- 1Richard T. Silver, M.D. Myeloproliferative Neoplasms (MPN) Center, Weill Cornell Medicine, New York, NY, United States
- 2Department of Biomedical Informatics, Stony Brook University, Stony Brook, NY, United States
The myeloproliferative neoplasms (MPNs) – polycythemia vera, essential thrombocytosis, and primary myelofibrosis – are chronic blood cancers that originate from hematopoietic stem cells carrying driver mutations which activate cytokine signaling pathways in hematopoiesis. MPNs are associated with high symptom burden and potentially fatal events including thrombosis and progression to more aggressive myeloid neoplasms. Despite shared driver mutations and cell of origin, MPNs have an extremely heterogenous clinical course. Their phenotypic heterogeneity, coupled with their natural history spanning several years to decades, makes personalized risk assessment difficult. Risk assessment is necessary to identify patients with MPNs most likely to benefit from clinical trials aimed at improving thrombosis-free, progression-free and/or overall survival. For MPN trials to be powered for survival endpoints with a feasibly attained sample size and study duration, risk models with higher sensitivity and positive predictive value are required. Traditional MPN risk models, generally linear models comprised of binary variables, fall short in making such trials feasible for patients with heterogenous phenotypes. Accurate and personalized risk modeling to expedite survival-focused interventional MPN trials is potentially feasible using machine learning (ML) because models are trained to identify complex predictive patterns in large datasets. With automated retrievability of large, longitudinal data from electronic health records, there is tremendous potential in using these data to develop ML models for accurate and personalized risk assessment.
Background on MPN clinical features, disease progression, and trial endpoints
Myeloproliferative neoplasms (MPNs) – including polycythemia vera (PV), essential thrombocytosis (ET), and primary myelofibrosis (PMF) – are chronic hematologic malignancies originating from hematopoietic stem cells that acquire mutations in genes involved in the activation of cytokine signal transduction pathways responsible for hematopoiesis (1). More than ~85% of MPN patients harbor mutually exclusive driver mutations in Janus kinase 2 (JAK2), calreticulin (CALR), and the thrombopoietin receptor (MPL), which are pivotal in disease initiation and propagation (1). However, the pathogenesis of MPNs is tremendously more complex, involving an intricate interplay of genetic, epigenetic, microenvironment, and inflammatory abnormalities (1). Consequently, the phenotype and clinical course are highly heterogenous, often complicated by various symptoms, thrombotic events, and progression to more aggressive myeloid neoplasms. “MPN progression” hereafter refers to the objective transition of ET/PV and prefibrotic PMF to secondary myelofibrosis (SMF) and overt PMF respectively, or ET/PV/PMF/SMF to accelerated-phase MPN (AP-MPN), or blast-phase MPN/acute myeloid leukemia (AML) as defined by the International Working Group-Myeloproliferative Neoplasms Research and Treatment (IWG-MRT), the World Health Organization (WHO) and the International Consensus Criteria (ICC) (2, 3). These prognosis-defining events occur over a highly variable timespan of a few years to several decades from initial diagnosis (4). Unfortunately, no predictive models exist for MPN progression, and current survival models do not capture biological or clinical heterogeneity and often rely heavily on non-modifiable risk factors like age.
Putative clinical and molecular risk factors for MPN progression (5) frequently reported include advanced age (6); prior thrombosis (7); elevated leukocyte count with emphasis on neutrophils (8) and higher neutrophil-to-lymphocyte ratio (9); type and mutational allele frequencies of driver mutations (e.g. JAK2, CALR, MPL) (10) or triple negative disease (11); high-risk coexisting mutations in genes involved in epigenetic regulation (e.g. IDH1/2), transcription regulation (e.g. TP53, RUNX1, and IKZF1), RNA splicing (e.g. SF3B1, U2AF1, and SRSF2) (12); cytogenetic abnormalities (13); and proinflammatory markers (14). Several prognostics scores including IPSET (15), AAA (16), DIPSS plus (17), MIPSS-ET/PV (18), MIPSS70 (19), and GIPSS (20), incorporate varying combinations of the above risk factors. The myriad potential risk factors for progression, coupled with the chronic heterogenous nature of MPNs, present significant challenges in classification, prognostication, and outcomes prediction, as well as in developing therapies that effectively prevent disease progression and improve survival.
Preventing MPN progression and related complications remains pivotal in clinical care to reduce and potentially eliminate excess mortality from MPNs (21). Unfortunately, unlike most oncology trials, MPN trials do not prioritize progression-free survival (PFS) or overall survival (OS) as primary endpoints. Instead, they target proxy endpoints such as hematologic response, spleen volume reduction (SVR), and symptom improvement, which do not necessarily predict survival (22, 23). Because MPN complications and mortality are uncommon events in randomly selected subgroup over a short study period (1-3 years), trials will be underpowered to meet a survival endpoint, unless a high-risk population is more accurately identified. Secondly, while OS is a well-defined but challenging endpoint to achieve, PFS or event-free survival (EFS) are more easily attainable but must be well-defined.
None of the trials leading to FDA approval of MPN-directed therapy were conducted with PFS or OS as the primary endpoint. The randomized COMFORT-1 and 2 trials, which compared the JAK inhibitor (JAKi) ruxolitinib to placebo and best available therapy for intermediate-2 and high-risk myelofibrosis, used SVR and symptom response as primary endpoints (24, 25). Although these trials were not powered for survival, post-hoc analyses with longer follow-up showed evidence for survival benefit (26). Extrapolating from these analyses, a similar 2-year study powered to detect an OS difference would require a sample size of approximately 1,900 patients (based on a hazard ratio of 0.70 [95% CI, 0.54–0.91]; with 80% power, alpha 0.05, 1:1 randomization) (26). Following the COMFORT trials, most studies have adopted similar endpoints of SVR and symptom response.
While disease-modifying therapies, such as interferon-alfa (IFN-α) show promise in depleting MPN stem cell pools and achieving durable molecular remissions (27–29), similar endpoint challenges remain. In the PROUD-PV/CONTINUATION-PV trials, which assessed ropeginterferon alfa-2b vs hydroxyurea control, the primary endpoint was non-inferiority in achieving complete hematological response with normal spleen size (PROUD-PV) and improved disease burden (CONTINUATION-PV) (28). Event-free survival (EFS) was later assessed through an extended 6–7-year follow-up analysis, showing a significantly higher EFS favoring ropeginterferon alfa-2b (0.94 vs 0.82; log-rank test; p = 0.04). However, clinical events were rare and median EFS was not reached (30).
The use of molecular response endpoints, such as JAK2V617F variant allele frequency (VAF) reduction (31), as a proxy for disease-modifying activity or survival has been suggested for clinical trial design, though its correlation to clinical outcomes remains debated (32). Both ruxolitinib (33, 34) and ropeginterferon (28–30) have shown sustained reductions in JAK2V617F VAF in ET and PV that correlates with improved PFS, EFS but not OS (35–37). While these reports are promising for the utility of VAF as a proxy, it is not clear it can reliably predict survival outcomes or be used to select/risk-stratify patients for survival-powered trials.
We conducted a prospective study across 107 ET, PV, and PMF patients, comparing JAK2V617F whole blood VAF to “MPN fitness” – a novel biomarker based on lineage specific biases in JAK2V617F differentiation and clonal expansion. We reported a stronger association between JAK2V617F-driven MPN stem and progenitor cell fitness and EFS compared to JAK2V617F VAF and EFS, with a significantly higher area under the curve (AUC) for MPN fitness than JAK2V617F VAF quartiles (0.8 vs 0.67, P = 0.003) (38). This work highlighted the complex biology underlying the heterogenous MPN phenotypes despite shared driver mutations, as well as the challenges in identifying the highest-risk patients for clinical trials using clinically available tests.
Despite proposed mechanisms for MPN treatment resistance and progression, the exact causes and predictors for these outcomes remain elusive (39, 40), and current risk stratification models fall short in many aspects. For example, in ET, no models currently exist to predict progression, even though CALR and MPL mutations in ET carry a significantly greater risk of progression to SMF compared to JAK2-mutated ET, as demonstrated by at least 3 recent independent cohorts (10). Similarly in PV, theELN/National Comprehensive Cancer Network (NCCN) risk stratification model (41) for thrombosis (with “high-risk” PV defined only by age > 60 years or a history of thrombosis) predicts only a 2-3% probability of thrombosis per year and does not predict progression risk (21). This positive predictive value (PPV) of ~0.03 at best would necessitate thousands of patients for a short-term study (Figure 1).
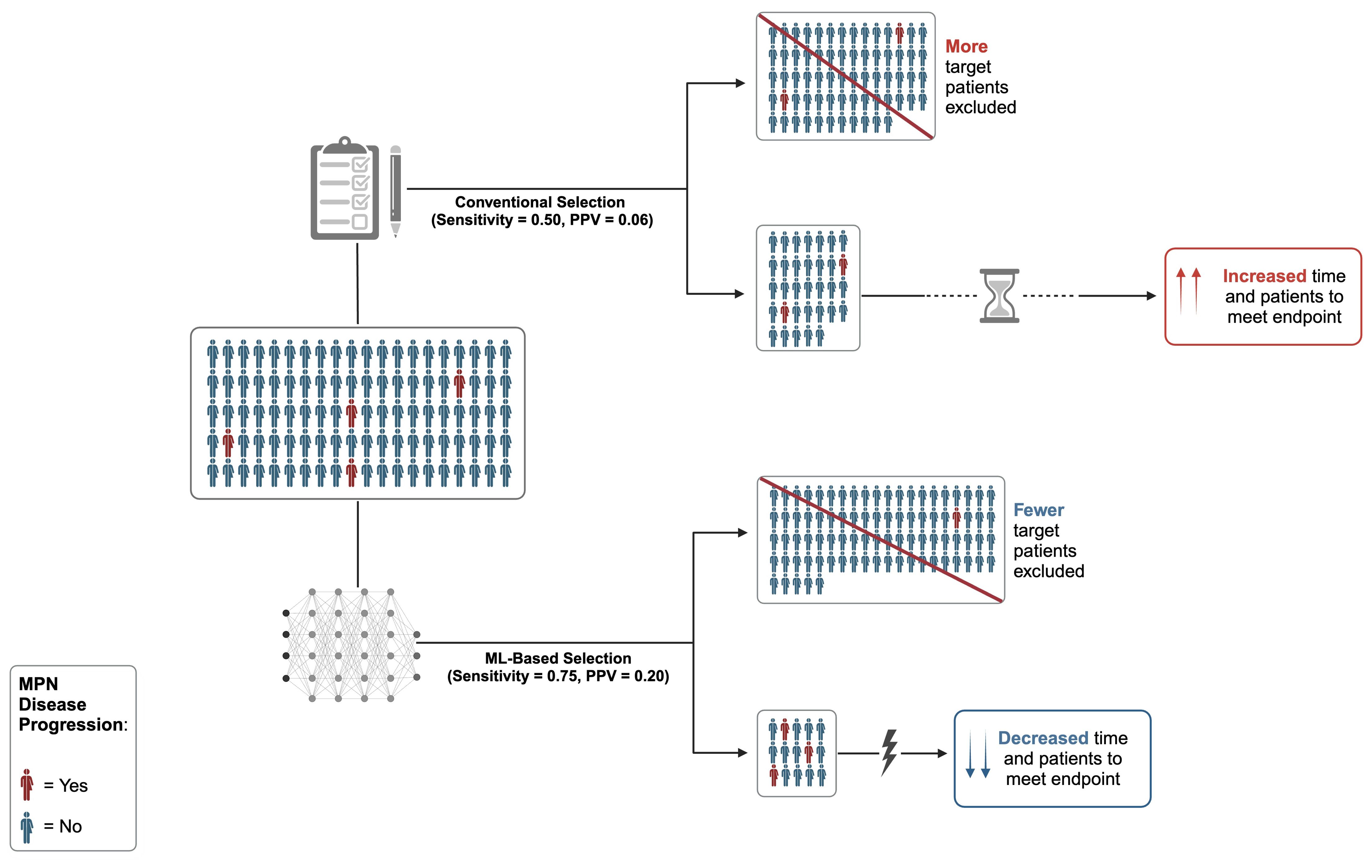
Figure 1. Standard MPN trial enrollment versus efficient MPN trial using artificial intelligence (AI) supported trial matching and machine learning (ML)-based risk stratification model with high positive predictive value (PPV). Cohort size and accrual time dramatically reduced using AI resources and high PPV predictive model. Figure was created in BioRender. Bliss, J. (2024) https://BioRender.com/l21l866.
The CYTO-PV study, which included a broad range of PV patients, reported a thrombosis incidence rate of 2.7% over a 3-year period in the group with stringent hematocrit control (target < 45%) (42). Although this rate is significantly higher than in the general population, it is still too low to justify a 2-year randomized clinical trial (RCT) designed to detect a statistically significant 50% reduction in thrombosis outcomes, which would require thousands of patients. If, however, one can more specifically enrich for those 2.7% who do experience thrombosis using more precise risk models, the required sample size could be much smaller, making it feasible to conduct an RCT targeting thrombosis-free survival.
In this setting, ML stands as a formidable tool in addressing these significant challenges in MPN research. ML techniques can dynamically model population- and patient-level risk using comprehensive datasets, overcoming limitations of traditional methods that often suffer from overfitting and confirmation bias. By objectively identifying actionable risk factors and providing individualized predictions, ML enables a precision oncology approach to modeling progression and event risks.
Introduction to machine learning in clinical research and trials
ML has a broad spectrum of medical applications that extend from image recognition for diagnostic analysis in radiology and pathology, natural language processing (NLP) and large language models (LLM) for the interpretation and transformation of unstructured data within electronic health records (EHR) into research-ready data (43–46). Specific use-cases of ML include leveraging NLP to efficiently transcribe pathology reports and other free-text sub-structured documents from the EHR into data tables for research, which has demonstrated high performance (47–51). Additionally, the analysis of histopathologic whole slide images (WSI) can be used to support classification and prognostication (52–54). In MPNs, ML has the potential to assist clinicians in disease diagnosis, classification, and prognostication (43, 55, 56) while also enabling more precise risk stratification models aimed at improving EFS (e.g. reducing thrombosis, AML transformation, or death) (57, 58).
ML encompasses algorithms designed to predict outcomes accurately, unlike conventional statistical analyses that focus on inferring relationships between covariates (55). In ML, a key distinction exists between classifier and regression algorithms, which differ in the nature of the output variable they are designed to predict. Classifier algorithms are used to predict categorical output variable, such as whether a patient will survive two years post-diagnosis. In contrast, regression models are used to model continuous outcomes, such as estimating median survival time. While classifier algorithms categorize individuals into distinct groups, regression models provide quantitative estimates which can then be discretized, allowing for more nuanced predictions (59).
Additionally, ML models are traditionally classified into supervised and unsupervised categories, though there are other strategies including reinforcement learning and semi-supervised learning. Supervised ML involves creating a model using a training dataset with known labels and then testing it to ensure applicability and generalizability beyond the training subset (43, 55). Supervised algorithms enhance their accuracy by minimizing a loss function (i.e., the discrepancy between expected outcomes and the actual results), which refines model hyperparameters to predict probabilities or continuous values (55). Examples of supervised ML in MPN research include the use of multiple LASSO (Least Absolute Shrinkage and Selection Operator) classifiers, which has now been surpassed by more sophisticated models for prediction tasks such as random forest and support vector machines, which in turn are being surpassed by even more sophisticated deep learning algorithms. An example of supervised ML in this space is our group’s use of a random forest ML models to classify predictors of thrombosis in PV patients (58). Conversely, unsupervised ML is valuable for discovering new data patterns when outcomes are unknown. Unsupervised algorithms undergo training to learn these associations without the need for direct labels. An example in MPN research is the use of Bayesian networks to analyze genetic data from MPN patients and discover genomic groupings within MPNs (60). Several other deep learning techniques have been used in the MPN space (61–66). Moving forward, ML can be leveraged towards two interrelated purposes: to optimize clinical trial matching algorithms and develop more accurate risk stratification systems that target survival endpoints more robustly.
Broadly, clinical trial eligibility criteria are notoriously complex and non-standardized, making patient screening a manual and inefficient endeavor (67). Owing to this, it is no surprise that less than 3% of oncology patients participate in RCTs (68) and about 20% of phase II-III oncology trials fail due to poor accrual (69). A recent survey found that the median duration from study planning to initiation exceeds 700 days (70) with recruitment resources costing approximately $1.2 billion in research spending and consuming up to 30% of drug development timelines (71). Traditional clinical trial design and accrual often fail to capture patient population complexity and heterogeneity (72). Recent efforts to validate ML algorithms for trial matching, such as IBM Watson for matching patients to breast and lung cancer trials, has shown high accuracy and positive predictive value (73–75). For example, in breast cancer, the Clinical Trial Matching Clinical Decision Support System (CDSS) achieved over 80% accuracy (75), while IBM Watson’s model achieved 91.6% accuracy in lung cancer trials [64], demonstrating its effectiveness in matching thousands of patient metrics with eligibility criteria in just 15.5 seconds per patient.
Beyond this, open-source tools have been created with promising results, including trial matching for pediatric leukemia patients and studies on ClinicalTrials.gov (76). Private entities have made strides in improving trial eligibility through ML (73, 77–82). Liu et al. utilized advanced statistical approaches, known as Trial Pathfinder, on data from over 60,000 patients with advanced non-small cell lung cancer to assess how individual features impact machine learning predictions (83). There are two primary ML-based methodologies for matching patients to appropriate clinical trials. The first, often referred to as the “structure-then-match” approach, involves restructuring eligibility criteria into a standardized format (76, 84–86). This allows for direct comparison with patient data, streamlining the initial screening process. Conversely, “end-to-end” systems leverage ML to identify patterns within both patient data and eligibility criteria (87). These patterns are then used to directly match patients with relevant trials, improving efficiency. Together, these examples illustrate a future where clinical trial leaders might soften specific criteria to streamline recruitment without compromising key trial endpoints.
Beyond improving trial matching efficiency, ML can enhance MPN risk stratification strategies to identify patients most likely to benefit from the intervention. Outcomes for trials making it to completion are disappointing especially given the high resource utilization. For example, among the top ten highest-grossing drugs in the U.S., for each patient who benefits from an approved drug, 3-24 patients do not benefit (72). In MPNs, the five FDA approved drugs – four of which are JAKi – were approved based on SVR, symptoms benefit, and/or hematologic response, but none were powered to evaluate survival benefit. Despite some post-hoc evidence of survival benefit, JAKi drugs are not used with the objective of preventing disease progression or prolonging life. In contrast, some oncology drugs fail to receive approval for not improving OS, despite improving PFS and other drugs are approved for long-term use based on preventing recurrence but without OS benefit. An example of the latter is breast cancer endocrine therapy, where extended use of aromatase inhibitors (e.g. 10 vs 5 years) has shown significant benefits in preventing recurrence, despite no impact on overall survival (5-year OS 93% (95% CI, 92 – 95) for letrozole and 94% (95% CI, 92 – 95) for placebo (HR 0.97; P = 0.83)) (88). The integration of ML in this area for MPNs is therefore of burgeoning interest, as it could enhance the accuracy of identifying patients who are most likely to benefit from long-term therapies and improve overall treatment outcomes.
ML carries the power to revolutionize clinical trial eligibility criteria and improve risk stratification by freely exploring robust data to gain a deeper understanding of clinical characteristics associated with outcomes of interest, leading to more targeted, efficient trials and better patient outcomes. Our approach aims to capitalize on big data and ML to develop accurate predictive models, making clinical trials powered to evaluate survival endpoints more feasibly.
Discussion and future directions on ML in MPN research and clinical trials
As previously mentioned, the heterogeneity and complexity of MPNs has posed significant challenges to the accurate prognostication of disease progression, morbidity, treatment response, and mortality. The application of ML in MPN research and clinical practice is emerging as an engine of discovery and the future of the field. The utility of ML in MPN diagnostics and drug discovery have been described elsewhere (89–94). Here, we look at the use of ML in MPN prognostication and its deployment for clinical trial design and accrual (Figure 1).
There have been some advancements in the use of ML systems in characterizing MPN progression. Bejan et al. (95) developed an algorithm to classify MF using NLP with negation detection of MF keywords, medications, and ICD coding, enriched with a separate algorithm to identify patients tested for JAK2V617F in the Synthetic Derivative de-identified research EHR. The group was able to predict MF and JAK2V617F status, showing the feasibility of creating a MPN database with retrospective genotyping of biobanked DNA. Li et al. used weighted gene co-expression network analysis (WGCNA) to identify genes associated with primary MF, which ended up including MPL, SLC4A1, CALR, and EPB42 (96). A support vector machine demonstrated high reliability with AUCs up to 0.922 (96). Shen et al. applied a LASSO model to the prediction of secondary MF using platelet transcriptome studies, demonstrating a proof-of-principle for disease risk stratification and progression (94). Ryou et al. developed a ML system to measure bone marrow reticulin fibrosis, a continuous index of fibrosis (CIF), which demonstrated excellent predictive accuracy when paired with megakaryocyte analysis in distinguishing between ET and pre-fibrotic MF (AUC 0.94) (52) and was applied in the analysis of outcomes of a phase II clinical trial (97). Verstovsek et al. developed a random survival forest (RSF) model to predict hydroxyurea resistance (98). The composite ROC-AUC was 0.71, suggesting that accessible clinical variables could be used to predict the likelihood of patients developing resistance to hydroxyurea prior to starting therapy (98). ML has also been used to assess likelihood of treatment resistance in other malignancies (99, 100) as well as prediction of drug synergy (101). Mora et al. (102) applied a RSF model which incorporated both phenotypic and genotypic variables at time of secondary MF diagnosis in the MYSEC PM (Myelofibrosis Secondary to PV and ET-Prognostic Model) database to identify predictors of thrombosis. The authors showed the model was able to predict thrombotic risk following secondary MF diagnosis.
Given the current exponential trajectory of ML development and its proposed uses in MPN research and treatment, the development of robust risk stratification models is critical. Our overarching solution to the challenges of powering MPN trials for survival is to capitalize on large data and ML capabilities to develop accurate predictive models for survival that would enrich for high-risk patients. To include automated workflows and incorporate ML approaches for big data analyses, our group has established an MPN-focused Research Data Repository (RDR) that consolidates meticulously curated information from our MPN-specific Research Electronic Data Capture (REDCap) databases, integrates both raw and processed data from EHRs, and incorporates data from external entities, such as the CDC’s National Death Index. This comprehensive collection encompasses all pertinent clinical, laboratory, and outcomes data, systematically organized in accordance with the Observational Medical Outcomes Partnership’s Common Data Model (OMOP-CDM) (103). The aim is to use these big data and informatic tools for development and global validation of ML-based risk prediction models.
If a ML model is rigorously validated, attention can be turned to ML-driven MPN clinical trials focused on the use of such models for targeted and efficient patient accrual. The ability to identify patients at highest risk for the clinical endpoint of interest (e.g. resistance to first line therapy, MPN associated thromboembolism, disease progression to MF or leukemia, etc.) will allow clinical investigators to perform trial accrual more rapidly and accelerate the time to events of interest. This should significantly reduce the time and cost of research in a field that has thus far been held back by these challenges secondary to the innate characteristics of MPNs.
In this paper, we have explored the role of machine learning as a transformative force in the clinical research landscape for MPNs. The inherent complexity and chronicity of MPNs pose substantial barriers in patient diagnosis, prognostication, and therapeutic interventions. ML offers a promising solution, with its capacity to effectively sift through vast datasets and unearth patterns that may elude conventional analysis. The prospective application of ML to enhance patient stratification and predict disease trajectories in MPNs is particularly noteworthy. As ML algorithms grow more sophisticated and undergo external validation, their integration into clinical trials will allow for accurate prognostication of MPNs, streamline patient selection for trials, assess efficacy of new therapeutic strategies more efficiently, and advance our ability to improve MPN morbidity and mortality. The future of MPN research and treatment is set to be deeply intertwined with the advancements in ML, promising a new era of personalized medicine that optimizes care for patients with these challenging malignancies.
Author contributions
JB: Conceptualization, Data curation, Investigation, Methodology, Writing – original draft, Writing – review & editing. SK: Conceptualization, Investigation, Methodology, Supervision, Writing – review & editing. JS: Conceptualization, Supervision, Writing – review & editing. GA-Z: Conceptualization, Investigation, Methodology, Project administration, Supervision, Writing – original draft, Writing – review & editing.
Funding
The author(s) declare financial support was received for the research, authorship, and/or publication of this article. GA-Z received relevant funding from the American Society of Hematology Junior Scholar Award (ASH).
Acknowledgments
We thank the American Society of Hematology (ASH), and the David L. Johns Family of the Cancer Research & Treatment Fund (CR&T) for research funding support.
Conflict of interest
The authors declare that the research was conducted in the absence of any commercial or financial relationships that could be construed as a potential conflict of interest.
Generative AI statement
The author(s) declare that no Generative AI was used in the creation of this manuscript.
Publisher’s note
All claims expressed in this article are solely those of the authors and do not necessarily represent those of their affiliated organizations, or those of the publisher, the editors and the reviewers. Any product that may be evaluated in this article, or claim that may be made by its manufacturer, is not guaranteed or endorsed by the publisher.
References
1. Spivak JL. Myeloproliferative Neoplasms. N Engl J Med. (2017) 376:2168–81. doi: 10.1056/NEJMra1406186
2. Tefferi A, Barosi G, Mesa RA, Cervantes F, Deeg HJ, Reilly JT, et al. International Working Group (IWG) consensus criteria for treatment response in myelofibrosis with myeloid metaplasia, for the IWG for Myelofibrosis Research and Treatment (IWG-MRT). Blood. (2006) 108:1497–503. doi: 10.1182/blood-2006-03-009746
3. Arber DA, Orazi A, Hasserjian RP, Borowitz MJ, Calvo KR, Kvasnicka H-M, et al. International Consensus Classification of Myeloid Neoplasms and Acute Leukemias: integrating morphologic, clinical, and genomic data. Blood. (2022) 140:1200–28. doi: 10.1182/blood.2022015850
4. Tefferi A, Guglielmelli P, Larson DR, Finke C, Wassie EA, Pieri L, et al. Long-term survival and blast transformation in molecularly annotated essential thrombocythemia, polycythemia vera, and myelofibrosis. Blood. (2014) 124:2507–13. doi: 10.1182/blood-2014-05-579136
5. Grinfeld J, Nangalia J, Baxter EJ, Wedge DC, Angelopoulos N, Cantrill R, et al. Classification and personalized prognosis in myeloproliferative neoplasms. N Engl J Med. (2018) 379:1416–30. doi: 10.1056/NEJMoa1716614
6. Adams PD, Jasper H, Rudolph KL. Aging-induced stem cell mutations as drivers for disease and cancer. Cell Stem Cell. (2015) 16:601–12. doi: 10.1016/j.stem.2015.05.002
7. Barbui T, Thiele J, Passamonti F, Rumi E, Boveri E, Ruggeri M, et al. Survival and disease progression in essential thrombocythemia are significantly influenced by accurate morphologic diagnosis: an international study. J Clin Oncol. (2011) 29:3179–84. doi: 10.1200/JCO.2010.34.5298
8. Boiocchi L, Gianelli U, Iurlo A, Fend F, Bonzheim I, Cattaneo D, et al. Neutrophilic leukocytosis in advanced stage polycythemia vera: hematopathologic features and prognostic implications. Mod Pathol. (2015) 28:1448–57. doi: 10.1038/modpathol.2015.100
9. Larsen MK, Skov V, Kjær L, Eickhardt-Dalbøge CS, Knudsen TA, Kristiansen MH, et al. Neutrophil-to-lymphocyte ratio and all-cause mortality with and without myeloproliferative neoplasms—a Danish longitudinal study. Blood Cancer J. (2024) 14:1–12. doi: 10.1038/s41408-024-00994-z
10. Abu-Zeinah G, Erdos K, Lee N, Lebbe A, Bouhali I, Khalid M, et al. Are thrombosis, progression, and survival in ET predictable? Blood Cancer J. (2024) 14:1–3. doi: 10.1038/s41408-024-01079-7
11. Milosevic Feenstra JD, Nivarthi H, Gisslinger H, Leroy E, Rumi E, Chachoua I, et al. Whole-exome sequencing identifies novel MPL and JAK2 mutations in triple-negative myeloproliferative neoplasms. Blood. (2016) 127:325–32. doi: 10.1182/blood-2015-07-661835
12. Luque Paz D, Kralovics R, Skoda RC. Genetic basis and molecular profiling in myeloproliferative neoplasms. Blood. (2023) 141:1909–21. doi: 10.1182/blood.2022017578
13. Tefferi A, Nicolosi M, Mudireddy M, Lasho TL, Gangat N, Begna KH, et al. Revised cytogenetic risk stratification in primary myelofibrosis: analysis based on 1002 informative patients. Leukemia. (2018) 32:1189–99. doi: 10.1038/s41375-018-0018-z
14. Chatain N, Koschmieder S, Jost E. Role of inflammatory factors during disease pathogenesis and stem cell transplantation in myeloproliferative neoplasms. Cancers. (2020) 12:2250. doi: 10.3390/cancers12082250
15. Barbui T, Finazzi G, Carobbio A, Thiele J, Passamonti F, Rumi E, et al. Development and validation of an International Prognostic Score of thrombosis in World Health Organization–essential thrombocythemia (IPSET-thrombosis). Blood. (2012) 120:5128–33. doi: 10.1182/blood-2012-07-444067
16. Tefferi A, Loscocco GG, Farrukh F, Szuber N, Mannelli F, Pardanani A, et al. A globally applicable “triple A” risk model for essential thrombocythemia based on Age, Absolute neutrophil count, and Absolute lymphocyte count. Am J Hematol. (2023) 98:1829–37. doi: 10.1002/ajh.27079
17. Gangat N, Caramazza D, Vaidya R, George G, Begna K, Schwager S, et al. DIPSS plus: a refined dynamic international prognostic scoring system for primary myelofibrosis that incorporates prognostic information from karyotype, platelet count, and transfusion status. J Clin Oncol. (2011) 29:392–7. doi: 10.1200/JCO.2010.32.2446
18. Tefferi A, Guglielmelli P, Lasho TL, Coltro G, Finke CM, Loscocco GG, et al. Mutation-enhanced international prognostic systems for essential thrombocythaemia and polycythaemia vera. Br J Haematol. (2020) 189:291–302. doi: 10.1111/bjh.16380
19. Guglielmelli P, Lasho TL, Rotunno G, Mudireddy M, Mannarelli C, Nicolosi M, et al. MIPSS70: mutation-enhanced international prognostic score system for transplantation-age patients with primary myelofibrosis. J Clin Oncol. (2018) 36:310–8. doi: 10.1200/JCO.2017.76.4886
20. Tefferi A, Guglielmelli P, Nicolosi M, Mannelli F, Mudireddy M, Bartalucci N, et al. GIPSS: genetically inspired prognostic scoring system for primary myelofibrosis. Leukemia. (2018) 32:1631–42. doi: 10.1038/s41375-018-0107-z
21. Abu-Zeinah G, Silver RT, Abu-Zeinah K, Scandura JM. Normal life expectancy for polycythemia vera (PV) patients is possible. Leukemia. (2022) 36:569–72. doi: 10.1038/s41375-021-01447-3
22. Tremblay D, Srisuwananukorn A, Ronner L, Podoltsev N, Gotlib J, Heaney ML, et al. European LeukemiaNet Response Predicts Disease Progression but Not Thrombosis in Polycythemia Vera. HemaSphere. (2022) 6:e721. doi: 10.1097/HS9.0000000000000721
23. Savona MR, Malcovati L, Komrokji R, Tiu RV, Mughal TI, Orazi A, et al. An international consortium proposal of uniform response criteria for myelodysplastic/myeloproliferative neoplasms (MDS/MPN) in adults. Blood. (2015) 125:1857–65. doi: 10.1182/blood-2014-10-607341
24. Verstovsek S, Mesa RA, Gotlib J, Levy RS, Gupta V, DiPersio JF, et al. A double-blind, placebo-controlled trial of ruxolitinib for myelofibrosis. N Engl J Med. (2012) 366:799–807. doi: 10.1056/NEJMoa1110557
25. Harrison C, Kiladjian J-J, Al-Ali HK, Gisslinger H, Waltzman R, Stalbovskaya V, et al. JAK Inhibition with Ruxolitinib versus best available therapy for myelofibrosis. N Engl J Med. (2012) 366:787–98. doi: 10.1056/NEJMoa1110556
26. Verstovsek S, Gotlib J, Mesa RA, Vannucchi AM, Kiladjian J-J, Cervantes F, et al. Long-term survival in patients treated with ruxolitinib for myelofibrosis: COMFORT-I and -II pooled analyses. J Hematol OncolJ Hematol Oncol. (2017) 10:156. doi: 10.1186/s13045-017-0527-7
27. Yacoub A, Mascarenhas J, Kosiorek H, Prchal JT, Berenzon D, Baer MR, et al. Pegylated interferon alfa-2a for polycythemia vera or essential thrombocythemia resistant or intolerant to hydroxyurea. Blood. (2019) 134:1498–509. doi: 10.1182/blood.2019000428
28. Gisslinger H, Klade C, Georgiev P, Krochmalczyk D, Gercheva-Kyuchukova L, Egyed M, et al. Ropeginterferon alfa-2b versus standard therapy for polycythaemia vera (PROUD-PV and CONTINUATION-PV): a randomised, non-inferiority, phase 3 trial and its extension study. Lancet Haematol. (2020) 7:e196–208. doi: 10.1016/S2352-3026(19)30236-4
29. Kiladjian J-J, Klade C, Georgiev P, Krochmalczyk D, Gercheva-Kyuchukova L, Egyed M, et al. Long-term outcomes of polycythemia vera patients treated with ropeginterferon Alfa-2b. Leukemia. (2022) 36:1408–11. doi: 10.1038/s41375-022-01528-x
30. Gisslinger H, Klade C, Georgiev P, Krochmalczyk D, Gercheva-Kyuchukova L, Egyed M, et al. Event-free survival in patients with polycythemia vera treated with ropeginterferon alfa-2b versus best available treatment. Leukemia. (2023) 37:2129–32. doi: 10.1038/s41375-023-02008-6
31. Guglielmelli P, Loscocco GG, Mannarelli C, Rossi E, Mannelli F, Ramundo F, et al. JAK2V617F variant allele frequency >50% identifies patients with polycythemia vera at high risk for venous thrombosis. Blood Cancer J. (2021) 11:199. doi: 10.1038/s41408-021-00581-6
32. Moliterno AR, Kaizer H, Reeves BN. JAK2V617F allele burden in polycythemia vera: burden of proof. Blood. (2023) 141:1934–42. doi: 10.1182/blood.2022017697
33. Kiladjian J-J, Zachee P, Hino M, Pane F, Masszi T, Harrison CN, et al. Long-term efficacy and safety of ruxolitinib versus best available therapy in polycythaemia vera (RESPONSE): 5-year follow up of a phase 3 study. Lancet Haematol. (2020) 7:e226–37. doi: 10.1016/S2352-3026(19)30207-8
34. Passamonti F, Palandri F, Saydam G, Callum J, Devos T, Guglielmelli P, et al. Ruxolitinib versus best available therapy in inadequately controlled polycythaemia vera without splenomegaly (RESPONSE-2): 5-year follow up of a randomised, phase 3b study. Lancet Haematol. (2022) 9:e480–92. doi: 10.1016/S2352-3026(22)00102-8
35. Harrison CN, Nangalia J, Boucher R, Jackson A, Yap C, O’Sullivan J, et al. Ruxolitinib Versus Best Available Therapy for Polycythemia Vera Intolerant or Resistant to Hydroxycarbamide in a Randomized Trial. J Clin Oncol. (2023) 41:3534–44. doi: 10.1200/JCO.22.01935
36. Guglielmelli P, Mora B, Gesullo F, Mannelli F, Loscocco GG, Signori L, et al. Clinical impact of mutated JAK2 allele burden reduction in polycythemia vera and essential thrombocythemia. Am J Hematol. (2024), 1550–9. doi: 10.1002/ajh.27400
37. Chen C-C, Chen JL, Lin AJ-H, Yu LH-L, Hou H-A. Association of JAK2V617F allele burden and clinical correlates in polycythemia vera: a systematic review and meta-analysis. Ann Hematol. (2024) 103:1947–65. doi: 10.1007/s00277-024-05754-4
38. Abu-Zeinah G, Di Giandomenico S, Choi D, Cruz T, Erdos K, Taylor E III, et al. Hematopoietic fitness of JAK2V617F myeloproliferative neoplasms is linked to clinical outcome. Blood Adv. (2022) 6:5477–81. doi: 10.1182/bloodadvances.2022007128
39. Patel KP, Newberry KJ, Luthra R, Jabbour E, Pierce S, Cortes J, et al. Correlation of mutation profile and response in patients with myelofibrosis treated with ruxolitinib. Blood. (2015) 126:790–7. doi: 10.1182/blood-2015-03-633404
40. Koppikar P, Bhagwat N, Kilpivaara O, Manshouri T, Adli M, Hricik T, et al. Heterodimeric JAK–STAT activation as a mechanism of persistence to JAK2 inhibitor therapy. Nature. (2012) 489:155–9. doi: 10.1038/nature11303
41. Barosi G, Mesa R, Finazzi G, Harrison C, Kiladjian J-J, Lengfelder E, et al. Revised response criteria for polycythemia vera and essential thrombocythemia: an ELN and IWG-MRT consensus project. Blood. (2013) 121:4778–81. doi: 10.1182/blood-2013-01-478891
42. Marchioli R, Finazzi G, Specchia G, Cacciola R, Cavazzina R, Cilloni D, et al. Cardiovascular events and intensity of treatment in polycythemia vera. N Engl J Med. (2013) 368:22–33. doi: 10.1056/NEJMoa1208500
43. Nagy M, Radakovich N, Nazha A. machine learning in oncology: what should clinicians know? JCO Clin Cancer Inform. (2020). doi: 10.1200/CCI.20.00049
44. Miotto R, Wang F, Wang S, Jiang X, Dudley JT. Deep learning for healthcare: review, opportunities and challenges. Brief Bioinform. (2018) 19:1236–46. doi: 10.1093/bib/bbx044
45. Wu S, Roberts K, Datta S, Du J, Ji Z, Si Y, et al. Deep learning in clinical natural language processing: a methodical review. J Am Med Inform Assoc. (2020) 27:457–70. doi: 10.1093/jamia/ocz200
46. Lee P, Bubeck S, Petro J. Benefits, limits, and risks of GPT-4 as an ai chatbot for medicine. N Engl J Med. (2023) 388:1233–9. doi: 10.1056/NEJMsr2214184
47. Zaccaria GM, Colella V, Colucci S, Clemente F, Pavone F, Vegliante MC, et al. Electronic case report forms generation from pathology reports by ARGO, automatic record generator for onco-hematology. Sci Rep. (2021) 11:23823. doi: 10.1038/s41598-021-03204-z
48. Odisho AY, Park B, Altieri N, DeNero J, Cooperberg MR, Carroll PR, et al. Natural language processing systems for pathology parsing in limited data environments with uncertainty estimation. JAMIA Open. (2020) 3:431–8. doi: 10.1093/jamiaopen/ooaa029
49. Gholipour M, Khajouei R, Amiri P, Hajesmaeel Gohari S, Ahmadian L. Extracting cancer concepts from clinical notes using natural language processing: a systematic review. BMC Bioinf. (2023) 24:405. doi: 10.1186/s12859-023-05480-0
50. Sholle E, Krichevsky S, Scandura J, Sosner C, Campion TR. Lessons learned in the development of a computable phenotype for response in myeloproliferative neoplasms. IEEE Int Conf Healthc Inform IEEE Int Conf Healthc Inform. (2018) 2018:328–31. doi: 10.1109/ICHI.2018.00045
51. Fu JT, Sholle E, Krichevsky S, Scandura J, Campion TR. Extracting and classifying diagnosis dates from clinical notes: A case study. J BioMed Inform. (2020) 110:103569. doi: 10.1016/j.jbi.2020.103569
52. Ryou H, Sirinukunwattana K, Aberdeen A, Grindstaff G, Stolz BJ, Byrne H, et al. Continuous indexing of fibrosis (CIF): improving the assessment and classification of MPN patients. Leukemia. (2023) 37:348–58. doi: 10.1038/s41375-022-01773-0
53. Aeffner F, Zarella MD, Buchbinder N, Bui MM, Goodman MR, Hartman DJ, et al. Introduction to digital image analysis in whole-slide imaging: a white paper from the digital pathology association. J Pathol Inform. (2019) 10:9. doi: 10.4103/jpi.jpi_82_18
54. Krichevsky S, Ouseph MM, Zhang Y, Abu-Zeinah G, Scandura JM, Gupta R. A deep learning-based pathomics methodology for quantifying and characterizing nucleated cells in the bone marrow microenvironment. Blood. (2023) 142:2294. doi: 10.1182/blood-2023-191272
55. Sidey-Gibbons JAM, Sidey-Gibbons CJ. Machine learning in medicine: a practical introduction. BMC Med Res Methodol. (2019) 19:64. doi: 10.1186/s12874-019-0681-4
56. Shahid AH, Singh MP. Computational intelligence techniques for medical diagnosis and prognosis: Problems and current developments. Biocybern BioMed Eng. (2019) 39:638–72. doi: 10.1016/j.bbe.2019.05.010
57. Manz K, Bahr J, Ittermann T, Döhner K, Koschmieder S, Brümmendorf TH, et al. Validation of myeloproliferative neoplasms associated risk factor RDW as predictor of thromboembolic complications in healthy individuals: analysis on 6849 participants of the SHIP-study. Leukemia. (2023) 37:1745–9. doi: 10.1038/s41375-023-01943-8
58. Abu-Zeinah G, Krichevsky S, Silver RT, Taylor E, Tremblay D, Srisuwananukorn A, et al. A novel machine learning-derived dynamic scoring system predicts risk of thrombosis in polycythemia vera (PV) patients. Blood. (2021) 138:3619. doi: 10.1182/blood-2021-149098
59. Sarker IH. Machine learning: algorithms, real-world applications and research directions. SN Comput Sci. (2021) 2:160. doi: 10.1007/s42979-021-00592-x
60. Angelopoulos N, Chatzipli A, Nangalia J, Maura F, Campbell PJ. Bayesian networks elucidate complex genomic landscapes in cancer. Commun Biol. (2022) 5:1–11. doi: 10.1038/s42003-022-03243-w
61. Nielsen FS, Pedersen MJ, Olsen MV, Larsen MS, Røge R, Jørgensen AS. Automatic Bone Marrow Cellularity Estimation in H&E Stained Whole Slide Images. Cytometry A. (2019) 95:1066–74. doi: 10.1002/cyto.a.23885
62. van Eekelen L, Pinckaers H, van den Brand M, Hebeda KM, Litjens G. Using deep learning for quantification of cellularity and cell lineages in bone marrow biopsies and comparison to normal age-related variation. Pathol (Phila). (2022) 54:318–27. doi: 10.1016/j.pathol.2021.07.011
63. Sirinukunwattana K, Aberdeen A, Theissen H, Sousos N, Psaila B, Mead AJ, et al. Artificial intelligence–based morphological fingerprinting of megakaryocytes: a new tool for assessing disease in MPN patients. Blood Adv. (2020) 4:3284–94. doi: 10.1182/bloodadvances.2020002230
64. Hagiya AS, Etman A, Siddiqi IN, Cen S, Matcuk GR Jr., Brynes RK, et al. Digital image analysis agrees with visual estimates of adult bone marrow trephine biopsy cellularity. Int J Lab Hematol. (2018) 40:209–14. doi: 10.1111/ijlh.12768
65. Wang C-W, Huang S-C, Lee Y-C, Shen Y-J, Meng S-I, Gaol JL. Deep learning for bone marrow cell detection and classification on whole-slide images. Med Image Anal. (2022) 75:102270. doi: 10.1016/j.media.2021.102270
66. D’Abbronzo G, D’Antonio A, De Chiara A, Panico L, Sparano L, Diluvio A, et al. Development of an artificial-intelligence-based tool for automated assessment of cellularity in bone marrow biopsies in ph-negative myeloproliferative neoplasms. Cancers. (2024) 16:1687. doi: 10.3390/cancers16091687
67. Ross J, Tu S, Carini S, Sim I. Analysis of eligibility criteria complexity in clinical trials. Summit Transl Bioinforma. (2010) 2010:46–50.
68. Unger JM, Vaidya R, Hershman DL, Minasian LM, Fleury ME. Systematic review and meta-analysis of the magnitude of structural, clinical, and physician and patient barriers to cancer clinical trial participation. JNCI J Natl Cancer Inst. (2019) 111:245–55. doi: 10.1093/jnci/djy221
69. Stensland KD, McBride RB, Latif A, Wisnivesky J, Hendricks R, Roper N, et al. Adult cancer clinical trials that fail to complete: an epidemic? JNCI J Natl Cancer Inst. (2014) 106:dju229. doi: 10.1093/jnci/dju229
70. Kelly D, Spreafico A, Siu LL. Increasing operational and scientific efficiency in clinical trials. Br J Cancer. (2020) 123:1207–8. doi: 10.1038/s41416-020-0990-8
71. Chaudhari N, Ravi R, Gogtay NJ, Thatte UM. Recruitment and retention of the participants in clinical trials: Challenges and solutions. Perspect Clin Res. (2020) 11:64–9. doi: 10.4103/picr.PICR_206_19
72. Schork NJ. Personalized medicine: Time for one-person trials. Nature. (2015) 520:609–11. doi: 10.1038/520609a
73. Alexander M, Solomon B, Ball DL, Sheerin M, Dankwa-Mullan I, Preininger AM, et al. Evaluation of an artificial intelligence clinical trial matching system in Australian lung cancer patients. JAMIA Open. (2020) 3:209–15. doi: 10.1093/jamiaopen/ooaa002
74. Helgeson J, Rammage M, Urman A, Roebuck MC, Coverdill S, Pomerleau K, et al. Clinical performance pilot using cognitive computing for clinical trial matching at Mayo Clinic. J Clin Oncol. (2018) 36:e18598–8. doi: 10.1200/JCO.2018.36.15_suppl.e18598
75. Haddad T, Helgeson JM, Pomerleau KE, Preininger AM, Roebuck MC, Dankwa-Mullan I, et al. Accuracy of an Artificial Intelligence System for Cancer Clinical Trial Eligibility Screening: Retrospective Pilot Study. JMIR Med Inform. (2021) 9:e27767. doi: 10.2196/27767
76. Yuan C, Ryan PB, Ta C, Guo Y, Li Z, Hardin J, et al. Criteria2Query: a natural language interface to clinical databases for cohort definition. J Am Med Inform Assoc. (2019) 26:294–305. doi: 10.1093/jamia/ocy178
77. Inc PH. Paradigm | Home . Available at: https://www.paradigm.inc (Accessed November 15, 2023).
78. Deep 6 AI. Deep6.ai . Available at: https://deep6.ai/ (Accessed November 15, 2023).
79. Antidote. Clinical Trial Patient Recruitment | Antidote . Available at: https://www.antidote.me (Accessed November 15, 2023).
80. Mendel AI - Know More, Know Now . Available at: https://www.mendel.ai/ (Accessed November 15, 2023).
81. Cancer Analysis and Clinical Trial Matching. Available at: https://massivebio.com/ (Accessed November 15, 2023).
82. Calaprice-Whitty D, Galil K, Salloum W, Zariv A, Jimenez B. Improving clinical trial participant prescreening with artificial intelligence (ai): a comparison of the results of ai-assisted vs standard methods in 3 oncology trials. Ther Innov Regul Sci. (2019). doi: 10.1177/2168479018815454
83. Liu R, Rizzo S, Whipple S, Pal N, Pineda AL, Lu M, et al. Evaluating eligibility criteria of oncology trials using real-world data and AI. Nature. (2021) 592:629–33. doi: 10.1038/s41586-021-03430-5
84. Weng C, Wu X, Luo Z, Boland MR, Theodoratos D, Johnson SB. EliXR: an approach to eligibility criteria extraction and representation. J Am Med Inform Assoc. (2011) 18:i116–24. doi: 10.1136/amiajnl-2011-000321
85. Kang T, Zhang S, Tang Y, Hruby GW, Rusanov A, Elhadad N, et al. EliIE: An open-source information extraction system for clinical trial eligibility criteria. J Am Med Inform Assoc. (2017) 24:1062–71. doi: 10.1093/jamia/ocx019
86. Bustos A, Pertusa A. Learning eligibility in cancer clinical trials using deep neural networks. Appl Sci. (2018) 8:1206. doi: 10.3390/app8071206
87. Wong C, Zhang S, Gu Y, Moung C, Abel J, Usuyama N, et al. Scaling clinical trial matching using large language models: a case study in oncology. Proc Mach Learn Res. (2023) 2023:1–18. doi: 10.48550/arXiv.2308.02180
88. Goss Paul E, Ingle James N, Pritchard Kathleen I, Robert Nicholas J, Muss H, Gralow J. et al. Extending Aromatase-Inhibitor Adjuvant Ther to 10 Years. N Engl J Med. (2016) 375:209–19. doi: 10.1056/NEJMoa1604700
89. Kimura K, Ai T, Horiuchi Y, Matsuzaki A, Nishibe K, Marutani S, et al. Automated diagnostic support system with deep learning algorithms for distinction of Philadelphia chromosome-negative myeloproliferative neoplasms using peripheral blood specimen. Sci Rep. (2021) 11:3367. doi: 10.1038/s41598-021-82826-9
90. Elsayed B, Elshoeibi AM, Elhadary M, Ferih K, Elsabagh AA, Rahhal A, et al. Applications of Artificial Intelligence in Philadelphia-Negative Myeloproliferative Neoplasms. Diagnostics. (2023) 13:1123. doi: 10.3390/diagnostics13061123
91. Bu Y, Gao R, Zhang B, Zhang L, Sun D. CoGT: Ensemble Machine Learning Method and Its Application on JAK Inhibitor Discovery. ACS Omega. (2023) 8:13232–42. doi: 10.1021/acsomega.3c00160
92. O’Sullivan JM, Mead AJ, Psaila B. Single-cell methods in myeloproliferative neoplasms: old questions, new technologies. Blood. (2023) 141:380–90. doi: 10.1182/blood.2021014668
93. Krishnan A, Du W, Fechter L, Gotlib J, Maecker H, Natu V, et al. Platelet transcriptome yields progressive markers in chronic myeloproliferative neoplasms and identifies putative targets of therapy. Exp Hematol. (2021) 100:S82. doi: 10.1016/j.exphem.2021.12.300
94. Shen Z, Du W, Perkins C, Fechter L, Natu V, Maecker H, et al. Platelet transcriptome identifies progressive markers and potential therapeutic targets in chronic myeloproliferative neoplasms. Cell Rep Med. (2021) 2. doi: 10.1016/j.xcrm.2021.100425
95. Bejan CA, Sochacki A, Zhao S, Xu Y, Savona M. Abstract 5303: Identification of myelofibrosis from electronic health records with novel algorithms and JAKextractor. Cancer Res. (2018) 78:5303. doi: 10.1158/1538-7445.AM2018-5303
96. Li W, Zhao Y, Wang D, Ding Z, Li C, Wang B, et al. Transcriptome research identifies four hub genes related to primary myelofibrosis: a holistic research by weighted gene co-expression network analysis. Aging. (2021) 13:23284–307. doi: 10.18632/aging.203619
97. Ryou H, Sirinukunwattana K, Wood R, Aberdeen A, Rittscher J, Weinberg OK, et al. Quantitative analysis of bone marrow fibrosis highlights heterogeneity in myelofibrosis and augments histological assessment: An Insight from a phase II clinical study of zinpentraxin alfa. HemaSphere. (2024) 8:e105. doi: 10.1002/hem3.105
98. Verstovsek S, Heidel FH, De Stefano V, Zuurman M, Bryan K, Afsharinejad A, et al. Prediction of Resistance to Hydroxyurea Therapy in Patients with Polycythemia Vera: A Machine Learning Study (PV-AIM). Blood. (2022) 140:6823–5. doi: 10.1182/blood-2022-157268
99. Qureshi R, Basit SA, Shamsi JA, Fan X, Nawaz M, Yan H, et al. Machine learning based personalized drug response prediction for lung cancer patients. Sci Rep. (2022) 12:18935. doi: 10.1038/s41598-022-23649-0
100. Ogunleye AZ, Piyawajanusorn C, Gonçalves A, Ghislat G, Ballester PJ. Interpretablemachine learning models to predict the resistance of breast cancer patients to doxorubicin from their microRNA profiles. Adv Sci. (2022) 9:2201501. doi: 10.1002/advs.202201501
101. Baptista D, Ferreira PG, Rocha M. A systematic evaluation of deep learning methods for the prediction of drug synergy in cancer. PloS Comput Biol. (2023) 19:e1010200. doi: 10.1371/journal.pcbi.1010200
102. Mora B, Guglielmelli P, Kuykendall A, Rumi E, Maffioli M, Palandri F, et al. Prediction of thrombosis in post-polycythemia vera and post-essential thrombocythemia myelofibrosis: a study on 1258 patients. Leukemia. (2022) 36:2453–60. doi: 10.1038/s41375-022-01673-3
Keywords: myeloproliferative neoplasms (MPNs), machine learning (ML), artificial intelligence (AI), clinical trials, prognostication, risk stratification, predictive modeling, personalized medicine
Citation: Bliss JW, Krichevsky S, Scandura J and Abu-Zeinah G (2024) Leveraging big data and artificial intelligence for smarter trials in myeloproliferative neoplasms. Front. Hematol. 3:1504327. doi: 10.3389/frhem.2024.1504327
Received: 30 September 2024; Accepted: 03 December 2024;
Published: 24 December 2024.
Edited by:
Adrián Mosquera Orgueira, University Hospital of Santiago de Compostela, SpainReviewed by:
Nikolaos Sousos, University of Oxford, United KingdomCopyright © 2024 Bliss, Krichevsky, Scandura and Abu-Zeinah. This is an open-access article distributed under the terms of the Creative Commons Attribution License (CC BY). The use, distribution or reproduction in other forums is permitted, provided the original author(s) and the copyright owner(s) are credited and that the original publication in this journal is cited, in accordance with accepted academic practice. No use, distribution or reproduction is permitted which does not comply with these terms.
*Correspondence: Ghaith Abu-Zeinah, Z2ZhMjAwMUBtZWQuY29ybmVsbC5lZHU=