are coloured by -log10 of the nominal p-value. * represents the categories which are also significant as per adjusted p-value < 0.05. The top facet shows results from the over enrichment with all the genes taken together.
High coverage (40x) WGS data from 31 selected chickens (Cobb400 = 13, Kadaknath = 18) was processed through the Genome Analysis Toolkit (GATK) v4.1.6 variant-calling pipeline (McKenna et al., 2010), identifying around 20 million variants. Of these, around 17 million biallelic variants were used to calculate LD decay. The LD plot showed r2 value below 0.2 at ~ 50 kb distance, wherein it started to flatten (Figure 3). The LD decay curve showed higher levels for Cobb400 compared to Kadaknath, which was consistent with the higher selection pressure on commercial broilers. Based on these results, 50 kb was considered the most appropriate window for candidate genomic regions.
Figure 3. Linkage disequilibrium (LD) decay in Cobb400 (broiler) and Kadaknath chickens calculated from the variants predicted from WGS data. Left plot shows decay in the distance less than 300 kb, right plot shows the same data in the distance 2 Mb.
To identify genetic variants of interest in these candidate regions we used the variants called from the WGS data. The variants were extracted and annotated with VEP v110 (McLaren et al., 2016) to specifically look for variants with a predicted HIGH and MODERATE impact on the encoding proteins which will include non-synonymous changes. A total of 55,269 variants spanning 108 genes were analysed from 36 regions (Table 3). As expected, most of these variants were present in intronic (45.8%), intergenic (36.2%) and untranslated regions (1.2%), while a very small proportion can be considered true non-synonymous exonic variants (0.6%) (Figure 4). HIGH impact variants (n = 5) included variants at splice-donor/acceptor sites (n = 2) or which result in stop gain (n = 2) or start loss (n = 1), while 348 variants were predicted with MODERATE impact resulting from missense variation. Moreover, 71 of these variants had deleterious effects according to SIFT score. Overall, around 1/5 of variants (n = 75) were novel (without rsID) with a predicted HIGH or MODERATE impact.
Figure 4. Pie chart representing genetic location of variants from WGS data present in GWAS candidate regions annotated with Variant Effect Predictor (VEP). The proportion represented is from 50,262 variants detected in candidate regions.
Linear regression analysis revealed that out of 282 tested variants, 149 showed significant association (F-test BH corrected p < 0.05) with the respective phenotype (Table 4). This included 87 variants associated with Helicobacter, 47 variants with Cloacibacillus, 8 variants with Enterotype, 3 variants with Sutterella, and 2 variants each with Campylobacter and Eisenbergiella. The significant associations spanned 28 genes across all phenotypes. The higher number of variants associated with Helicobacter and Cloacibacillus were observed to have high LD among each other (Supplementary Figures S13–S16) potentially corresponding to the same haplotype.
Table 4. Variants from WGS data showing significant (BH adjusted p-value < 0.05) associations with respective trait/genera.
We have investigated the relationship between host genetic variation and the abundance or presence of specific microbial genera within the chicken caecal microbiota, providing insights into host-microbiome interactions.
We observed that 6 out of 10 microbial genera exhibited non-zero heritability estimates, indicating that while some microbial genera show a measurable genetic component, others do not. This is in line with previous studies, which also demonstrated that genetic variation in abundance or occurrence of microbes is not present for all (Goodrich et al., 2016a; Feng et al., 2022). Interestingly, studies comparing human and mouse microbiomes have reported higher heritability estimates in mice, which were sampled in controlled laboratory settings (Goodrich et al., 2016b). Similar factors could impact the current study as samples were collected from natural settings such as poultry farms. Despite these limitations, heritability trends were consistent with relevant prior field studies. For instance, our study showed a significant heritability of 0.2 for Campylobacter which is comparable with previous studies (0.11 and 0.25) (Psifidi et al., 2016b; Psifidi et al., 2021), while similar levels of heritability were also observed for Escherichia and Lactobacillus (Mignon-Grasteau et al., 2015). The low heritability of some microbial taxa could also stem from the inability of current short-read sequencing technologies to reliably achieve species-level resolution (Deng et al., 2021). This limitation hinders reliable heritability estimates and complicates cross-study comparisons, as true genetic influences are obscured by methodological inconsistencies.
GWAS analysis across bird ecotypes revealed multiple novel genetic associations with the abundance of different genera. However, we didn’t observe any overlaps in candidate regions between across-ecotype (current study) GWAS and within-ecotype-GWAS (Dai et al., 2022) even though both displayed similar levels of heritability. These findings suggest that while genetic influence on microbiome composition may be consistent across ecotypes, the specific genetic architecture of such traits may vary significantly. In addition, the present study demonstrated the usefulness of joint analysis, since the bigger sample size made it possible to reveal further genetic associations common between different chicken ecotypes. This is in accordance with previous chicken genetic studies (Banos et al., 2020). While multiple associations were observed on the same chromosomes, we did not observe any overlapping candidate regions between different genera/studied traits. Campylobacter and Cloacibacillus showed the highest number (n = 9) of significant SNP-genera associations. Interestingly, Cloacibacillus exhibited low heritability (h² = 0.084), emphasising that environmental factors play a much more important role than genetic ones. Such findings align with prior microbiome GWAS studies, which frequently report significant associations despite low heritability estimates (Rothschild et al., 2018; Grieneisen et al., 2021). The significant associations with Campylobacter were distributed over GGC2-4, GGC8, GGC10, GGC11, GGC14, and GGC25. However, this study did not identify any of the previously reported associations with Campylobacter in broiler chickens and the Barred Rock breed (Connell et al., 2013; Psifidi et al., 2021). Overall, a higher number of associations were observed on GGC1 (n = 9) across multiple genera. Of note, Cloacibacillus (5 out of 9) and Parasutterella (3 out of 5) had the most significant associations on GGC1.
Host-microbiome crosstalk is a complex, bidirectional process with multiple factors influencing them including immunological/inflammatory, regulatory/hormonal, signalling and metabolic processes (Sun et al., 2017; Zheng et al., 2020; Maciel-Fiuza et al., 2023). Several markers linked with candidate genes identified in this study are implicated in immune pathways and host-microbiome interactions. For instance, genes associated with Campylobacter abundance [cytochrome P450 family2 subfamily J (CYP2J), sorting nexin 25 (SNX25), and UFM1 specific peptidase 2 (Ufsp2)] are involved in immune regulation. CYP2J genes metabolise arachidonic acid, a precursor for inflammatory mediators in several immune-related disorders (Clarke et al., 2010). SNX25 regulates TGF-β signalling, enhancing receptor degradation, and Ufsp2 suppresses responses to interferon-γ (IFN-γ) and lipopolysaccharides (LPS), key microbial interaction molecules (Balce et al., 2021; Nishimura et al., 2021). A gene associated with Eisenbergiella abundance [T-box 6 (TBX6)] modulates Notch1 signalling, which is linked to bacterial infection responses (Yasuhiko et al., 2006; Gallenstein et al., 2023). Similarly, genes associated with Cloacibacillus abundance, such as calmodulin-lysine N-methyltransferase (CAMKMT), prolyl endopeptidase-like (PREPL), and solute carrier family 3 member 1(SLC3A1), have roles in immune response modulation and were implicated in high IFN-γ levels in cattle exposed to avian tuberculin purified protein derivative (Badia-Bringue et al., 2023). Moreover, WGS data analysis revealed specific candidate variants in CAMKMT, PREPL, SLC3A1, and TBX6 genes that may be involved in genera abundance modulation.
Another noteworthy association was the potential involvement of the C1qA, C1qB, and C1qC genes in Enterotype shaping. C1q is the recognition component of the C1 complex which initiates the classical pathway of complement activation by binding with and sensing immunological complexes like antigen-antibody complex, and non-immunological components like bacterial LPS (Mellors et al., 2020). Various studies have highlighted the role of the C1q molecule against microbial infections, describing how C1q deficiency leads to increased host susceptibility to various bacterial infections, and conversely showing the over-expression of C1q genes post bacterial infection (Lu et al., 2008; Rychlik et al., 2014). Moreover, studies in humans have shown that mutations in C1q genes lead to deficiency or lower levels of C1q and are strongly associated with clinical presentation in the form of encapsulated bacterial infections and systemic lupus erythematosus (SLE) (van Schaarenburg et al., 2016). The significant association in the current study was observed to be downstream of the C1qA gene and we observed 3 novel variants in WGS analysis (GGC21:6,073,737; GGC21:6,073,941; and GGC21:6,074,132) in C1qB gene showing significant association with Enterotype. All these taken together point towards potential interactions among gut microbiome members, components of the host complement system, and efferocytosis mechanisms, the outcome of which could shape the overall microbiota structure in the caeca. Efferocytosis is a process by which phagocytic cells remove dead and apoptotic cells, and has been previously linked with the inflammatory response and inflammatory disorders (Ge et al., 2022). Previous studies have reported that the gut microbiome, through its diverse array of metabolites, exerts a regulatory influence on efferocytosis in peripheral tissues, facilitating immune resolution and tissue repair while maintaining systemic homeostasis, as evidenced by its impact on macrophage polarisation and apoptotic cell clearance in inflammatory contexts (Li et al., 2021; Saavedra et al., 2022; Gorreja et al., 2023; Traughber et al., 2024).
The candidate region for Enterotype association also harboured two Ephrin (Eph) receptor genes (EPHA8 and EPHB2), while in the candidate region for Parasutterella abundance another Ephrin receptor gene EPHA3 was located. Eph receptors are receptor tyrosinase kinases that are involved in transducing extracellular signals inside the cells through ligand-induced activation (Lisabeth et al., 2013). Several EPH receptors and their ligands are also involved in immunological processes. For instance, EPHB2 mutations and expression have been linked with colorectal cancer, while EPHA2 and EPHA3 are shown to have roles as viral entry receptors for herpesvirus and Epstein-Barr virus (Genander et al., 2009; Darling and Lamb, 2019). Other studies also report that Eph receptors are manipulated by bacteria for immune evasion, while other studies have described their involvement in the activation of immune cells (Funk and Orr, 2013; Yu et al., 2014). Moreover, a significant association (GGC21:6,119,830) from WGS analysis with Enterotype trait further underscores the importance of these genes in host-microbiome interactions. However, further studies are needed to confirm this speculation and establish detailed interactions and mechanisms.
The current study also identified several other genes in the candidate regions of association which code for proteins found in the extracellular matrix (ECM) that interact with membrane receptors. Gut bacteria engage in intricate interactions with the ECM and produce a variety of enzymes and metabolites that can degrade ECM components, influencing tissue architecture and permeability (Derkacz et al., 2021). ECM components can serve as signalling molecules that affect bacterial behaviour, including biofilm formation and virulence. The ability of gut microbes to interact with the ECM also highlights the bidirectional communication between host tissues and the microbiota, which is crucial for maintaining gut homeostasis (Franchi et al., 2024). Notably, BCAN (brevican, associated with Campylobacter) and HAPLN2 (hyaluronan and proteoglycan link protein 2, associated with Campylobacter) proteins involved in the binding of hyaluronic acid; G-protein coupled receptors GRM7 (glutamate metabotropic receptor 7, associated with Enterococcus abundance) and TACR3 (tachykinin receptor 3, associated with Campylobacter); glutamate receptor GRIN2A (associated with Eisenbergiella); heat-shock protein binding genes CHORDC1 (cysteine and histidine rich domain containing 1, associated with Parasutterella abundance), DNAJB6 (DnaJ heat shock protein family (Hsp40) member B6, associated with Campylobacter abundance), and LOC425431 (dnaJ homolog subfamily A member 1-like, associated with Campylobacter abundance) were observed in the candidate regions. Of these, many genes have been previously shown to interact with bacteria and viruses in the gut. For instance, the GRM7 gene was identified as a candidate gene in GWAS with antibody titres for Salmonella enterica serovar Gallinarum in chicken (Psifidi et al., 2016a). The CHORDC1 gene was observed to be upregulated post-infection from Reovirus, Hepatitis B virus, and Newcastle disease virus in chickens (Walugembe et al., 2020; Fogel et al., 2021). Transcription of the gene DNAJB6 was observed to be significantly upregulated by porcine circovirus type 2 infection in porcine cell lines (Han et al., 2020) and also observed to be significantly overexpressed in chicken cell lines after treatment with Salmonella enterica serovar Typhimurium derived lipopolysaccharide (Slawinska et al., 2016).
The current study identified associations between bacterial abundance or occurrence and genes involved in the metabolism of Retinol (Vitamin A1), Thiamine (Vitamin B1), Vitamin B6, Nicotinamide (Vitamin B3) and Folate. These micronutrients serve as essential cofactors for microbial metabolic pathways, influencing bacterial growth and survival. For instance, B vitamins, particularly B12 and folate, are critical for microbial metabolism and have been shown to impact the microbiota (Guetterman et al., 2022), while Vitamin A, through its active form, retinoic acid, affects gut immune responses, which in turn modulates the microbial environment (Pham et al., 2021). In our study, the AOX1 (aldehyde oxidase 1) gene was observed in the candidate region for Helicobacter association. AOX1 is involved in the oxidation of 9-cis and all-trans retinal (active form of Vitamin A) into the corresponding retinoic acid; conversion of 1-Methylnicotinamide (a primary metabolite of nicotinamide and shown to have roles in immune modulation of T cells in cancer) to 1-methyl-2-pyridone-5-carboxamide or 1-methyl-4-pyridone-5-carboxamide; and oxidation of Pyridoxal (the vitamin B6 precursor) to 4-pyridoxic acid (Terao et al., 2016; Kilgour et al., 2021). Another gene, AK7 (adenylate kinase 7) (associated with Enterotype), is involved in the conversion of thiamine diphosphate, the most abundant physiological form of thiamine and vital coenzyme for enzymatic reactions, to thiamine triphosphate (Bettendorff, 2021). Both in AK7 and AOX1 we identified variants significantly associated with Enterotype and Helicobacter, respectively. FOLH1 (folate hydrolase 1) and NAALAD2 (N-acetylated alpha-linked acidic dipeptidase 2), which are involved in cellular transport and absorption specifically related to folate metabolism, were also observed to be associated with Parasutterella (belonging to proteobacterial phylum). Several Proteobacteria organisms in the gut are known to modulate folate bioavailability and in turn regulate the host expression of receptors (Rais et al., 2016; Engevik et al., 2019).
Interplay between host genetics and gut microbiota reflects a co-evolutionary process in which hosts and microbes have adapted to one another over time. While certain genetic variants are clearly associated with alterations in the gut microbiome, the precise mechanisms remain an area of active investigation, tackled by many across humans and animals/birds studies (Spor et al., 2011; Bonder et al., 2016; Kurilshikov et al., 2017). Genetic variation, particularly in immune-related genes, play a pivotal role in shaping the gut environment by promoting the growth of specific microbial communities while inhibiting others. However, the microbiome is highly breed-specific, as genetic differences may influence gut morphology, immune function, and metabolic processes, leading to distinct microbial compositions between breeds (Pandit et al., 2018; Cao et al., 2020; Paul et al., 2021; Davies et al., 2022). Additionally, environmental factors such as diet significantly influence the gut microbiota, making more challenging to dissect the genetic contribution (Spor et al., 2011). While birds were sampled from farms that provided standard poultry feed, diet-related microbiome variations can still arise due to differences in feed formulation, storage conditions, and microbial contamination (Benson et al., 2010). Moreover, geographical location further contributes to microbiota diversity, as climate, humidity, and altitude affect microbial colonisation and gut health (Kers et al., 2018). To reduce the noise, the farms sampled in this study were selected from a single state of India, thereby restricting to smaller geographic area. The selected farms were following similar managemental practices, and half of the selected farms reared both chicken lines simultaneously reducing the environmental noise. Moreover, we have incorporating environmental and farming variables into our statistical models, a strategy supported by previous research to reduce environmental noise in the analysis (Awany et al., 2018). Additionally, the use of high-dimensional genotyping arrays, while providing broad coverage, may still miss rare or structural variants contributing to microbiota variation, a limitation highlighted in GWAS studies on complex traits (Awany et al., 2018). Nevertheless, the interaction between the identified SNP-containing genes and symbiotic microorganisms provides crucial insights into host-microbiome relationships that can support breeding and selection strategies. Understanding how specific genetic variants modulate microbial composition and function can enable targeted breeding approaches that enhance beneficial microbial colonisation, indirectly improving disease resistance, nutrient utilisation, overall growth performance and reducing zoonotic burden. Future research integrating whole-genome sequencing and multi-omics approaches will be essential for a more comprehensive understanding of host-microbiota genetic interactions. By integrating microbiome-informed selection into genetic improvement programs, poultry breeding can move toward optimising gut health alongside other important health, production and sustainability traits.
This study identifies candidate genes that provide a foundation for further exploration into the intricate interactions between host genetic polymorphisms and the chicken caecal microbiome. These findings offer valuable insights into how specific host genetic variants may modulate microbial composition and subsequently impact health outcomes. However, given the complex interplay between genetic and environmental factors including diet, these results must be interpreted cautiously and validated in larger populations under controlled conditions. Future studies integrating functional genomics, transcriptomics, and metabolomics will help establish causal relationships between host and the gut microbiota. Additionally, exploring host genetic-microbe interactions in multiple chicken breeds and production systems will help refine microbiome-based selection strategies and ensure their broader applicability in poultry breeding programs.
The datasets presented in this study can be found in online repositories. The 16S rRNA gene sequence data is available from EBI-ENA (https://www.ebi.ac.uk/ena/browser/search) under Project ID PRJEB15343, SRA ID ERP017060.
The animal studies were approved by Ethical Review Panel of Anand Agricultural University (AAU) (now Kamdhenu University) and the Clinical Research Ethical Review Board (CRERB) of the Royal Veterinary College. The studies were conducted in accordance with the local legislation and institutional requirements. Written informed consent was obtained from the owners for the participation of their animals in this study.
AH: Data curation, Formal analysis, Investigation, Methodology, Software, Visualization, Writing – original draft, Writing – review & editing. XD: Data curation, Formal analysis, Investigation, Methodology, Software, Visualization, Writing – original draft, Writing – review & editing. CD: Methodology, Software, Supervision, Writing – review & editing. MH: Formal analysis, Investigation, Software, Writing – review & editing. BF: Formal analysis, Software, Writing – review & editing. MC: Formal analysis, Writing – review & editing. RP: Formal analysis, Investigation, Writing – review & editing. JG: Funding acquisition, Writing – review & editing. FT: Funding acquisition, Project administration, Writing – review & editing. PK: Funding acquisition, Investigation, Resources, Writing – review & editing. CJ: Funding acquisition, Resources, Writing – review & editing. DB: Conceptualization, Funding acquisition, Project administration, Resources, Supervision, Writing – review & editing. AP: Conceptualization, Funding acquisition, Project administration, Resources, Supervision, Writing – review & editing.
The author(s) declare financial support was received for the research, authorship, and/or publication of this article. The research work was funded by the Department of Biotechnology (DBT, reference BT/IN/Indo-UK/FADH/49/CGJ/2013) India, the Biotechnology and Biological Sciences Research Council (BBSRC, reference BB/L00478X/1) UK and the UKRI Global Challenges Research Fund (Grant No. BB/S011269/1, the One Health Poultry Hub) UK. The funding agencies had no role in sample collection, conducting experiments, data analysis or manuscript writing.
The authors are thankful to all the farmers for their permission and support with the sample collection. The authors would like to acknowledge the efforts of other research staff (Subhash Jakhesara, Anand Patel, Mithil Parekh, Shriram Patel, Khushal Patel and Jalpa Thakkar) in their assistance with the sample collection.
The authors declare that the research was conducted in the absence of any commercial or financial relationships that could be construed as a potential conflict of interest.
The author(s) DB, AP, XD declared that they were an editorial board member of Frontiers, at the time of submission. This had no impact on the peer review process and the final decision.
The author(s) declare that no Generative AI was used in the creation of this manuscript.
All claims expressed in this article are solely those of the authors and do not necessarily represent those of their affiliated organizations, or those of the publisher, the editors and the reviewers. Any product that may be evaluated in this article, or claim that may be made by its manufacturer, is not guaranteed or endorsed by the publisher.
MC is now employed by the European Food Safety Authority (EFSA). However, the present article is published under the sole responsibility of the authors and may not be considered as an EFSA scientific output. The positions and opinions presented in this article are those of the authors alone and do not necessarily represent the views/any official position or scientific works of EFSA. To know about the views or scientific outputs of EFSA, please consult its website www.efsa.europa.eu.
The Supplementary Material for this article can be found online at: https://www.frontiersin.org/articles/10.3389/frmbi.2025.1539923/full#supplementary-material
Arumugam M., Raes J., Pelletier E., Le Paslier D., Yamada T., Mende D. R., et al. (2011). Enterotypes of the human gut microbiome. Nature 473, 174–180. doi: 10.1038/nature09944
Aulchenko Y. S., Ripke S., Isaacs A., van Duijn C. M. (2007). GenABEL: an R library for genome-wide association analysis. Bioinformatics 23, 1294–1296. doi: 10.1093/bioinformatics/btm108
Awad W. A., Ghareeb K., Abdel-Raheem S., Bohm J. (2009). Effects of dietary inclusion of probiotic and synbiotic on growth performance, organ weights, and intestinal histomorphology of broiler chickens. Poult Sci. 88, 49–56. doi: 10.3382/ps.2008-00244
Awany D., Allali I., Dalvie S., Hemmings S., Mwaikono K. S., Thomford N. E., et al. (2018). Host and microbiome genome-wide association studies: current state and challenges. Front. Genet. 9, 637. doi: 10.3389/fgene.2018.00637
Badia-Bringue G., Canive M., Vazquez P., Garrido J. M., Fernandez A., Juste R. A., et al. (2023). Association between High Interferon-Gamma Production in Avian Tuberculin-Stimulated Blood from Mycobacterium avium subsp. paratuberculosis-Infected Cattle and Candidate Genes Implicated in Necroptosis. Microorganisms 11, 1817. doi: 10.3390/microorganisms11071817
Balce D. R., Wang Y. T., McAllaster M. R., Dunlap B. F., Orvedahl A., Hykes B. L. Jr., et al. (2021). UFMylation inhibits the proinflammatory capacity of interferon-gamma-activated macrophages. Proc. Natl. Acad. Sci. U.S.A. 118, e2011763118. doi: 10.1073/pnas.2011763118
Banos G., Lindsay V., Desta T. T., Bettridge J., Sanchez-Molano E., Vallejo-Trujillo A., et al. (2020). Integrating genetic and genomic analyses of combined health data across ecotypes to improve disease resistance in indigenous african chickens. Front. Genet. 11, 543890. doi: 10.3389/fgene.2020.543890
Benson A. K., Kelly S. A., Legge R., Ma F., Low S. J., Kim J., et al. (2010). Individuality in gut microbiota composition is a complex polygenic trait shaped by multiple environmental and host genetic factors. Proc. Natl. Acad. Sci. U S A. 107, 18933–18938. doi: 10.1073/pnas.1007028107
Berry E. D., Wells J. E. (2016). Reducing foodborne pathogen persistence and transmission in animal production environments: challenges and opportunities. Microbiol. Spectr. 4. doi: 10.1128/microbiolspec.PFS-0006-2014
Bettendorff L. (2021). Update on thiamine triphosphorylated derivatives and metabolizing enzymatic complexes. Biomolecules 11, 1645. doi: 10.3390/biom11111645
Bokulich N. A., Kaehler B. D., Rideout J. R., Dillon M., Bolyen E., Knight R., et al. (2018). Optimizing taxonomic classification of marker-gene amplicon sequences with QIIME 2’s q2-feature-classifier plugin. Microbiome 6, 90. doi: 10.1186/s40168-018-0470-z
Bolger A. M., Lohse M., Usadel B. (2014). Trimmomatic: a flexible trimmer for Illumina sequence data. Bioinformatics 30, 2114–2120. doi: 10.1093/bioinformatics/btu170
Bolyen E., Rideout J. R., Dillon M. R., Bokulich N. A., Abnet C. C., Al-Ghalith G. A., et al. (2019). Reproducible, interactive, scalable and extensible microbiome data science using QIIME 2. Nat. Biotechnol. 37, 852–857. doi: 10.1038/s41587-019-0209-9
Bonder M. J., Kurilshikov A., Tigchelaar E. F., Mujagic Z., Imhann F., Vila A. V., et al. (2016). The effect of host genetics on the gut microbiome. Nat. Genet. 48, 1407–1412. doi: 10.1038/ng.3663
Callahan B. J., McMurdie P. J., Rosen M. J., Han A. W., Johnson A. J., Holmes S. P. (2016). DADA2: High-resolution sample inference from Illumina amplicon data. Nat. Methods 13, 581–583. doi: 10.1038/nmeth.3869
Cao J., Hu Y., Liu F., Wang Y., Bi Y., Lv N., et al. (2020). Metagenomic analysis reveals the microbiome and resistome in migratory birds. Microbiome 8, 26. doi: 10.1186/s40168-019-0781-8
Chen Y., Akhtar M., Ma Z., Hu T., Liu Q., Pan H., et al. (2023). Chicken caecal microbiota reduces abdominal fat deposition by regulating fat metabolism. NPJ Biofilms Microbiomes. 9, 28. doi: 10.1038/s41522-023-00390-8
Clarke G., Fitzgerald P., Hennessy A. A., Cassidy E. M., Quigley E. M., Ross P., et al. (2010). Marked elevations in pro-inflammatory polyunsaturated fatty acid metabolites in females with irritable bowel syndrome. J. Lipid Res. 51, 1186–1192. doi: 10.1194/jlr.P000695
Clavijo V., Florez M. J. V. (2018). The gastrointestinal microbiome and its association with the control of pathogens in broiler chicken production: A review. Poult Sci. 97, 1006–1021. doi: 10.3382/ps/pex359
Connell S., Meade K. G., Allan B., Lloyd A. T., Downing T., O’Farrelly C., et al. (2013). Genome-wide association analysis of avian resistance to Campylobacter jejuni colonization identifies risk locus spanning the CDH13 gene. G3 (Bethesda). 3, 881–890. doi: 10.1534/g3.113.006031
Dai X., Hinsu A. T., Dadousis C., Pandit R. J., Crotta M., Limon G., et al. (2022). “Genome-wide association studies of chicken caecal microbiota,” in 12th World Congress on Genetics Applied to Livestock Production (WCGALP) (Wageningen Academic Publishers, Rotterdam (The Netherlands).
Danecek P., Bonfield J. K., Liddle J., Marshall J., Ohan V., Pollard M. O., et al. (2021). Twelve years of SAMtools and BCFtools. Twelve years SAMtools BCFtools. Gigascience 10, giab008. doi: 10.1093/gigascience/giab008
Darling T. K., Lamb T. J. (2019). Emerging roles for eph receptors and ephrin ligands in immunity. Front. Immunol. 10, 1473. doi: 10.3389/fimmu.2019.01473
Davies C. S., Worsley S. F., Maher K. H., Komdeur J., Burke T., Dugdale H. L., et al. (2022). Immunogenetic variation shapes the gut microbiome in a natural vertebrate population. Microbiome 10, 41. doi: 10.1186/s40168-022-01233-y
Deng S., Caddell D. F., Xu G., Dahlen L., Washington L., Yang J., et al. (2021). Genome wide association study reveals plant loci controlling heritability of the rhizosphere microbiome. ISME J. 15, 3181–3194. doi: 10.1038/s41396-021-00993-z
Derkacz A., Olczyk P., Olczyk K., Komosinska-Vassev K. (2021). The role of extracellular matrix components in inflammatory bowel diseases. J. Clin. Med. 10, 1122. doi: 10.3390/jcm10051122
Derqaoui S., Oukessou M., Attrassi K., Elftouhy F. Z., Nassik S. (2022). Detection of sutterella spp. in broiler liver and breast. Front. Vet. Sci. 9, 859902. doi: 10.3389/fvets.2022.859902
Dittoe D. K., Olson E. G., Ricke S. C. (2022). Impact of the gastrointestinal microbiome and fermentation metabolites on broiler performance. Poult Sci. 101, 101786. doi: 10.1016/j.psj.2022.101786
Durinck S., Spellman P. T., Birney E., Huber W. (2009). Mapping identifiers for the integration of genomic datasets with the R/Bioconductor package biomaRt. Nat. Protoc. 4, 1184–1191. doi: 10.1038/nprot.2009.97
Engevik M. A., Morra C. N., Roth D., Engevik K., Spinler J. K., Devaraj S., et al. (2019). Microbial metabolic capacity for intestinal folate production and modulation of host folate receptors. Front. Microbiol. 10, 2305. doi: 10.3389/fmicb.2019.02305
Feng Y., Liu D., Liu Y., Yang X., Zhang M., Wei F., et al. (2022). Host-genotype-dependent caecal microbes are linked to breast muscle metabolites in Chinese chickens. iScience 25, 104469. doi: 10.1016/j.isci.2022.104469
Fogel E. J., Samouha A., Goel S., Maitra R. (2021). Transcriptome signature of immune cells post reovirus treatment in KRAS mutated colorectal cancer. Cancer Manag Res. 13, 6743–6754. doi: 10.2147/CMAR.S324203
Franchi M., Piperigkou Z., Mastronikolis N. S., Karamanos N. (2024). Extracellular matrix biomechanical roles and adaptation in health and disease. FEBS J. 291, 430–440. doi: 10.1111/febs.v291.3
Funk S. D., Orr A. W. (2013). Ephs and ephrins resurface in inflammation, immunity, and atherosclerosis. Pharmacol. Res. 67, 42–52. doi: 10.1016/j.phrs.2012.10.008
Gallenstein N., Tichy L., Weigand M. A., Schenz J. (2023). Notch signalling in acute inflammation and sepsis. Int. J. Mol. Sci. 24, 3458. doi: 10.3390/ijms24043458
Ge C., Luo X., Wu L., Lv Y., Hu Z., Yu D., et al. (2023). Plant essential oils improve growth performance by increasing antioxidative capacity, enhancing intestinal barrier function, and modulating gut microbiota in Muscovy ducks. Poult Sci. 102, 102813. doi: 10.1016/j.psj.2023.102813
Ge Y., Huang M., Yao Y. M. (2022). Efferocytosis and its role in inflammatory disorders. Front. Cell Dev. Biol. 10, 839248. doi: 10.3389/fcell.2022.839248
Genander M., Halford M. M., Xu N. J., Eriksson M., Yu Z., Qiu Z., et al. (2009). Dissociation of EphB2 signalling pathways mediating progenitor cell proliferation and tumour suppression. Cell 139, 679–692. doi: 10.1016/j.cell.2009.08.048
Gilroy R., Ravi A., Getino M., Pursley I., Horton D. L., Alikhan N. F., et al. (2021). Extensive microbial diversity within the chicken gut microbiome revealed by metagenomics and culture. PeerJ 9, e10941. doi: 10.7717/peerj.10941
Goodrich J. K., Davenport E. R., Beaumont M., Jackson M. A., Knight R., Ober C., et al. (2016a). Genetic determinants of the gut microbiome in UK twins. Cell Host Microbe 19, 731–743. doi: 10.1016/j.chom.2016.04.017
Goodrich J. K., Davenport E. R., Waters J. L., Clark A. G., Ley R. E. (2016b). Cross-species comparisons of host genetic associations with the microbiome. Science 352, 532–535. doi: 10.1126/science.aad9379
Gorreja F., Bendix M., Rush S. T. A., Maasfeh L., Savolainen O., Dige A., et al. (2023). Faecal supernatants from patients with crohn’s disease induce inflammatory alterations in M2 macrophages and fibroblasts. Cells 13, 60. doi: 10.3390/cells13010060
Grieneisen L., Dasari M., Gould T. J., Bjork J. R., Grenier J. C., Yotova V., et al. (2021). Gut microbiome heritability is nearly universal but environmentally contingent. Science 373, 181–186. doi: 10.1126/science.aba5483
Guetterman H. M., Huey S. L., Knight R., Fox A. M., Mehta S., Finkelstein J. L. (2022). Vitamin B-12 and the gastrointestinal microbiome: A systematic review. Adv. Nutr. 13, 530–558. doi: 10.1093/advances/nmab123
Han C., Du Q., Zhu L., Chen N., Luo L., Chen Q., et al. (2020). Porcine DNAJB6 promotes PCV2 replication via enhancing the formation of autophagy in host cells. Vet. Res. 51, 61. doi: 10.1186/s13567-020-00783-z
Hay M. C., Hinsu A. T., Koringa P. G., Pandit R. J., Liu P.-Y., Parekh M. J., et al. (2023). Chicken caecal enterotypes in indigenous Kadaknath and commercial Cobb chicken lines are associated with Campylobacter abundance and influenced by farming practices. Front. Microbiomes 2. doi: 10.3389/frmbi.2023.1301609
Hedman H. D., Vasco K. A., Zhang L. (2020). A review of antimicrobial resistance in poultry farming within low-resource settings. Anim. (Basel) 10, 1264. doi: 10.3390/ani10081264
Hiippala K., Kainulainen V., Kalliomaki M., Arkkila P., Satokari R. (2016). Mucosal prevalence and interactions with the epithelium indicate commensalism of sutterella spp. Front. Microbiol. 7, 1706. doi: 10.3389/fmicb.2016.01706
Hinsu A. T., Thakkar J. R., Koringa P. G., Vrba V., Jakhesara S. J., Psifidi A., et al. (2018). Illumina next generation sequencing for the analysis of eimeria populations in commercial broilers and indigenous chickens. Front. Vet. Sci. 5, 176. doi: 10.3389/fvets.2018.00176
Kaakoush N. O., Sodhi N., Chenu J. W., Cox J. M., Riordan S. M., Mitchell H. M. (2014). The interplay between Campylobacter and Helicobacter species and other gastrointestinal microbiota of commercial broiler chickens. Gut Pathog. 6, 18. doi: 10.1186/1757-4749-6-18
Kers J. G., Velkers F. C., Fischer E. A. J., Hermes G. D. A., Stegeman J. A., Smidt H. (2018). Host and environmental factors affecting the intestinal microbiota in chickens. Front. Microbiol. 9, 235. doi: 10.3389/fmicb.2018.00235
Kilgour M. K., MacPherson S., Zacharias L. G., Ellis A. E., Sheldon R. D., Liu E. Y., et al. (2021). 1-Methylnicotinamide is an immune regulatory metabolite in human ovarian cancer. Sci. Adv. 7, eabe1174. doi: 10.1126/sciadv.abe1174
Kranis A., Gheyas A. A., Boschiero C., Turner F., Yu L., Smith S., et al. (2013). Development of a high density 600K SNP genotyping array for chicken. BMC Genomics 14, 59. doi: 10.1186/1471-2164-14-59
Kurilshikov A., Wijmenga C., Fu J., Zhernakova A. (2017). Host genetics and gut microbiome: challenges and perspectives. Trends Immunol. 38, 633–647. doi: 10.1016/j.it.2017.06.003
Lê S., Josse J., Husson F. (2008). FactoMineR: an R package for multivariate analysis. J. Stat. Software 25, 1–18. doi: 10.18637/jss.v025.i01
Li H., Durbin R. (2009). Fast and accurate short read alignment with Burrows-Wheeler transform. Bioinformatics 25, 1754–1760. doi: 10.1093/bioinformatics/btp324
Li W., Zhang X., He Z., Chen Y., Li Z., Meng T., et al. (2020). In vitro and in vivo antioxidant activity of eucalyptus leaf polyphenols extract and its effect on chicken meat quality and cecum microbiota. Food Res. Int. 136, 109302. doi: 10.1016/j.foodres.2020.109302
Li Y., Zhang S. X., Yin X. F., Zhang M. X., Qiao J., Xin X. H., et al. (2021). The gut microbiota and its relevance to peripheral lymphocyte subpopulations and cytokines in patients with rheumatoid arthritis. J. Immunol. Res. 2021, 6665563. doi: 10.1155/2021/6665563
Lim M. Y., You H. J., Yoon H. S., Kwon B., Lee J. Y., Lee S., et al. (2017). The effect of heritability and host genetics on the gut microbiota and metabolic syndrome. Gut 66, 1031–1038. doi: 10.1136/gutjnl-2015-311326
Lisabeth E. M., Falivelli G., Pasquale E. B. (2013). Eph receptor signalling and ephrins. Cold Spring Harb. Perspect. Biol. 5, a009159. doi: 10.1101/cshperspect.a009159
Lu J. H., Teh B. K., Wang L., Wang Y. N., Tan Y. S., Lai M. C., et al. (2008). The classical and regulatory functions of C1q in immunity and autoimmunity. Cell Mol. Immunol. 5, 9–21. doi: 10.1038/cmi.2008.2
Mach N., Berri M., Estelle J., Levenez F., Lemonnier G., Denis C., et al. (2015). Early-life establishment of the swine gut microbiome and impact on host phenotypes. Environ. Microbiol. Rep. 7, 554–569. doi: 10.1111/emi4.2015.7.issue-3
Maciel-Fiuza M. F., Muller G. C., Campos D. M. S., do Socorro Silva Costa P., Peruzzo J., Bonamigo R. R., et al. (2023). Role of gut microbiota in infectious and inflammatory diseases. Front. Microbiol. 14, 1098386. doi: 10.3389/fmicb.2023.1098386
McKenna A., Hanna M., Banks E., Sivachenko A., Cibulskis K., Kernytsky A., et al. (2010). The Genome Analysis Toolkit: a MapReduce framework for analysing next-generation DNA sequencing data. Genome Res. 20, 1297–1303. doi: 10.1101/gr.107524.110
McLaren W., Gil L., Hunt S. E., Riat H. S., Ritchie G. R., Thormann A., et al. (2016). The ensembl variant effect predictor. Genome Biol. 17, 122. doi: 10.1186/s13059-016-0974-4
McMurdie P. J., Holmes S. (2013). phyloseq: an R package for reproducible interactive analysis and graphics of microbiome census data. PloS One 8, e61217. doi: 10.1371/journal.pone.0061217
Mellors J., Tipton T., Longet S., Carroll M. (2020). Viral evasion of the complement system and its importance for vaccines and therapeutics. Front. Immunol. 11, 1450. doi: 10.3389/fimmu.2020.01450
Mignon-Grasteau S., Narcy A., Rideau N., Chantry-Darmon C., Boscher M. Y., Sellier N., et al. (2015). Impact of selection for digestive efficiency on microbiota composition in the chicken. PloS One 10, e0135488. doi: 10.1371/journal.pone.0135488
Moeller A. H., Degnan P. H., Pusey A. E., Wilson M. L., Hahn B. H., Ochman H. (2012). Chimpanzees and humans harbour compositionally similar gut enterotypes. Nat. Commun. 3, 1179. doi: 10.1038/ncomms2159
Nishimura K., Tanaka T., Takemura S., Tatsumi K., Wanaka A. (2021). SNX25 regulates proinflammatory cytokine expression via the NF-kappaB signal in macrophages. PloS One 16, e0247840. doi: 10.1371/journal.pone.0247840
Oakley B. B., Lillehoj H. S., Kogut M. H., Kim W. K., Maurer J. J., Pedroso A., et al. (2014). The chicken gastrointestinal microbiome. FEMS Microbiol. Lett. 360, 100–112. doi: 10.1111/fml.2014.360.issue-2
OECD/FAO. (2024). OECD-FAO Agricultural Outlook 2024-2033. Paris/FAO, Rome: OECD Publishing. doi: 10.1787/4c5d2cfb-en
Pandit R. J., Hinsu A. T., Patel N. V., Koringa P. G., Jakhesara S. J., Thakkar J. R., et al. (2018). Microbial diversity and community composition of caecal microbiota in commercial and indigenous Indian chickens determined using 16s rDNA amplicon sequencing. Microbiome 6, 115. doi: 10.1186/s40168-018-0501-9
Paul S. S., Chatterjee R. N., Raju M., Prakash B., Rama Rao S. V., Yadav S. P., et al. (2021). Gut Microbial Composition Differs Extensively among Indian Native Chicken Breeds Originated in Different Geographical Locations and a Commercial Broiler Line, but Breed-Specific, as Well as Across-Breed Core Microbiomes, Are Found. Microorganisms 9, 391. doi: 10.3390/microorganisms9020391
Pham V. T., Dold S., Rehman A., Bird J. K., Steinert R. E. (2021). Vitamins, the gut microbiome and gastrointestinal health in humans. Nutr. Res. 95, 35–53. doi: 10.1016/j.nutres.2021.09.001
Psifidi A., Banos G., Matika O., Desta T. T., Bettridge J., Hume D. A., et al. (2016a). Genome-wide association studies of immune, disease and production traits in indigenous chicken ecotypes. Genet. Sel Evol. 48, 74. doi: 10.1186/s12711-016-0252-7
Psifidi A., Crotta M., Pandit R., Fosso B., Koringa P., Limon G., et al. (2018). “Identification of SNP markers affecting gut microbiome composition in chicken,” in Proceedings of the world congress on genetics applied to livestock production. (New Zealand: WCGALP Archive).
Psifidi A., Fife M., Howell J., Matika O., van Diemen P. M., Kuo R., et al. (2016b). The genomic architecture of resistance to Campylobacter jejuni intestinal colonisation in chickens. BMC Genomics 17, 293. doi: 10.1186/s12864-016-2612-7
Psifidi A., Kranis A., Rothwell L. M., Bremner A., Russell K., Robledo D., et al. (2021). Quantitative trait loci and transcriptome signatures associated with avian heritable resistance to Campylobacter. Sci. Rep. 11, 1623. doi: 10.1038/s41598-020-79005-7
Purcell S., Neale B., Todd-Brown K., Thomas L., Ferreira M. A., Bender D., et al. (2007). PLINK: a tool set for whole-genome association and population-based linkage analyses. Am. J. Hum. Genet. 81, 559–575. doi: 10.1086/519795
Quast C., Pruesse E., Yilmaz P., Gerken J., Schweer T., Yarza P., et al. (2013). The SILVA ribosomal RNA gene database project: improved data processing and web-based tools. Nucleic Acids Res. 41, D590–D596. doi: 10.1093/nar/gks1219
Rais R., Jiang W., Zhai H., Wozniak K. M., Stathis M., Hollinger K. R., et al. (2016). FOLH1/GCPII is elevated in IBD patients, and its inhibition ameliorates murine IBD abnormalities. JCI Insight 1, e88634. doi: 10.1172/jci.insight.88634
Ramasamy K. T., Reddy M. R., Raveendranathan D. N., Murugesan S., Chatterjee R. N., Ullengala R., et al. (2010). Differential expression of Toll-like receptor mRNA in White Leghorn and indigenous chicken of India. Vet. Res. Commun. 34, 633–639. doi: 10.1007/s11259-010-9431-0
Ramayo-Caldas Y., Mach N., Lepage P., Levenez F., Denis C., Lemonnier G., et al. (2016). Phylogenetic network analysis applied to pig gut microbiota identifies an ecosystem structure linked with growth traits. ISME J. 10, 2973–2977. doi: 10.1038/ismej.2016.77
Rothschild D., Weissbrod O., Barkan E., Kurilshikov A., Korem T., Zeevi D., et al. (2018). Environment dominates over host genetics in shaping human gut microbiota. Nature 555, 210–215. doi: 10.1038/nature25973
Rychlik I., Elsheimer-Matulova M., Kyrova K. (2014). Gene expression in the chicken caecum in response to infections with non-typhoid Salmonella. Vet. Res. 45, 119. doi: 10.1186/s13567-014-0119-2
Saavedra P. H. V., Trzeciak A., Wang Z., Saitz Rojas W., Zago G., Docampo M. D., et al. (2022). A microbiota-derived metabolite instructs peripheral efferocytosis. bioRxiv 504322. doi: 10.1101/2022.08.17.504322
Sergeant M. J., Constantinidou C., Cogan T. A., Bedford M. R., Penn C. W., Pallen M. J. (2014). Extensive microbial and functional diversity within the chicken caecal microbiome. PloS One 9, e91941. doi: 10.1371/journal.pone.0091941
Slawinska A., Hsieh J. C., Schmidt C. J., Lamont S. J. (2016). Heat stress and lipopolysaccharide stimulation of chicken macrophage-like cell line activates expression of distinct sets of genes. PloS One 11, e0164575. doi: 10.1371/journal.pone.0164575
Spor A., Koren O., Ley R. (2011). Unravelling the effects of the environment and host genotype on the gut microbiome. Nat. Rev. Microbiol. 9, 279–290. doi: 10.1038/nrmicro2540
Sun M., Wu W., Liu Z., Cong Y. (2017). Microbiota metabolite short chain fatty acids, GPCR, and inflammatory bowel diseases. J. Gastroenterol. 52, 1–8. doi: 10.1007/s00535-016-1242-9
Terao M., Romao M. J., Leimkuhler S., Bolis M., Fratelli M., Coelho C., et al. (2016). Structure and function of mammalian aldehyde oxidases. Arch. Toxicol. 90, 753–780. doi: 10.1007/s00204-016-1683-1
Traughber C. A., Timinski K., Prince A., Bhandari N., Neupane K., Khan M. R., et al. (2024). Disulfiram reduces atherosclerosis and enhances efferocytosis, autophagy, and atheroprotective gut microbiota in hyperlipidaemic mice. J. Am. Heart Assoc. 13, e033881. doi: 10.1161/JAHA.123.033881
van Schaarenburg R. A., Magro-Checa C., Bakker J. A., Teng Y. K., Bajema I. M., Huizinga T. W., et al. (2016). C1q deficiency and neuropsychiatric systemic lupus erythematosus. Front. Immunol. 7, 647. doi: 10.3389/fimmu.2016.00647
Vaser R., Adusumalli S., Leng S. N., Sikic M., Ng P. C. (2016). SIFT missense predictions for genomes. Nat. Protoc. 11, 1–9. doi: 10.1038/nprot.2015.123
Walugembe M., Amuzu-Aweh E. N., Botchway P. K., Naazie A., Aning G., Wang Y., et al. (2020). Genetic basis of response of Ghanaian local chickens to infection with a lentogenic newcastle disease virus. Front. Genet. 11, 739. doi: 10.3389/fgene.2020.00739
Wang J., Linnenbrink M., Kunzel S., Fernandes R., Nadeau M. J., Rosenstiel P., et al. (2014). Dietary history contributes to enterotype-like clustering and functional metagenomic content in the intestinal microbiome of wild mice. Proc. Natl. Acad. Sci. U S A. 111, E2703–E2710. doi: 10.1073/pnas.1402342111
Yang J., Lee S. H., Goddard M. E., Visscher P. M. (2011). GCTA: a tool for genome-wide complex trait analysis. Am. J. Hum. Genet. 88, 76–82. doi: 10.1016/j.ajhg.2010.11.011
Yasuhiko Y., Haraguchi S., Kitajima S., Takahashi Y., Kanno J., Saga Y. (2006). Tbx6-mediated Notch signalling controls somite-specific Mesp2 expression. Proc. Natl. Acad. Sci. U S A. 103, 3651–3656. doi: 10.1073/pnas.0508238103
Yin L., Zhang H., Tang Z., Xu J., Yin D., Zhang Z., et al. (2021). rMVP: A memory-efficient, visualization-enhanced, and parallel-accelerated tool for genome-wide association study. Genomics Proteomics Bioinf. 19, 619–628. doi: 10.1016/j.gpb.2020.10.007
Yu M., Liang W., Wen S., Zhao T., Zhu M. X., Li H. H., et al. (2014). EphB2 contributes to human naive B-cell activation and is regulated by miR-185. FASEB J. 28, 3609–3617. doi: 10.1096/fj.13-247759
Yu G., Wang L. G., Han Y., He Q. Y. (2012). clusterProfiler: an R package for comparing biological themes among gene clusters. OMICS 16, 284–287. doi: 10.1089/omi.2011.0118
Yuan Z., Yan W., Wen C., Zheng J., Yang N., Sun C. (2020). Enterotype identification and its influence on regulating the duodenum metabolism in chickens. Poult Sci. 99, 1515–1527. doi: 10.1016/j.psj.2019.10.078
Zhang C., Dong S. S., Xu J. Y., He W. M., Yang T. L. (2019). PopLDdecay: a fast and effective tool for linkage disequilibrium decay analysis based on variant call format files. Bioinformatics 35, 1786–1788. doi: 10.1093/bioinformatics/bty875
Zhang R., Wu H., Li Y., Huang Z., Yin Z., Yang C. X., et al. (2023). GWLD: an R package for genome-wide linkage disequilibrium analysis. G3 (Bethesda) 13, jkad154. doi: 10.1093/g3journal/jkad154
Zhang B., Zhang H., Yu Y., Zhang R., Wu Y., Yue M., et al. (2021). Effects of Bacillus Coagulans on growth performance, antioxidant capacity, immunity function, and gut health in broilers. Poult Sci. 100, 101168. doi: 10.1016/j.psj.2021.101168
Zheng D., Liwinski T., Elinav E. (2020). Interaction between microbiota and immunity in health and disease. Cell Res. 30, 492–506. doi: 10.1038/s41422-020-0332-7
Keywords: GWAS, WGS, chicken, enterotypes, microbiota, caecal microbiome, immune system
Citation: Hinsu A, Dai X, Dadousis C, Hay M, Fosso B, Crotta M, Pandit R, Guitian J, Tomley F, Koringa P, Joshi C, Blake D and Psifidi A (2025) Unveiling host-genetic drivers of caecal microbial communities in chickens through genome-wide association studies. Front. Microbiomes 4:1539923. doi: 10.3389/frmbi.2025.1539923
Received: 05 December 2024; Accepted: 23 January 2025;
Published: 18 February 2025.
Edited by:
Mattia Pirolo, University of Copenhagen, DenmarkReviewed by:
Guangqi Gao, Inner Mongolia Agricultural University, ChinaCopyright © 2025 Hinsu, Dai, Dadousis, Hay, Fosso, Crotta, Pandit, Guitian, Tomley, Koringa, Joshi, Blake and Psifidi. This is an open-access article distributed under the terms of the Creative Commons Attribution License (CC BY). The use, distribution or reproduction in other forums is permitted, provided the original author(s) and the copyright owner(s) are credited and that the original publication in this journal is cited, in accordance with accepted academic practice. No use, distribution or reproduction is permitted which does not comply with these terms.
*Correspondence: Ankit Hinsu, YWhpbnN1QHJ2Yy5hYy51aw==; Androniki Psifidi, YXBzaWZpZGlAcnZjLmFjLnVr
†Present address: Xiaoxia Dai, Department of Agricultural and Environmental Sciences, University of Milan, Milan, Italy
Matteo Crotta, European Food Safety Authority (EFSA), Parma, Italy
‡These authors have contributed equally to this work and share first authorship
Disclaimer: All claims expressed in this article are solely those of the authors and do not necessarily represent those of their affiliated organizations, or those of the publisher, the editors and the reviewers. Any product that may be evaluated in this article or claim that may be made by its manufacturer is not guaranteed or endorsed by the publisher.
Research integrity at Frontiers
Learn more about the work of our research integrity team to safeguard the quality of each article we publish.