- Department of Food Informatics and Computational Science Hub, University of Hohenheim, Stuttgart, Germany
ChatGPT uses a so called Large Language Model (LLM) to provide textual output of analyzed data. Those LLMs are one example for Generative Artificial Intelligence (AI), which focuses on creating new content, e.g., text, images, or music, based on learned patterns. Recently, applications in the food industry and agriculture started to apply Generative AI. This mini review provides an overview about applications of Generative AI in the agri-food supply chain and discusses open research challenges, also in combination with digital twins.
1 Introduction
The release of ChatGPT in November 2022 revolutionized how we interact with technology, making natural language processing more accessible and user-friendly. ChatGPT uses the GPT model, a so-called Large Language Model (LLM). An LLM is an advanced Artificial Intelligence (AI) system trained on vast amounts of text data to understand and generate human-like language. Its potential lies in its ability to perform a wide range of language-related tasks, such as translation, summarization, and content creation, with high accuracy and coherence. LLMs are one prominent example of Generative AI, a branch of AI focused on creating new content, such as text, images, or music, based on patterns and data it has learned.
Recently, the potential of Generative AI has also been seen in the food industry and agriculture. In this mini-review, we want to provide an overview of the applications of Generative AI in the agri-food supply chain. Therefore, a structured literature review was applied to answer the following research questions:
Next, Section 2 provides a detailed description of the methodology for the literature review. Then, Section 3 presents the findings of the study, i.e., the identified applications that use Generative AI. Afterward, Section 4 interprets the results and explores the implications of the findings by deriving open research challenges and summarizing the potential of Generative AI for agri-food applications, also in combination with digital twins. Lastly, Section 5 concludes this paper by summarizing the main findings.
2 Methods and materials
We applied a structured literature review using pre-defined search terms in the databases Scopus, IEEE Xplore, and ACM Digital Library; IEEE and ACM are chosen as important publishers in the field of computer science. The search was applied in July 2024.
The main target of this study is Generative AI. Additionally, LLMs were targeted as another study by Ma et al. (2024) has shown that LLMs are commonly used in food science. The following procedure was applied for the literature review.
First, the following four search terms were applied in all three mentioned libraries (“OR” has a higher binding as “AND” and is interpreted first):
For each search term, we first searched in Scopus before searching in the IEEE and ACM databases. In this order, we also processed the papers. In total, the search using the search terms in the three mentioned libraries resulted in 194 identified publications.
Second, the author of this paper checked the titles and the abstracts. If a paper fits the topic of applying Generative AI in an agriculture or food industry use case, it was added to the list and completely analyzed. Further, already-found elements in one of the other databases or using one of the other search terms were excluded. Also, papers that are not providing original research, e.g., correctums and editorials, are excluded. Papers describing applications in medicine or pharmacy are also excluded. Additionally, some papers use LLMs as abbreviations for other terms, e.g., “low-phytate soybean meal”, “legume leaf meal”, or “Low lactose milk”; such papers were excluded. Lastly, three papers that were not retrievable were excluded. After those checks, 45 papers were remaining.
Third, the author read all remaining papers and extracted the following information, if the paper suited. (1) The exact scope of the paper (food, nutrition, or agriculture) was extracted. (2) The used type of media was extracted. Those were mainly text, some approaches also used or generated pictures. (3) Details about the application were extracted. After analyzing each paper in detail, 17 more papers were excluded as the focus did not fit or Generative AI was not a remarkable aspect of the application. Consequently, 28 papers were remaining and included - the identified applications will be presented in the following Section 3. Table 1 presents an overview of the relevant numbers.
3 Results
This section describes the relevant properties extracted as part of the literature review w.r.t. the domain and the specific applications. Table 2 shows the extracted characteristics of the analyzed papers.
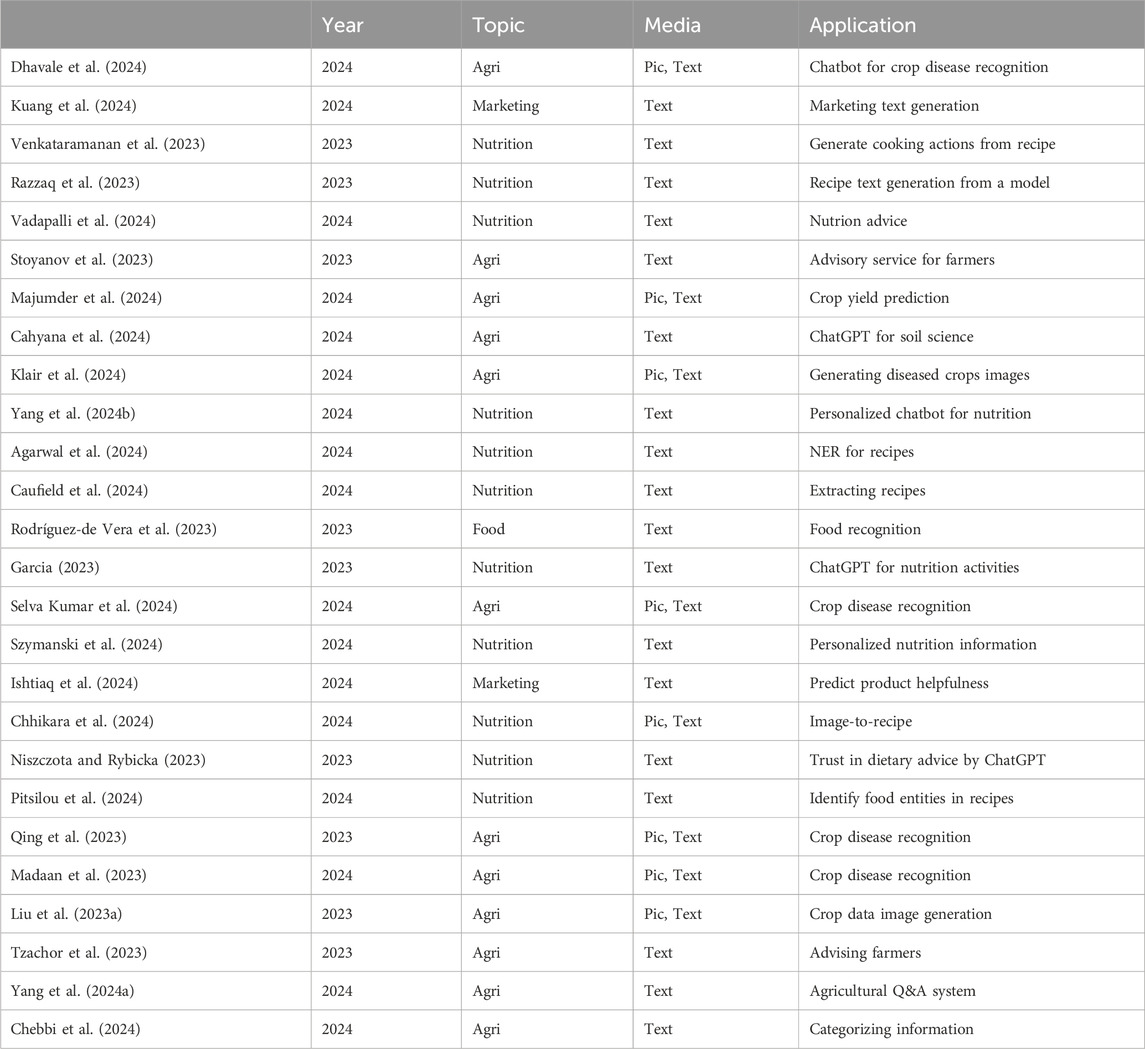
Table 2. Overview on the captured data for the analyzed approaches. Misses the two review articles by Ma et al. (2024) and Liu et al. (2023b). “Pic” refers to pictures.
Please note: Three papers (Ma et al., 2024; Liu et al., 2023b; Yang et al., 2024a) are literature reviews. As the studies by Ma et al. (2024) and Liu et al. (2023b) present not a specific approach, we do not analyze them in this section. However, Yang et al. (2024a) was included as it focused one single type of system rather than providing an overview of different system types. Hence, this is seen as a single system and included in the analysis. Consequently, the following results are derived from the remaining 26 works.
3.1 Applications of generative AI in agriculture
Applications in agriculture often focus on the identification of crop diseases. Here, one can distinguish (i) applications that combine procedures for recognizing picture and text information (Selva Kumar et al., 2024; Qing et al., 2023) from (ii) applications integrating LLMs for recommendations to handle identified diseases (Madaan et al., 2023), also, e.g., as chatbots (Dhavale et al., 2024). As the balancing of data between crop data with diseases and data with healthy crops is a crucial issue, some works describe how to generate data of crop diseases using Generative AI, mainly focusing on generating pictures (Klair et al., 2024; Liu et al., 2023a). Another important topic related to crops is crop yield prediction (Majumder et al., 2024).
A second stream of research focuses on the extraction of information for farmers and other stakeholders from textual data. Those works use LLMs, sometimes pre-trained with specific knowledge, to query the relevant data in potentially very large datasets (Stoyanov et al., 2023; Tzachor et al., 2023; Yang et al., 2024a). Other works use the original implementation of ChatGPT and study the applicability for specific topics, e.g., for questions related to soil science (Cahyana et al., 2024). Chebbi et al. (2024) uses Named Entity Recognition (NER) to optimize the information provision.
3.2 Applications of generative AI in food and nutrition
For nutrition, many use cases are related to food recognition or recipes generation. The first works focus on the application of ChatGPT. Garcia (2023) describes the application of ChatGPT in different activities of the Nutrition Care Process. However, Niszczota and Rybicka (2023) describes the trust issues of consumers for recipes generated by ChatGPT. A second class of works focuses on food recognition, describing the application of (pre-trained) LLMs for recipe generation (Rodríguez-de Vera et al., 2023), also using image-to-recipe techniques (Chhikara et al., 2024). Another stream of research targets the transformation of food models into textual information in the form of recipes using LLMs (Venkataramanan et al., 2023; Razzaq et al., 2023). Important elements of the second and third research streams are techniques for identifying food entities in cooking recipes (Pitsilou et al., 2024), NER Models for recipes (Agarwal et al., 2024), and zero-shot learning for the extraction of food recipes to tackle the issues of missing labels for recipes (Caufield et al., 2024). Lastly, research also focuses on providing individualized recipes and personalized nutrition information (Vadapalli et al., 2024; Szymanski et al., 2024), also using chatbots (Yang et al., 2024b).
Another research stream targets applications in marketing for the food industry. Those include the generation of marketing texts for the Food & Beverage industry (Kuang et al., 2024) and predicting product helpfulness through customer reviews using LLMs (Ishtiaq et al., 2024). Additional applications of LLMs for food science are presented in (Ma et al., 2024).
4 Discussion
This section discusses the findings from the literature review. First, it summarizes the potential of Generative AI for food processing analysis focusing on the main applications, how LLMs are applied, and which type of content is generated and processed. Second, aspects for Generative AI in the agri-food chain which has been insufficiently covered yet in research are described, focusing in the integration of digital twins, explainability of the applied machine learning, and trust issue for the result of the machine learning algorithms. Last, the section states relevant threats to validity.
4.1 Potential of generative AI for the agri-food chain
4.1.1 Main applications
Generative AI supports crop management by accurately identifying and diagnosing crop diseases through advanced image and text recognition techniques, enabling timely and effective interventions. Additionally, it aids in predicting crop yields and optimizing resource allocation, helping farmers make data-driven decisions to enhance productivity and sustainability. Regarding food/nutrition, Generative AI supports recipe generation by creating personalized and innovative recipes based on individual dietary preferences and nutritional needs, enhancing the user experience in meal planning. It also facilitates the generation of recipes and ingredient lists from food images.
4.1.2 Using LLMs
For both identified research streams, Generative AI, mainly LLMs, works “in both directions”: for extracting information from data and for generating text to represent data. So far, the analyzed approaches often use Generative AI for information search or generic models such as GPT for information provision. Especially for communication with humans—consumers, but also other stakeholders in the agri-food chain—Generative AI has large potential, especially to “translate” the content of models, e.g., numeric simulation for food processing. Similar to domain-specific languages, which are specialized programming languages designed to efficiently solve problems within a specific domain (Fowler, 2010), it would be beneficial to use customized LLMs, i.e., pre-train them. Further, food-specific foundation models (e.g., Qi et al., 2023) rather than generic models as GPT might be beneficial here. Further research is required in this direction.
4.1.3 Multi-modal approaches
The analysis approaches mainly use text (and hence LLMs) as a database; only applications for crop disease handling and one application for image-to-recipe extraction apply or generate picture data. Whereas such multi-modal approaches might be challenging, they promise large potential. For example, Klair et al. (2024) and Liu et al. (2023a) describe how to generate data of crop diseases using Generative AI. This is very important as often the amount of such data is not large enough, and collecting additional such data is costly. However, balancing data between data items with relevant anomalies and normal instances is as important for machine learning-based analysis as a high amount of data. Especially, the generation of images is still in its infancy. Especially if very exact representations, e.g., of the crops and the details of the diseases, are required, the image generation needs to be very exact and has less creative freedom. Therefore, commonly available generators, such as DALL-E, are not suitable—individually trained neural networks, e.g., based on Generative Adversarial Networks, are more applicable, cf. Liu et al. (2023a). Here, high potential for future research exists, also in the area of product anomaly detection in food processing.
4.2 Research challenges
4.2.1 Digital (food) twins
Generative AI and digital food twins can be intertwined to create highly accurate and dynamic virtual models of food products and processes (Liu et al., 2023b). Digital twins, which are virtual replicas of physical objects or systems, can use real-time data to simulate and predict food production outcomes (Henrichs et al., 2022), while Generative AI can enhance the generation of these models by interpreting textual data. Generative AI enhances data augmentation and integration in digital (food) twins by creating high-quality, hard-to-collect information, facilitating comprehensive data analysis despite the heterogeneous nature of the data. In virtual simulations, Generative AI enables the creation of diverse scenarios, e.g., allowing farmers to explore various strategies and potential outcomes, thus optimizing decision-making processes and reducing the cost and risk associated with manual scenario design. Additionally, AI-generated content can simplify complex knowledge graph-based reasoning into more practical and understandable recommendations for stakeholders in the agri-food chain, bridging the gap between technical insights and actionable advice - which can also increase users’ trust into those systems. By integrating Generative AI, digital twins can also predict the sensory attributes and shelf life of new food products, ensuring higher quality and consistency. This requires transforming the data streams to categorical textual information. This large potential and wide set of application is not well studied so far.
4.2.2 Explainability
Machine learning in food applications has the potential to revolutionize areas such as personalized nutrition, food safety monitoring, agricultural processes, and food processing operations by analyzing large datasets to predict trends, real-time data analysis to improve efficiency, and autonomous decision-making (Krupitzer and Stein, 2024). However, a significant challenge with machine learning, especially deep learning, is its lack of explainability; the complex and opaque nature of deep learning models often makes it difficult to understand how decisions are made. This “black box” issue can lead to trust and accountability problems, as stakeholders may find it hard to interpret the reasoning behind specific recommendations or actions taken by the system. The synergy between Generative AI and model-based approaches, e.g., combined with digital twins, might also benefit from using Generative AI for communicating the analysis outcomes. For example, rather than solely presenting new machine configurations to the line operators or to farmers, the digital twin could also explain them using Generative AI (Krupitzer et al., 2022). However, this requires Generative AI to understand the model, which again results in the need for food-specific Generative AI models, especially LLMs; additionally, it must be possible to flexibly customize those models with a company’s own data. Of course is the explainability of Generative AI itself another important topic.
4.2.3 Trust
Potential trust issues of users for Generative AI include concerns about the accuracy and reliability of the generated content, as users may fear that AI-produced information could be misleading or incorrect (Niszczota and Rybicka, 2023). In general, there is also apprehension about data privacy and security, as users and companies worry about how their personal information is used and protected by AI systems. Ethical considerations are another significant factor, with users questioning the transparency of AI decision-making processes and the potential for bias in AI-generated outcomes (see the previous paragraph on explainability). Additionally, the lack of human oversight and accountability in AI operations can lead to skepticism, as users might be uncertain about the AI’s ability to handle complex, nuanced situations appropriately. This might be critical in a sensitive domain like food processing, where accountability and law regulations are especially important topics. Finally, the potential misuse of Generative AI for malicious purposes, such as generating fake information, further exacerbates trust issues among users and might be especially an issue when food certification procedures rely on generated text information.
4.3 Threats to validity
Due to its short nature, this mini-review cannot provide a thorough analysis of the topic. Further, the analyzed works could not be analyzed in detail, e.g., comparing the applied techniques of Generative AI. Lastly, additional papers can be found when explicitly searching for ChatGPT, e.g., Zhao et al. (2023); however, this work should not be limited to specific systems.
5 Conclusion
This paper presents an overview of applications of Generative AI in the agri-food chain. In contrast to Ma et al. (2024), the overview is not limited to LLMs. Based on the analysis of 26 works, the most prominent applications are crop disease detection in agriculture and recipes generation for food/nutrition use cases.
The integration of Generative AI for the food industry is still in its infancy. Challenges lie especially in the multimodal integration of various data sources, the need for food-specific models rather than foundation models such as GPT, and potential user trust issues.
However, the potential of Generative AI in agri-food applications is very large, especially for the seamless integration of users with machines. In this regard, Generative AI can help by generating explanations of machine decisions and communicating them to users. Further, it has a large potential in information extraction from large data repositories with textual data.
Author contributions
CK: Conceptualization, Data curation, Formal Analysis, Methodology, Writing–original draft, Writing–review and editing.
Funding
The author(s) declare that financial support was received for the research, authorship, and/or publication of this article.
Acknowledgments
Text elements of the publication have been generated using ChatGPT with the model GPT-4o and intensively checked by the author before being integrated into the document.
Conflict of interest
The author declares that the research was conducted in the absence of any commercial or financial relationships that could be construed as a potential conflict of interest.
Publisher’s note
All claims expressed in this article are solely those of the authors and do not necessarily represent those of their affiliated organizations, or those of the publisher, the editors and the reviewers. Any product that may be evaluated in this article, or claim that may be made by its manufacturer, is not guaranteed or endorsed by the publisher.
References
Agarwal, A., Kapuriya, J., Agrawal, S., Konam, A. V., Goel, M., Gupta, R., et al. (2024). “Deep learning based named entity recognition models for recipes,” in Proceedings of the 2024 Joint International Conference on Computational Linguistics, Language Resources and Evaluation (LREC-COLING 2024), Torino, Italia.
Cahyana, D., Hadiarto, A., Irawan, , Hati, D. P., Pratamaningsih, M. M., Karolinoerita, V., et al. (2024). Application of chatgpt in soil science research and the perceptions of soil scientists in Indonesia. Artif. Intell. Geosciences 5, 100078. doi:10.1016/j.aiig.2024.100078
Caufield, J. H., Hegde, H., Emonet, V., Harris, N. L., Joachimiak, M. P., Matentzoglu, N., et al. (2024). Structured prompt interrogation and recursive extraction of semantics (spires): a method for populating knowledge bases using zero-shot learning. Bioinformatics 40, btae104. doi:10.1093/bioinformatics/btae104
Chebbi, A., Kniesel, G., Abdennadher, N., and Dimarzo, G. (2024). “Enhancing named entity recognition for agricultural commodity monitoring with large language models,” in Proceedings of the 4th Workshop on Machine Learning and Systems, Geneva, Switzerland, 22 April, 2024.
Chhikara, P., Chaurasia, D., Jiang, Y., Masur, O., and Ilievski, F. (2024). “Fire: food image to recipe generation,” in Proceedings of the IEEE/CVF Winter Conference on Applications of Computer Vision (WACV), Waikoloa, HI, USA, 03–08 January, 2024.
Dhavale, C., Pawar, T., Singh, A., Pole, S., and Sabat, K. (2024). “Revolutionizing farming: Gan-enhanced imaging, cnn disease detection, and llm farmer assistant,” in 2024 2nd International Conference on Computer, Communication and Control, Indore, India, 08–10 February, 2024.
Garcia, M. B. (2023). Chatgpt as a virtual dietitian: exploring its potential as a tool for improving nutrition knowledge. Appl. Syst. Innov. 6, 96. doi:10.3390/asi6050096
Henrichs, E., Noack, T., Pinzon Piedrahita, A. M., Salem, M. A., Stolz, J., and Krupitzer, C. (2022). Can a byte improve our bite? an analysis of digital twins in the food industry. Sensors 22, 115. doi:10.3390/s22010115
Ishtiaq, A., Munir, K., Raza, A., Samee, N. A., Jamjoom, M. M., and Ullah, Z. (2024). Product helpfulness detection with novel transformer based bert embedding and class probability features. IEEE Access 12, 55905–55917. doi:10.1109/ACCESS.2024.3390605
Klair, Y. S., Agrawal, K., and Kumar, A. (2024). “Impact of generative ai in diagnosing diseases in agriculture,” in 2024 2nd International Conference on Disruptive Technologies (ICDT), Greater Noida, India, 15–16 March, 2024.
Krupitzer, C., Noack, T., and Borsum, C. (2022). Digital food twins combining data science and food science: system model, applications, and challenges. Processes 10, 1781. doi:10.3390/pr10091781
Krupitzer, C., and Stein, A. (2024). Unleashing the potential of digitalization in the agri-food chain for integrated food systems. Annu. Rev. Food Sci. Technol. 15, 307–328. doi:10.1146/annurev-food-012422-024649
Kuang, A. C. L., Lim, T. M., Tan, C. W., Ho, C. F., and Husaini, N. A. (2024). Ai ads: practicability of text generation for f&b marketing. J. Logist. Inf. Serv. Sci. 11, 324–345. doi:10.33168/JLISS.2024.0220
Liu, B., Zhang, H., Liu, J., and Wang, Q. (2023a). “Acigs: an automated large-scale crops image generation system based on large visual language multi-modal models,” in 2023 20th Annual IEEE International Conference on Sensing, Communication, and Networking (SECON), Madrid, Spain, 11–14 September, 2023.
Liu, J., Zhou, Y., Li, Y., Li, Y., Hong, S., Li, Q., et al. (2023b). “Exploring the integration of digital twin and generative ai in agriculture,” in 2023 15th International Conference on Intelligent Human-Machine Systems and Cybernetics (IHMSC), Hangzhou, China, 26–27 August, 2023.
Ma, P., Tsai, S., He, Y., Jia, X., Zhen, D., Yu, N., et al. (2024). Large language models in food science: innovations, applications, and future. Trends Food Sci. & Technol. 148, 104488. doi:10.1016/j.tifs.2024.104488
Madaan, V., Bindal, G., Singh, S., Yadav, S. K., Singh, A., Sinha, P., et al. (2023). “Integrating language models and machine learning for crop disease detection for farmer guidance,” in Workshop on Advances in Computational Intelligence (ACI-2023) co-located with the 2nd International Conference on Artificial Intelligence and Data Science (ICAIDS-2023), Hyderabad, Telangana, India, 29–30 December, 2023.
Majumder, S., Khandelwal, Y., and K, S. (2024). “Computer vision and generative ai for yield prediction in digital agriculture,” in 2024 2nd International Conference on Networking and Communications (ICNWC).
Niszczota, P., and Rybicka, I. (2023). The credibility of dietary advice formulated by chatgpt: robo-diets for people with food allergies. Nutrition 112, 112076. doi:10.1016/j.nut.2023.112076
Pitsilou, V., Papadakis, G., and Skoutas, D. (2024). “Using llms to extract food entities from cooking recipes,” in 2024 IEEE 40th International Conference on Data Engineering Workshops (ICDEW), Utrecht, Netherlands, 13–16, May 2024.
Qi, Z., Yu, Y., Tu, M., Tan, J., and Huang, Y. (2023). Foodgpt: a large language model in food testing domain with incremental pre-training and knowledge graph prompt. arXiv:2308.10173. doi:10.48550/arXiv.2308.10173
Qing, J., Deng, X., Lan, Y., and Li, Z. (2023). Gpt-aided diagnosis on agricultural image based on a new light yolopc. Comput. Electron. Agric. 213, 108168. doi:10.1016/j.compag.2023.108168
Razzaq, M. S., Maqbool, F., Ilyas, M., and Jabeen, H. (2023). Evorecipes: a generative approach for evolving context-aware recipes. IEEE Access 11, 74148–74164. doi:10.1109/ACCESS.2023.3296144
Rodríguez-de Vera, J. M., Villacorta, P., Estepa, I. G., Bolaños, M., Sarasúa, I., Nagarajan, B., et al. (2023). “Dining on details: llm-guided expert networks for fine-grained food recognition,” in Proceedings of the 8th International Workshop on Multimedia Assisted Dietary Management, 29 October, 2023.
Selva Kumar, S., Khan, A. K. M. A., Banday, I. A., Gada, M., and Shanbhag, V. V. (2024). “Overcoming llm challenges using rag-driven precision in coffee leaf disease remediation,” in 2024 International Conference on Emerging Technologies in Computer Science for Interdisciplinary Applications (ICETCS), Bengaluru, India, 22–23 April, 2024.
Stoyanov, S., Kumurdjieva, M., Tabakova-Komsalova, V., and Doukovska, L. (2023). “Using llms in cyber-physical systems for agriculture - zemela,” in 2023 International Conference on Big Data, Knowledge and Control Systems Engineering (BdKCSE), Bengaluru, India, 22–23 April, 2024.
Szymanski, A., Wimer, B. L., Anuyah, O., Eicher-Miller, H. A., and Metoyer, R. A. (2024). “Integrating expertise in llms: crafting a customized nutrition assistant with refined template instructions,” in Proceedings of the CHI Conference on Human Factors in Computing Systems, Sofia, Bulgaria, 02–03 November, 2023.
Tzachor, A., Devare, M., Richards, C., Pypers, P., Ghosh, A., Koo, J., et al. (2023). Large language models and agricultural extension services. Nat. food 4, 941–948. doi:10.1038/s43016-023-00867-x
Vadapalli, J., Gupta, S., Karki, B., and Tsai, C.-H. (2024). “Incorporating citizen-generated data into large language models,” in Proceedings of the 25th Annual International Conference on Digital Government Research, New York, NY, USA, 11 June, 2024.
Venkataramanan, R., Roy, K., Raj, K., Prasad, R., Zi, Y., Narayanan, V., et al. (2023). “Cook-gen: robust generative modeling of cooking actions from recipes,” in 2023 IEEE International Conference on Systems, Man, and Cybernetics (SMC), Honolulu, Oahu, HI, USA, 01–04 October, 2023.
Yang, T., Mei, Y., Xu, L., Yu, H., and Chen, Y. (2024a). Application of question answering systems for intelligent agriculture production and sustainable management: a review. Resour. Conservation Recycl. 204, 107497. doi:10.1016/j.resconrec.2024.107497
Yang, Z., Khatibi, E., Nagesh, N., Abbasian, M., Azimi, I., Jain, R., et al. (2024b). Chatdiet: empowering personalized nutrition-oriented food recommender chatbots through an llm-augmented framework. Smart Health 32, 100465. doi:10.1016/j.smhl.2024.100465
Keywords: generative artificial intelligence, generative AI, agri-food value chain, food industry, agriculture, nutrition
Citation: Krupitzer C (2024) Generative artificial intelligence in the agri-food value chain - overview, potential, and research challenges. Front. Food. Sci. Technol. 4:1473357. doi: 10.3389/frfst.2024.1473357
Received: 30 July 2024; Accepted: 17 September 2024;
Published: 25 September 2024.
Edited by:
Carlos M. Corvalan, Purdue University, United StatesReviewed by:
Dominik Geier, Technical University of Munich, GermanyCopyright © 2024 Krupitzer. This is an open-access article distributed under the terms of the Creative Commons Attribution License (CC BY). The use, distribution or reproduction in other forums is permitted, provided the original author(s) and the copyright owner(s) are credited and that the original publication in this journal is cited, in accordance with accepted academic practice. No use, distribution or reproduction is permitted which does not comply with these terms.
*Correspondence: Christian Krupitzer, Y2hyaXN0aWFuLmtydXBpdHplckB1bmktaG9oZW5oZWltLmRl