- 1Science and Technology Policy Institute, Sejong, South Korea
- 2Institute of Convergence Science, Yonsei University, Seoul, South Korea
- 3Department of Library and Information Science, Yonsei University, Seoul, South Korea
Social media has attracted the attention of the academic community as an emerging communication channel. This channel opens a new opportunity to measure the impact of social use of scholarly publications in social media (altmetrics) that supplements our understanding on the scholarly impact of publications (bibliometrics). Two different channels, social media and journal, are known to establish various citation patterns statistically. However, thematic difference between altmetrics and bibliometrics structurally and contextually is unknown. Therefore, we perform document co-citation network analysis for structural comparison and topic modeling for contextual comparison. We also suggest Spearman’s correlation for statistical comparison. A case study is done for the publications from Journal of the Association for Information Science and Technology and the tweets mentioning the publications. We identified a weak correlation between scholarly impact and social use of these publications. We also found the structures of the traditional citations and Twitter citations share common but high interest in information retrieval system and impact analysis, while Twitter citations have diverse interest in data mining, network analysis, and information behavior as well. In addition, from content analysis, we found the two citation patterns to have both common and distinct characteristics. Specifically, the topics covered by both citation patterns show intersections and exclusive contexts. In conclusion, the traditional citation patterns and the Twitter citation patterns in Information Science are different statistically, structurally, and contextually. We suspect that intentional and unintentional citing behaviors are the main factor for the thematic difference and will be examined on the future.
Introduction
Social media has attracted the academic community as an emerging communication channel. This channel has brought the change in citation pattern and behavior and creates an opportunity to investigate new methods of measuring scholarly impact, including social use. Scholarly documents are mentioned online ~35,000 times each day, i.e., once every 2.5 s (Rouhi et al., 2015). The present study indicates that scholarly communication on social media influences both scholarly and social impacts of a research product. Social impact indicates the social usage of a scholarly publication. Thus, altmetrics, which calculates the social impact of a research product, has been developed. Alternative metrics, altmetrics, is a new metrics of measuring scholarly impact that complements bibliometrics approaches. According to OECD (2016), this new metrics will be increasingly used alongside more traditional bibliometrics to assess scholarly impact.
A Twitter citation is the application of an academic citation to Twitter. Priem and Costello (2010) defined a Twitter citation as “direct and indirect links describing a scientific paper in a tweet.” They also stressed the importance of citation analysis of scholarly communication in Twitter. According to the previous studies, there is a significant correlation between traditional and Twitter citations (Priem et al., 2012; Haustein et al., 2013, 2014; Thelwall et al., 2013). These studies found that, relative to measuring the impact of a publication, Twitter citations complement traditional citations. Twitter citations can reflect both scholarly and social impacts of a publication (Eysenbach, 2011; Bornmann, 2012, 2013, 2015; Priem, 2014). However, the relationship between scholarly communication in information science via scientific journals and social media is unknown. Besides, these studies could not delineate thematic similarities and differences of citation patterns between the traditional journal publications and tweets structurally and contextually.
The scholarly communication channel extension caused by Twitter necessitates the identification of the features in the traditional citations and Twitter citations in multiple perspectives. Statistical analysis based on citation counts helps us comprehend the difference between two different kinds of citations in influence flow only. However, this analysis can be augmented by document co-citation analysis and topic modeling in thematic. In macro level, document co-citation analysis results in knowledge structures of titles in the traditional citations and the Twitter citations. In micro level, topic modeling extracts topics from the two different citations.
Therefore, the objective of this study is to thematically compare traditional and Twitter citation patterns using structural and contextual content analyses, along with statistical analysis. Specifically, in this study, we answer three research questions: (1) “Are traditional and Twitter citations in information science significantly different in statistical perspective?” (2) “Do traditional and Twitter citations in information science have a structural difference when constructing networks of co-occurring papers in their reference list?” (3) “Are traditional and Twitter citations in information science contextually different in topics?” To answer these questions, we employ Spearman’s correlation analysis, document co-citation analysis, and Dirichlet multinomial regression (DMR) topic modeling analysis. We select papers published in the Journal of the Association for Information Science and Technology (JASIST), which is an eminent information science journal, as a case.
In our study, we seek for the degree of the statistical correlation between scholarly impact and social use of these publications. Also, we examine the difference of knowledge structures in the traditional citations and the Twitter citations. Finally, we investigate the topical difference in the two different sorts of citations. In this sense, one contribution is supporting structural and contextual ways to compare bibliometrics and altmetrics in content level. The other contribution is verifying different thematic patterns of the traditional citations and Twitter citations involving information science.
Related Works
Altmetrics has been introduced to measure the impact of scholarly communication on the Web (Thelwall et al., 2013). They are web-based metrics used to evaluate the impact of a scholarly product using a social media platform (Priem et al., 2010; Bornmann, 2014). The development of various social media platforms has allowed scholars to diversify communication channels. In altmetrics, reference to a scholarly product on a social media platform, such as Twitter is considered a citation. According to Thelwall et al. (2013), altmetrics measures the number of people responding to a research paper or research data via social media. Altmetrics is also defined as an activity that calculates the impact of a research product and are used to compute the social impact of a research product in a multifaceted and integrated manner.
The impact factor of a research product, calculated using the traditional citations, is based on the number of scholarly citations; therefore, the calculation cannot include the impact on non-scholars (Bar-Ilan et al., 2012). The impact factor is evaluated by counting the number of citations after a certain period after the publication date. Typically, a research product is a journal rather than a paper, and the impact factor does not reveal the influence of any individual paper.
However, altmetrics can quantify the scholarly impact of an individual paper based on traditional citations and can scale its social impact based on Twitter citations. Thus, the measurement covers both scholars and non-scholars. Therefore, Twitter citations complement traditional citations by weighting the scholarly impact of an individual research product (Bar-Ilan et al., 2012; Costas et al., 2014).
Altmetrics has several advantages (Thelwall et al., 2013). First, it values the scholarly impact in diverse communities, which the traditional bibliometrics cannot do. Second, altmetrics can evaluate the aggregate scholarly impact of previously undetected actions of a scholar, such as searching, reading, and archiving. Mohammadi and Thelwall (2014) discovered that altmetrics reveals the impact of papers in social sciences and humanities, whereas traditional bibliometrics is more appropriate to detect the impact of papers in science and engineering. Third, altmetrics ranks responses to a research product via the Web; thus, the time between publication and the response to a paper is short. In addition, based on quantitative evaluation, one can predict the future impact of a product. Fourth, altmetrics provides a way to assess the impact of a researcher’s activities because it can incorporate data from non-scholarly sources. Finally, a scholar can verify the various impacts of research products that the scholar is interested in; thus, scholars can evaluate the multifaceted impact of their research (Liu, 2014).
The present studies report that scholarly communication via social media (e.g., Twitter) and the Web is increasing (Thelwall et al., 2013). Most scholars who post tweets mention studies they are interested in (Priem and Costello, 2010). Priem and Costello (2010) defined a Twitter citation as “direct and indirect URLs referring to scholarly literature in a tweet.” They classified a Twitter citation as a secondary citation if a mediating webpage exists between a tweet and a paper published on the web. Otherwise, a Twitter citation is categorized as a primary citation.
A Twitter citation is made by referring to a URL or the paper title in the tweet, as shown in Figure 1. In the figure, the URL directs the user to a webpage related to the paper. If Twitter user A mentions paper B in a tweet, the number of Twitter citations for that paper is one. If Twitter user B retweets user A’s tweet, the number of Twitter citations is two. The present studies define a Twitter citation in three ways (Weller et al., 2011): (1) a Twitter citation references the content of a research product or contains a URL to the research product; (2) a scholar posts on Twitter; and (3) a hashtag relevant to the research product is included in a tweet. In this study, we count tweets and retweets. Twitter citation is computed when one of the following conditions are met: (1) if the URL of a scholarly publication is mentioned in a tweet, (2) if the title of the publication is mentioned in a tweet, or (3) if one of two tweets is retweeted.
Studies on altmetrics, e.g., Twitter citation analysis, are new to bibliometrics. However, active scholarly communication on Twitter is increasing. The authors of previous altmetrics studies collected tweets from Twitter manually. Recently, many journals have begun to provide the number of Twitter citations, including the Public Library of Science publishing project and the Journal of Medical Internet Research. Thus, it is becoming evident that publishers should treat the social impact of research products, such as scientific papers, as important (Eysenbach, 2011).
The present studies of Twitter citations often analyze the patterns and relationships between Twitter citations and traditional citations using qualitative content analysis. Priem and Costello (2010) conducted content analysis by interviewing 26 people about their motivation and Twitter citation pattern. They collected 2,483 recent tweets (~100 from each interviewee) and analyzed the conversation mediated by citations, their responsiveness, and their impact. They stated that Twitter citations influence researchers’ thought processes; thus, an analysis of Twitter citations can reveal the nature of this scholarly communication on Twitter. Some scholars have studied tweets that contain a conference name posted during the conference period (Letierce et al., 2010; Weller et al., 2011). Their studies resulted in tweet patterns according to the topics, number of tweets, and domains of the literature cited. In particular, Weller et al. (2011) collected 2,425 and 1,206 tweets relative to two conferences. They examined topics from URLs in tweets and the distributions of retweets and mentions over time.
In addition, the present studies investigated the relationship between traditional and Twitter citations. These studies include statistical analysis but exclude content analysis. Eysenbach (2011) analyzed 4,208 tweets, containing the titles of 286 articles in medical research and examined the distribution of Twitter citations over time. This study clarified that the tweet counts referring to papers can predict Twitter citation counts with the explanation power of 27%. This result was based on a correlation analysis of tweet counts and traditional citation counts and a multivariate linear model. The citation counts of frequently cited papers were 11 times greater than those of the others. The number of tweets mentioning a certain paper is a predictor of the citation counts of the paper.
Costas et al. (2014) analyzed the relationships between the indicators of altmetrics other than a Twitter citation and the number of citations using factor analysis and correlation analysis. Bar-Ilan (2012) discovered a significant correlation between citation counts and Mendeley reader numbers (i.e., an indicator of altmetrics) for papers published in JASIST.
We use text mining to transform our citation dataset to networks and topics to compare traditional and Twitter citations structurally and contextually. The contributions of this study are as follows. We show a statistical relationship between traditional and Twitter citations in information science. We reveal similarities and differences between traditional and Twitter citations using document co-citation network analysis. In addition, we compare the topical citation patterns of Twitter and traditional citations using statistical topic modeling.
Methodology
Data Collection
As shown in Figure 2, we apply correlation analysis, document co-citation analysis, and topic modeling analysis to two corpora. We only include journal papers in the corpora, because the papers published in journals are more complete and comprehensive than conference and review papers. Conference papers can be characterized as a brief reporting of preliminary findings. Review papers are not new and known to summarize the current state of a topic.
First, we collect 1,999 journal papers in JASIST between 2001 and 2014 from Scopus. For each paper, we extract title, author names, journal name, publication date, citation counts, abstract, and references.
Second, we also collect 2,155 tweets, containing “JASIST” or “Journal of Association for Information Science and Technology,” but posted between 2007 and 2014 from Twitter. For each tweet, we extract tweet ID, user ID, body, retweet counts, publication time, and keywords using the Twitter API of TOPSY (Table 1). TOPSY was a twitter and general social media analytics tool. In particular, 1,382 records of the 2,155 tweets cited 402 papers; 1,174 records of the 1,382 records contained a URL for the paper, 191 records included titles, and 18 records included the DOI.
Third, as shown in Figure 3, we distil tweet data as similar as data from journal papers. If a tweet includes a URL, we use the Java JSoup Parser1 to extract the title, author names, publication date, journal name, references, and abstract by parsing the webpage corresponding to the URL. If a tweet contains a title, we retrieve author names, publication date, journal name, citation count, abstract, and references through the Scopus API2 by using the title. If a tweet contains two or more URLs or titles, we consider them as unique citations.
Statistical Pattern Comparison: Correlation Analysis
The correlation between traditional and Twitter citation counts provides insight into the relationship between the scholarly and social impacts of scientific literature. Therefore, in this study, we apply Spearman’s correlation analysis to papers from Scopus and tweets dealing with information science using SPSS. We select 131 common papers from both Scopus and Twitter. For Twitter citation counts, we add the number of tweets citing the papers and the number of retweets.
Structural Pattern Comparison: Document Co-citation Network Analysis
Phase 1: Construct Document Co-citation Networks
We build document co-citation networks for both traditional and Twitter citations. A document co-citation network is a network where nodes are the titles of papers listed in reference sections. Edges between two titles are built if they appear in the same reference list. The weight of an edge is the frequency of co-occurrence of two titles in reference sections.
In case of tweet, we take the reference list of a paper cited by a tweet with “JASIST” or “Journal of the Association of Information Science and Technology.” We assume that this tweet extends scholarly communication. The tweet is affected by its citing paper directly and the references of the paper indirectly. A Twitter user may cite a paper in a tweet without knowing the reference papers of the paper. However, the reference papers can be considered as the elements of an implicit historical context of the tweet. This historical context can be shown in a document co-citation network.
Phase 2: Analyze Document Co-citation Networks
For each document co-citation network, we visualize it and analyze it by using Gephi 0.8.23. We first concentrate on weighted degree centralities of nodes. The weighted degree centrality of a node indicates how many times the node has been cited with other nodes. The node with high weighted degree centrality is the popular paper cited in the traditional citations or the Twitter citations.
And then, we apply a community detection algorithm (Blondel et al., 2008) to the network for partitioning the networks into modules of relevant nodes. This algorithm is an agglomerative clustering procedure that calculates the internal density of a module in order to separate sparse linkages and maintain highly dense linkages. In this algorithm, modularity implies the degree of nodes that become modules. Thus, a network with high modularity means that the frequency of co-occurring nodes is small; the network has many clusters (Wasserman and Faust, 1994). We select the top several modules in the order of their sizes.
We label a module using frequent terms in the titles and abstracts with high weighted degree centrality in the module. The frequent terms are selected after lemmatizing terms and removing stop words using Stanford CoreNLP4.
In the end, we compare the top central nodes (i.e., equivalent to papers designated by titles) of the traditional document co-citation network and the Twitter document co-citation network by modules. We also compare the two networks by their heavy weighted edges. In this way, we detect thematic similarity and difference between scholarly and social media communication.
Contextual Pattern Comparison: DMR Topic Model
We apply a DMR topic model (Mimno and McCallum, 2012) to our datasets. DMR provides a probability distribution over terms for each topic after we predefine the number of topics. The terms with high probability (i.e., weight) are representative words for the topic the terms are assigned to. Such terms allow a user to recognize the concept of the topic. In our study, each topic was labeled by three doctors and three master degree students. They read the top frequent words and abstracts of the top papers representing the topic. Then, they label a proper name for the concept the words and the abstracts stand for. Note that DMR takes a third parameter to regress topics over the parameter. In this study, we set citation time (i.e., year) as the third parameter. For a traditional citation, the publication date of a paper citing another paper is citation time. For a Twitter citation, the date a tweet that includes a reference to a paper is posted indicates citation time.
We examine the similarity and differences between two dynamic topics from traditional and Twitter citations in information science. The two topics should be comparable and similar. We define two topics as similar if the cosine similarity between two vectors from the two topics is greater than a given threshold, e.g., 0.5, where the cosine similarity is a value between 0 and 1. The two vectors are the frequency vectors of the top 20 terms in the two topics. If a topic is similar to two different topics, we pair the topic with the most similar topic.
Thereafter, we compare two sets of time series data that represent the content of traditional and Twitter citations of the same concept (i.e., a label). We draw the increase and decrease in publication of Twitter citations for each topic over time as time series data. If a topic has a positive value, its proportion is increasing, and the magnitude of the value indicates the extent of the proportional increase. Similarly, if a topic has a negative value, its proportion is decreasing, and the magnitude of the value represents the extent of the proportional decrease. The slope is a marginal change in the proportional increase and decrease.
We compare the increases and decreases in topical trends because we concentrate on the degree of changes in topic proportions over time. A traditional citation originates from scholarly communication, whereas a Twitter citation comes from social media communication. The two communication channels have different response times. A tweet mentions a paper instantly, whereas the journal publication takes several months or years to be available to the public (Thelwall et al., 2013).
Results
Descriptive Statistics
Frequently cited papers in both traditional and Twitter citations are listed in Table 2. Here, common and frequently cited terms on both sides are “social network” and “Twitter.” This implies that both scholars in information science and people who pay attention to information science are interested in social networking services, particularly Twitter. In addition, the scholarly impact, including citations, is another major interest for scholars and non-scholars. This indicates that papers delineating an impact analysis in bibliometrics are widely cited in publications and tweets. Web-based information retrieval and information behavior are other popular themes that occur in both traditional and Twitter citations. Popular concepts, such as SNS, information retrieval and scholarly impact in traditional and Twitter citation are similar, but non-popular concepts, such as Knowledge management system and open access are different in the two citations.
Statistical Pattern Comparison: Correlation Analysis
Our correlation analysis between the traditional and Twitter citation counts (i.e., the sum of the citation counts of tweet and retweets) in information science results in 0.393 at a significance level of 0.001. Therefore, we confirm a significant but weak relationship between the two counts. This implies that, in information science, the impact of a paper in social media is greater as the impact of the paper in scholarly communication increases. However, the relationship is weak, which indicates that impactful topics in both communication channels differ.
This finding complies with present studies of traditional and Twitter citation patterns. The Twitter citations can reveal the impact of papers in social science and humanities, which are difficult to detect using bibliometrics (Eysenbach, 2011), as personal information management tools (Mohammadi and Thelwall, 2014). The publication cycles of social science journals are often longer than those of science or engineering journals; thus, the citation cycles of social science journals are also longer than those of science or engineering journals. This results in lower citation counts in social science papers compared to science or engineering papers. Therefore, the impact of social science papers tends to depreciate. Some previous studies that have indicated weak correlations between traditional and Twitter citations have suspected that the difference in citation behaviors and the delivering contents between traditional and Twitter citations causes this weak correlation (Bornmann, 2015). However, they have not provided thematic differences. We support topical dissemblance in the following.
Structural Pattern Comparison: Document Co-citation Network Analysis
Each document co-citation network contains edges with weights greater than 1. The traditional document co-citation network includes 1,301 nodes and 2,161 edges. The average degree centrality of this network is 3.32. The Twitter document co-citation network has 1,288 nodes and 3,384 edges, and the average degree centrality of the network is 5.26. On average, the Twitter document co-citation network has nodes with more connections.
The traditional document co-citation network is visualized in Figure 4A, and the Twitter document co-citation network is visualized in Figure 4B. The different colors represent various modules in each network, after applying a community detection algorithm. Note that each figure only shows the eight largest modules for each network. In this case, the size of the Twitter document co-citation network is larger than that of the traditional document co-citation network. The number of nodes in the traditional and Twitter networks is 509 and 707, respectively. The three largest modules in the Twitter network comprise ~56% of the 707 nodes, and the three largest modules in the traditional network comprise ~48% of the 509 nodes. The mean proportions of the modules of the two networks are similar. However, the variance in the module proportions of the traditional document co-citation network is 10.24 and that of the Twitter document co-citation network is 28.03. That is, modules are more centralized in the Twitter network than in the traditional network. As can be seen, Figure 4B shows denser clusters than Figure 4A.
In the traditional document co-citation network, the most popular module is the information retrieval system (17.84%). The following popular modules are citation metrics (15.69%), webometrics (13.73%), co-citation analysis (13.14%), collaboration analysis (11.57%), impact analysis (11.37%), informetrics (9.41%), and search engine (7.06%).
In the Twitter document co-citation network, the most popular module is scholarly communication (22.35%), followed by the information retrieval system (19.94%), citation analysis (13.72%), data mining (9.62%), impact analysis (9.48%), network analysis (8.35%), information behavior (8.35%), and citation network analysis (8.20%).
Thus far, we have observed differences between the traditional and Twitter document co-citation networks relative to both total and module levels. Here, we speculate prominent edges in the networks. Table S2A in Supplementary Material shows the 25 strong ties in the traditional document co-citation network. Similarly, Table S2B in Supplementary Material shows the 25 highly weighted edges in the Twitter document co-citation network.
In the traditional document co-citation network, the strongest tie was made between two papers, i.e., “Mapping Authors in Intellectual Space: A Technical Overview” and “Author Co-citation: a Literature Measure of Intellectual Structure.” They are all labeled co-citation analysis in the modules of the traditional document co-citation network. This means that they are cited concurrently and frequently in traditional citations. Although the largest module in the traditional document co-citation network is information retrieval system, the strongest tie is in the co-citation analysis module. This implies that information retrieval system is a widely used theme in traditional citations in information science. However, co-citation analysis is a theme that the traditional citations are referred in a pair frequently. We can also verify that papers in the co-citation analysis module occur in Table S2A in Supplementary Material many times. This indicates that the theme, i.e., co-citation analysis, is central in traditional citations. The second strongest tie is constructed between two papers in the informetrics and citation analysis modules. The third and fourth strongest edges are between two papers within the impact analysis module. The impact analysis is based on citation counts and an example of the papers is “A Hirsch-type Index for Journals.” The fifth, sixth, and seventh strongest connections are made between two papers in the information retrieval system module. In addition, several papers in the same module are listed in Table S2A in Supplementary Material. These results comply with the finding that information retrieval system is widespread in traditional citations, and the theme is not a significantly dominant module in information science for JASIST.
In the Twitter document co-citation network, the strongest connection is between two papers, i.e., “Information Behavior” and “Information Needs and Uses.” They are assigned to a module of the Twitter document co-citation network labeled information behavior. However, we have already confirmed that scholarly communication, information retrieval system, and citation analysis were dominant modules in the Twitter document co-citation network. Comparing the module proportions, Twitter users cite a small set of papers in information behavior, or a small set of papers in information behavior is available such that some pairs of information behavior papers are referenced frequently in Twitter citations. Among the items listed in Table S2B in Supplementary Material, the papers in information behavior frequently occur with papers in other modules. We assume that Twitter users are interested in information behavior in relation to other themes. For example, image archives created an intersection between the information retrieval system and information behavior modules. In the document co-citation network of Twitter citations, the data mining module was identified and the papers in this module were frequently coupled with the papers in network analysis.
Contextual Pattern Comparison: DMR Topic Model
To examine the difference between traditional and Twitter citations over time, we extract dynamic topics from the two sides by applying DMR to the traditional and Twitter citation records. The records are reconfigured because the same record can be cited several times over a certain period. The reconfigured records of the traditional citations include 23,377 records and those of the Twitter citations have 1,092 records. We fix the number of topics to 10. The DMR results for the reconfigured traditional and Twitter citation records are given in Table 3. Each row shows a topic number, a topic label, the top 15 frequent terms in the topic, and the titles of three representative papers. Experts, including three doctoral and master’s students in information science, label each topic by reading its frequent terms and representative papers. In addition, we set two topics with cosine similarity greater than 0.5 with the same label.
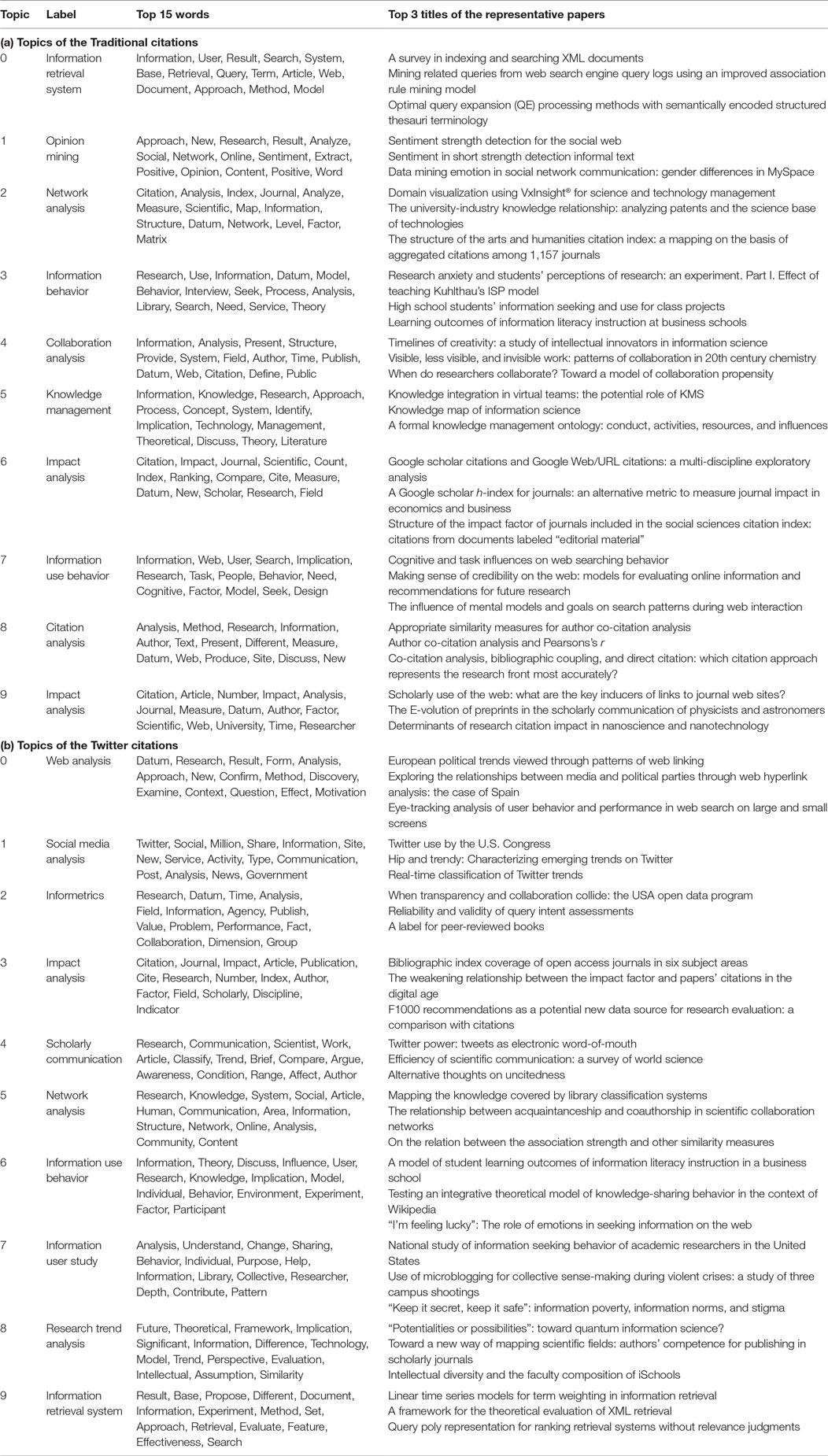
Table 3. Descriptions of the topics of (a) traditional and (b) Twitter citations from a DMR topic model.
Figure 5A shows the increase and decrease in the traditional citations of each topic over time. Overall, all topics decrease but many topics increase, with the exception of topics 0 and 3. Topic 0 (gray) is labeled information retrieval system. Its proportion increased in 2007 but decreased after that. In 2015, the proportion was still decreasing, and the extent of the decrease was the largest. This implies that, for traditional citations, the popularity of information retrieval system has decreased. Topic 1 (yellow) is labeled opinion mining, which was one of the most rapidly decreasing topics between 2007 and 2010. After 2010, its marginal decrease in proportion became increasingly smaller. Since 2013, its proportion has increased. Now, its increasing degree is the greatest. This indicates that opinion mining has become more popular recently. Topic 2 (blue) is network analysis. Similar to topic 1, its popularity and proportion have increased. Its proportion was in decline until 2013, but the marginal decrement also decreased. This implies that network analysis was a minor topic in the past but is now important. Topic 3 (green) is information behavior. It is similar to topic 0. In the past, it was popular but now it is a minor topic. Topic 7 (dark yellow) is similar to topic 3.
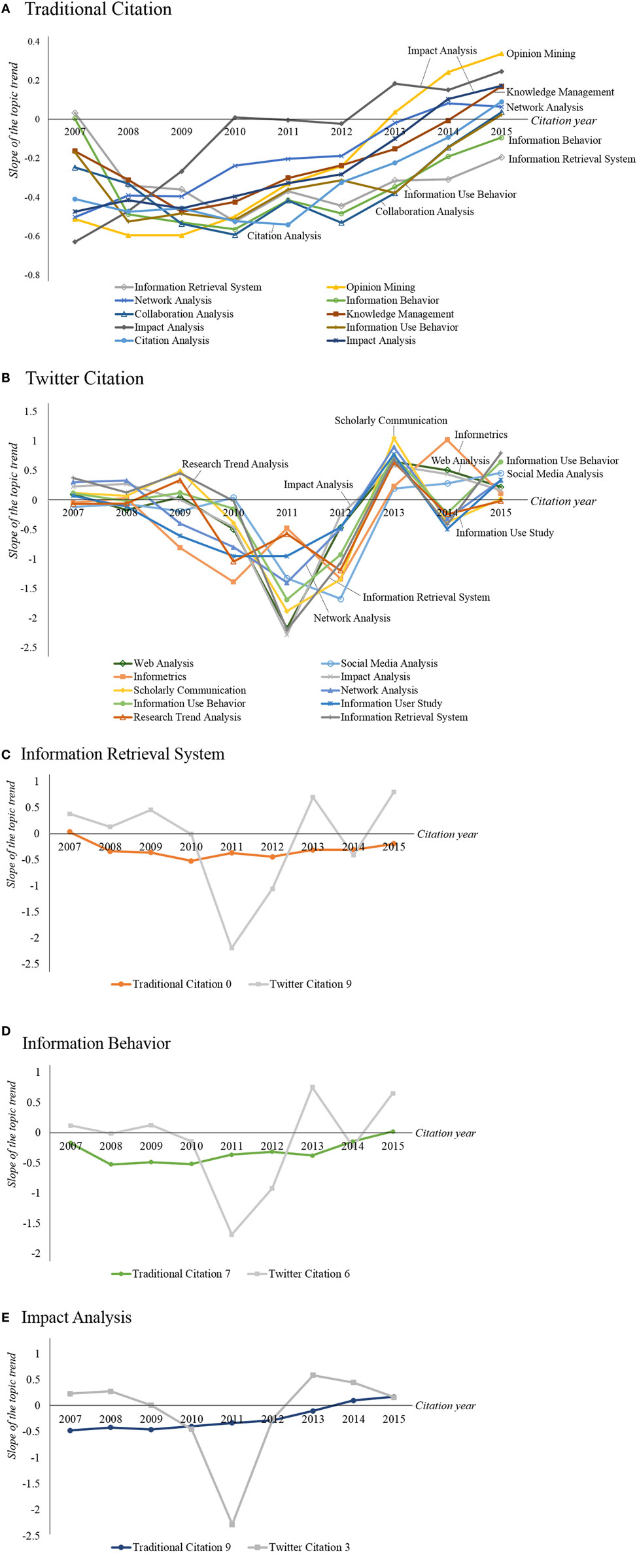
Figure 5. Topical trends of (A) traditional and (B) Twitter citations; comparison between traditional and Twitter citation trends for (C) topic 0 (traditional) and topic 9 (Twitter), (D) topic 7 (traditional) and topic 6 (Twitter), and (E) topic 9 (traditional) and topic 3 (Twitter); The y-axis is the slope of the topic trend and the x-axis is the citation year.
Topic 4 (dark blue) is collaboration analysis, which has a similar pattern as topic 3. Topic 5 (red) is knowledge management, and its proportion has decreased since 2007 but has been increasing since mid-2013. Topic 6 (dark gray) is impact analysis, and its decrease in proportion was the greatest in 2007; however, the rate of decrease has diminished drastically since. Its proportion became stable between 2010 and 2012. After 2012, its citation is increasing. This implies that investigating the impact became important and popular in a short period. Topic 9 (navy blue) is similar to topic 6. Topic 8 (sky blue) is citation analysis, and its pattern is similar to topic 9. Consequently, opinion mining and impact analysis are currently the hottest topics in traditional citations. Knowledge management, network analysis, and citation analysis are also hot topics in the same citations. Collaboration analysis is a recent topic that has increased in proportion. However, information retrieval system and information behavior are popular topics in the citations.
Figure 5B shows the increase and decrease in Twitter citations for each topic over time. In total, all topics increased or decreased slightly until 2010, but their proportions decreased between 2010 and 2012. Thus, the incremented and decremented patterns of the topics form a u-shape. The numbers of citations made between 2007 and 2014 are 3, 3, 77, 303, 202, 179, 188, and 129. The change in the numbers between 2010 and 2011 is substantially large – (101); thus, all topics must have faced a proportional decrease. By comparing Figures 5A,B, it can be seen that the increase and decrease in Twitter citations for each topic over time have more fluctuations. Topic 0 (grass green) is web analysis, topic 1 (light blue) is social media analysis, topic 2 (orange) is informetrics, topic 3 (gray) is impact analysis, topic 4 (yellow) is scholarly communication, topic 5 (blue) is network analysis, topic 6 (green) is information behavior, topic 7 (sky blue) is information user study, topic 8 (red) is research trend analysis, and topic 9 (dark gray) is information retrieval system. The Twitter citations in the papers covering informetrics were in decline for 4 years following 2009. However, the rate of reduction decreased in 2011 along with the research trend analysis, unlike the other topics. From 2013, the citation rate of Twitter users for informetrics increased. In particular, in 2014, the number of tweets mentioning informetrics in social media increased greatly. Topics, such as research trend analysis, web analysis, social media analysis, and impact analysis show similar increasing and decreasing patterns as informetrics. In 2015, Twitter citations for all topics increased. Among them, impact analysis, information behavior, social media analysis, network analysis, and information user study are the top five increasing topics. We believe that social media encouraged people to pay attention to social influence, e.g., the word-of-mouth effect, via new media and user behavior within such new media. Specifically, network analysis became a popular tool for non-scholars.
We confirm that the prominent increasing and decreasing topics are similar in both traditional and Twitter citations. For example, impact analysis and network analysis are increasing. However, information retrieval system is falling. While traditional citations focus on scholarly impact, collaboration, and mining public opinion, Twitter citations focus on measuring social media impact using informetrics and network analysis.
We select the top 30 frequent keywords for each topic obtained from DMR. Then, we extract similar topics from traditional citations and Twitter citations by calculating the cosine similarity between two topics with 30 keywords for each topic. The common topics are information retrieval system, information behavior, and impact analysis. Here, we choose three pairs of topics.
One pair is topic 0 from traditional citations and topic 9 from Twitter citations, with a cosine similarity of 0.62. The two topics are in information retrieval system. Figures 5C–E show the trends of the two topics over time. The y-axis is the slope of the topic trend and the x-axis is the citation year. When a line crosses the x-axis, the topical trend encounters a min/max point. Thus, citing information retrieval system papers in publications has shown a downward trend since 2007. The slope of this decreasing trend is nearly stable. However, the trend for citing information retrieval system papers in tweets fluctuates. In Twitter, papers describing information retrieval system were cited increasingly until 2010. Then, the trend decreased from 2010 to mid-2012. Thereafter, the trend has been increasing. Another pair comprises topic 7 from traditional citations and topic 6 from Twitter citations, with a cosine similarity of 0.48. The two topics are related to information behavior. In traditional citation, the slope is downward. In mid-2009, the slope moved downward until mid-2012. Its impact increased in 2013 but decreased almost immediately thereafter. However, the impact of information behavior papers increased gradually. The third pair is topic 9 from traditional citations and topic 3 from Twitter citations, with a cosine similarity of 0.52. The two topics are related to impact analysis. In traditional citation, the impact of impact analysis had been rising from 2014. The impact of impact analysis fell from 2009 to mid-2012 but increased thereafter.
Discussion
To extend our knowledge of traditional and twitter citation based on our findings shown in Table 4, we describe four details from the findings. First, in information science, traditional citations correlate with Twitter citations. Subsequently, papers with greater scholarly impact tend to have a greater social impact. This implies that the social impact measured by Twitter citations complements the scholarly impact calculated from traditional citations in information science. However, the degree of correlation between the scholarly and the social impact is weak. With document co-citation network analysis and DMR topic modeling analysis, we found that the content of traditional and Twitter citations deals with slightly different topics.
Second, through document co-citation network analysis, we discovered that information retrieval system and impact analysis are popular topics in both traditional and Twitter citations. In traditional citations, the information retrieval system topic is the most impactful; bibliometrics is also influential. In Twitter citations, scholarly communication is the most influential. In addition, bibliometrics, data mining, network analysis, and information behavior are considered important. This implies that Twitter citations cover more diverse topics than traditional citations.
Third, the topic modeling results over time comply with our document co-citation network analysis. Information retrieval system and impact analysis were popular topics in both traditional and Twitter citations. Network analysis and information use behavior were also common topics. Traditional citations often covered subjects that are well-defined in information science, whereas Twitter citations involved trendy subjects, such as social media and web analysis. This means that Twitter users cite and share state-of-art topics.
Finally, document co-citation network analyses and topic analyses of traditional and Twitter citations revealed similarities and differences between them. The document co-citation network analysis revealed that traditional citations include content relevant to bibliometrics. Besides, the citations involve text mining and information behavior in topic analysis. However, the document co-citation network analysis and topic analysis of Twitter citations share similar results. In particular, topics related to text mining are distinct in topic analysis. According to the analyses, information retrieval system is a consistently influential topic. In addition, document co-citation network analysis enables us to observe the scholarly and social impacts at a macro level, and topic analysis allows us to speculate the impacts at a certain time at a lower level.
Conclusion
As scholarly communication channels become increasingly diversified, altmetrics has received increasing attention because it can complement the present measures for impact evaluation of a research product based on traditional citations. Altmetrics measures the impact of a research product through various social media platforms. Among such platforms, scholars and non-scholars use Twitter, which became one of the most important sources for altmetrics (Rouhi et al., 2015). However, the previous studies of traditional and Twitter citations only performed correlation analysis and content analysis. Thus, the thematic relationship between traditional and Twitter citations has not been covered to date.
In this study, we applied text mining to traditional and Twitter citations in the field of information science to examine the scholarly impact and social impact of topics. We collected JASIST publications and tweets stating the publications to extract the content of traditional and Twitter citations. First, we tested if the traditional and Twitter citation counts were correlated. Then, we built document co-citation networks from the publications and tweets to compare the networks, modules, and edges. We then applied statistical topic modeling over time to the traditional citation content and Twitter citation content. We analyzed the topic and term distributions for each topic. In addition, we examined the difference in the patterns of similar topics of traditional and Twitter citation content. With a series of experiments, we identified the complementary power of a Twitter citation over traditional citation.
This study demonstrates a weak correlation between traditional and Twitter citations in information science. The relationship between the scholarly and the social impacts of information science research is analyzed in structural and contextual perspectives. However, we restricted our dataset to JASIST and Twitter for our examination. Besides, the JASIST papers cited by tweets occupy 20% of all the JASIST papers and the twitter users who cited the JASIST papers are mainly public accounts. Our results show that the traditional citations and the Twitter citations are different statistically, structurally, and contextually. We guess that the main factor for discrepancy between the two kinds of citations is citing behavior with different motivations. A scholar cites another paper on purpose and lists it on the reference section. However, a Twitter user cites a paper to share or to promote it to friends associated by social media but the user would not be aware of the reference list of the paper. Unintentionally, the user gets influenced by the papers on the reference list. Such different citing behaviors seem to cause difference in the traditional document co-citation network and the Twitter document co-citation network. Additionally, the contexts of the traditional citations and the Twitter citations are also influenced by the factor. In future, we will cover more papers in other information science journals and include various social media platforms for greater generalization to examine whether and how intentional and unintentional citing behaviors result in different effects on the future. Our future work will help discover the relationship between bibliometrics and altmetrics in various fields. In particular, scholarly and socially influential topics will be found for scientific and commercial uses.
Author Contributions
HJ makes substantial contributions to conception and design, acquisition of data, analysis and interpretation of data. KL participates in drafting the article or revising it critically for important intellectual content. MS (corresponding author) gives final approval of the version to be submitted and any revised version.
Conflict of Interest Statement
The authors declare that the research was conducted in the absence of any commercial or financial relationships that could be construed as a potential conflict of interest.
Funding
This work was supported by the Ministry of Education of the Republic of Korea and the National Research Foundation of Korea (NRF-2015S1A3A2046711).
Supplementary Material
The Supplementary Material for this article can be found online at http://journal.frontiersin.org/article/10.3389/frma.2016.00006
Footnotes
References
Bar-Ilan, J., Haustein, S., Peters, I., Priem, J., Shema, H., and Terliesner, J. (2012). “Beyond citations: scholars’ visibility on the social web. arXiv preprint,” in 17th International Conference on Science and Technology Indicators (Montreal, Canada). arXiv:1205.5611.
Blondel, V. D., Guillaume, J. L., Lambiotte, R., and Lefebvre, E. (2008). Fast unfolding of communities in large networks. J. Stat. Mech. 2008, 10. doi: 10.1088/1742-5468/2008/10/P10008
Bornmann, L. (2012). Measuring the societal impact of research. EMBO Rep. 13, 673–676. doi:10.1038/embor.2012.99
Bornmann, L. (2013). What is societal impact of research and how can it be assessed? A literature survey. J. Am. Soc. Inf. Sci. Technol. 64, 217–233. doi:10.1002/asi.22803
Bornmann, L. (2014). Do altmetrics point to the broader impact of research? An overview of benefits and disadvantages of altmetrics. J. Informetr. 8, 895–903. doi:10.1016/j.joi.2014.09.005
Bornmann, L. (2015). Alternative metrics in scientometrics: a meta-analysis of research into three altmetrics. Scientometrics 103, 1123–1144. doi:10.1007/s11192-015-1565-y
Costas, R., Zahedi, Z., and Wouters, P. (2014). Do “altmetrics” correlate with citations? Extensive comparison of altmetric indicators with citations from a multidisciplinary perspective. J. Assoc. Inf. Sci. Technol. 66, 2003–2019. doi:10.1002/asi.23309
Eysenbach, G. (2011). Can tweets predict citations? Metrics of social impact based on Twitter and correlation with traditional metrics of scientific impact. J. Med. Internet Res. 13, e123. doi:10.2196/jmir.2012
Haustein, S., Peters, I., Sugimoto, C. R., Thelwall, M., and Larivière, V. (2014). Tweeting biomedicine: an analysis of tweets and citations in the biomedical literature. J. Am. Soc. Inf. Sci. Technol. 65, 656–669. doi:10.1002/asi.23101
Haustein, S., Thelwall, M., Larivière, V., and Sugimoto, C. R. (2013). “On the relation between altmetrics and citations in medicine,” in Proceedings of the 18th International Conference on Science and Technology Indicators (STI) September 4-6 (Berlin, Germany: European Network of Indicators Designers (ENID)), 164–166.
Letierce, J., Passant, A., Breslin, J. G., and Decker, S. (2010). “Using Twitter during an academic conference: the# iswc2009 use-case,” in ICWSM, Galway, Ireland.
Mimno, D., and McCallum, A. (2012). “Topic models conditioned on arbitrary features with dirichlet-multinomial regression,” in Proceedings of the 24th Annual Conference on Uncertainty in Artificial Intelligence 2008, Helsinki, Finland.
Mohammadi, E., and Thelwall, M. (2014). Mendeley readership altmetrics for the social sciences and humanities: research evaluation and knowledge flows. J. Assoc. Inf. Sci. Technol. 65, 1627–1638. doi:10.1002/asi.23071
OECD. (2016). “Public research system trends and issues,” in OECD Science, Technology and Industry Outlook 2016. Available at: https://www.innovationpolicyplatform.org/sti/e-outlook
Priem, J. (2014). “Altmetrics,” in Beyond Bibliometrics: Harnessing Multi-Dimensional Indicators of Performance, eds B. Cronin and C. R. Sugimoto (Cambridge, MA: MIT Press), 263.
Priem, J., and Costello, K. L. (2010). How and why scholars cite on Twitter. Proc. Am. Soc. Inf. Sci. Technol. 47, 1–4. doi:10.1002/meet.14504701201
Priem, J., Piwowar, H. A., and Hemminger, B. M. (2012). “Altmetrics in the wild: using social media to explore scholarly impact,” in ACM Web Science Conference Workshop on Altmetrics June 21, Evanston.
Priem, J., Taraborelli, D., Groth, P., and Neylon, C. (2010). Altmetrics: A Manifesto. Available at: http://altmetrics.org/manifesto/
Rouhi, S., Gunn, W., Burks, R., and Deards, K. D. (2015). “Redefining value: alternative metrics and research outputs,” in Library Conference Presentations and Speeches (Lincoln: Libraries at University of Nebraska-Lincoln), 115.
Small, H. (1973). Co-citation in the scientific literature: a new measure of the relationship between two documents. J. Am. Soc. Inf. Sci. 24, 265–269. doi:10.1002/asi.4630240406
Thelwall, M., Haustein, S., Larivière, V., and Sugimoto, C. R. (2013). Do altmetrics work? Twitter and ten other social web services. PLoS ONE 8:e64841. doi:10.1371/journal.pone.0064841
Wasserman, S., and Faust, K. (1994). Social Network Analysis: Methods and Applications. Cambridge: Cambridge University Press.
Weller, K., Dröge, E., and Puschmann, C. (2011). “Citation analysis in Twitter: approaches for defining and measuring information flows within Tweets during scientific conferences,” in Making Sense of Microposts (#MSM2011), Workshop at Extended Semantic Web Conference (ESWC 2011), eds M. Rowe, M. Stankovic, A.-S. Dadzie, and M. Hardey (Crete).
Keywords: altmetrics, Twitter citation, text mining, document co-citation analysis, topic modeling
Citation: Jung H, Lee K and Song M (2016) Examining Characteristics of Traditional and Twitter Citation. Front. Res. Metr. Anal. 1:6. doi: 10.3389/frma.2016.00006
Received: 14 June 2016; Accepted: 04 August 2016;
Published: 18 August 2016
Edited by:
Judit Bar-Ilan, Bar-Ilan University, IsraelCopyright: © 2016 Jung, Lee and Song. This is an open-access article distributed under the terms of the Creative Commons Attribution License (CC BY). The use, distribution or reproduction in other forums is permitted, provided the original author(s) or licensor are credited and that the original publication in this journal is cited, in accordance with accepted academic practice. No use, distribution or reproduction is permitted which does not comply with these terms.
*Correspondence: Min Song, bWluLnNvbmcmI3gwMDA0MDt5b25zZWkuYWMua3I=