- 1Department of Psychology, Palo Alto University, Palo Alto, CA, United States
- 2Neuroscience Program, SRI International, Menlo Park, CA, United States
- 3School of Medicine, Stanford University, Stanford, CA, United States
Introduction: Autism Spectrum Disorder (ASD) is characterized by deficits in social cognition, self-referential processing, and restricted repetitive behaviors. Despite the established clinical symptoms and neurofunctional alterations in ASD, definitive biomarkers for ASD features during neurodevelopment remain unknown. In this study, we aimed to explore if activation in brain regions of the default mode network (DMN), specifically the medial prefrontal cortex (MPC), posterior cingulate cortex (PCC), superior temporal sulcus (STS), inferior frontal gyrus (IFG), angular gyrus (AG), and the temporoparietal junction (TPJ), during resting-state functional magnetic resonance imaging (rs-fMRI) is associated with possible phenotypic features of autism (PPFA) in a large, diverse youth cohort.
Methods: We used cross-sectional parent-reported PPFA data and youth rs-fMRI brain data as part of the two-year follow-up of the Adolescent Brain Cognitive Development (ABCD) study. Our sample consisted of 7,106 (53% male) adolescents aged 10-13. We conducted confirmatory factor analyses (CFAs) to establish the viability of our latent measurements: features of autism and regional brain activation. Structural regression analyses were used to investigate the associations between the six brain regions and the PPFA.
Results: We found that activation in the MPC (β = .16, p < .05) and the STS (β = .08, p < .05), and being male (β = .13, p < .05), was positively associated with PPFA. In contrast, activation in the IFG (β = −.08, p < .05) was negatively associated.
Discussion: Our findings suggest that regions of the “social brain” are associated with PPFA during early adolescence. Future research should characterize the developmental trajectory of social brain regions in relation to features of ASD, specifically brain regions known to mature relatively later during development.
1 Introduction
Autism spectrum disorder (ASD) is associated with deficits in social cognitive processes and self-referential processes (1, 2). In addition to social impairment, ASD is clinically associated with restricted, repetitive behaviors and interests (3). ASD symptoms can be observed at a sub-clinical threshold, and it is established that a broad autism phenotype exists, which captures a range of social functioning on a continuum (4).
Neurofunctional substrates of ASD have been characterized by atypical brain activation (5) and altered functional connectivity (FC) among brain regions (6) during resting-state functional magnetic resonance imaging (rs-fMRI). Studies consistently highlight abnormalities in several key brain networks associated with social cognition, communication, and sensory processing. For instance, reduced connectivity within the default mode network (DMN), including regions such as the medial prefrontal cortex (MPC) and posterior cingulate cortex (PCC), has been linked to social deficits in ASD (7, 8). Similarly, atypical functioning of the salience network (SN), particularly in regions like the anterior insula and anterior cingulate cortex (ACC), has been associated with challenges in processing socially relevant stimuli (9). Other studies have pointed to hyper- or hypoconnectivity in the amygdala, a region critical for emotion regulation, as being involved in ASD-related emotion processing difficulties (10).
Despite this body of evidence, ASD does not have a single, agreed-upon biomarker or identifiable neural structure that characterizes the condition (11). It is also unclear how features of autism are commonly represented during neurodevelopment. This lack of a definitive biomarker has prompted further investigation into more specific neural processes, such as self-referential processes associated with the DMN, which is activated during rs-fMRI (12, 13). Poor self-referential processing in ASD is linked to poor theory of mind, a critical social cognitive process (2). DMN activation in brain regions associated with self-referential processing may provide insight into mechanisms associated with features of autism during development in adolescents who do not have an ASD diagnosis but show subclinical ASD symptoms. We aim to build upon existing research looking at select neural correlates of the DMN related to self-referencing and theory of mind in ASD (14) by examining youth during early adolescence, a critical period of self-identity development and social development, when social cognition and self-reflection may be most indicated.
Brain structures of interest in the present study include the MPC, PCC, superior temporal sulcus (STS), inferior frontal gyrus (IFG), angular gyrus (AG), and the temporoparietal junction (TPJ). These brain regions of the DMN have neurofunctional overlap with the regions of what some have termed the theory of mind network [ToMN; (15, 16)], a functional network involved in social cognition (17, 18). These brain structures can be categorized based on functional purpose in the context of thinking about and interacting with the social world and are collectively considered regions of the “social brain” (15, 19). The MPC is linked with mentalizing or theory of mind (i.e., mentalizing or reflecting on the emotional/mental states of self and others), person perception, self-perception, and self-referential thinking (2, 20, 21). The temporal regions contribute to conceptual and semantic knowledge related to mentalizing (22). The PCC has been theorized as a sub-component for self-referential and other social cognitive processes (20, 23). The STS and TPJ are linked with predicting biological movement, assignment of agency to self and others (i.e., theory of mind and self-other distinction), perspective taking, and empathy (15, 24). The shared functions of these brain regions as they relate to features of autism, specifically during adolescent development, are not yet fully understood. Maturation of brain regions is not uniform during adolescence, with frontal brain regions maturing later (25, 26) and less neurodevelopmental change seen in ASD (27) influencing social development and broad autism phenotype during adolescence (28).
In this study, we examined rs-fMRI activation in regions that are known to be involved in social cognition, especially those social cognitive processes related to phenotypic features of autism. Brain activation during rs-fMRI is known to be heterogeneous (29) and changes throughout development (30). Accordingly, we tested whether intrinsic activity in brain regions of interest are associated with possible phenotypic features of autism (PPFA) in a large, diverse youth sample across the United States.
2 Method
2.1 Participants
We analyzed cross-sectional data from the two-year follow-up (2YFU) visit from the Adolescent Brain Cognitive Development (ABCD) study (5.1 data release). The data included in the study is available on the NIMH Data Archive via data access request. The study used resting-state functional magnetic resonance imaging (rs-fMRI) data and parent report data of 7,108 adolescents between the ages of 10–13 (M = 11.95, SD = 0.65). Table 1 describes demographic characteristics of the sample. Our analysis’ inclusion and exclusion criteria were consistent with the criteria for participation in the ABCD study (31). Accordingly, children with a confirmed diagnosis of moderate or severe ASD were excluded from ABCD study enrollment at baseline. Although the ABCD study does not have data on clinically diagnosed ASD, it is possible that youth with mild ASD and ASD feature endorsement are included. Additionally, our sample was limited to participants with brain imaging data collected at their 2YFU visit, thus does not include all participants actively enrolled in the ABCD study. The 2YFU time point was used because it was the only time point that had data for our variables of interest in the 5.1 data release.
2.2 Measures
2.2.1 Brain regions
We used the temporal variability of 22 cortical parcellations from the Destrieux brain atlas (32) during rs-fMRI. Temporal variability describes fluctuations in the BOLD (blood-oxygen-level-dependent) signal over a specified time course (i.e., the length of the rs-fMRI scan) from which neural activity in the region is inferred (33–35). The cortical parcellations used for analysis were grouped to form brain regions of interest: MPC, PCC, STS, IFG, AG, and TPJ. The Destrieux atlas’ anatomical parcellation names (index numbers) used for each region are as follows:
MPC: The left and right anterior part of the cingulate gyrus and sulcus (6, 80).
The left and right middle-anterior part of the cingulate gyrus and sulcus (7, 81).
PPC: The left and right posterior part of the cingulate gyrus and sulcus (8, 82).
The left and right posterior-dorsal part of the cingulate gyrus (9, 83).
The left and right posterior-ventral part of the cingulate gyrus (10, 84).
STS: The left and right STS (73, 147).
IFG: The left and right opercular part of the inferior frontal gyrus (12, 86).
The left and right orbital part of the inferior frontal gyrus (13, 87).
The left and right triangular part of the inferior frontal gyrus (14, 88).
AG: The left and right AG (25, 99).
TPJ: The left and right supramarginal gyrus (26, 100).
2.2.2 Phenotypic features of autism
The study uses three parent-reported questions with 5-point scales ranging from “Not at all” to “Nearly every day” related to PPFA taken from Module #18 (autism spectrum disorders) of the computerized Kiddie Schedule for Affective Disorders and Schizophrenia for DSM-5 (KSADS-COMP) (36). The questions include:
(1) “In the past two weeks, how often has your child had trouble maintaining eye contact and looking at you or other people when they are talking with your child?”
(2) “In the past two weeks, how often have you worked real hard to keep routines and activities the same so your child would not get upset?”
(3) “In the past two weeks, how often did your child do unusual body movements like hand flapping, head weaving, body rocking, or body spinning?”
2.3 Data analysis
We tested our hypothesis using confirmatory factor analysis (CFA) and structural equation modeling with the lavaan package in R (37). CFA for fMRI data has been an established approach with several advantages compared to data reduction techniques such as principal components analysis and partial least squares (38). We estimated all models using diagonally weighted least squares because of its appropriate use for ordinal data that do not meet the assumptions of non-normality (39), which is true of the features of autism data used in our study. We evaluated model fit using chi-square (χ2), Comparative Fit Index (CFI; values > .90 suggest acceptable fit and values > .95 suggest good fit), Tucker-Lewis Index (TLI; values > .90 suggest acceptable fit and values > .95 suggest good fit), standardized root mean residual (SRMR; values < .08 suggest good fit), root mean square error approximation (RMSEA; values < .08 suggest good fit).
Initially, we conducted CFAs in order to establish the viability of our latent measurements of PPFA as reported by parents and brain regions of interest, specifically the MPC, PCC, STS, IFG, AG, and the TPJ. We used the 22 parcellations from the Destrieux atlas as observed indicators for the six-factor CFA for the brain regions of the MPC, PCC, STS, IFG, AG, and TPJ. For our primary analysis, we regressed the latent factor of features of autism onto the six latent factors of the brain regions of interest and sex assigned at birth.
3 Results
3.1 Confirmatory factor analysis of PPFA
Figure 1 shows the results of the CFA for PPFA suggesting adequate model fit (CFI = 1.00, TLI = 1.00, SRMR = 0.00, RMSEA = 0.00, χ2 < 0.001) with loadings ranging from .28 to.50 (see Figure 1). Thus, the three parent-report questions from the K-SADS questionnaire appropriately form a latent construct characterized as PPFA.
3.2 Confirmatory factor analysis for brain regions of interest
Figure 2 shows the CFA for the brain regions of interest converging with an adequate model fit (CFI = .97, TLI = .96, SRMR = .08, RMSEA = .03, χ2 < .001), thus indicating the six brain regions of interest for our analysis: MPC, PCC, STS, IFG, AG, and TPJ. Correlations between the targeted brain regions ranged from moderate to large and were all positive in direction (see Table 2).
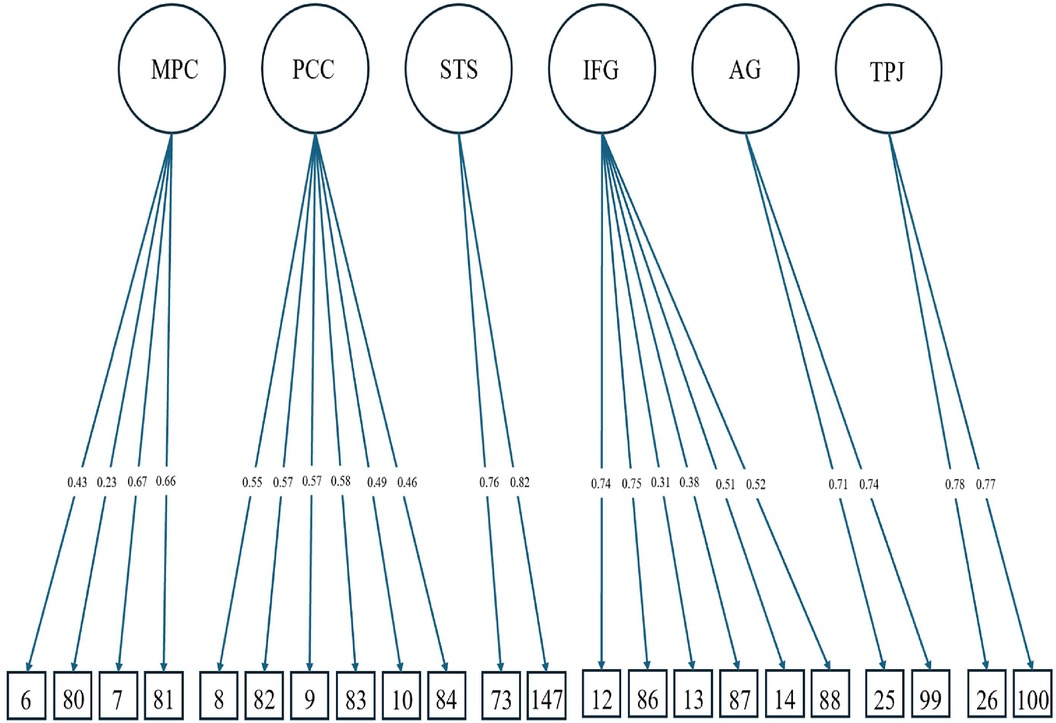
Figure 2. Model for six factor CFA for brain regions of interest with destrieux atlas cortical parcellations as the observed indicators. See Table 2 for correlations among latent factors. The parcellations numbers correspond with the Destrieux atlas’ index number as follows: the left and right anterior part of the cingulate gyrus and sulcus (6, 80), the left and right middle-anterior part of the cingulate gyrus and sulcus (7, 81), the left and right posterior part of the cingulate gyrus and sulcus (8, 82), the left and right posterior-dorsal part of the cingulate gyrus (9, 83), the left and right posterior-ventral part of the cingulate gyrus (10, 84), the left and right STS (73, 147), the left and right opercular part of the inferior frontal gyrus (12, 86), the left and right orbital part of the inferior frontal gyrus (13, 87), the left and right triangular part of the inferior frontal gyrus (14, 88), the left and right AG (25, 99), the left and right supramarginal gyrus (26, 100).
3.3 Regression analysis of selected brain regions and sex assigned at birth predicting PPFA
Figure 3 shows the results of the structural regression model, which converged with adequate fit (CFI = .94, TLI = .93, SRMR = .03, RMSEA = .07, χ2 < .001). The structural regression of a latent factor of PPFA regressed onto brain regions interest and sex assigned at birth showed activation in the MPC (β = .17, p < .05) and the STS (β = .08, p < .05) to be positively associated with PPFA. Additionally, being assigned male at birth was positively associated with PPFA (β = .13, p < .01). Conversely, activation in the IFG (β = −.08, p < .05) showed to be negatively associated with PPFA. There was not a significant association between the PCC (β = −.11, p = .13), AG (β = .02, p = .71), and TPJ (β = −.05, p = .19) with parent-reported PPFA in our youth cohort (see Figures 3, 4). Point-biserial correlations between sex assigned at birth and neurofunctional activity of the six brain regions showed that female participants have greater activation in all identified regions, and the difference was small in magnitude, ranging from .05 to .15 (see Table 2). The above reported PPFA–activation relationships in MPC, STS, and IFG regions were significant after controlling for neurofunctional differences between males and females.
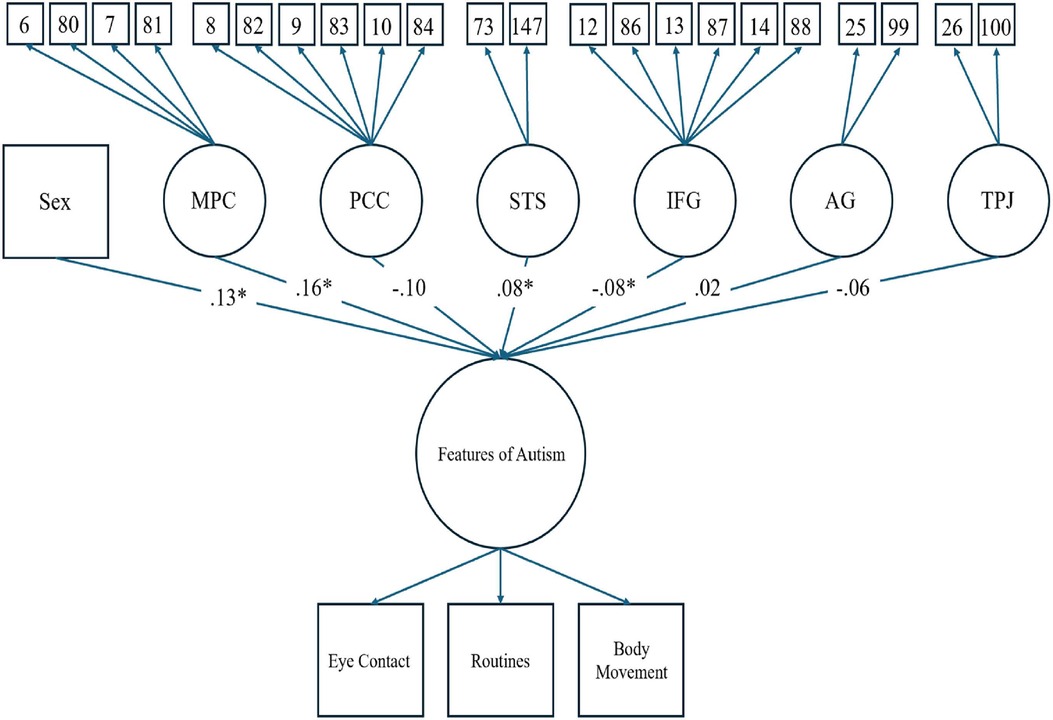
Figure 3. Full structural regression model (measurement and structural) for a latent factor of PPFA predicted by sex assigned at birth and six latent factors of brain regions of interest. Regression loadings from the six brain regions and sex assigned at birth are listed. An asterisk indicates a significant relationship at the .05 level. Loading values for the latent factor of features of autism and the six brain regions are identical to those from the preliminary CFAs (see Figures 1, 2).
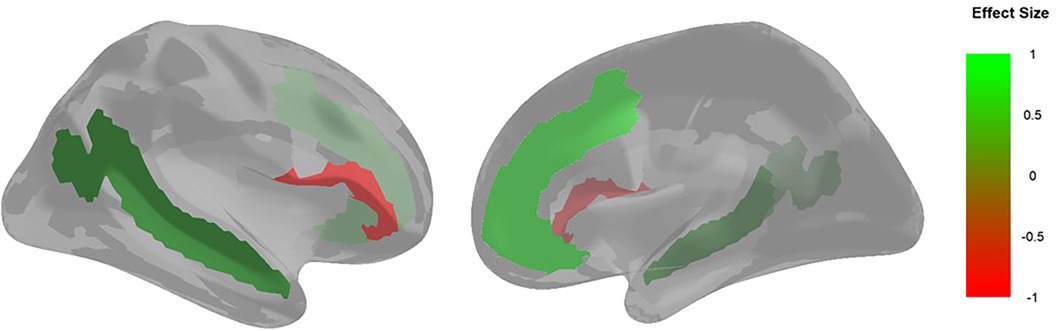
Figure 4. Brain visualization of areas with activation that are significantly associated with PPFA. The image on the left is a lateral view of the right hemisphere, and the image on the right is a medial view of the right hemisphere. The colors represent the standardized regression loadings from the structural regression model which are significant at the .05 level. Areas with a positive effect size (i.e., greater resting-state activation associated with PPFA) are green. Areas with a negative effect size (i.e., less resting-state activation associated with PPFA) are red.
4 Discussion
Our findings suggest that resting-state neurofunctional activity is indicative of PPFA in early adolescence. We found that greater resting-state activation in the MPC and the STS is associated with increased PPFA as reported by parents. These findings are concordant with literature that relates the MPC and the STS to self-referential thinking (20, 40), a cognitive process consistent with resting-state activity. Activation in areas related to self-referential thinking have been linked to a heightened focus on internal cues [i.e., thoughts and perceptions; (41)]. Difficulties in distinguishing between one's own thoughts and perspectives and those of others is a hallmark of autism that contributes to social challenges (42, 43). Furthermore, we found that less resting-state activation in the IFG is associated with increased PPFA. The IFG has been implicated in emotion recognition and regulation (44). Interestingly, there is evidence of IFG hypoactivation in early adolescence (around age 12) in youth with ASD, but no differences in IFG activation in adults with ASD compared to group-matched control adults (45). In this context, our finding of hypoactivation in the IFG being associated with greater PPFA in early adolescence is consistent with the literature (46–48) and builds upon previous findings as we find IFG hypoactivation during resting state in youth at ages 10–13 years.
Additionally, we find that anterior brain regions (MPC, STS, IFG) have a greater association with PPFA compared to posterior regions (PCC, AG, TPJ). This is in line with the posterior to anterior brain maturation pattern occurring during early adolescence through adulthood (28) and is consistent with altered fronto-posterior brain connectivity being associated with autistic features/traits (49). Thus, as frontal brain regions continue to mature, there is potential for a reduction in phenotypic features of autism observed in youth during early adolescence. We also found that being assigned male at birth was also associated with greater PPFA. This is consistent with sex differences in puberty onset (50) and brain maturation (51), with males showing later onset and maturation compared to females. Notably, this finding could also reflect differences between the presentation of features of autism, with females having displaying greater behavior camouflaging (52), thus less likely to be noticed and reported on the parent-report measure. Altogether, our results provide novel evidence that neural activation in DMN brain structures, which align with regions of the ToMN, during resting state are associated with PPFA in early adolescence.
Our study focuses on a cohort of preadolescents without a clinical diagnosis of ASD at baseline who express a range of parent-reported PPFA at the second-year follow-up. Therefore, our results infer how neurofunctional activity in regions of the DMN relates to PPFA among a diverse cohort of preadolescents, where PPFA was based on three questions, which cannot capture the full complexity and heterogeneity of ASD. Additionally, although the symptoms-measure scale was intended to capture features of ASD, the specific features (i.e., eye contact, maintaining a routine, and unusual body movement) can occur in conjunction with other neurodevelopmental disorders or psychopathologies, such as attention-deficit/hyperactivity disorder, anxiety, or depression. Therefore, these transdiagnostic features should not be understood as exclusive to ASD, but rather as indicators of neurodevelopment that is consistent with ASD features. Future research should aim to identify and disambiguate contributory factors that manifest as both distinct resting-state neurofunctional activity and transdiagnostic features of atypical psychosocial development. Furthermore, our study's focus on three questions related to PPFA limits the scope of how neurofunctional differences in the DMN impact functioning. Our findings should be extended with research investigating how differences in DMN activity relate to domains of functioning in adolescence, such as social engagement, academic performance, and emotion regulation.
Beyond limitations related to the measurement of features of autism, the interpretation of our study results is limited due to being cross-sectional. It is not understood if greater resting-state activation in the MPC and STS and less activation in the IFG can predict features of autism throughout adolescence and young adulthood. Longitudinal studies are warranted to characterize the trajectories of these examined brain regions, specifically of brain regions known to mature relatively later in development. Considering that alteration in the DMN has also been associated with social anxiety and other mental health disorders (53, 54), future research is needed to explore if intrinsic social brain activation pattern associated with PPFA during early adolescence in youth without a diagnosis of ASD is a risk factor for developing socioemotional mental health issues, such as social anxiety, during late adolescence and young adulthood. Overall, a better understanding of the neurofunctional substrates of features of autism will provide a better understanding of its heterogeneity, developmental timing, and targeted treatment needs.
Data availability statement
The data analyzed in this study is subject to the following licenses/restrictions: A data use agreement is needed to access the ABCD data and sharing of raw data to those without access is not allowed. Requests to access these datasets should be directed to https://nda.nih.gov/abcd.
Ethics statement
The studies involving humans were approved by Palo Alto University Institutional Review Board. The studies were conducted in accordance with the local legislation and institutional requirements. Written informed consent for participation in this study was provided by the participants’ legal guardians/next of kin.
Author contributions
RH: Conceptualization, Data curation, Formal Analysis, Investigation, Methodology, Visualization, Writing – original draft, Writing – review & editing. LH: Writing – review & editing. EM-O: Conceptualization, Supervision, Writing – review & editing, Methodology. AC: Visualization, Writing – review & editing. AH: Writing – review & editing. OK: Writing – review & editing. MG-L: Writing – review & editing. FB: Writing – review & editing, Supervision. TS: Conceptualization, Supervision, Writing – review & editing, Methodology.
Funding
The author(s) declare that no financial support was received for the research, authorship, and/or publication of this article.
Conflict of interest
The authors declare that the research was conducted in the absence of any commercial or financial relationships that could be construed as a potential conflict of interest.
Publisher's note
All claims expressed in this article are solely those of the authors and do not necessarily represent those of their affiliated organizations, or those of the publisher, the editors and the reviewers. Any product that may be evaluated in this article, or claim that may be made by its manufacturer, is not guaranteed or endorsed by the publisher.
References
1. Leekam S. Social cognitive impairment and autism: what are we trying to explain? Philos Trans R Soc B. (2016) 371(1686):20150082. doi: 10.1098/rstb.2015.0082
2. Lombardo MV, Barnes JL, Wheelwright SJ, Baron-Cohen S. Self-referential cognition and empathy in autism. PLoS One. (2007) 2(9):e883. doi: 10.1371/journal.pone.0000883
3. American Psychiatric Association. Diagnostic and Statistical Manual of Mental Disorders. 5th ed. Arlington, VA: American Psychiatric Association (2022). doi: 10.1176/appi.books.9780890425787
4. De Groot K, Van Strien JW. Evidence for a broad autism phenotype. Adv Neurodev Disord. (2017) 1:129–40. doi: 10.1007/s41252-017-0021-9
5. Lau WKW, Leung MK, Lau BWM. Resting-state abnormalities in autism spectrum disorders: a meta-analysis. Sci Rep. (2019) 9(1):3892. doi: 10.1038/s41598-019-40427-7
6. Hull JV, Dokovna LB, Jacokes ZJ, Torgerson CM, Irimia A, Van Horn JD. Resting-state functional connectivity in autism spectrum disorders: a review. Front Psychiatry. (2017) 7:205. doi: 10.3389/fpsyt.2016.00205
7. Assaf M, Jagannathan K, Calhoun VD, Miller L, Stevens MC, Sahl R, et al. Abnormal functional connectivity of default mode sub-networks in autism spectrum disorder patients. NeuroImage. (2010) 53(1):247–56. doi: 10.1016/j.neuroimage.2010.05.067
8. Padmanabhan A, Lynch CJ, Schaer M, Menon V. The default mode network in autism. Biol Psychiatr Cognit Neurosci Neuroimaging. (2017) 2(6):476–86. doi: 10.1016/j.bpsc.2017.04.004
9. Uddin LQ, Supekar K, Lynch CJ, Khouzam A, Phillips J, Feinstein C, et al. Salience network–based classification and prediction of symptom severity in children with autism. JAMA Psychiatry. (2013) 70(8):869. doi: 10.1001/jamapsychiatry.2013.104
10. Kleinhans NM, Johnson LC, Richards T, Mahurin R, Greenson J, Dawson G, et al. Reduced neural habituation in the amygdala and social impairments in autism spectrum disorders. Am J Psychiatry. (2009) 166(4):467–75. doi: 10.1176/appi.ajp.2008.07101681
11. Frye RE, Vassall S, Kaur G, Lewis C, Karim M, Rossignol D. Emerging biomarkers in autism spectrum disorder: a systematic review. Ann Transl Med. (2019) 7(23):792. doi: 10.21037/atm.2019.11.53
12. Long XY, Zuo XN, Kiviniemi V, Yang Y, Zou QH, Zhu CZ, et al. Default mode network as revealed with multiple methods for resting-state functional MRI analysis. J Neurosci Methods. (2008) 171(2):349–55. doi: 10.1016/j.jneumeth.2008.03.021
13. Yeshurun Y, Nguyen M, Hasson U. The default mode network: where the idiosyncratic self meets the shared social world. Nat Rev Neurosci. (2021) 22(3):181–92. doi: 10.1038/s41583-020-00420-w
14. Ilzarbe D, Lukito S, Moessnang C, O'Daly OG, Lythgoe DJ, Murphy CM, et al. Neural correlates of theory of mind in autism spectrum disorder, attention-deficit/hyperactivity disorder, and the comorbid condition. Front Psychiatry. (2020) 11:544482. doi: 10.3389/fpsyt.2020.544482
15. Mars RB, Neubert FX, Noonan MP, Sallet J, Toni I, Rushworth MF. On the relationship between the “default mode network” and the “social brain”. Front Hum Neurosci. (2012) 6:189. doi: 10.3389/fnhum.2012.00189
16. Soares C, Gonzalo G, Castelhano J, Castelo-Branco M. The relationship between the default mode network and the theory of mind network as revealed by psychedelics—a meta-analysis. Neurosci Biobehav Rev. (2023) 152:105325. doi: 10.1016/j.neubiorev.2023.105325
17. Kana RK, Maximo JO, Williams DL, Keller TA, Schipul SE, Cherkassky VL, et al. Aberrant functioning of the theory-of-mind network in children and adolescents with autism. Mol Autism. (2015) 6(1):1–12. doi: 10.1186/s13229-015-0052-x
18. Schurz M, Radua J, Tholen MG, Maliske L, Margulies DS, Mars RB, et al. Toward a hierarchical model of social cognition: a neuroimaging meta-analysis and integrative review of empathy and theory of mind. Psychol Bull. (2021) 147(3):293. doi: 10.1037/bul0000303
19. Frith CD. The social brain? Philos Trans R Soc B. (2007) 362(1480):671–8. doi: 10.1098/rstb.2006.2003
20. Burrows CA, Laird AR, Uddin LQ. Functional connectivity of brain regions for self-and other-evaluation in children, adolescents and adults with autism. Dev Sci. (2016) 19(4):564–80. doi: 10.1111/desc.12400
21. Ochsner KN, Knierim K, Ludlow DH, Hanelin J, Ramachandran T, Glover G, et al. Reflecting upon feelings: an fMRI study of neural systems supporting the attribution of emotion to self and other. J Cogn Neurosci. (2004) 16:1746–72. doi: 10.1162/0898929042947829
22. Spreng RN, Andrews-Hanna JR. The default network and social cognition. Brain Map Encycl Ref. (2015) 3(December 2015):165–9. doi: 10.1016/B978-0-12-397025-1.00173-1
23. Leech R, Smallwood J. The posterior cingulate cortex: insights from structure and function. Handb Clin Neurol. (2019) 166:73–85. doi: 10.1016/B978-0-444-64196-0.00005-4
24. Hein G, Knight RT. Superior temporal sulcus—it’s my area: or is it? J Cogn Neurosci. (2008) 20(12):2125–36. doi: 10.1162/jocn.2008.20148
25. Andrews JL, Ahmed SP, Blakemore SJ. Navigating the social environment in adolescence: the role of social brain development. Biol Psychiatry. (2021) 89(2):109–18. doi: 10.1016/j.biopsych.2020.09.012
26. Gerván P, Soltész P, Filep O, Berencsi A, Kovács I. Posterior–anterior brain maturation reflected in perceptual, motor and cognitive performance. Front Psychol. (2017) 8:674. doi: 10.3389/fpsyg.2017.00674
27. O’Hearn K, Schroer E, Minshew N, Luna B. Lack of developmental improvement on a face memory task during adolescence in autism. Neuropsychologia. (2010) 48(13):3955–60. doi: 10.1016/j.neuropsychologia.2010.08.024
28. O’Hearn K, Lynn A. Age differences and brain maturation provide insight into heterogeneous results in autism spectrum disorder. Front Hum Neurosci. (2023) 16:957375. doi: 10.3389/fnhum.2022.957375
29. Wen T, Mitchell DJ, Duncan J. The functional convergence and heterogeneity of social, episodic, and self-referential thought in the default mode network. Cerebral Cortex. (2020) 30(11):5915–29. doi: 10.1093/cercor/bhaa166
30. Fan F, Liao X, Lei T, Zhao T, Xia M, Men W, et al. Development of the default-mode network during childhood and adolescence: a longitudinal resting-state fMRI study. NeuroImage. (2021) 226:117581. doi: 10.1016/j.neuroimage.2020.117581
31. Garavan H, Bartsch H, Conway K, Decastro A, Goldstein RZ, Heeringa S, et al. Recruiting the ABCD sample: design considerations and procedures. Dev Cogn Neurosci. (2018) 32:16–22. doi: 10.1016/j.dcn.2018.04.004
32. Destrieux C, Fischl B, Dale A, Halgren E. Automatic parcellation of human cortical gyri and sulci using standard anatomical nomenclature. Neuroimage. (2010) 53(1):1–15. doi: 10.1016/j.neuroimage.2010.06.010
33. Casey BJ, Cannonier T, Conley MI, Cohen AO, Barch DM, Heitzeg MM, et al. The adolescent brain cognitive development (ABCD) study: imaging acquisition across 21 sites. Dev Cogn Neurosci. (2018) 32:43–54. doi: 10.1016/j.dcn.2018.03.001
34. Hagler DJ, Hatton SN, Cornejo MD, Makowski C, Fair DA, Dick AS, et al. Image processing and analysis methods for the adolescent brain cognitive development study. NeuroImage. (2019) 202:116091. doi: 10.1016/j.neuroimage.2019.116091
35. Hu J, Du J, Xu Q, Yang F, Zeng F, Weng Y, et al. Dynamic network analysis reveals altered temporal variability in brain regions after stroke: a longitudinal resting-state fMRI study. Neural Plast. (2018) 2018:1–10. doi: 10.1155/2018/9394156
36. Barch DM, Albaugh MD, Baskin-Sommers A, Bryant BE, Clark DB, Dick AS, et al. Demographic and mental health assessments in the adolescent brain and cognitive development study: updates and age-related trajectories. Dev Cogn Neurosci. (2021) 52(2021):101031. doi: 10.1016/j.dcn.2021.101031
37. Rosseel Y. Lavaan: an R package for structural equation modeling. J Stat Softw. (2012) 48(2):1–36. doi: 10.18637/jss.v048.i02
38. Lahey BB, McNealy K, Knodt A, Zald DH, Sporns O, Manuck SB, et al. Using confirmatory factor analysis to measure contemporaneous activation of defined neuronal networks in functional magnetic resonance imaging. Neuroimage. (2012) 60(4):1982–91. doi: 10.1016/j.neuroimage.2012.02.002
39. Mindrila D. Maximum likelihood (ML) and diagonally weighted least squares (DWLS) estimation procedures: a comparison of estimation bias with ordinal and multivariate non-normal data. Int J Digit Soc. (2010) 1(1):60–6. doi: 10.20533/ijds.2040.2570.2010.0010
40. Gusnard DA, Akbudak E, Shulman GL, Raichle ME. Medial prefrontal cortex and self-referential mental activity: relation to a default mode of brain function. Proc Natl Acad Sci U S A. (2001) 98(7):4259–64. doi: 10.1073/pnas.071043098
41. Whitfield-Gabrieli S, Ford JM. Default mode network activity and connectivity in psychopathology. Annu Rev Clin Psychol. (2012) 8(1):49–76. doi: 10.1146/annurev-clinpsy-032511-143049
42. Gökçen E, Frederickson N, Petrides KV. Theory of mind and executive control deficits in typically developing adults and adolescents with high levels of autism traits. J Autism Dev Disord. (2016) 46:2072–87. doi: 10.1007/s10803-016-2735-3
43. Lombardo MV, Chakrabarti B, Bullmore ET, Wheelwright SJ, Sadek SA, Suckling J, et al. Shared neural circuits for mentalizing about the self and others. J Cogn Neurosci. (2010) 22(7):1623–35. doi: 10.1162/jocn.2009.21287
44. Wada S, Honma M, Masaoka Y, Yoshida M, Koiwa N, Sugiyama H, et al. Volume of the right supramarginal gyrus is associated with a maintenance of emotion recognition ability. PLoS One. (2021) 16(7):e0254623. doi: 10.1371/journal.pone.0254623
45. Bastiaansen JA, Thioux M, Nanetti L, van der Gaag C, Ketelaars C, Minderaa R, et al. Age-related increase in inferior frontal gyrus activity and social functioning in autism spectrum disorder. Biol Psychiatry. (2011) 69(9):832–8. doi: 10.1016/j.biopsych.2010.11.007
46. Bookheimer SY, Wang AT, Scott A, Sigman M, Dapretto M. Frontal contributions to face processing differences in autism: evidence from fMRI of inverted face processing. J Int Neuropsychol Soc. (2008) 14(6):922–32. doi: 10.1017/S135561770808140X
47. Dapretto M, Davies MS, Pfeifer JH, Scott AA, Sigman M, Bookheimer SY, et al. Understanding emotions in others: mirror neuron dysfunction in children with autism spectrum disorders. Nat Neurosci. (2006) 9(1):28–30. doi: 10.1038/nn1611
48. Uddin LQ, Davies MS, Scott AA, Zaidel E, Bookheimer SY, Iacoboni M, et al. Neural basis of self and other representation in autism: an fMRI study of self-face recognition. PLoS One. (2008) 3(10):e3526. doi: 10.1371/journal.pone.0003526
49. Tarasi L, Magosso E, Ricci G, Ursino M, Romei V. The directionality of fronto-posterior brain connectivity is associated with the degree of individual autistic traits. Brain Sci. (2021) 11(11):1443. doi: 10.3390/brainsci11111443
50. Torvik FA, Flatø M, McAdams TA, Colman I, Silventoinen K, Stoltenberg C. Early puberty is associated with higher academic achievement in boys and girls and partially explains academic sex differences. J Adolesc Health. (2021) 69(3):503–10. doi: 10.1016/j.jadohealth.2021.02.001
51. Kurth F, Gaser C, Luders E. Development of sex differences in the human brain. Cogn Neurosci. (2021) 12(3–4):155–62. doi: 10.1080/17588928.2020.1800617
52. Wood-Downie H, Wong B, Kovshoff H, Mandy W, Hull L, Hadwin JA. Sex/gender differences in camouflaging in children and adolescents with autism. J Autism Dev Disord. (2021) 51:1353–64. doi: 10.1007/s10803-020-04615-z
53. Sheline YI, Barch DM, Price JL, Rundle MM, Vaishnavi SN, Snyder AZ, et al. The default mode network and self-referential processes in depression. Proc Natl Acad Sci U S A. (2009) 106(6):1942–7. doi: 10.1073/pnas.0812686106
Keywords: rs-fMRI (resting state fMRI), default mode network (DMN), features of autism spectrum, preadolescence, neuroactivation
Citation: Hickson R, Hebron L, Muller-Oehring EM, Cheu A, Hernandez A, Kiss O, Gombert-Labedens M, Baker FC and Schulte T (2024) Resting-state fMRI activation is associated with parent-reported phenotypic features of autism in early adolescence. Front. Child Adolesc. Psychiatry 3:1481957. doi: 10.3389/frcha.2024.1481957
Received: 17 August 2024; Accepted: 21 October 2024;
Published: 5 November 2024.
Edited by:
Sara Carucci, Binaghi Hospital, ItalyReviewed by:
Peter Nagy, Bethesda Children’s Hospital, HungaryValeria Parlatini, University of Southampton, United Kingdom
Chiara Scoppola, University of Rome Tor Vergata, Italy
Copyright: © 2024 Hickson, Hebron, Muller-Oehring, Cheu, Hernandez, Kiss, Gombert-Labedens, Baker and Schulte. This is an open-access article distributed under the terms of the Creative Commons Attribution License (CC BY). The use, distribution or reproduction in other forums is permitted, provided the original author(s) and the copyright owner(s) are credited and that the original publication in this journal is cited, in accordance with accepted academic practice. No use, distribution or reproduction is permitted which does not comply with these terms.
*Correspondence: Robert Hickson, aGlja3NvbjQ5MkBnbWFpbC5jb20=; Tilman Schulte, dGlsbWFuLnNjaHVsdGVAc3JpLmNvbQ==