- 1Edinburgh Medical School, College of Medicine and Veterinary Medicine, The University of Edinburgh, Edinburgh, United Kingdom
- 2Scottish Microelectronics Centre, Institute for Integrated Micro and Nano Systems, School of Engineering, The University of Edinburgh, Edinburgh, United Kingdom
- 3School of Informatics, Bayes Centre, The University of Edinburgh, Edinburgh, United Kingdom
- 4College of Medicine and Veterinary Medicine, The Queen's Medical Research Institute, The University of Edinburgh, Edinburgh, United Kingdom
- 5Department of Plastic Surgery, National Healthcare System Lothian, Edinburgh, United Kingdom
Current myoelectric upper limb prostheses do not restore sensory feedback, impairing fine motor control. Mechanotactile feedback restoration with a haptic sleeve may rectify this problem. This randomised crossover within-participant controlled study aimed to assess a prototype haptic sleeve's effect on routine grasping tasks performed by eight able-bodied participants. Each participant completed 15 repetitions of the three tasks: Task 1—normal grasp, Task 2—strong grasp and Task 3—weak grasp, using visual, haptic, or combined feedback All data were collected in April 2021 in the Scottish Microelectronics Centre, Edinburgh, UK. Combined feedback correlated with significantly higher grasp success rates compared to the vision alone in Task 1 (p < 0.0001), Task 2 (p = 0.0057), and Task 3 (p = 0.0170). Similarly, haptic feedback was associated with significantly higher grasp success rates compared to vision in Task 1 (p < 0.0001) and Task 2 (p = 0.0015). Combined feedback correlated with significantly lower energy expenditure compared to visual feedback in Task 1 (p < 0.0001) and Task 3 (p = 0.0003). Likewise, haptic feedback was associated with significantly lower energy expenditure compared to the visual feedback in Task 1 (p < 0.0001), Task 2 (p < 0.0001), and Task 3 (p < 0.0001). These results suggest that mechanotactile feedback provided by the haptic sleeve effectively augments grasping and reduces its energy expenditure.
Introduction
According to the closed loop theory of motor control, movement of a healthy human hand is governed by co-dependant feedforward muscle control and sensory feedback (1). Based on the latter, feedforward muscle control is adjusted to achieve economy of movement and the lowest possible metabolic energy expenditure (2), therefore closing the loop. When limb loss occurs, the loop of motor control becomes disrupted. The feedforward component of the loop may be partially restored with myoelectric prostheses (3). However, these devices do not restore sensory feedback, leaving the loop of motor control open (4). As a result, prosthetic users only have uncertain feedforward control at their disposal (5), making them unable to perceive tactile properties of handled objects and experience diminished motor control (6). They cannot feel their prosthetic grip force, leading to application of excessive force (resulting in excessive energy expenditure and muscle fatigue) and crushing of handled objects (7–9). In order to achieve satisfactory prosthetic performance, users heavily rely on visual feedback, which in turn increases cognitive load (10, 11). For these reasons, prosthesis embodiment remains poor, as reflected in the prosthesis abandonment rate of 40% (12).
Considering the above, it is unsurprising that most amputees agree restoring sensory feedback is as important as restoring feedforward muscle control (7, 13–15). Restoring tactile feedback, a type of sensory feedback, is especially promising. It has been shown to not only significantly improve grasp success rate (16–18) but also significantly decrease grip force (19–22). Restoring tactile feedback is also predicted to reduce prosthesis abandonment rate (23), providing a strong rationale for development of tactile feedback modalities.
So far, invasive and non-invasive tactile feedback modalities have been developed (24). Invasive modalities, such as targeted sensory re-innervation, direct peripheral nervous system stimulation and central nervous system stimulation, are promising due to their potential to elicit near-natural touch sensations (25–27). However, their clinical utility remains challenging (28). They carry a number of risks, such as nerve damage (29) and paraesthesia (30), have been tested on a limited number of volunteers and face much reluctance from amputees (31).
Non-invasive modalities, such as vibrotactile, electrotactile and mechanotactile feedback systems (28) are comparatively better characterised and constitute a more acceptable alternative as they require no surgical interventions (31). Yet, non-invasive modalities are not without their caveats. The main criticism of vibrotactile and electrotactile feedbacks is centred around their dissimilarity to endogenous tactile feedback, making them difficult to understand. Both are discontinuous (composed of discrete vibration or electric current bursts) and modality mismatched (vibrations or electric currents felt on the skin usually encode grip pressure), contrary to biological feedback (32). In contrast, mechanotactile feedback is both continuous and modality matched (pressure applied to skin encodes grip pressure). As such, it mimics natural tactile feedback, making the artificial feedback more intuitive to understand (32, 33). However, these advantages are balanced out by mechanotactile devices being larger, heavier, and of greater energy demands than their electrotactile and vibrotactile counterparts, inhibiting their development (34).
Disadvantages associated with invasive and non-invasive tactile feedback modalities contribute to their clinical and commercial unavailability. Mechanotactile feedback, as the only non-invasive modality providing continuous and modality matched feedback, seems to have an underdeveloped potential. Hence, research into how its current caveats can be resolved is warranted.
This study aimed to test the utility of a new mechanotactile feedback restoration device, a prototype haptic sleeve. Haptic sleeves are sleeve-shaped, variable compression devices which have so far demonstrated utility in robot-assisted surgery (35), social touch mimicking (36), and virtual reality enhancement (37). They are lightweight and thin, addressing the problems of heaviness and large size characteristic of contemporary mechanotactile feedback devices. While haptic band devices have been developed to provide sensory feedback in rehabilitation robotics, they have not uniformly been integrated into a prosthetic sleeve which is an integral part of the socket (38–41). Where a pneumatic device has been integrated into the socket, it has been at a discrete point instead of providing distributed sensory feedback across the residual limb (42). Our study demonstrates a soft socket that integrates haptic feedback across its inner surface whilst being capable of supporting the terminal device without need for any additional material.
The primary aim of the study was to be achieved by assessing the impact of the haptic sleeve on grasp success rate and energy expenditure of grasping. Grasp success rate was chosen as it is a simple, concrete metric which is in wide use in tactile feedback restoration studies (16–18). However, it is an indirect measure of tactile feedback impact on motor control, making it difficult to speculate about a cause-and-effect relationship. Therefore, changes in energy expenditure of grasping were recorded, too, as they are a more direct and robust basis for establishing a causal link between feedback restoration and improvement in outcomes (2). It was hypothesised that using the haptic sleeve will result in higher grasp success rate and reduced energy expenditure of grasping.
Materials and Methods
Design of the Haptic Sleeve
Wearable Sleeve
The prototype haptic sleeve used in the experiments was designed by the research team and manufactured by Koalaa Prostheses (London, UK) and can be seen in Supplementary Figure 1. Once mounted, the sleeve extended from the participant's proximal forearm to their wrist, allowing for a secure and comfortable fit while leaving enough space for the EMG electrode placement immediately distal to the elbow joint. The device was designed to compress the forearm proportionally to the pressure detected at prosthetic fingertips, thus delivering continuous mechanotactile feedback. To execute this function, the sleeve had a small motor (RS Pro 951D, RS Components, London, UK) mounted on its lateral side, as well as a pulley system with a thread wrapped around the sleeve equidistantly several times. Clockwise rotation of the motor resulted in winding of the thread around the sleeve, tightening it and therefore compressing the user's forearm. Anticlockwise rotation of the motor unwound the strings, untightening the sleeve and reducing the compression.
Electrical Design
To provide all the required analogue and digital inputs and outputs to the system, an Arduino Uno REV3 microprocessor (Arduino, Massachusetts, USA) was used. Connected to the microprocessor via a protoboard were two force-sensing resistors (FSRs) (Interlink Electronics FSR400, California, USA), three separate push button switches, a L298N 2A motor driver (HandsOn Tech, Johor, Malaysia), adjustable power supply (30V 3A Tenma 72-2540) and a Y-bridge. The electronic circuit obtained functioned to detect force applied at FSRs on prosthetic fingertips and translate it into rotation of the haptic sleeve's motor. It also recorded the EMG signals whilst they were used for the myoelectric prosthesis control. Simultaneous EMG signal recording and use was enabled by the Y-bridge which split the EMG signals from the electrodes into two channels. One channel connected to the prosthesis, facilitating myoelectric control, while the other channel connected to the microprocessor, allowing signal recording.
Software/Hardware Interface
The microprocessor was programmed using the Arduino Integrated Development Environment (Arduino, Massachusetts, USA). Its main functions were to record and save experimental readings, as well as interpret the FSR readings by comparing their current averaged force readings to their previous averaged value. If the new value was greater than the previous one, the motor engaged for 0.1 s at the speed proportional to the new value, tightening the sleeve. However, if the new average force was smaller than the previous one, the motor retained its current position. In this way, the haptic sleeve could provide continuous, proportional mechanotactile feedback. The Pulse Width Modulation (PWM) of the motor at time t is defined by the following equations:
Where is the new average force, Ft−1 equals the previous average force, FIndex is equal to the force from the index finger sensor, FThumb is the force from the thumb sensor, FMAX equals the maximum force of the sensor and PWMMax is the maximum PWM value.
The minimum force applied by the device was 0N, while the maximum force that the sleeve could generate was 5.1N.
All data recorded by the microprocessor during the experimental attempts was transferred to a PC via a USB serial cable and read and displayed in real-time via PuTTY application. Once each run was completed the application was closed and the data saved as a .txt file.
Ethical Considerations
The study was approved by the Informatics Research Ethics Board of the University of Edinburgh (2019/23785). Written consent was obtained from all participants prior to any experimentation.
Participant Selection
Participant inclusion criteria were being able-bodied and over the age of eighteen. Exclusion criteria were having a musculoskeletal disorder or prior experience with myoelectric control.
Experimental Setup
Figure 1 and Supplementary Figure 1 depict the experimental setup. The electronic circuit, the Y-bridge and the biological hand were all positioned on the table. The myoelectric prosthetic hand was clamped on the edge of the table so that the experimental object it grasped was unsupported. The prosthesis used in this study was a six degree-of-freedom Nexus Hand (COVVI, Leeds, UK). However, only one degree of freedom was used as this allowed optimal replication of the grasping motion. Participants operated the prosthesis using two 50 Hz Össur surface electrodes with built-in EMG signal amplifiers and philtres (Össur, Reykjavik, Iceland). One electrode was adhered to the skin over the forearm extensor digitorum communis muscle group whist the other over the flexor digitorum superficial is muscle group. The experimental object was a 295 ml plastic tumbler cup. When the grasp force applied was >4.5N, the cup broke, producing a distinctive, loud noise. After each breakage, the cup was replaced with a new one.
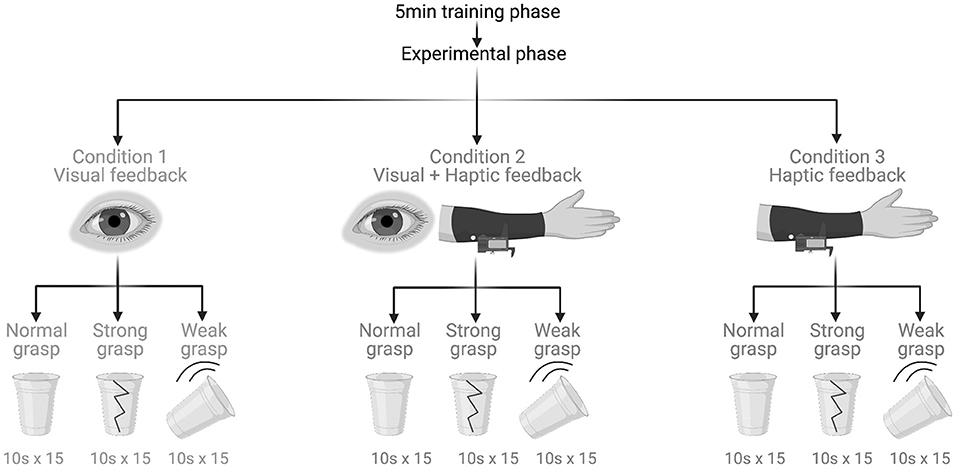
Figure 1. Experimental protocol and tasks. Created with BioRender.com.
Experimental Protocol
This study was a crossover randomised within-participant controlled trial. It began with a 5-min-long training phase consisting of guided familiarisation with myoelectric control. Next was the experimental phase made up of three experimental tasks that were performed under three feedback conditions (Figure 2). All participants completed all tasks under all conditions.
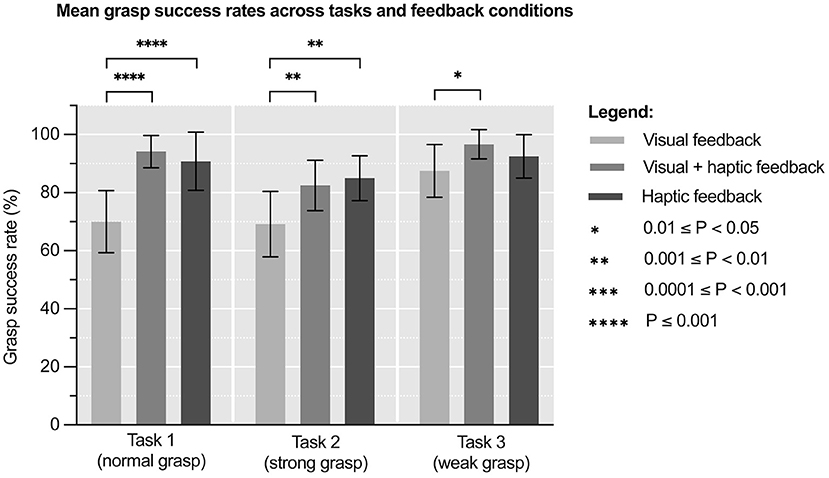
Figure 2. Mean grasp success rates (%) across tasks and feedback conditions. Error bars represent standard deviation. N = 8.
Sensory Feedback Conditions
Under the visual feedback condition, the participants did not wear the haptic sleeve and had their vision unobstructed. Thus, it was employed as a control condition. Under the visual plus haptic feedback condition, participants could still see but they also received mechanotactile feedback through the haptic sleeve. Under the haptic feedback condition, participants still received mechanotactile feedback but this time their vision was disabled by a blindfold. Any incidental auditory feedback was attenuated with the use of white noise-emitting headphones that participants wore at all times.
Experimental Tasks
Under each condition, participants had to perform fifteen 10-s-long repetitions of each task. In Task 1, normal grasp, the participants were instructed to grasp the experimental object with the myoelectric prosthesis so that the object neither breaks nor drops. Task 2, strong grasp, was the same as Task 1 but the instruction was to grasp the object as strongly as possible without breaking it. Task 3, weak grasp, was again the same as Task 1 but the command was to grasp the object as lightly as possible without dropping it. The purpose of the varying grasp strengths was to assess the impact of haptic feedback on grip force adjustment.
Randomisation
Simple randomisation was performed to obtain a unique sequence of feedback conditions and tasks for each participant. The sequences were generated in Research Randomizer (Social Psychology Network, USA) and the participants were blinded to their allocated sequence. The aim was to reduce confounding of the results by learning effects.
Outcome Measures
The primary outcome measure was grasp success rate, expressed as a percentage of successful attempts. A successful attempt was defined as neither breaking nor dropping the experimental object. The secondary outcome measure was energy expenditure of grasping, equal to the indefinite integral of the EMG curve.
Sample Size Calculation
Sample size calculation was performed based on preliminary primary outcome results for the first four participants with a mean of 75% grasp success rate and standard deviation of 9.97 with visual feedback alone, compared to a mean 98.25% and standard deviation of 3.03 with haptic feedback alone. Glass' delta between these two groups demonstrated an effect size of 2.33. Using this effect size, with a power of 80% and two-sided α level of 0.05, a desired sample size of eight participants was calculated using G*Power 3.1 (HHU, Düsseldorf, Germany).
Statistical Analysis
All statistical analyses were conducted in Prism 9.1.0 (GraphPad, California, USA). The threshold for statistical significance was adopted at p < 0.05. Shapiro-Wilk test of normality was performed first and showed all data were parametric. To determine if there was significance between groups, two-way analysis of variance (ANOVA) with repeated measures were conducted as there were two factors influencing the data (feedback condition and task). Partial eta squared () was calculated as an effect size measure of any significant results. Post-hoc Tukey's test was used to further characterise any statistical significance.
Results
Study Demographics
In total, eight volunteers were recruited between March and April 2021. All of them met inclusion criteria and none were excluded. Hence, all volunteers were randomised; they completed all experimental tasks and were included in the analyses. Supplementary Table 1 summarises their demographic characteristics. All experiments were conducted in April 2021 at the Scottish Microelectronics Centre, Edinburgh, UK.
Grasp Success Rate
Figure 3 and Supplementary Table 2 show mean grasp success rates across feedback conditions and tasks. One way repeated measures ANOVA of these results revealed significant variation in grasp success rates under different feedback conditions in Tasks 1 & 2, but not during Task 3: Task 1 [F(1.91, 13.4) = 48.5, p < 0.0001], Task 2 [F(1.25, 8.75) = 11.5, p = 0.006] and Task 3 [F(1.99, 14.0) = 3.47, p = 0.06]. The effect size of this variation was = 0.87 (95% CI: 0.70–0.91) for Task 1, = 0.62 (95% CI: 0.24–0.73) for Task 2 and = 0.42 (95% CI: 0.04–0.59) for Task 3. This means that ~87, 62, and 42% of variability in the results, respectively, can be attributed to feedback condition.
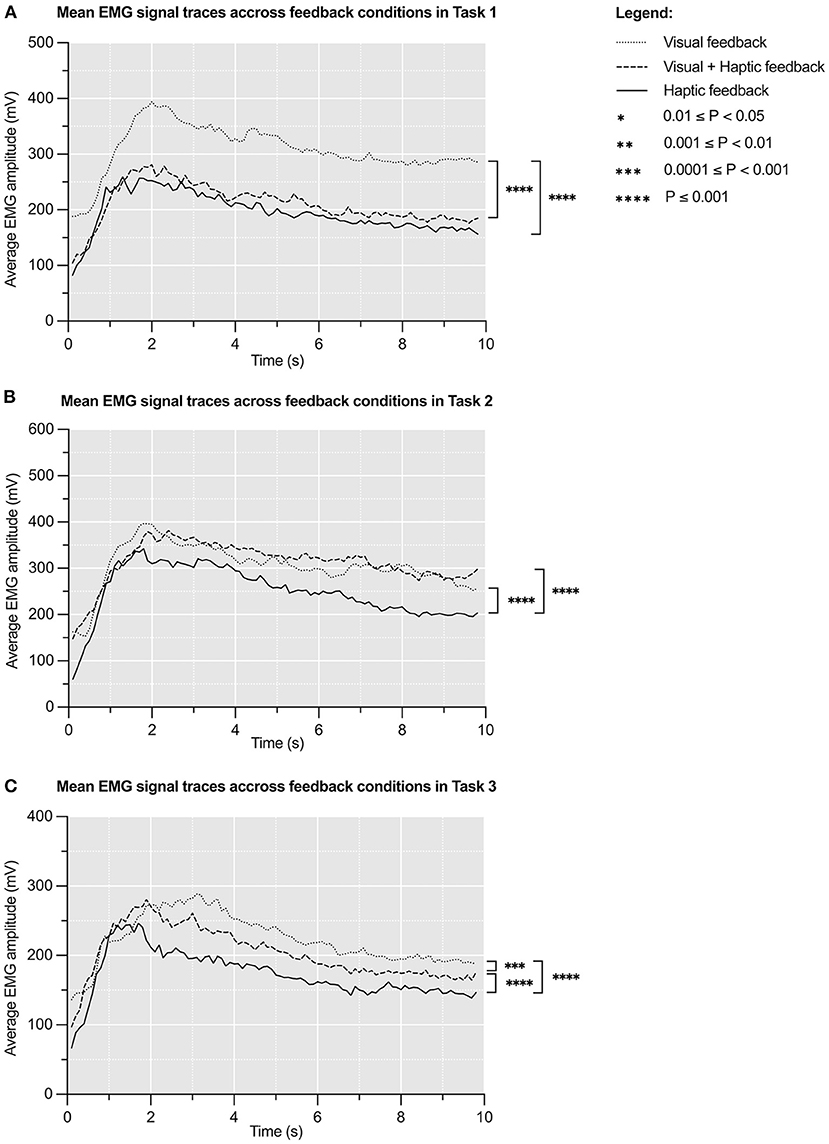
Figure 3. Mean EMG signal (mV) traces over 10s across feedback conditions in Task 1 (A), Task 2 (B), and Task 3 (C). The initial large peak corresponds to grasping the experimental object and the latter plateau corresponds to sustained grip. N = 8.
A post hoc Tukey's test showed that the mean grasp success rates under visual plus haptic feedback condition were significantly higher compared to those under visual feedback condition in all tasks: Task 1 (+34.6%, p < 0.0001), Task 2 (+19.2%, p = 0.006), and Task 3 (+10.5%, p = 0.017). It also showed that the mean grasp success rates under haptic feedback condition were significantly higher compared to those under visual feedback condition in Task 1 (+29.7%, p < 0.0001) and Task 2 (+22.8%, p = 0.0015). No significant differences were found between mean grasp success rates under visual plus haptic and haptic feedback condition in any of the tasks.
Energy Expenditure
Figure 4 represents mean EMG signal traces during all grasping attempts in respective tasks. These curves are timelines of participants' electromyographic activity (43). Mean areas under the EMG curves are presented in Supplementary Table 3. One way repeated measures ANOVA of these results revealed significant variation in mean areas under the EMG curves under different feedback conditions in all tasks: Task 1 [F(1.31, 9.18) = 9.545, p < 0.009], Task 2 [F(1.82, 12.8) = 6.36, p < 0.01), and Task 3 [F(1.20, 8.41) = 9.51, p < 0.01]. The effect size of this variation was = 0.31 (95% CI: 0.24–0.37) for Task 1, = 0.12 (95% CI: 0.07–0.17) for Task 2 and = 0.24 (95% CI: 0.17–0.30) for Task 3. This means that feedback condition accounts for ~31, 12, and 24% of variability in the results across Task 1, 2, and 3, respectively.
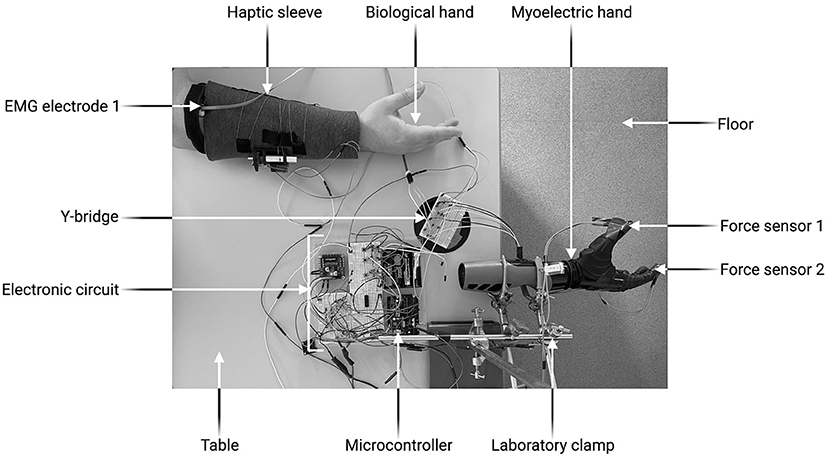
Figure 4. Experimental set-up. Created with BioRender.com.
A post hoc Tukey's test demonstrated that the haptic feedback condition was associated with significantly lower mean energy expenditure compared to the visual feedback condition in all tasks: Task 1 (−36.7%, p < 0.0001), Task 2 (−18.1%, p < 0.0001), and Task 3 (−22.4%, p < 0.0001). It also showed that the visual plus haptic feedback condition correlated with significantly lower mean energy expenditure compared to the visual feedback condition in Task 1 (−31.8%, p < 0.0001) and Task 3 (−8.7%, p = 0.0003).
Discussion
Key Findings and Interpretation
This study was the first to adapt and test a wearable sleeve that integrates haptic feedback across the prosthetic socket as a method of mechanotactile feedback restoration. It showed that using the device correlates with higher grasp success rate and lower muscle energy expenditure, which is consistent with the initial hypothesis. These findings are clinically relevant. Difficulty grasping and muscle fatigue are some of the most important factors contributing to high prosthesis abandonment rate (12). Minimising their impact could increase prostheses function, potentially elevating amputees' overall quality of life.
Haptic Feedback Correlates With Higher Grasp Success Rate
Conditions including haptic feedback were found to be associated with significantly higher grasp success rates in all tasks (Figure 3). Additionally, feedback condition accounted for most variability in Task 1 and 2, suggesting a possible causative relationship. Correlation between non-invasive tactile feedback restoration and higher grasp success rates is well-established in bionic literature. Studies on electrotactile (16), vibrotactile (17), and mechanotactile (18) feedback all report the same trend. It is proposed that better grasp success rates result from participants utilising the feedback to better control the force they are applying (44). Another interesting finding was no significant difference in grasp success rates under visual plus haptic and haptic feedback condition in any of the tasks. There are two important implications to this. Firstly, it suggests that haptic feedback reduced participants' reliance on vision, a desirable phenomenon documented in other studies on tactile feedback (10, 11). Secondly, it might mean that the device's feedback delay is equal to, or even shorter than, visual feedback delay of 250 ms (45), making haptic feedback quick enough to be readily used (46).
Haptic Feedback Correlates With Lower Energy Expenditure
Addition of haptic feedback was also noted to be correlated with significantly lower energy expenditure of grasping in all tasks, when the averaged EMG data was investigated across all trials (Figure 4). Moreover, it was estimated to be responsible for 12–31% of the variability in the results, suggesting a possible cause-and-effect relationship. It is challenging to compare this finding to existing literature as no previous study has calculated energy expenditure to assess sensory feedback restoration. Even studies that use EMG signals as a feedback modality (47, 48) do not report any outcomes in terms of energy expenditure. The reduction in energy expenditure demonstrated in this study can be explained by the closed loop motor control theory (1). According to the theory, sensory feedback is constantly used to achieve economy of movement and the lowest possible energy expenditure (2). Additionally, in prosthetic control systems, sensory feedback can partially rectify the inherent uncertainty of the feedforward myoelectric control, improving the overall motor control (5). Thus, it is possible that mechanotactile feedback provided by the sleeve closed participants' motor control loops effectively enough to allow for motor control and energy expenditure to be optimised.
Strengths
Simultaneous EMG Signal Recording and Use
Muscle EMG traces are considered one of the most accurate methods of metabolic energy expenditure estimation for individual muscles and muscle groups (49). Measuring energy expenditure is of high importance in prosthetic research as it correlates with physical fatigue of prosthesis use (50). Fatigue, if excessive, leads to prosthesis abandonment (12, 51). Previous studies on tactile feedback restoration struggled to reliably record EMG signals due to inability to simultaneously record EMG signals and use them for myoelectric control (43). A Y-bridge, as used in this study, circumvents this issue by splitting the EMG signal registered by the electrodes into two independent channels. The technique is simple yet reliable and can be easily incorporated into future studies of this kind.
Experimental Sequence Randomisation
Haptic feedback use is characterised by a learning curve whereby functional outcomes improve with practise (52, 53). To reduce the confounding effects of learning on this study's results, simple randomisation of experimental task sequence was performed. Not only did this distribute the learning bias across feedback conditions and tasks, but also hindered learning by eliminating predictability (54). Despite each participant performing a unique sequence of tasks, significant changes were found in favour of haptic feedback, which corroborates the study's internal validity.
Adequate Sample Size and Power
The required sample size was met and therefore the study is 80% powered for the primary outcome to keep the probability of Type II error at 0.2 and, thus achieves a statistically sound balance with Type I error probability of 0.05 (55). However, as this study particularly looked at the impact of the tasks on EMG activity as a marker of energy expenditure, and there was limited previous data to calculate the sample size, our calculations were based on the pilot data of 4 participants. Consequently, while the significant results obtained in this investigation may reflect a true effect, further work with amputee participants should help corroborate these findings.
Limitations
Lack of Amputee Participants
Due to the concurrent COVID-19 pandemic, recruiting amputee participants was made impossible. Considering the situation, the study was adapted to accommodate able-bodied participants. However, this was a suboptimal solution due to a number of significant differences between a residual limb and a healthy hand. During the amputation procedure, certain muscles and nerves are partially or completely removed, impeding subsequent EMG signal generation and making it more variable compared to able-bodied counterparts (56). Several changes may occur after the amputation, too. These include residual limb muscle atrophy, phantom limb pain or sensations, as well as contracture and neuromata formation (57). As a result, amputee participants may find it more difficult to not only generate EMG signals sufficient for myoelectric control, but also perceive and interpret the mechanotactile feedback provided by the haptic sleeve. Thus, the study's generalisability to amputee population is compromised.
Incomplete Natural Sensory Feedback Disablement
One common criticism of using able-bodied participants in studies assessing sensory feedback restoration is that these volunteers have their natural sensory feedback intact, which can confound the results (28). Although this study experimental setup meant that natural tactile feedback was negligible (a healthy hand did not touch the experimental object), participants still had their proprioception intact (they could move their biological hands). As a consequence, they were able to understand their hands' position even with their vision disabled, which could arguably result in better performance compared to amputees. To fully disable all sensory feedback, peripheral nerve blocks (58) or inflatable cuffs (59) have been used in similar studies in the field. However, the benefit of using these methods has to be balanced against their invasiveness and painfulness, as well as the requirement for additional ethical considerations.
Implications for Future Research and Clinical Practise
Future research should aim to further develop and test the haptic sleeve. Firstly, the device should be adapted for amputee use and assessed in the target user group. Additionally, the effects of the haptic sleeve need to be studied on a greater range of manipulative tasks. For that, established clinical tests assessing user performance in myoelectric control can be used, such as the Southampton Hand Assessment Procedure or the Action Research Arm Test (60). Any significant effects can later be studied over time to characterise the learning curve.
In order to successfully introduce the haptic sleeve onto the market and into clinical practise, it needs to be made portable. The current prototypic electronic circuit can be miniaturised into a compact control platform which will easily fit within the sleeve. If future studies corroborate clinical benefits of using the haptic sleeve, the device may become an integral element of the rehabilitation process after an upper limb amputation. Thanks to the sleeve, future amputees might be able to achieve better prosthetic function, which may translate into greater prosthesis embodiment, reduced phantom limb pain, enhanced quality of life and wider job opportunities.
Conclusion
This study demonstrates that a haptic sleeve can be an effective tool for mechanotactile feedback restoration. Use of the device correlates with significantly higher grasp success rates and significantly lower energy expenditure of grasping in healthy volunteers. These findings are likely due to the haptic sleeve improving control of the applied force, decreasing reliance on vision and closing the motor control loop. Further research into the area is warranted and should focus on adapting the device for amputee use and improving its portability. With these enhancements, the haptic sleeve may help amputees recover more function and improve their quality of life.
Data Availability Statement
The raw data supporting the conclusions of this article will be made available by the authors, without undue reservation.
Author Contributions
AR conceived the study, with VB, AM, SV, and AS contributing to the design and implementation of the work. VB and AM collected the data and analysed the data with AR. All authors contributed to drafting and reviewing the manuscript before final approval for submission.
Funding
The Academy of Medical Sciences supported this study through a Starter Grant for Clinical Lecturers (SGL021/1004) to the corresponding author.
Conflict of Interest
The authors declare that the research was conducted in the absence of any commercial or financial relationships that could be construed as a potential conflict of interest.
Publisher's Note
All claims expressed in this article are solely those of the authors and do not necessarily represent those of their affiliated organizations, or those of the publisher, the editors and the reviewers. Any product that may be evaluated in this article, or claim that may be made by its manufacturer, is not guaranteed or endorsed by the publisher.
Acknowledgments
The authors would like to thank Gino La for his creative input into the initial haptic sleeve design, as well as Kianoush Nazarpour, Sigrid Dupan, and Ivan Vujaklija for their invaluable advice on the study design and EMG signal recording.
Supplementary Material
The Supplementary Material for this article can be found online at: https://www.frontiersin.org/articles/10.3389/fresc.2022.806479/full#supplementary-material
Supplementary Figure 1. Data flow through the experimental set-up. Created with BioRender.com.
Supplementary Table 1. Demographic characteristics of the study population. Numbers in brackets represent standard deviation. BMI, Body Mass Index. N = 8.
Supplementary Table 2. Mean grasp success rates (%) across tasks and feedback conditions. Numbers in brackets represent standard deviation. N = 8.
Supplementary Table 3. Mean energy expenditures (mV) across tasks and feedback conditions. Numbers in brackets represent standard deviation. N = 8.
References
1. Adams JA. A closed-loop theory of motor learning. J Mot Behav. (1971) 3:111–50. doi: 10.1080/00222895.1971.10734898
2. Sparrow WA, Newell KM. Metabolic energy expenditure and the regulation of movement economy. Psychon Bull Rev. (1998) 5:173–96. doi: 10.3758/BF03212943
3. Belter JT, Segil JL, Dollar AM, Weir RF. Mechanical design and performance specifications of anthropomorphic prosthetic hands: a review. J Rehabil Res Dev. (2013) 50:599–618. doi: 10.1682/JRRD.2011.10.0188
4. Roche AD, Lakey B, Mendez I, Vujaklija I, Farina D, Aszmann OC. Clinical perspectives in upper limb prostheses: an update. Curr Surg Reports. (2019) 7:5. doi: 10.1007/s40137-019-0227-z
5. Saunders I, Vijayakumar S. The role of feed-forward and feedback processes for closed-loop prosthesis control. J Neuroeng Rehabil. (2011) 8:60. doi: 10.1186/1743-0003-8-60
6. Sensinger JW, Dosen S. A review of sensory feedback in upper-limb prostheses from the perspective of human motor control. Front Neurosci. (2020) 14:345. doi: 10.3389/fnins.2020.00345
7. Pylatiuk C, Schulz S, Döderlein L. Results of an internet survey of myoelectric prosthetic hand users. Prosthet Orthot Int. (2007) 31:362–70. doi: 10.1080/03093640601061265
8. Jang CH, Yang HS, Yang HE, Lee SY, Kwon JW, Yun BD, et al. A survey on activities of daily living and occupations of upper extremity amputees. Ann Rehabil Med. (2011) 35:907. doi: 10.5535/arm.2011.35.6.907
9. Cordella F, Ciancio AL, Sacchetti R, Davalli A, Cutti AG, Guglielmelli E, et al. Literature review on needs of upper limb prosthesis users. Front Neurosci. (2016) 10:209. doi: 10.3389/fnins.2016.00209
10. Raveh E, Friedman J, Portnoy S. Visuomotor behaviors and performance in a dual-task paradigm with and without vibrotactile feedback when using a myoelectric controlled hand. Assist Technol. (2018) 30:274–80. doi: 10.1080/10400435.2017.1323809
11. Wheeler J, Bark K, Savall J, Cutkosky M. Investigation of rotational skin stretch for proprioceptive feedback with application to myoelectric systems. IEEE Trans Neural Syst Rehabil Eng. (2010) 18:58–66. doi: 10.1109/TNSRE.2009.2039602
12. Biddiss EA, Chau TT. Upper limb prosthesis use and abandonment: a survey of the last 25 years. Prosthet Orthot Int. (2007) 31:236–57. doi: 10.1080/03093640600994581
13. Lewis S, Russold MF, Dietl H, Kaniusas E. User demands for sensory feedback in upper extremity prostheses. MeMeA. (2012) - (2012). IEEE Symp Med Meas Appl Proc. (2012). 188–91. doi: 10.1109/MeMeA.2012.6226669
14. Wijk U, Carlsson I. Forearm amputees' views of prosthesis use and sensory feedback. J Hand Ther. (2015) 28:269–78. doi: 10.1016/j.jht.2015.01.013
15. Farina D, Amsüss S. Reflections on the present and future of upper limb prostheses. Expert Rev Med Devices. (2016) 13:321–4. doi: 10.1586/17434440.2016.1159511
16. Jorgovanovic N, Dosen S, Djozic DJ, Krajoski G, Farina D. Virtual grasping: closed-loop force control using electrotactile feedback. Comput Math Methods Med. (2014) 2014:120357. doi: 10.1155/2014/120357
17. Clemente F, D'Alonzo M, Controzzi M, Edin BB, Cipriani C. Non-invasive, temporally discrete feedback of object contact and release improves grasp control of closed-loop myoelectric transradial prostheses. IEEE Trans Neural Syst Rehabil Eng. (2016) 24:1314–22. doi: 10.1109/TNSRE.2015.2500586
18. Fallahian N, Saeedi H, Mokhtarinia H, Tabatabai Ghomshe F. Sensory feedback add-on for upper-limb prostheses. Prosthet Orthot Int. (2017) 41:314–7. doi: 10.1177/0309364616677653
19. Chaubey P, Rosenbaum-Chou T, Daly W, Boone D. Closed-loop vibratory haptic feedback in upper-limb prosthetic users. J Prosthetics Orthot. (2014) 26:120–7. doi: 10.1097/JPO.0000000000000030
20. Xu H, Zhang D, Huegel JC, Xu W, Zhu X. Effects of different tactile feedback on myoelectric closed-loop control for grasping based on electrotactile stimulation. IEEE Trans Neural Syst Rehabil Eng. (2016) 24:827–36. doi: 10.1109/TNSRE.2015.2478153
21. Rosenbaum-Chou T, Daly W, Austin R, Chaubey P, Boone DA. Development and real world use of a vibratory haptic feedback system for upper-limb prosthetic users. J Prosthetics Orthot. (2016) 28:136–44. doi: 10.1097/JPO.0000000000000107
22. Schoepp KR, Dawson MR, Schofield JS, Carey JP, Hebert JS. Design and integration of an inexpensive wearable mechanotactile feedback system for myoelectric prostheses. IEEE J Transl Eng Heal Med. (2018) 6:2100711. doi: 10.1109/JTEHM.2018.2866105
23. Dietrich C, Walter-Walsh K, Preißler S, Hofmann GO, Witte OW, Miltner WHR, et al. Sensory feedback prosthesis reduces phantom limb pain: Proof of a principle. Neurosci Lett. (2012) 507:97–100. doi: 10.1016/j.neulet.2011.10.068
24. Antfolk C, D'Alonzo M, Rosén B, Lundborg G, Sebelius F, Cipriani C. Sensory feedback in upper limb prosthetics. Expert Rev Med Devices. (2013) 10:45–54. doi: 10.1586/erd.12.68
25. Branner A, Stein RB, Normann RA. Selective stimulation of cat sciatic nerve using an array of varying-length microelectrodes. J Neurophysiol. (2001) 85:1585–94. doi: 10.1152/jn.2001.85.4.1585
26. Badia J, Boretius T, Pascual-Font A, Udina E, Stieglitz T, Navarro X. Biocompatibility of chronically implanted transverse intrafascicular multichannel electrode (TIME) in the rat sciatic nerve. IEEE Trans Biomed Eng. (2011) 58:2324–32. doi: 10.1109/TBME.2011.2153850
27. Flesher SN, Collinger JL, Foldes ST, Weiss JM, Downey JE, Tyler-Kabara EC, et al. Intracortical microstimulation of human somatosensory cortex. Sci Transl Med. (2016) 8:361ra141. doi: 10.1126/scitranslmed.aaf8083
28. Stephens-Fripp B, Alici G, Mutlu R. A review of non-invasive sensory feedback methods for transradial prosthetic hands. IEEE Access. (2018) 6:6878–99. doi: 10.1109/ACCESS.2018.2791583
29. Kovacs GTA, Storment CW, Rosen JM. Regeneration microelectrode array for peripheral nerve recording and stimulation. IEEE Trans Biomed Eng. (1992) 39:893–902. doi: 10.1109/10.256422
30. Tan DW, Schiefer MA, Keith MW, Anderson JR, Tyler J, Tyler DJ. A neural interface provides long-term stable natural touch perception. Sci Transl Med. (2014) 6:257ra138. doi: 10.1126/scitranslmed.3008669
31. Benz HL, Yao J, Rose L, Olgac O, Kreutz K, Saha A, et al. Upper extremity prosthesis user perspectives on unmet needs and innovative technology. In: (2016) 38th Annual International Conference of the IEEE Engineering in Medicine and Biology Society (EMBC) [Internet]. IEEE (2016). p. 287–90. Available online at: http://ieeexplore.ieee.org/document/7590696/ (accessed October 31, 2021).
32. Schofield JS, Evans KR, Carey JP, Hebert JS. Applications of sensory feedback in motorized upper extremity prosthesis: a review. Expert Rev Med Devices. (2014) 11:499–511. doi: 10.1586/17434440.2014.929496
33. Wijk U, Carlsson IK, Antfolk C, Björkman A, Rosén B. Sensory feedback in hand prostheses: a prospective study of everyday use. Front Neurosci. (2020) 14:663. doi: 10.3389/fnins.2020.00663
34. Li G, Zhang L, Sun Y, Kong J. Towards the sEMG hand: internet of things sensors and haptic feedback application. Multimed Tools Appl. (2019) 78:29765–82. doi: 10.1007/s11042-018-6293-x
35. Wurdemann HA, Secco EL, Nanayakkara T, Althoefer K, Mucha L, Rohr K. Mapping tactile information of a soft manipulator to a haptic sleeve in RMIS. In: Poorten E, Capiluppi M, Fiorini P, editors. Proceedings of 3rd Joint Workshop on New Technologies for Computer and Robot Assisted Surgery. Verona (2013) p. 140–1.
36. Culbertson H, Nunez CM, Israr A, Lau F, Abnousi F, Okamura AM. A social haptic device to create continuous lateral motion using sequential normal indentation. In:. (2018) IEEE Haptics Symposium (HAPTICS) [Internet]. IEEE; (2018). p. 32–9. Available online at: https://ieeexplore.ieee.org/document/8357149/ (accessed October 31, 2021).
37. Zhu M, Memar AH, Gupta A, Samad M, Agarwal P, Visell Y, et al. PneuSleeve: in-fabric multimodal actuation and sensing in a soft, compact, and expressive haptic sleeve. Conf Hum Factors Comput Syst Proc. (2020) 1–12. doi: 10.1145/3313831.3376333
38. Hussain I, Meli L, Pacchierotti C, Prattichizzo D. A soft robotic supernumerary finger and a wearable cutaneous finger interface to compensate the missing grasping capabilities in chronic stroke patients. In:. (2017) IEEE World Haptics Conference (WHC) [Internet]. IEEE; (2017). p. 183–8. Available online at: http://ieeexplore.ieee.org/document/7989898/ (accessed October 31, 2021).
39. Meli L, Hussain I, Aurilio M, Malvezzi M, O'Malley MK, Prattichizzo D. The hBracelet: a wearable haptic device for the distributed mechanotactile stimulation of the upper limb. IEEE Robot Autom Lett. (2018) 3:2198–205. doi: 10.1109/LRA.2018.2810958
40. Casini S, Morvidoni M, Bianchi M, Catalano M, Grioli G, Bicchi A. Design and realization of the CUFF - Clenching upper-limb force feedback wearable device for distributed mechano-tactile stimulation of normal and tangential skin forces. IEEE Int Conf Intell Robot Syst. (2015) 2015:1186–93. doi: 10.1109/IROS.2015.7353520
41. Battaglia E, Clark JP, Bianchi M, Catalano MG, Bicchi A, O'Malley MK. The Rice Haptic Rocker: Skin stretch haptic feedback with the Pisa/IIT SoftHand. In:. (2017) IEEE World Haptics Conference (WHC) [Internet]. IEEE; (2017). p. 7–12. Available online at: http://ieeexplore.ieee.org/document/7989848/ (accessed October 31, 2021).
42. Barontini F, Catalano MG, Grioli G, Bianchi M, Bicchi A. Wearable integrated soft haptics in a prosthetic socket. IEEE Robot Autom Lett. (2021) 6:1785–92. doi: 10.1109/LRA.2021.3060432
43. Reaz MBI, Hussain MS, Mohd-Yasin F. Techniques of EMG signal analysis: detection, processing, classification and applications. Biol Proced Online. (2006) 8:11–35. doi: 10.1251/bpo115
44. De Nunzio AM, Dosen S, Lemling S, Markovic M, Schweisfurth MA, Ge N, et al. Tactile feedback is an effective instrument for the training of grasping with a prosthesis at low- and medium-force levels. Exp Brain Res. (2017) 235:2547–59. doi: 10.1007/s00221-017-4991-7
45. Shimada S, Qi Y, Hiraki K. Detection of visual feedback delay in active and passive self-body movements. Exp Brain Res. (2010) 201:359–64. doi: 10.1007/s00221-009-2028-6
46. Shimada S, Fukuda K, Hiraki K. Rubber hand illusion under delayed visual feedback. PLoS ONE. (2009) 4:e6185. doi: 10.1371/journal.pone.0006185
47. Dosen S, Markovic M, Somer K, Graimann B, Farina D. EMG Biofeedback for online predictive control of grasping force in a myoelectric prosthesis. J Neuroeng Rehabil. (2015) 12:55. doi: 10.1186/s12984-015-0047-z
48. Schweisfurth MA, Markovic M, Dosen S, Teich F, Graimann B, Farina D. Electrotactile EMG feedback improves the control of prosthesis grasping force. J Neural Eng. (2016) 13:056010. doi: 10.1088/1741-2560/13/5/056010
49. Blake OM, Wakeling JM. Estimating changes in metabolic power from EMG. Springerplus. (2013) 2:229. doi: 10.1186/2193-1801-2-229
50. Sahlin K, Tonkonogi M, Söderlund K. Energy supply and muscle fatigue in humans. Acta Physiol Scand. (1998) 162:261–6. doi: 10.1046/j.1365-201X.1998.0298f.x
51. Østlie K, Lesjø IM, Franklin RJ, Garfelt B, Skjeldal OH, Magnus P. Prosthesis rejection in acquired major upper-limb amputees: a population-based survey. Disabil Rehabil Assist Technol. (2012) 7:294–303. doi: 10.3109/17483107.2011.635405
52. Graczyk EL, Resnik L, Schiefer MA, Schmitt MS, Tyler DJ. Home use of a neural-connected sensory prosthesis provides the functional and psychosocial experience of having a hand again. Sci Rep. (2018) 8:9866. doi: 10.1038/s41598-018-26952-x
53. Cuberovic I, Gill A, Resnik LJ, Tyler DJ, Graczyk EL. Learning of artificial sensation through long-term home use of a sensory-enabled prosthesis. Front Neurosci. (2019) 13:853. doi: 10.3389/fnins.2019.00853
54. Lim CY, In J. Randomization in clinical studies. Korean J Anesthesiol. (2019) 72:221–32. doi: 10.4097/kja.19049
55. Serdar CC, Cihan M, Yücel D, Serdar MA. Sample size, power and effect size revisited: simplified and practical approachin pre-clinical, clinical and laboratory studies. Biochem Medica. (2021) 31:010502. doi: 10.11613/BM.2021.010502
56. McClanahan A, Moench M, Fu Q. Dimensionality analysis of forearm muscle activation for myoelectric control in transradial amputees. PLoS ONE. (2020) 15:e0242921. doi: 10.1371/journal.pone.0242921
57. Yoo S. Complications following an amputation. Phys Med Rehabil Clin N Am. (2014) 25:169–78. doi: 10.1016/j.pmr.2013.09.003
58. Pohlmeyer EA, Jordon LR, Kim P, Miller LE. A fully implanted drug delivery system for peripheral nerve blocks in behaving animals. J Neurosci Methods. (2009) 182:165–71. doi: 10.1016/j.jneumeth.2009.06.006
59. Galán F, Baker MR, Alter K, Baker SN. Degraded EEG decoding of wrist movements in absence of kinaesthetic feedback. Hum Brain Mapp. (2015) 36:643–54. doi: 10.1002/hbm.22653
Keywords: haptic, mechanotactile, sensory feedback, sensory restoration, prosthetic, prosthesis, hand, upper limb
Citation: Borkowska VR, McConnell A, Vijayakumar S, Stokes A and Roche AD (2022) A Haptic Sleeve as a Method of Mechanotactile Feedback Restoration for Myoelectric Hand Prosthesis Users. Front. Rehabilit. Sci. 3:806479. doi: 10.3389/fresc.2022.806479
Received: 31 October 2021; Accepted: 21 March 2022;
Published: 25 April 2022.
Edited by:
Oskar C. Aszmann, Medical University of Vienna, AustriaCopyright © 2022 Borkowska, McConnell, Vijayakumar, Stokes and Roche. This is an open-access article distributed under the terms of the Creative Commons Attribution License (CC BY). The use, distribution or reproduction in other forums is permitted, provided the original author(s) and the copyright owner(s) are credited and that the original publication in this journal is cited, in accordance with accepted academic practice. No use, distribution or reproduction is permitted which does not comply with these terms.
*Correspondence: Aidan D. Roche, YWlkYW4ucm9jaGVAZWQuYWMudWs=