- 1Center for Biological Research Margarita Salas, Spanish National Research Council (CSIC), Madrid, Spain
- 2National Center for Biotechnology, Spanish National Research Council (CSIC), Madrid, Spain
- 3Institute of Mathematical Sciences, Spanish National Research Council (CSIC), Madrid, Spain
- 4Department of Cellular Biology, Genetics, and Physiology, University of Malaga, Málaga, Spain
- 5Mechatronics and Interactive Systems - MIST Research Center, Universidad Tecnológica Indoamérica, Quito, Ecuador
Introduction: SARS-CoV-2 variants are defined by specific genome-wide mutations compared to the Wuhan genome. However, non-clade-defining mutations may also impact protein structure and function, potentially leading to reduced vaccine effectiveness. Our objective is to identify mutations across the entire viral genome rather than focus on individual mutations that may be associated with vaccine failure and to examine the physicochemical properties of the resulting amino acid changes.
Materials and methods: Whole-genome consensus sequences of SARS-CoV-2 from COVID-19 patients were retrieved from the GISAID database. Analysis focused on Dataset_1 (7,154 genomes from Italy) and Dataset_2 (8,819 sequences from Spain). Bioinformatic tools identified amino acid changes resulting from codon mutations with frequencies of 10% or higher, and sequences were organized into sets based on identical amino acid combinations.
Results: Non-defining mutations in SARS-CoV-2 genomes belonging to clades 21 L (Omicron), 22B/22E (Omicron), 22F/23A (Omicron) and 21J (Delta) were associated with vaccine failure. Four sets of sequences from Dataset_1 were significantly linked to low vaccine coverage: one from clade 21L with mutations L3201F (ORF1a), A27- (S) and G30- (N); two sets shared by clades 22B and 22E with changes A27- (S), I68- (S), R346T (S) and G30- (N); and one set shared by clades 22F and 23A containing changes A27- (S), F486P (S) and G30- (N). Booster doses showed a slight improvement in protection against Omicron clades. Regarding 21J (Delta) two sets of sequences from Dataset_2 exhibited the combination of non-clade mutations P2046L (ORF1a), P2287S (ORF1a), L829I (ORF1b), T95I (S), Y145H (S), R158- (S) and Q9L (N), that was associated with vaccine failure.
Discussion: Vaccine coverage associations appear to be influenced by the mutations harbored by marketed vaccines. An analysis of the physicochemical properties of amino acid revealed that primarily hydrophobic and polar amino acid substitutions occurred. Our results suggest that non-defining mutations across the proteome of SARS-CoV-2 variants could affect the extent of protection of the COVID-19 vaccine. In addition, alteration of the physicochemical characteristics of viral amino acids could potentially disrupt protein structure or function or both.
1 Introduction
Severe acute respiratory syndrome coronavirus 2 (SARS-CoV-2), first reported in Wuhan (China) in late 2019, soon spread around the globe. To date, more than 700 million people have been infected and 6.97 million have died worldwide. Despite the availability of vaccines, SARS-CoV-2 remains a cause for concern. Like other RNA viruses, SARS-CoV-2 is endowed with a high mutation rate and high viral load and accumulates mutations with each replication cycle. As a result, viral variants that differ in one or more nucleotides are continuously being generated in infected hosts (1–3). The nomenclature of SARS-CoV-2 variants plays a critical role in facilitating their clear identification, tracking, and global collaboration in understanding the evolutionary dynamics of the virus. For Nextstrain clade naming, a new major clade earns its designation once it attains a 20% frequency on a global scale, regardless of the time frame. When computing these frequencies, it is crucial to ensure a relatively uniform sampling of sequences across different times and geographical locations due to the considerable disparity in sequencing efforts among countries. Clade names are formulated using a standardized protocol, typically derived from the year of emergence and the subsequent available letter in the alphabet. Additionally, a newly identified clade must exhibit a minimum of two mutations differentiating it from its parent major clade. This systematic approach ensures consistency and accuracy in clade designation, facilitating the clear identification and tracking of viral lineages in genomic surveillance studies. SARS-CoV-2 has undergone several genetic mutations leading to the emergence of various clades since its first identification in 2019. A recent study using Belgian data revealed an enhanced immune escape capability exhibited by the Omicron variant compared to the Alpha and Delta variants, resulting in a substantial reduction in the protective efficacy conferred by both acquired immunity and vaccination. Furthermore, a decline in vaccine effectiveness over time was observed, underscoring the significance of booster doses to sustain long-term immunity (4).
In the Netherlands population, the effectiveness of primary and booster vaccination against SARS-CoV-2 infection was estimated overall and in four risk groups defined by age and medical conditions during the Delta and Omicron BA.1/BA.2 periods. The findings underscored the advantages of booster vaccinations in reducing infection rates, particularly within at-risk groups (5).
Investigations of the complete of SARS-CoV-2 proteome have often been limited to analyzing only a few sequences or individual sequences, rather than taking advantage of a large sequencing dataset. However, a direct RNA sequencing approach was employed to assess the SARS-CoV-2 transcriptome in Vero E6 cells, and mass spectrometry was used to explore the proteome and phosphoproteome of these virus-infected cells (6). Furthermore, a proteomic analysis of proteins extracted from nasopharyngeal swabs of 12 patients diagnosed with COVID-19 identified 13 different SARS-CoV-2 proteins. Additionally, host proteome analysis revealed that several key host proteins were uniquely expressed in patients with COVID-19 (7). In a separate study, distinct epitopes of seven different proteins were identified using the complete SARS-CoV-2 virus genome obtained from the NCBI database. However, the 12 protein sequences of the genome were formatted as FASTA files using RefSeq accessions (8). Furthermore, a proteome-wide study of SARS-CoV-2 assessed its potential to induce autoimmune diseases by segmenting the proteome into peptides and identifying shared peptides with experimentally confirmed human T-cell and B-cell epitopes (9).
The SARS-CoV-2 genome consists of a single-stranded, unsegmented, positive-polarity RNA molecule [(+) ssRNA] 29,903 nucleotides in length encoding 13 ORFs (10). Two-thirds of the viral genome corresponds to ORF1a and ORF1b, which express the two polyproteins pp1a and pp1ab, the latter though a − 1 ribosomal frameshift, and that are processed by two viral proteases into 16 non-structural proteins (nsp). ORF1a encodes nsp1 to nsp11 and ORF1b comprises nsp12 to nsp16. Non-structural proteins make up the replication and transcription machinery and are responsible for the maintenance of the viral genome (11). Some nsp proteins are targets for antiviral drugs such as nsp12, the RNA-dependent RNA polymerase (RdRp), and nsp5, the 3C-like protease (Mpro, 3CLpro) (12). In addition, nsp3, the papain-like protease (PLpro) is also a therapeutical target for antivirals (13).
The structural proteins are, namely, the surface glycoprotein or spike (S), the envelope protein (E), the membrane glycoprotein (M), and the nucleocapsid phosphoprotein (N). The S protein has been shown to play a major role in virus attachment and entry into cells, being a key antigen for development of vaccines and neutralizing antibodies, and as a pharmacological target (14–16). The E protein plays a key role in the pathogenesis of the virus affecting the binding of SARS-CoV-2 to the tight junction proteins (17). The M protein is responsible for maintaining the shape of the virion by spanning the membrane bilayer and facilitates budding of the viral particles from the host cells (18). Interestingly, the M protein was found to elicit IgM response during the acute phase of SARS-CoV-2 infection (19). The nucleocapsid function is to maintain the genome structure inside the envelope (20). The N protein has been identified as an important target for T-cell response, making it a suitable candidate for next-generation COVID-19 vaccines against emerging variants (21–23).
There are nine accessory proteins, ORF3a, 3d, 6, 7a, 7b, 8, 9b, 14, and 10, which are produced from the encoding accessory genes ORF3a, ORF3d, ORF6, ORF7a, ORF7b, ORF8, ORF9b, ORF14 and ORF10, respectively (24). Although the exact functions of SARS-CoV-2 accessory proteins remain to be determined, studies of other coronaviruses suggest that they are not essential for viral replication but can modulate replication and pathogenesis through interaction with host pathways including antiviral activation (25, 26) and viral translation (27).
The existing literature predominantly concentrates on isolated mutations within the S protein rather than examining mutations as a network involving multiple proteins. Individually, mutations in SARS-CoV-2 might not pose significant risks, however their collective effect in tandem with other mutations could amplify the virus’s transmissibility and virulence. Consequently, relying solely on information about distinct segments of the virus might provide an incomplete understanding. Therefore, a comprehensive study of mutations across the entire SARS-CoV-2 genome and proteome becomes essential. Such a holistic approach is critical in understanding the virus’s mechanisms for evading vaccines and is instrumental in the development of effective vaccines and therapies.
Mutations (including deletions) that alter protein sequence, may affect physicochemical properties and folding conformation of proteins resulting in changes in biological functions. Amino acid positions in proteins could be assigned a single conservation number based on the alignment of homologous proteins for quantification of amino acid substitutions (28, 29).
Given that structural variations due to mutations could affect vaccine effectiveness and drug function, and thus the severity of COVID-19, this work involved an extensive genome-wide mutation combination analysis of SARS-CoV-2 in vaccinated patients from Italian and Spanish populations as two independent datasets. Analyzing more than 7,500 proteomes from each population increases the statistical power and reliability of the results. Our objective was to identify mutations across the entire viral genome, rather than focusing on individual mutations, that may be associated with vaccine failure, in addition to analyze the physicochemical properties of the resulting amino acid changes. This study involved aligning SARS-CoV-2 genomic sequences from both vaccinated and unvaccinated COVID-19 patients using the Wuhan genome as reference. We examined the frequency of specific mutation sets and analyzed their physicochemical properties to understand how these mutations may affect the structure and function of viral proteins. This approach provides a comprehensive view of the genetic diversity of SARS-CoV-2 variants circulating in different geographic regions and contributes to a deeper understanding of the underlying mechanisms of the vaccine effectiveness, which is crucial for informing public health strategies and vaccine development efforts.
2 Materials and methods
2.1 SARS-CoV-2 genome sequences
Sequences of COVID-19 patients were retrieved from the Global Initiative on Sharing All Influenza Data (GISAID) (30): (a) Dataset_1 contained 7,154 aligned consensus sequences of SARS-CoV-2 genomes isolated from patients of Friuli-Venezia Giulia (Italy) from January 01, 2021 to June 24, 2023. Of these, 2,419 were fully vaccinated and 1,667 received a booster dose vaccination against the Omicron variant; (b) Dataset_2 contained 8,819 aligned genomes mainly from Catalonia (Spain) since January 01, 2021 to July 25, 2022. Of those, 2,969 were completely vaccinated and 699 received the third COVID-19 vaccine dose against Omicron. Table 1 shows a description of the datasets. Datasets are available in the Supplementary material.
The NetAlign CLI software was used for sequence alignment (version 2.4.0) (31). Genome and coding sequences (CDS) of SARS-CoV-2 Wuhan-Hu-1 reference sequence NC_045512.2 were retrieved from GenBank. SARS-CoV-2 variants and mutations were sourced from the CoVariant website,1 which employs the Nextstrain nomenclature for identification of variants.
2.2 Mutations of interest
Changes in genome, including indeterminations during sequencing base calling, were referred to as non-synonymous or missense mutations when the codons contained mutations (substitutions or deletions) with a frequency of 10% or higher in the genomic sequence alignments. These were referred as mutation of interest (MOI). Sequences sharing the same combination of MOIs (haplotypes) were grouped together into set of sequences. Non-defining mutations were also referred to as additional MOIs.
2.3 Bioinformatic tools and statistical analysis
To identify amino acid changes within SARS-CoV-2 genomes, codon translation in coding sequences (CDS) was accomplished using scripts written in the R programming language (version 4.1.0) (32) and Python (version 3.8.11) (33). The Biopython library (version 1.76) (34) was employed for managing the amino acid alphabet. Qualitative variable analysis was performed using the Chi-square test from the scipy.stats Python module (scipy version 1.9.3) (35). We employed the trackViewer package (version 1.34.0) (36) for creating visual representations of mutations in SARS-CoV-2 proteins. Furthermore, EMBOSS Seqret (version 6.6.0.0) (37) was employed to record significant mutation combinations in mega non-interleaved output format. Data manipulation and analysis were carried out using libraries like Matplotlib (version 3.5.1) (38) and Pandas (version 1.2.3) (39). In-house scripts used in this study were developed by the authors. Scripts used in our analysis are openly available at: https://github.com/papersarscov2proteome/.
Undetermined amino acids and special characters from the Biopython dictionary (B, Z, J, U, and O) were represented as ‘X.’ Sets containing ‘X’ were excluded from further analysis, while those with a frequency of 1% or greater were retained for statistical examination. Statistical significance was determined with p values <0.05 (following False Discovery Rate (FDR) correction) (40). Vaccine coverage was identified when expected frequencies exceeded observed frequencies.
Genetic distances estimation within each sequence set was carried out using MEGA (41). The mean distance was calculated using the Bootstrap method for variance estimation, with 1,000 bootstrap replications, employing the p-distance model for amino acid substitution type. Ambiguous positions were eliminated for each sequence pair using the pairwise deletion option.
2.4 Patients
We categorized individuals as having complete active immunity or fully vaccinated (FV) as those who had received a minimum of 2 doses (or 1 dose in the case of the Janssen vaccine) at least 14 days prior to infection, regardless of the specific infectious variant. For FV patients who were infected by the Omicron variant, those who had received a third COVID-19 vaccine dose (or a second dose of Janssen vaccine) were designated as booster patients. Vaccines referred to as others include Sinovac and Sinopharm. Individuals who did not meet any of the above criteria were classified as not fully vaccinated.
2.5 Residue conservation
Conservation analysis stands as one of the most widely used methods for predicting functionally significant residues in protein sequences. Residue conservation, as defined by Livingstone et al. (29), employs two distinct methods to quantify a singular conservation score for each position. For both, the physicochemical properties assessed for the 20 amino acids take into account whether the molecules are hydrophobic, polar, small, proline, tiny, aliphatic, aromatic, positive, negative and charged. In this study, we used the method 1 which considers any property exhibiting positive or negative conservation. A deletion is considered to possess all of these properties for the conservation index calculation. In this work high conservation index refers around a range of 10–8, intermediate to 7 and 6, and values equal to or less than 5 with low conservation.
3 Results
3.1 Datasets description
For Dataset_1, we analyzed a total of 7,154 SARS-CoV-2 genome aligned consensus sequences. Out of these, 2,419 were from fully vaccinated (FV) COVID-19 patients, and 2097 had been infected with the Omicron variant (Table 1). In the FV group (comprising 2,419 out of 7,154 individuals), 59.9% had received the Pfizer vaccine, followed by 34.2% who had received the Moderna vaccine. A negligible proportion, less than 5%, had received the J&J-Janssen and Oxford-AstraZeneca vaccines. The predominant variant across several clades was Omicron, representing 50% of the total population (Table 1). Figure 1 illustrates the flowchart followed.
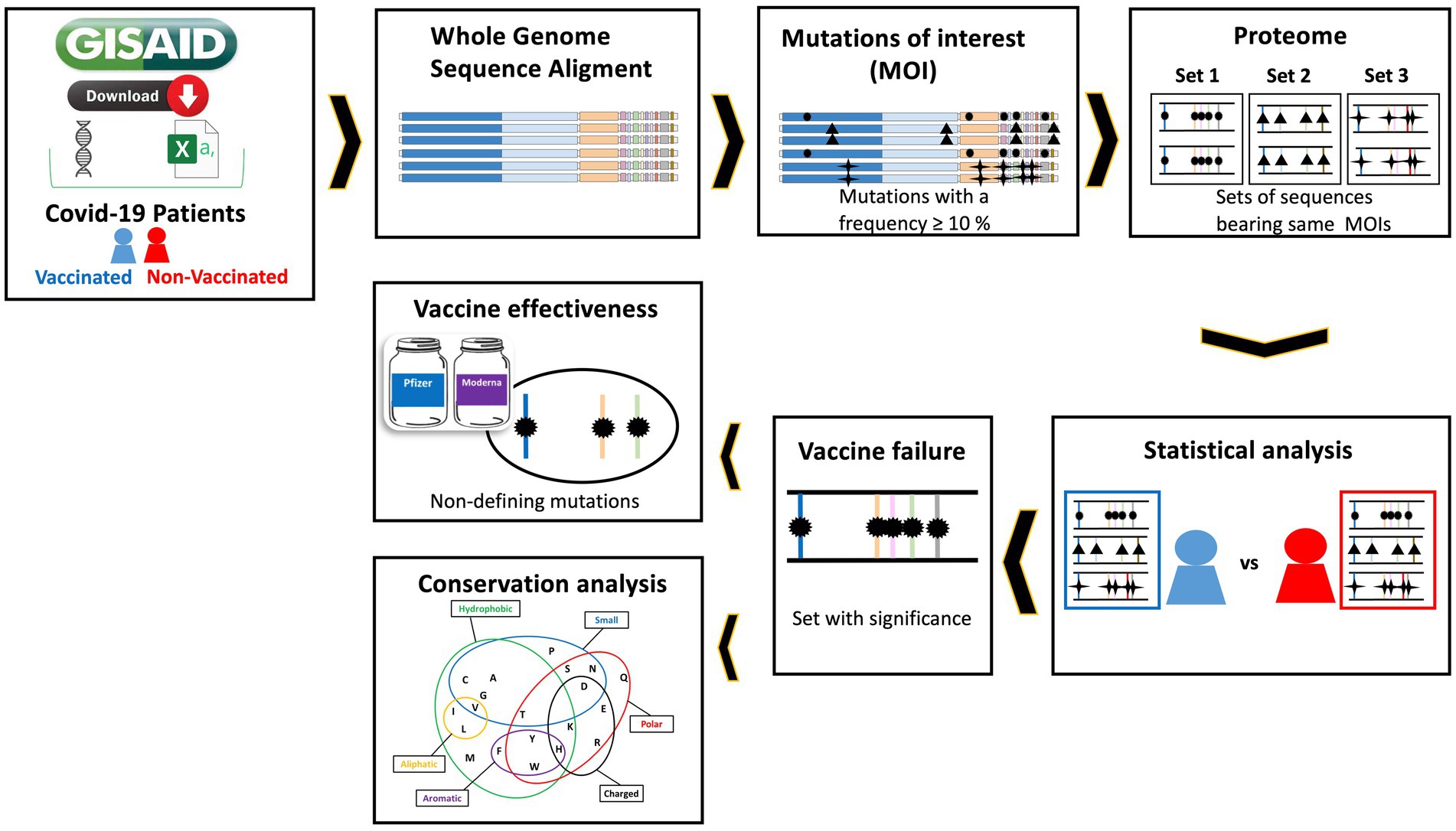
Figure 1. Flowchart of the methodology used in this study. 1. Sequences and metadata of vaccinated and unvaccinated COVID-19 patients from Italy and Spain were downloaded from GISAID. 2. The SARS-CoV-2 genome sequences of the COVID-19 patients were aligned to the Wuhan reference genome. 3. The mutations of interest (MOIs) are then defined. 4. Next, the proteomes are obtained and those sequences that have the same combination of MOIs are grouped together. 5 and 6. Statistical analysis is now performed using a Chi-square test to identify sets of sequences between fully vaccinated and unvaccinated patients that are associated with vaccine failure. 7. The role of non-clade-defining mutations in the risk of vaccine effectiveness is then investigated by comparing populations. 8. Finally, the physicochemical properties of the MOIs in the sequence sets associated with vaccine failure are analyzed.
We identified 119 MOIs which combinations resulted in eight sets of sequences with a frequency greater than 1% ranging from Set1_ds1 to Set8_ds1 (Figure 2; Supplementary Figure S2A). For the sequence sets associated with vaccine failure, the timeline revealed a peak of cases belonging to clade 21L (Omicron) in May 2022, as shown by Set5_ds1. However, clades 22B and 22E of the Omicron variant exhibited two peaks, observed in Set6_ds1 and Set7_ds1 in September and December, respectively. By April 2023, the majority of infections were attributed to clades 22F and 23A of the Omicron variant. Detailed information on the clades associated with each set of sequences is provided in Tables 2, 3.
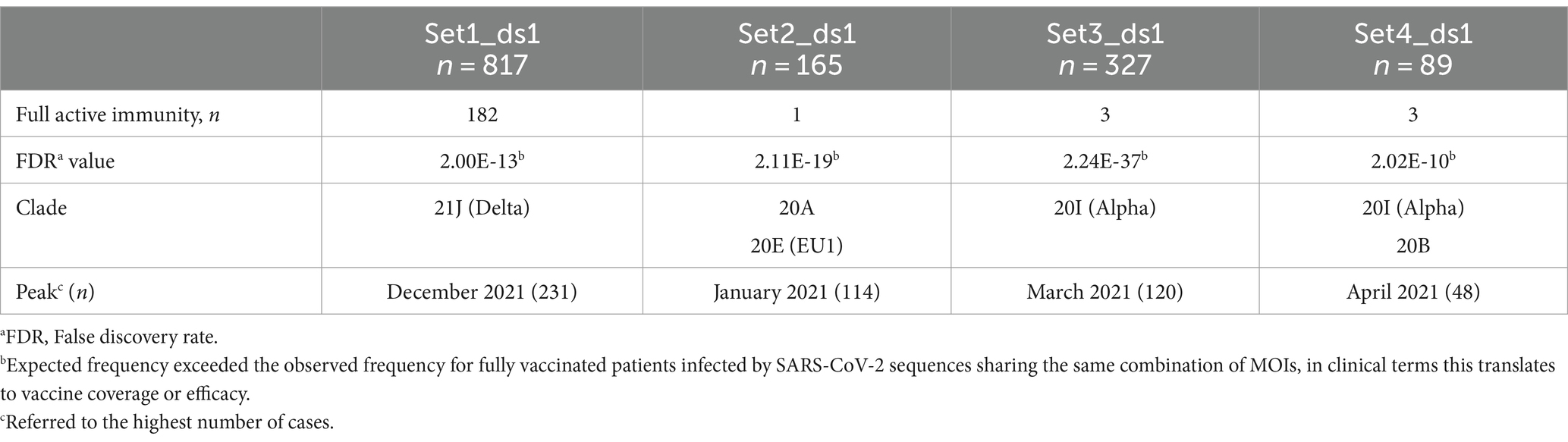
Table 2. Descriptive statistics of non-Omicron set of sequences within Dataset_1, comparing fully vaccinated and non-fully vaccinated COVID-19 patients.
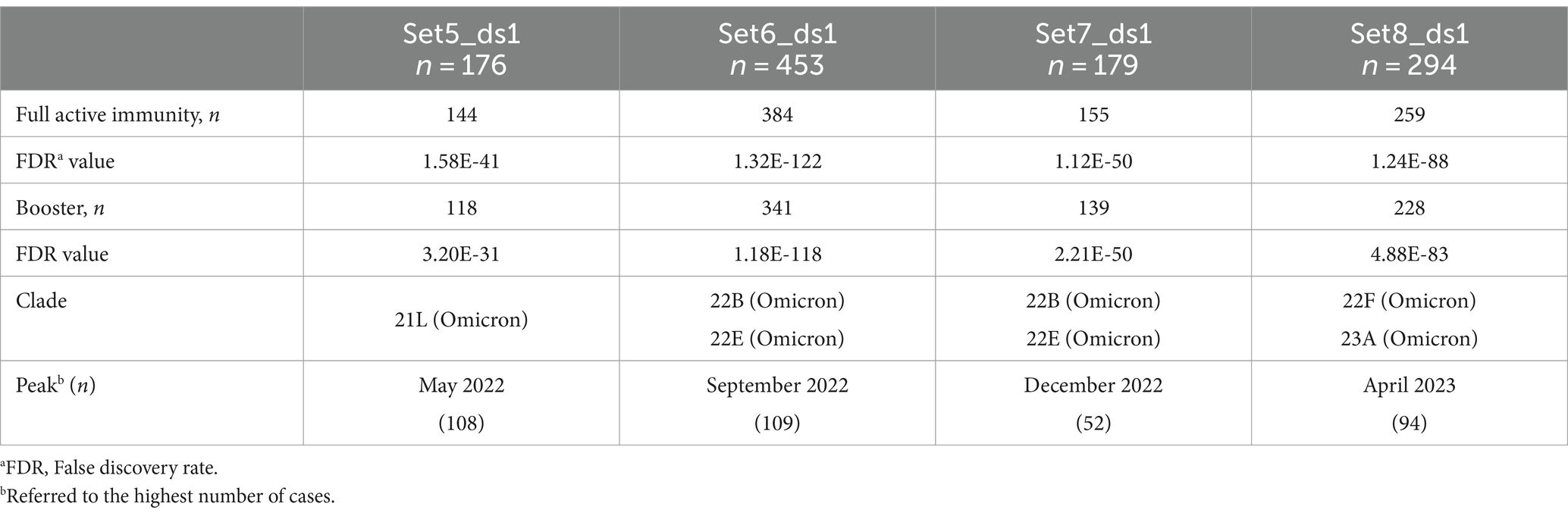
Table 3. Descriptive statistics of Omicron set of sequences of Dataset_1, comparing fully vaccinated and booster doses with non-fully vaccinated COVID-19 patients.
For Dataset_2, we analyzed aligned consensus sequences from 8,819 COVID-19 patients. Of these, 2,969 were from fully vaccinated patients with 2,163 of them infected with non-Omicron variants (Table 1). Pfizer was the most frequently administered vaccine (74.0%), followed by AstraZeneca (17.3%). Infection with the 21J (Delta) variant occurred in 51.3% of cases (Table 1).
Nine sets of sequences were derived from the 119 MOIs, as it is depicted in Figure 3 and Supplementary Figure S2B. The incidence of SARS-CoV-2 infection for the sets significantly associated with vaccine failure displayed two peaks, in July 2021 and November 2021, during the 21J (Delta) wave (Tables 4, 5).
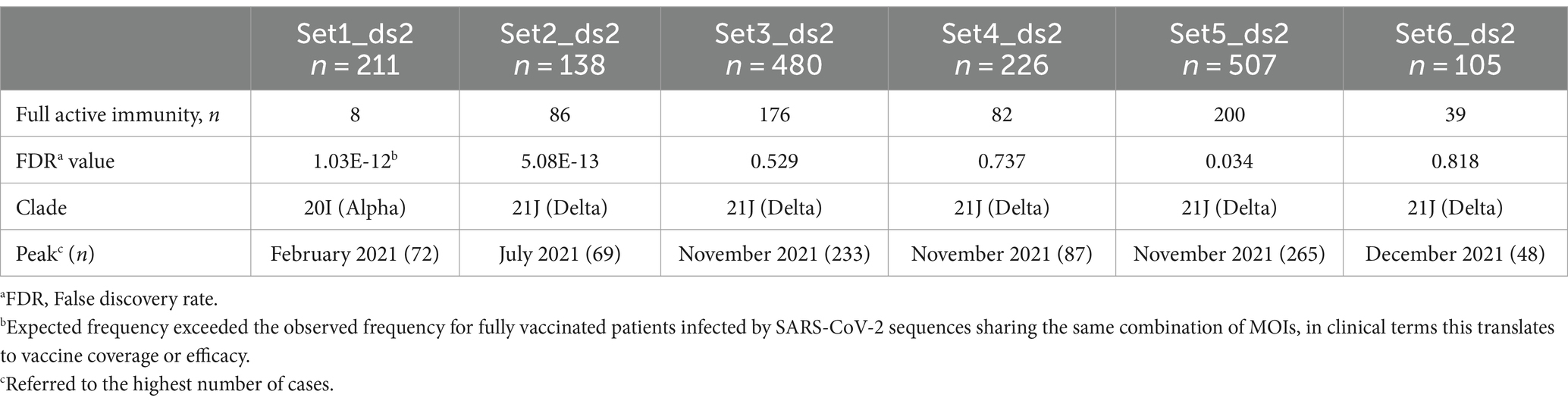
Table 4. Descriptive statistics for non-Omicron sets in Dataset_2 among fully vaccinated and non-fully vaccinated COVID-19 patients.
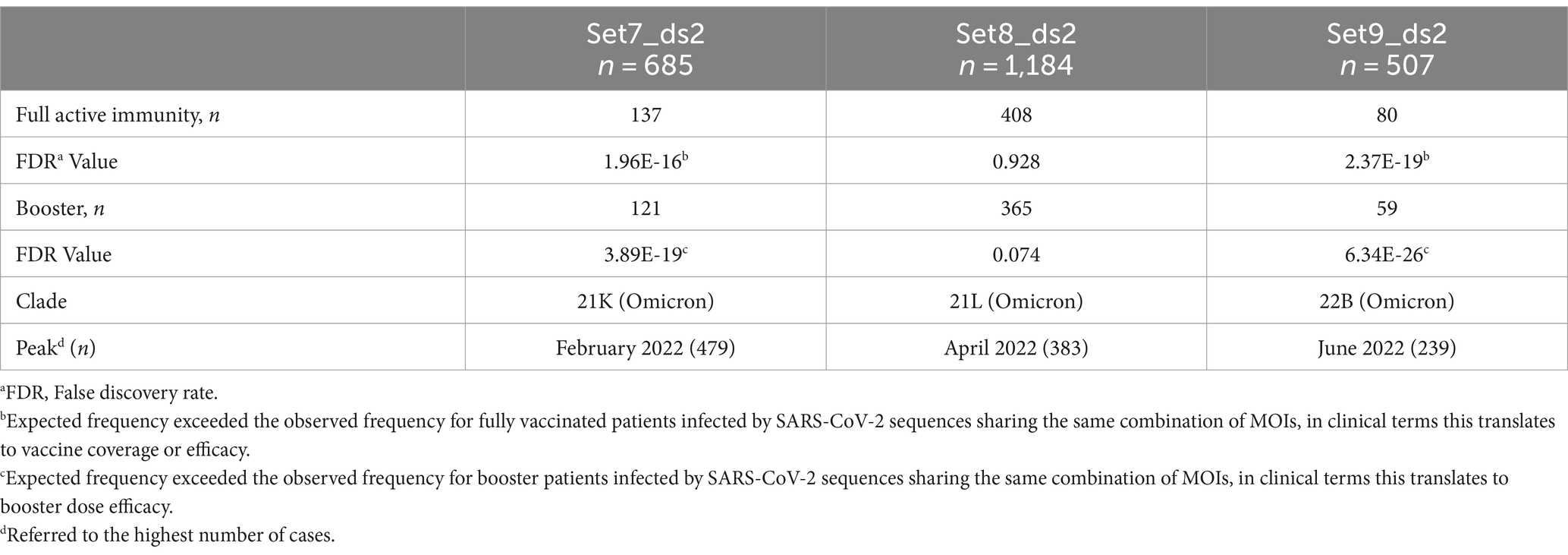
Table 5. Descriptive statistics comparing fully vaccinated and booster-dosed COVID-19 patients with those who are not fully vaccinated for Omicron sets of sequences.
Additional timeline information for these sequence sets is available in the Supplementary material, provided as images named SetNumber_Dataset_TotalNumber.png.
When comparing both datasets, we identified seven MOIs that were exclusively present in Dataset_1. These included T3255I (ORF1a), E3965E (ORF1a), I4205I (ORF1a), R346T (S), T478K (S), F486V (S), F486P (S), and F8F (ORF3a). Five of these mutations were defining mutations of the Omicron clades (T3255I (ORF1a), R346T (S), T478K (S), F486V (S), F486P (S)), three did not belong to any Nextstrain Clade (E3965E (ORF1a), I4205I (ORF1a), and F8F (ORF3a)), and two were associated with the 21J (Delta) clade (T3255I (ORF1a) and T478K (S)). On the other hand, Dataset_2 exclusively contained seven MOIs, with none of them overlapping with Dataset_1. Among these, three were defining mutations of the 21K (Omicron) clade (T95I (S), V143- (S), and G496S (S)), while four lacked Nextstrain Clade assignments (L829I (ORF1b), Y1759Y (ORF1b), Y145H (S), and Q9L (N)).
3.2 Associations with vaccine failure
3.2.1 Dataset_1
When applying a Chi-square test, individuals who were fully vaccinated and infected with non-Omicron variants showed a statistically significant association with vaccine coverage. This trend was observed from Set1_ds1 to Set4_ds1. Thus, variants that peaked in December, January, March, and April and 2021 were strongly linked to vaccine protection (Table 2). However, Omicron cases in sets of sequences, Set5_ds1 (21L, FDR = 1.58 × 10−41), Set6_ds1 and Set7_ds1 (22B and 22E, FDR = 1.32 × 10−122 and 1.12 × 10−50, respectively), as well as Set8_ds1 (22F and 23A, FDR = 1.24 × 10−88), displayed a strong association with COVID-19 infection (Table 3; Supplementary Table S1). The booster dose demonstrated a slight improvement in protection against viral infection, with the exception of Set7_ds1. When comparing Set7_ds1 to Set6_ds2 from the same clade, we observed that both shared the same non-defining MOIs A27- (S), I68- (S), R346T (S) and G30- (N), with the only difference being the presence of a ‘T’ at locus 346 in the spike protein (Table 6).
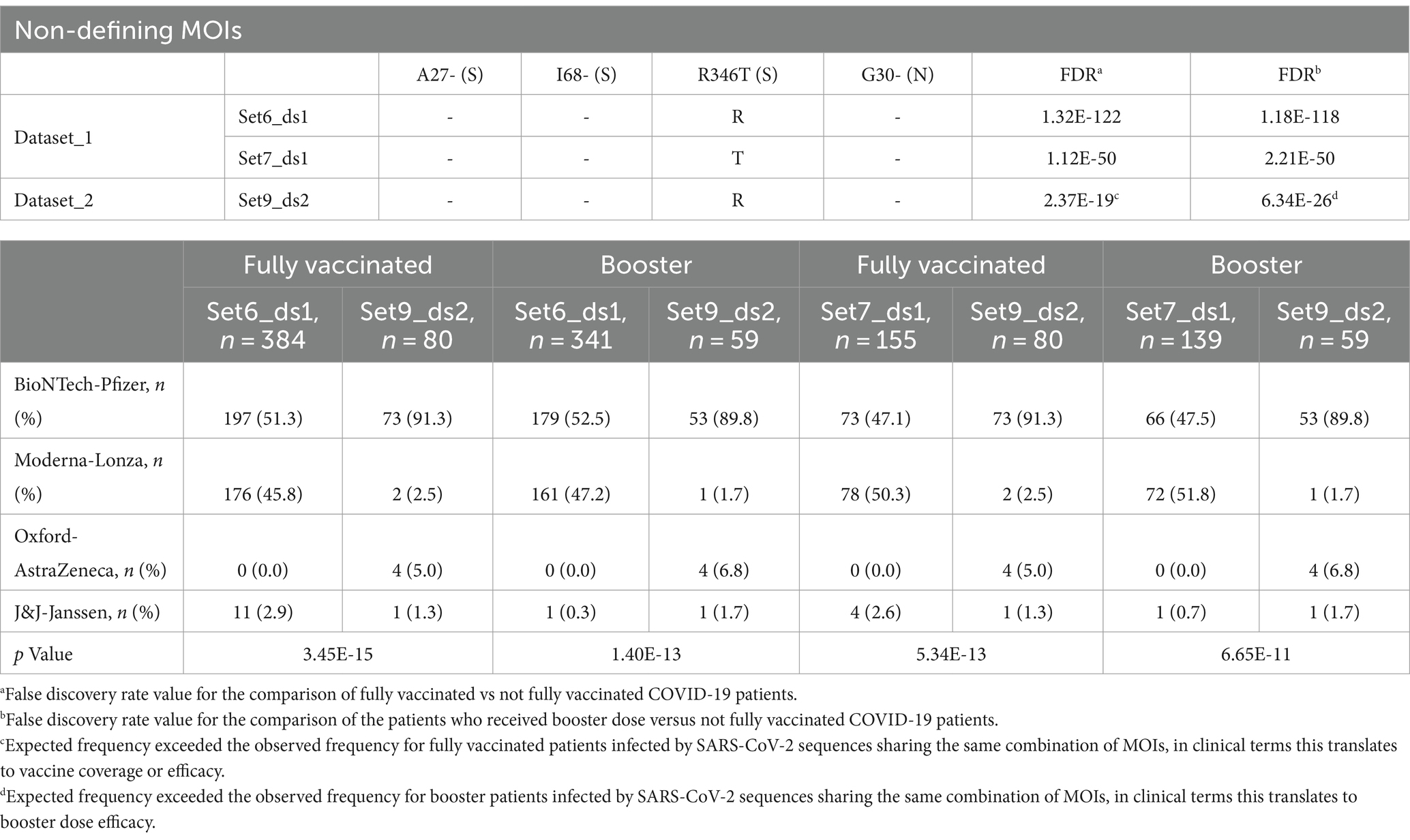
Table 6. Set of sequences sharing the same combination of MOIs in Dataset_1 and Dataset_2 for 22B (Omicron) and 22E (Omicron) variants and their distribution based on vaccine brands.
3.2.2 Dataset_2
In the 21J (Delta) clade, five distinct sequence sets (Set2_ds2, Set3_ds2, Set4_ds2, Set5_ds2, and Set6_ds2) were identified, all sharing the same residue substitutions in three non-defining mutations: P2046L (ORF1a), P2287S (ORF1a), and R158- (S) (Table 7). However, the distinguishing factor among these sets was the presence of four additional non-defining mutations, specifically L829I (ORF1b), T95I (S), Y145H (S), and Q9L (N) (Table 7). Two of these sets, Set2_ds2 (FDR = 5.08 × 10−13) and Set5_ds2 (FDR = 0.034), showed significant associations with low vaccine coverage, although they differed in the defining mutation G142D (S) (Supplementary Figure S2B). Both high-risk sets shared non-defining haplotypes, having a threonine at position 829 in the ORF1a and a leucine at position 9 in the N protein (Supplementary Figure S2B; Table 7). On the other hand, Set1_ds2 (clade 20I, Alpha variant) and two sets of sequences infected by the Omicron variant, namely Set7_ds2 (21K) and Set9_ds2 (22B), exhibited a strong association with reduced susceptibility to SARS-CoV-2 infection.
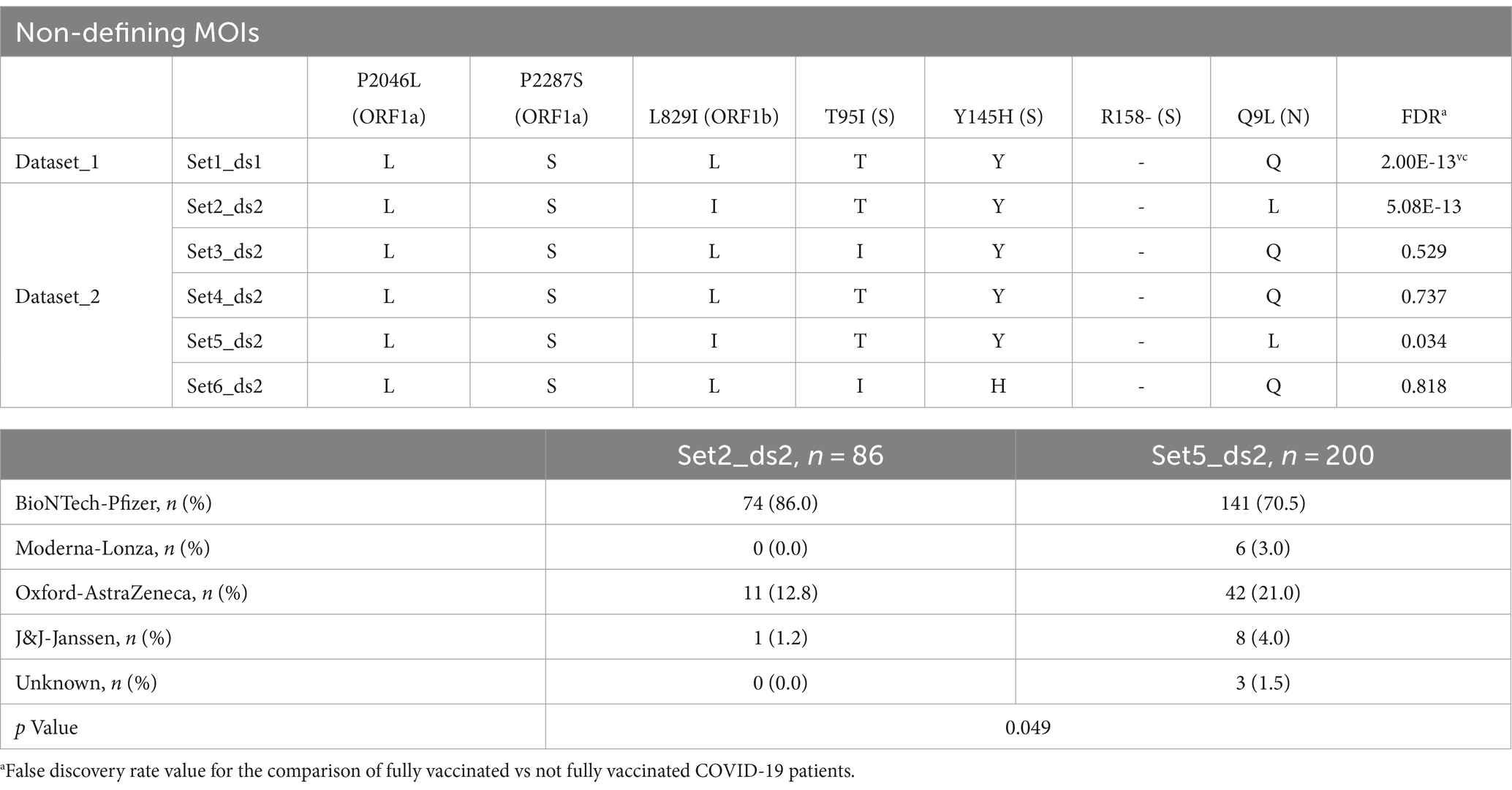
Table 7. Set of sequences sharing the same combination of MOIs in Dataset_1 and Dataset_2 for 21J (Delta) variant and their distribution based on vaccine brands.
Additionally, it was observed that the booster dose was found to provide increased protection against the Omicron 21K and 22B clades (Table 5; Supplementary Table S1).
3.3 Conservation analysis
3.3.1 Dataset_1
The conservation scores, used to assess the overall functionality based on the physicochemical properties of the amino acid substitutions, revealed a strong conservation index in 38.7% of the changes. However, 37.0% of these substitutions exhibited low conservation similarities (Table 8; Supplementary Figure S2A; Supplementary Table S2). These substitutions were predominantly composed of hydrophobic (66.2%) and polar (55.4%) amino acids (Table 8). For a more in-depth analysis of these changes, we grouped the set of sequences associated with vaccine failure caused by the Omicron variant considering clades 21L, 22B/22E and 22F/23A, as this is the only variant that was associated with vaccine failure. The analysis identified 52 loci that differed between Set5_ds1, Set6_ds1, Set7_ds1, and Set8_ds1 resulting in 55 amino acids changes (Supplementary Table S2) possessing mainly hydrophobic (63.6%) and polar (61.8%) properties (Table 8). Of these substitutions, 40.4% showed an intermediate conservation index. Focusing on non-clade-defining MOIs, we identified 11 loci that differed between Set5_ds1, Set6_ds1, Set7_ds1, and Set8_ds1, including L3201F (ORF1a), G662S (ORF1b), V213G/E (S), G339D/H (S), R346T (S), G446S (S), L452R (S), F486V/P (S), Q493R (S), D3N (M) and D61L (ORF6). These differences resulted in 14 amino acid changes, primarily characterized by polar (64.3%) and small (57.7%) properties (Table 8).
3.3.2 Dataset_2
The conservation index, assessing properties that are positively or negatively conserved, displayed high values in 39.5% of the MOIs, while 37.0% showed low conservation values (Table 8; Supplementary Figure S2B; Supplementary Table S3). The predominant physicochemical properties affected by these changes were hydrophobic (69.1%) and polar (53.1%) (Table 8). Only the 21J variant was found to be associated with vaccine escape so it was analyzed in detail. This analysis identified 26 loci that differed between set of sequences ranging from Set2_ds2 to Set6_ds2 resulting in 26 amino acid changes characterized predominantly by their hydrophobic (69.2%) property (Table 8). Substitutions with a conservation index corresponding to high and intermediate conservation were dominant (34.6 and 38.5%, respectively). Non-defining mutations of 21J (Delta) included P2046L, P2287S, L829I, T95I, Y145H, R158-, and Q9L which were characterized by hydrophobic (83.3%) and aliphatic (66.7%) amino acid substitutions. These changes were mainly led to an intermediate conservation of physicochemical properties.
3.4 Vaccine effectiveness
Given the significant role of non-clade-defining mutations in the risk of vaccine failure, the next step was to conduct a joint analysis of both datasets. This analysis aimed to identify non-clade defining MOIs associated with vaccine failure caused by the Omicron (21L, 22B and 22E) and Delta (21J) clades, which are common variants present in both datasets but were not initially linked to vaccine failure in the same manner.
Both set5_ds1 and set8_ds2 belonged to the 21L clade and shared three additional mutations: L3201F (ORF1a), A27- (S), and G30- (S) (Table 9). However, while set5 was associated with vaccine failure even after receiving a booster dose (FDR = 1.58 × 10−41 and 3.20 × 10−31, respectively), set8_ds2 did not exhibit the same level of association. The Chi-Square test revealed statistically significant differences in vaccine distribution between fully vaccinated individuals (FDR = 3.58 × 10−17) and those who received a booster dose (FDR = 1.71 × 10−20) when comparing set5_ds1 and set8_ds2, respectively. Set5_ds1 was primarily associated with the Pfizer and Moderna vaccines (61.1 and 35.4% for fully vaccinated, and 59.3 and 39.0% for booster doses, respectively). In contrast, set8_ds2 was predominantly associated with Pfizer and AstraZeneca vaccines (77.9 and 13.6% for fully vaccinated, and 78.9 and 14.5% for booster doses, respectively). Furthermore, the analysis of the genetic distance in the sets of sequences showed remarkable differences between sets, with the genetic distance of set8_ds2 being 3.8 times higher compared to set5_ds (Figure 3).
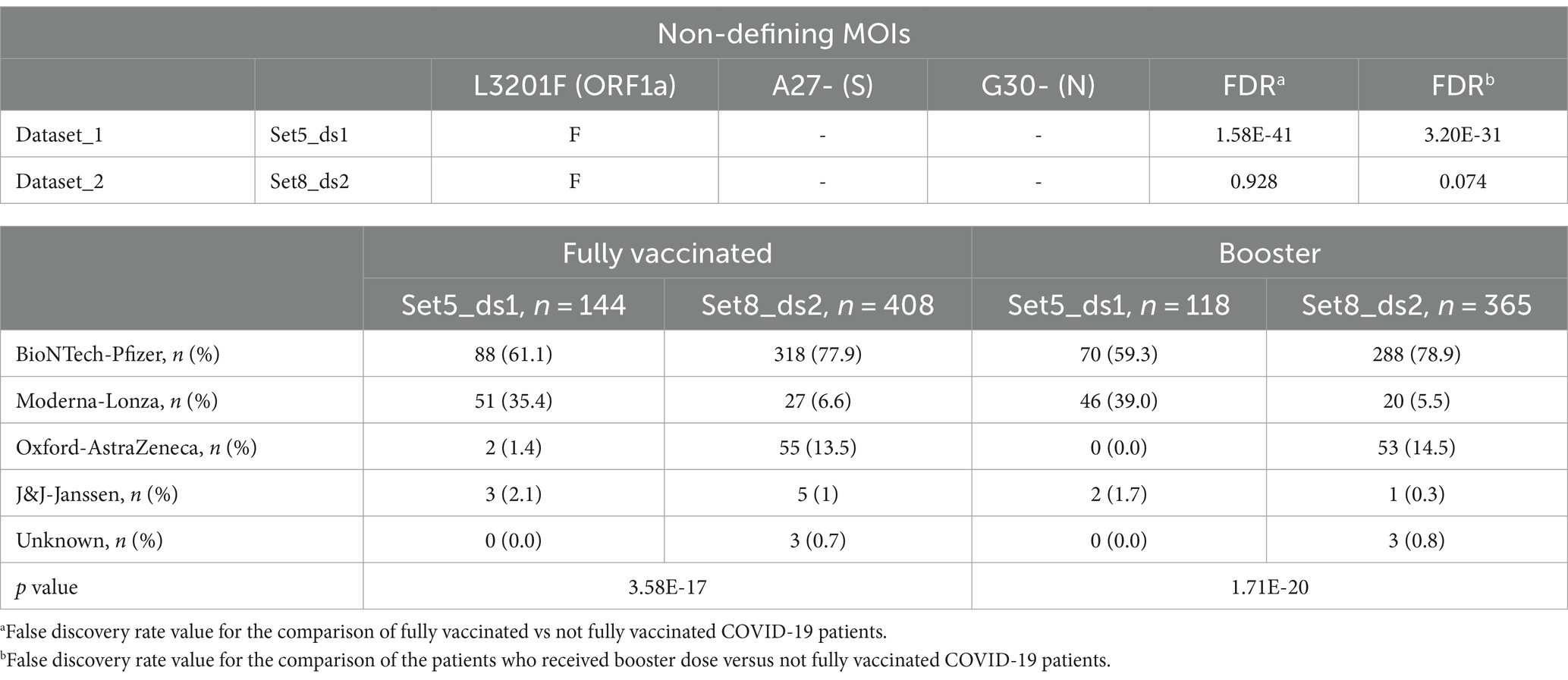
Table 9. Set of sequences sharing the same combination of MOIs in Dataset_1 and Dataset_2 for 21L (Omicron) variant and their distribution based on vaccine brands.
For the sets of sequences isolated from COVID-19 patients infected with clades 22B and 22E of the Omicron variant (Table 6), set6_ds1 and set7_ds1 (Dataset_1) and set9_ds2 (Datataset_2) shared the same non-clade defining mutations in the spike (A27-, I68- and R346T) and nucleocapsid (G30-). However, from Dataset_1 only two sequence sets corresponding to fully vaccinated patients (set6_ds1 FDR = 1.32 × 10−122; set7_ds1 FDR = 1.12 × 10−50) and patients who received the third dose (set6_ds1 FDR = 1.18 × 10−118; set7_ds1 FDR = 2.21 × 10−50) were associated with vaccine failure. Fully vaccinated patients from Dataset_1 primarily received Pfizer (51.3% for set6_ds1 and 47.1% for set7_ds1) and Moderna (45.8% for set6_ds1 and 50.3% for set7_ds1) vaccines, in contrast to Dataset_2, where 91.3% of patients received Pfizer. Statistically significant differences were found when comparing the FV distribution of these sets (FDR = 3.45 × 10−15 for set6_ds1 vs. set9_ds2 and 5.34 × 10−13 for set7_ds1 vs. set9_ds2, respectively). For patients who received a booster dose, the main vaccine brands and their associations with vaccine failure remained consistent (FDR = 1.40 × 10−13 (set6_ds1 vs. set9_ds2); FDR = 6.65 × 10–11 (set7_ds1 vs. set9_ds2)). Set6_ds1 and set7_ds1 displayed comparable genetic distances among sequences. However, set9_ds2 exhibited a higher genetic distance (Figure 4).
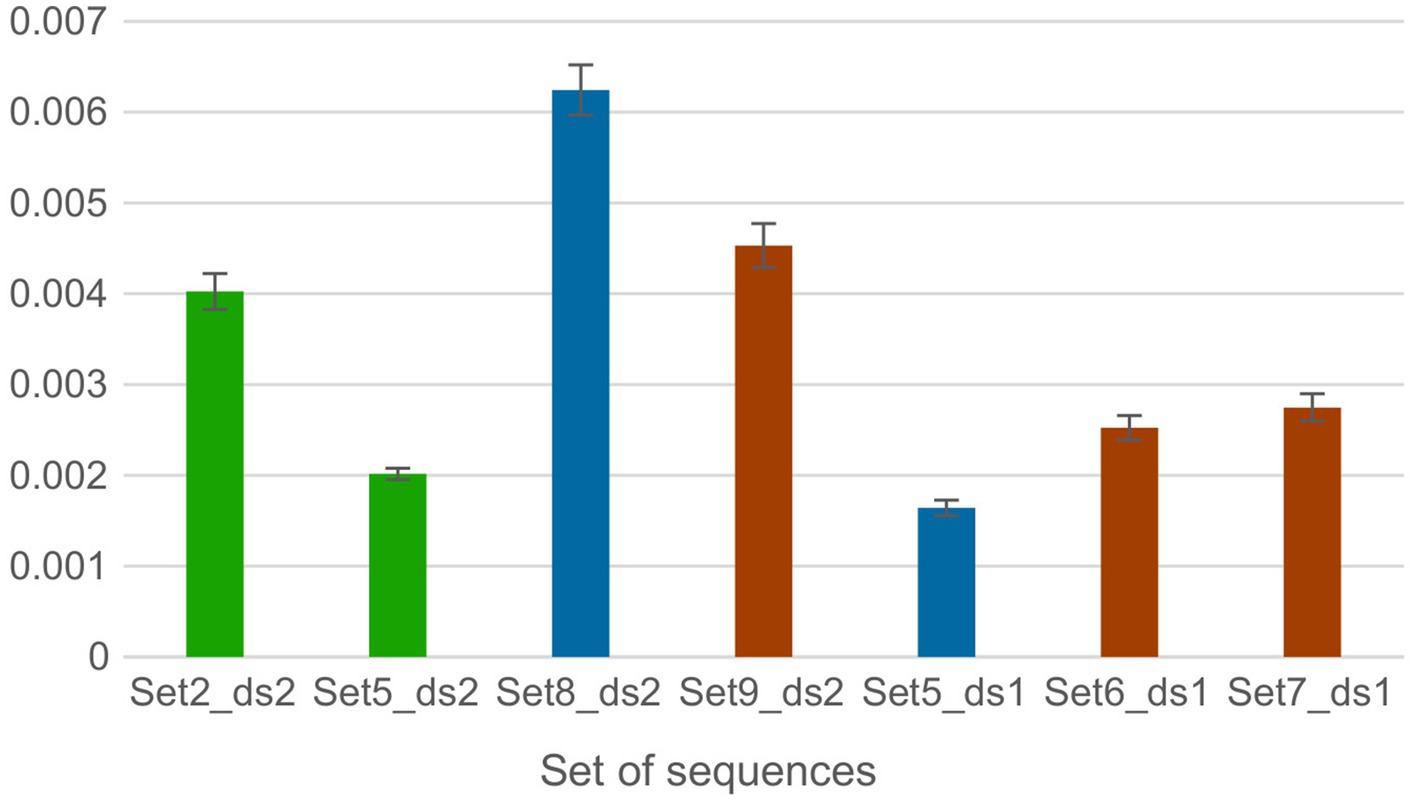
Figure 4. Genetic distances between amino acids per site within each sequence set of Dataset 1 and 2 obtained by averaging all sequence pairs, along with the standard error estimates The average distance was calculated using the Bootstrap method for variance estimation, with 1,000 bootstrap replicates. The p-distance model was used for the amino acid substitution type. Ambiguous positions were removed for each pair of sequences using the pairwise deletion option. The bars are organized based on the datasets and are color-coded according to sets of sequences that share the same MOIs.
In both datasets, the set of sequences identified as 21J (Delta) shared three additional MOIs, specifically P2046L (ORF1a), P2287S (ORF1a) and R158- (S) (Table 7). Dataset_2 exclusively featured four mutations including L829I (ORF1b), T95I (S), Y145H (S) and Q9L (N). In contrast, these mutations did not meet the MOI criteria for Dataset_2, bearing the wild type amino acids.
Only Set2_ds2 and Set5_ds2 from Dataset_2 were associated with vaccine failure. The vaccine distribution between these risk sets exhibited marginal significance (FDR = 0.049), with the major vaccine brands being both Pfizer and AstraZeneca. The genetic distances for set2_ds2 were twice as high as those observed in set5_ds2 (Figure 4).
There was no correlation between the variability of amino acids in the sets of sequences, measured by the genetic distance, and the loss of vaccine effectiveness for the compared clades.
4 Discussion
Mutations and deletions in SARS-CoV-2 proteins can significantly alter their structure and function. This study investigated the impact of mutations of interest (MOI) across the entire SARS-CoV-2 proteome on vaccine escape using two datasets. The analysis revealed several mutations and combinations of residues that may influence vaccine coverage, particularly concerning the Delta (clade 21J) and Omicron BA.2 (clade 21L) variants.
For the Delta variant, mutations at P2046L (ORF1a), P2287S (ORF1a), L829I (ORF1b), R158- (S) and Q9L (N) were identified as potentially crucial for vaccine effectiveness. Similarly, mutations such as L3201F (ORF1a), A27- (S) and G30- (N) in the Omicron variant were found to impact vaccine effectiveness. However, deletions A27- (S), I68- (S) and G30- (N), along with the R346T mutation in the spike protein of the Omicron variant, may further compromise vaccine effectiveness.
Observations of mutations in the spike protein, particularly A27-, I68-, and R158- located within the N-terminal domain (NTD), and the R346T mutation within the Receptor Binding Domain (RBD), align with previous studies. Molecular dynamics simulations have shown the critical involvement of NTD residues in interactions with monoclonal antibodies (42), suggesting potential immune evasion risks for viruses carrying mutations in these regions. Furthermore, mutations within the RBD have been shown to affect the binding affinity to the ACE2 receptor, indicating potential shifts in the binding free energy of the RBD-ACE2 complex and modified chemical interactions, leading to increased stability (43).
Limited published data on vaccine efficacy or immunity related to the A27-(S) deletion were found. However, the spike 68–76 deletion within the NTD was identified in a human hepatoma cell clone termed Huh7.5-adapted-SARS2, indicating genetic adaptations. This modified version of SARS-CoV-2 effectively infiltrated A549 lung cancer cells, inducing cellular damage, a capability absent in the original strain, which exhibited no infectivity toward A549 cells. Additionally, the Spike 68–76 deletion variant displayed increased susceptibility to IFN-α2b treatment in comparison to the wild-type SARS-CoV-2 strain. However, the Spike 68–76 deletion was not found in SARS-CoV-2 isolates obtained from VERO E6 cells (44). In the context of vaccine stability and effectiveness, it suggests that despite the presence of the 68–76 deletion in vaccine batches (CoronaVac), it might not drastically alter the vaccine’s effectiveness. The R158- (S) mutation in combination with E156G/157 deletion and L452R mutation has been suggested to exhibit higher infectivity in spike-pseudotyped viruses (45). However, experimental evidence points to the 156–158 deletion notably diminishing the neutralization capacity against antibodies present in the sera of convalescent COVID-19 patients and vaccinated individuals (42, 46, 47).
The R346T change in the RBD is a key mutation for neutralization escape, enhanced fusogenicity, and enhanced S protein processing. Structural modeling suggests that R346T appears to disrupt salt bridge formation between the S protein and class III monoclonal antibodies (e.g., Cilgavimab), lowering effectiveness (48, 49). However, in our study the set of sequences containing R indicates poorer vaccine coverage than T for the Omicron clades 22B/22E.
The outcomes related to spike mutations impacting the infectivity of SARS-CoV-2 appear to exhibit a wide-ranging scope. Conversely, the available data regarding the influence of mutations occurring in other viral proteins seems comparatively constrained.
An analysis conducted on 244 SARS-CoV-2 positive samples, gathered during the second wave of the pandemic, indicated that mutations P2046L and P2287S in the nsp3 (ORF1a) gene might contribute to persistent symptomatic COVID-19 infections post-vaccination (50). Additionally, an investigation involving severe, moderate, and mild COVID-19 cases, encompassing individuals who were either partially or fully vaccinated (with Covishield/Covaxin) or unvaccinated, revealed a marginal association of the P2287S mutation with disease severity (51). The nsp3 protein in the SARS-CoV-2 virus constitutes a crucial component of the viral replicase complex, contributing significantly to multiple functions associated with viral replication (52), transcription (53), and modulation of the host immune response (54), but no specific data on the impact of the L3201F mutation on these functions have been found in the literature.
A study aimed at modeling the fitness of several SARS-CoV-2 lineages by combining the effect of individual mutations introduced a scalable hierarchical Bayesian regression model to analyze all available SARS-CoV-2 genomes. The study identified the L829I (ORF1b) mutation in nsp12, which promotes an amino acid change in the RdRp (RNA-dependent RNA polymerase) thumb subdomain that could affect the function of the enzyme. RdRp plays a critical role in replicating and transcribing the viral genome in RNA viruses like SARS-CoV-2 (55).
The N protein consists of different structural components, namely, an N-arm, an N-terminal RNA-binding domain, a linker region containing serine/arginine-rich loops (SR-rich region), a C-terminal RNA-binding domain, and a C-tail (56–61). Some regions of the N-arm (amino acids 1–46) have been identified as immunodominant epitopes. Specifically, five monoclonal antibodies were developed through a study of epitopes targeting the N protein of SARS-CoV-2. The research revealed that one particular antibody had a specific affinity for the N-arm region of the N protein (62), suggesting the possible involvement of mutations identified in this study such as Q9L and G30- in immune evasion.
As mentioned, some of the substitutions and deletions associated with vaccine failure in our study seem to be in line with previous studies. However, others have not been previously linked to vaccine coverage or immunity. Therefore, a genome-wide analysis of SARS-CoV-2 mutations, and their effects on the proteome, could help to understand the molecular basis of viral vaccine escape, in connection with vaccine and therapeutic drug development.
The effect of COVID-19 booster-dose vaccination against the Omicron variant has been reviewed by a study that identified a total of 27 published studies supporting the effectiveness of booster dose vaccine (63). Our results are consistent with the improved effectiveness of the booster dose against the Omicron variant in Dataset_2, where the vaccines show high coverage further improved by the administration of the third dose for clades 21L and 22B. However, in Dataset_1 where the vaccine is not effective for Omicron clades, administration of the third dose showed a slight protection for clades 21L, 22B/22E, and 22F/23A. We hypothesize that the discrepancies observed between these datasets might be attributed to the molecular composition of the administered vaccines.
Full implementation of SARS-CoV-2 vaccines is a major goal facing the COVID-19 pandemic. A comparative analysis of COVID-19 vaccine characteristics, adverse events, efficacy and effectiveness reported that all vaccines up to 22nd September 2021 appeared to be safe and effective tools against all variants of concern to prevent severe COVID-19, hospitalization, and death. However, the evidence varies greatly depending on the vaccines considered (64). In addition, the effectiveness of BNT162b2/Comirnaty vaccine (Pfizer) against the Omicron variant has been reported as 60% (65). Conversely, the effectiveness of the Spikevax/mRNA-1273 vaccine (Moderna) was published for symptomatic and asymptomatic cases, without specifying effectiveness for different clades of SARS-CoV-2 (66–68). In this line, in our study, the set of sequences associated with vaccine failure were mainly related to nucleic acid-based vaccines developed by BioNTech-Pfizer and Moderna-Lonza. However, Omicron cases where the vaccine exhibited a protective effect showed a high percentage of the Pfizer brand.
Investigation of the physicochemical attributes of amino acids enables an understanding of the intricate dynamics between viral proteins and the host immune system. This exploration provides valuable insights into viral pathogenicity, contributes to vaccine design and shapes strategies for drug development. In our study a significant number of substitutions, evaluated by conservation scores, showed a robust conservation index, indicating a strong correlation with amino acid physicochemical properties in approximately one-third of the changes. Furthermore, a comparable proportion of these substitutions exhibited lower conserved similarities in both datasets, implying an equal prevalence of such disparities among substitutions analyzed using conservation scores. Analysis of the physicochemical properties of amino acid changes revealed a predominant occurrence of hydrophobic and polar amino acid substitutions in both datasets. Substitutions in the Omicron variant (clades 21L, 22B/22E, and 22F/23A) were predominantly characterized by hydrophobic and polar properties. However, the non-defining mutations of each Omicron clade were mainly polar and small. The 21J (Delta) clade sequence set featured mainly amino acid substitutions with hydrophobic properties. The non-defining mutations of 21J (Delta) were distinguished by prevalent hydrophobic and aliphatic amino acid substitutions. This is agreed with the research that showed the significant role of hydrophobic residues in the spike protein, enhancing interactions in the Delta variant (69). Recently, it was highlighted that the defining mutations in the Delta and Omicron variants markedly impact hydrophobicity, polarity, and charge distribution in all regions of the N-protein (70).
The role of missense mutations and deletions in SARS-CoV-2 has been recognized as pivotal for vaccine effectiveness and residue interactions, highlighting the need to elucidate the molecular basis of these substitutions and deletions for advancing vaccine and drug development. Our investigation identified six mutations significantly associated with reduced vaccine coverage, such as P2046L (ORF1a), P2287S (ORF1a), L3201F (ORF1a), L829I (ORF1b), R346T (S), and Q9L (N), along with the four deletions A27- (S), I68- (S), R158- (S), and G30- (N). Analysis of whole proteome sequences of SARS-CoV-2 derived from COVID-19 patients revealed a correlation between non-clade-defining mutations and vaccine effectiveness. Currently approved vaccines primarily target the spike protein. Thus, changes in this protein could challenge vaccine effectiveness. Our findings support a proteome perspective in SARS-CoV-2 vaccine design, which could improve vaccine effectiveness. In addition, we found that amino acid substitutions exhibited predominantly hydrophobic and polar properties. Understanding the physicochemical properties of amino acid substitutions is crucial, as it reveals how these modifications affect protein structure, function, and interactions. This understanding provides valuable insights into disease mechanisms and the identification of potential therapeutic targets.
Data availability statement
The original contributions presented in the study are included in the article/Supplementary material, further inquiries can be directed to the corresponding author.
Author contributions
EU: Data curation, Formal analysis, Methodology, Writing – original draft, Writing – review & editing. AG-P: Formal analysis, Validation, Writing – original draft, Writing – review & editing, Funding acquisition. DH: Formal analysis, Methodology, Writing – original draft, Writing – review & editing. CeG: Writing – original draft, Writing – review & editing, Data curation. CaG: Funding acquisition, Supervision, Writing – original draft, Writing – review & editing. CS: Conceptualization, Funding acquisition, Writing – original draft, Writing – review & editing, Formal analysis. NC: Conceptualization, Funding acquisition, Supervision, Writing – original draft, Writing – review & editing.
Funding
The author(s) declare that financial support was received for the research, authorship, and/or publication of this article. This research work was supported by the European Commission–NextGenerationEU (Regulation EU 2020/2094), through CSIC’s Global Health Platform (PTI Salud Global). Project: PID2021-124662OB-I00 financed by MCIN/AEI/10.13039/501100011033/and by FEDER “A way to make Europe.” With support from the AXA-ICMAT Chair in Adversarial Risk Analysis. The research was also made possible through the Proyectos Estratégicos Orientados a la Transición Ecológica y a la Transición Digital under the code “TED2021-129970B-C21.” AG-P acknowledges funding by the Programa Operativo FEDER 2014–2020 and Consejería de Economía y Conocimiento, Junta de Andalucía (grant number CV20-10932).
Acknowledgments
We gratefully acknowledge all data contributors, i.e., the authors and their originating laboratories responsible for obtaining the specimens, and their submitting laboratories for generating the genetic sequence and metadata and sharing via the GISAID Initiative, on which this research is based. Thanks to David Ríos Insua, Roi Naveiro Flores, Simón Rodríguez Santana, and Daniel García Rasines for the academic support and to Miguel Álvarez-Herrera and Paula Ruiz-Rodríguez for their assistance in preparing the Spanish dataset.
Conflict of interest
The authors declare that the research was conducted in the absence of any commercial or financial relationships that could be construed as a potential conflict of interest.
The author(s) declared that they were an editorial board member of Frontiers, at the time of submission. This had no impact on the peer review process and the final decision.
Publisher’s note
All claims expressed in this article are solely those of the authors and do not necessarily represent those of their affiliated organizations, or those of the publisher, the editors and the reviewers. Any product that may be evaluated in this article, or claim that may be made by its manufacturer, is not guaranteed or endorsed by the publisher.
Supplementary material
The Supplementary material for this article can be found online at: https://www.frontiersin.org/articles/10.3389/fpubh.2024.1386596/full#supplementary-material
Footnotes
References
1. Gorbalenya, AE, Baker, SC, Baric, RS, de Groot, RJ, Drosten, C, Gulyaeva, AA, et al. The species severe acute respiratory syndrome-related coronavirus: classifying 2019-nCoV and naming it SARS-CoV-2. Nat Microbiol. (2020) 5:536–44. doi: 10.1038/s41564-020-0695-z
2. Islam, MA. A review of SARS-CoV-2 variants and vaccines: viral properties, mutations, vaccine efficacy, and safety. Inf Med. (2023) 2:247–61. doi: 10.1016/j.imj.2023.08.005
3. Islam, MA, Haque, MA, Rahman, MA, Hossen, F, Reza, M, Barua, A, et al. A review on measures to rejuvenate immune system: natural mode of protection against coronavirus infection. Front Immunol. (2022) 13:837290. doi: 10.3389/fimmu.2022.837290
4. Braeye, T, Catteau, L, Brondeel, R, van Loenhout, JAF, Proesmans, K, Cornelissen, L, et al. Vaccine effectiveness against transmission of alpha, delta and omicron SARS-COV-2-infection, Belgian contact tracing, 2021–2022. Vaccine. (2023) 41:3292–300. doi: 10.1016/j.vaccine.2023.03.069
5. Huiberts, AJ, de Gier, B, Hoeve, CE, de Melker, HE, Hahné, SJM, den Hartog, G, et al. Vaccine effectiveness of primary and booster COVID-19 vaccinations against SARS-CoV-2 infection in the Netherlands from July 12, 2021 to June 6, 2022: A prospective cohort study. Int J Infect Dis. (2023) 133:36–42. doi: 10.1016/j.ijid.2023.04.401
6. Davidson, AD, Williamson, MK, Lewis, S, Shoemark, D, Carroll, MW, Heesom, KJ, et al. Characterisation of the transcriptome and proteome of SARS-CoV-2 reveals a cell passage induced in-frame deletion of the furin-like cleavage site from the spike glycoprotein. Genome Med. (2020) 12:68. doi: 10.1186/s13073-020-00763-0
7. Tushir, S, Kamanna, S, Nath, SS, Bhat, A, Rose, S, Aithal, AR, et al. Proteo-genomic analysis of SARS-CoV-2: A clinical landscape of single-nucleotide polymorphisms, COVID-19 proteome, and host responses. J Proteome Res. (2021) 20:1591–601. doi: 10.1021/acs.jproteome.0c00808
8. Ezaj, MMA, Junaid, M, Akter, Y, Nahrin, A, Siddika, A, Afrose, SS, et al. Whole proteome screening and identification of potential epitopes of SARS-CoV-2 for vaccine design-an immunoinformatic, molecular docking and molecular dynamics simulation accelerated robust strategy. J Biomol Struct Dyn. (2022) 40:6477–502. doi: 10.1080/07391102.2021.1886171
9. Karami Fath, M, Jahangiri, A, Ganji, M, Sefid, F, Payandeh, Z, Hashemi, ZS, et al. SARS-CoV-2 proteome harbors peptides which are able to trigger autoimmunity responses: implications for infection, vaccination, and population coverage. Front Immunol. (2021) 12:12. doi: 10.3389/fimmu.2021.705772
10. Islam, MA, Al, MA, Arman, MS, Shahi, S, Sakif, TI, Hossain, M, et al. Some common deleterious mutations are shared in SARS-CoV-2 genomes from deceased COVID-19 patients across continents. Sci Rep. (2023) 13:18644. doi: 10.1038/s41598-023-45517-1
11. Mishra, SK, and Tripathi, T. One year update on the COVID-19 pandemic: Where are we now? Acta Trop. (2021) 214:105778. doi: 10.1016/j.actatropica.2020.105778
12. Molavi, Z, Razi, S, Mirmotalebisohi, SA, Adibi, A, Sameni, M, Karami, F, et al. Identification of FDA approved drugs against SARS-CoV-2 RNA dependent RNA polymerase (RdRp) and 3-chymotrypsin-like protease (3CLpro), drug repurposing approach. Biomed Pharmacother. (2021) 138:111544. doi: 10.1016/j.biopha.2021.111544
13. Osipiuk, J, Azizi, SA, Dvorkin, S, Endres, M, Jedrzejczak, R, Jones, KA, et al. Structure of papain-like protease from SARS-CoV-2 and its complexes with non-covalent inhibitors. Nat Commun. (2021) 12:743. doi: 10.1038/s41467-021-21060-3
14. Shang, J, Wan, Y, Luo, C, Ye, G, Geng, Q, Auerbach, A, et al. Cell entry mechanisms of SARS-CoV-2. Proc Natl Acad Sci U S A. 117:11727–34. doi: 10.1073/pnas.2003138117
15. Jackson, CB, Farzan, M, Chen, B, and Choe, H. Mechanisms of SARS-CoV-2 entry into cells. Nat Rev. (2022) 23:3–20. doi: 10.1038/s41580-021-00418-x
16. Zipeto, D, da Fonseca, PJ, Argañaraz, GA, and Argañaraz, ER. ACE2/ADAM17/TMPRSS2 interplay may be the Main risk factor for COVID-19. Front Immunol. (2020) 11:576745. doi: 10.3389/fimmu.2020.576745
17. Rahman, MS, Hoque, MN, Islam, MR, Islam, I, Mishu, ID, Rahaman, MM, et al. Mutational insights into the envelope protein of SARS-CoV-2. Gene Rep. (2021) 22:100997. doi: 10.1016/j.genrep.2020.100997
18. Bianchi, M, Benvenuto, D, Giovanetti, M, Angeletti, S, Ciccozzi, M, and Pascarella, S. Sars-CoV-2 envelope and membrane proteins: structural differences linked to virus characteristics? Biomed Res Int. (2020) 2020:1–6. doi: 10.1155/2020/4389089
19. Jörrißen, P, Schütz, P, Weiand, M, Vollenberg, R, Schrempf, IM, Ochs, K, et al. Antibody response to SARS-CoV-2 membrane protein in patients of the acute and convalescent phase of COVID-19. Front Immunol. (2021) 12:679841. doi: 10.3389/fimmu.2021.679841
20. Gao, T, Gao, Y, Liu, X, Nie, Z, Sun, H, Lin, K, et al. Identification and functional analysis of the SARS-COV-2 nucleocapsid protein. BMC Microbiol. (2021) 21:58. doi: 10.1186/s12866-021-02107-3
21. Matchett, WE, Joag, V, Stolley, JM, Shepherd, FK, Quarnstrom, CF, Mickelson, CK, et al. Cutting edge: Nucleocapsid vaccine elicits spike-independent SARS-CoV-2 protective immunity. J Immunol. (2021) 207:376–9. doi: 10.4049/jimmunol.2100421
22. Dangi, T, Class, J, Palacio, N, Richner, JM, and PP, MM. Combining spike- and nucleocapsid-based vaccines improves distal control of SARS-CoV-2. Cell Rep. (2021) 36:109664. doi: 10.1016/j.celrep.2021.109664
23. Le, BN, Tan, AT, Kunasegaran, K, Tham, CYL, Hafezi, M, Chia, A, et al. SARS-CoV-2-specific T cell immunity in cases of COVID-19 and SARS, and uninfected controls. Nature. (2020) 584:457–62. doi: 10.1038/s41586-020-2550-z
24. Yadav, R, Chaudhary, JK, Jain, N, Chaudhary, PK, Khanra, S, Dhamija, P, et al. Cells role of structural and non-structural proteins and therapeutic targets of SARS-CoV-2 for COVID-19. Cells. (2021) 10:821. doi: 10.3390/cells10040821
25. Comar, CE, Goldstein, SA, Li, Y, Yount, B, Baric, RS, and Weiss, SR. Antagonism of dsRNA-induced innate immune pathways by NS4a and NS4b accessory proteins during MERS coronavirus infection. MBio. (2019) 10:19. doi: 10.1128/mBio.00319-19
26. Thornbrough, JM, Jha, BK, Yount, B, Goldstein, SA, Li, Y, Elliott, R, et al. Middle east respiratory syndrome coronavirus NS4b protein inhibits host RNase L activation. MBio. (2016) 7:e00258. doi: 10.1128/mBio.00258-16
27. Nakagawa, K, Narayanan, K, Wada, M, and Makino, S. Inhibition of stress granule formation by Middle East respiratory syndrome coronavirus 4a accessory protein facilitates viral translation, leading to efficient virus replication. J Virol. (2018) 92:18. doi: 10.1128/JVI.00902-18
28. Zvelebil, MJ, Barton, GJ, Taylor, WR, and Sternberg, MJE. Prediction of protein secondary structure and active sites using the alignment of homologous sequences. J Mol Biol. (1987) 195:957–61. doi: 10.1016/0022-2836(87)90501-8
29. Livingstone, CD, and Barton, GJ. Protein sequence alignments: a strategy for the hierarchical analysis of residue conservation. CABIOS. (1993) 9:745–56. doi: 10.1093/bioinformatics/9.6.745
30. Elbe, S, and Buckland-Merrett, G. Data, disease and diplomacy: GISAID’s innovative contribution to global health. Global Chall. (2017) 1:33–46. doi: 10.1002/gch2.1018
31. Liang, Z, Xu, M, Teng, M, and Niu, L. NetAlign: A web-based tool for comparison of protein interaction networks. Bioinformatics. (2006) 22:2175–7. doi: 10.1093/bioinformatics/btl287
32. Ihaka, R, and Gentleman, R. R: A language for data analysis and graphics. J Comput Graph Stat. (1996) 5:299–314. doi: 10.1080/10618600.1996.10474713
34. Cock, PJA, Antao, T, Chang, JT, Chapman, BA, Cox, CJ, Dalke, A, et al. Biopython: freely available Python tools for computational molecular biology and bioinformatics. Bioinformatics. (2009) 25:1422–3. doi: 10.1093/bioinformatics/btp163
35. Virtanen, P, Gommers, R, Oliphant, TE, Haberland, M, Reddy, T, Cournapeau, D, et al. SciPy 1.0: fundamental algorithms for scientific computing in Python. Nat Methods. (2020) 17:261–72. doi: 10.1038/s41592-019-0686-2
36. Ou, J, and Zhu, LJ. trackViewer: A Bioconductor package for interactive and integrative visualization of multi-omics data. Nature Methods. (2019) 16:453–4. doi: 10.1038/s41592-019-0430-y
37. Rice, P, Longden, I, and Bleasby, A. EMBOSS: the European molecular biology open software suite. Trends Genet. (2000) 16:276–7. doi: 10.1016/S0168-9525(00)02024-2
38. Hunter, JD. Matplotlib: A 2D graphics environment. Comput Sci Eng. (2007) 9:90–5. doi: 10.1109/MCSE.2007.55
39. McKinney, W. (2010). Data structures for statistical computing in python. In: Proceedings of the 9th Python in Science Conference. p. 51–56.
40. Benjamini, Y. (1995). Controlling the false discovery rate-A practical and powerful approach to multiple testing. Article in Journal of the Royal Statistical Society Series B: Methodological. Available online at: https://www.researchgate.net/publication/221995234 (Accessed February 10, 2024).
41. Tamura, K, Stecher, G, and Kumar, S. MEGA11: molecular evolutionary genetics analysis version 11. Mol Biol Evol. (2021) 38:3022–7. doi: 10.1093/molbev/msab120
42. Zhou, D, Dejnirattisai, W, Supasa, P, Liu, C, Mentzer, AJ, Ginn, HM, et al. Evidence of escape of SARS-CoV-2 variant B.1.351 from natural and vaccine-induced sera. Cell. (2021) 184:2348–2361.e6. doi: 10.1016/j.cell.2021.02.037
43. Peka, M, and Balatsky, V. The impact of mutation sets in receptor-binding domain of SARS-CoV-2 variants on stability of the RBD-ACE2 complex. Future Virol. (2023) 18:225–42. doi: 10.2217/fvl-2022-0152
44. Ramirez, S, Fernandez-Antunez, C, Galli, A, Underwood, A, Pham, LV, Ryberg, LA, et al. Overcoming culture restriction for SARS-CoV-2 in human cells facilitates the screening of compounds inhibiting viral replication. Antimicrob Agents Chemother. (2021) 65:e0009721. doi: 10.1128/AAC.00097-21
45. Mishra, T, Dalavi, R, Joshi, G, Kumar, A, Pandey, P, Shukla, S, et al. SARS-CoV-2 spike E156G/Δ157-158 mutations contribute to increased infectivity and immune escape. Life Sci Alliance. (2022) 5:e202201415. doi: 10.26508/lsa.202201415
46. Planas, D, Veyer, D, Baidaliuk, A, Staropoli, I, Guivel-Benhassine, F, Rajah, MM, et al. Reduced sensitivity of SARS-CoV-2 variant Delta to antibody neutralization. Nature. (2021) 596:276–80. doi: 10.1038/s41586-021-03777-9
47. Edara, VV, Lai, L, Sahoo, MK, Floyd, K, Sibai, M, Solis, D, et al. Infection and vaccine-induced neutralizing antibody responses to the SARS-CoV-2 B.1.617.1 variant. bioRxiv. (2021). doi: 10.1101/2021.05.09.443299
48. Qu, P, Evans, JP, Faraone, JN, Zheng, YM, Carlin, C, Anghelina, M, et al. Enhanced neutralization resistance of SARS-CoV-2 omicron subvariants BQ.1, BQ.1.1, BA.4.6, BF.7, and BA.2.75.2. Cell Host Microbe. (2023) 31:9–17.e3. doi: 10.1016/j.chom.2022.11.012
49. Wang, Q, Li, Z, Ho, J, Guo, Y, Yeh, AY, Mohri, H, et al. Resistance of SARS-CoV-2 omicron subvariant BA.4.6 to antibody neutralisation. Lancet Infect Dis. (2022) 22:1666–8. doi: 10.1016/S1473-3099(22)00694-6
50. Banerjee, A, Mazumder, A, Roy, J, Majumdar, A, Chatterjee, A, Biswas, N, et al. Evolution of Delta variant by non-spike signature co-appearing mutations: trailblazer of COVID-19 disease outcome. bioRxiv. (2022). doi: 10.1101/2022.04.05.487103
51. Nilgiriwala, K, Kadam, P, Patel, G, Shaikh, A, Mestry, T, Vaswani, S, et al. Genomics of postvaccination SARS-CoV-2 infections during the Delta dominated second wave of COVID-19 pandemic, from Mumbai metropolitan region (MMR), India. J Med Virol. (2022) 94:4206–15. doi: 10.1002/jmv.27861
52. Jiang, Y, Tong, K, Yao, R, Zhou, Y, Lin, H, Du, L, et al. Genome-wide analysis of protein–protein interactions and involvement of viral proteins in SARS-CoV-2 replication. Cell Biosci. (2021) 11:140. doi: 10.1186/s13578-021-00644-y
53. Lei, J, Kusov, Y, and Hilgenfeld, R. Nsp3 of coronaviruses: Structures and functions of a large multi-domain protein. Antiviral Res. (2018) 149:58–74. doi: 10.1016/j.antiviral.2017.11.001
54. Low, ZY, Zabidi, NZ, Yip, AJW, Puniyamurti, A, Chow, VTK, and Lal, SK. SARS-CoV-2 non-structural proteins and their roles in host immune evasion. Viruses. (2022) 14:1991. doi: 10.3390/v14091991
55. Obermeyer, F, Jankowiak, M, Barkas, N, Schaffner, SF, Pyle, JD, Yurkovetskiy, L, et al. Analysis of 6.4 million SARS-CoV-2 genomes identifies mutations associated with fitness. Science (1979). (2022) 376:1327–32. doi: 10.1126/science.abm1208
56. Schiavina, M, Pontoriero, L, Uversky, VN, Felli, IC, and Pierattelli, R. The highly flexible disordered regions of the SARS-CoV-2 nucleocapsid N protein within the 1–248 residue construct: sequence-specific resonance assignments through NMR. Biomol NMR Assign. (2021) 15:219–27. doi: 10.1007/s12104-021-10009-8
57. Chang, CK, Hou, MH, Chang, CF, Hsiao, CD, and Huang, TH. The SARS coronavirus nucleocapsid protein - forms and functions. Antivir Res. (2014) 103:39–50. doi: 10.1016/j.antiviral.2013.12.009
58. Peng, Y, Du, N, Lei, Y, Dorje, S, Qi, J, Luo, T, et al. Structures of the SARS -CoV-2 nucleocapsid and their perspectives for drug design. EMBO J. (2020) 39:e105938. doi: 10.15252/embj.2020105938
59. Baggen, J, Vanstreels, E, Jansen, S, and Daelemans, D. Cellular host factors for SARS-CoV-2 infection. Nat Microbiol Nat Res. (2021) 6:1219–32. doi: 10.1038/s41564-021-00958-0
60. V’kovski, P, Kratzel, A, Steiner, S, Stalder, H, and Thiel, V. Coronavirus biology and replication: implications for SARS-CoV-2. Nat Rev Microbiol. (2021) 19:155–70. doi: 10.1038/s41579-020-00468-6
61. Peng, TY, Lee, KR, and Tarn, WY. Phosphorylation of the arginine/serine dipeptide-rich motif of the severe acute respiratory syndrome coronavirus nucleocapsid protein modulates its multimerization, translation inhibitory activity and cellular localization. FEBS J. (2008) 275:4152–63. doi: 10.1111/j.1742-4658.2008.06564.x
62. Yang, J, Phan, VM, Heo, CK, Van, NH, Lim, WH, Cho, EW, et al. Development of nucleocapsid-specific monoclonal antibodies for SARS-CoV-2 and their ELISA diagnostics on an automatic microfluidic device. Sens Actuators B Chem. (2023) 380:133331. doi: 10.1016/j.snb.2023.133331
63. Chenchula, S, Karunakaran, P, Sharma, S, and Chavan, M. Current evidence on efficacy of COVID‐19 booster dose vaccination against the omicron variant: A systematic review. J Med Virol. (2022) 94:2969–76. doi: 10.1002/jmv.27697
64. Fiolet, T, Kherabi, Y, MacDonald, CJ, Ghosn, J, and Peiffer-Smadja, N. Comparing COVID-19 vaccines for their characteristics, efficacy and effectiveness against SARS-CoV-2 and variants of concern: A narrative review. Clin Microbiol Infect. (2022) 28:202–21. doi: 10.1016/j.cmi.2021.10.005
65. Tartof, SY, Frankland, TB, Puzniak, L, Slezak, JM, Hong, V, Takhar, H, et al. BNT162b2 against COVID-19-associated emergency department and urgent care visits among children 5-11 years of age: A test negative design. J Pediatric Infect Dis Soc. (2023) 12:177–9. doi: 10.1093/jpids/piad005
66. El Sahly, HM, Baden, LR, Essink, B, Doblecki-Lewis, S, Martin, JM, Anderson, EJ, et al. Efficacy of the mRNA-1273 SARS-CoV-2 vaccine at completion of blinded phase. N Engl J Med. (2021) 385:1774–85. doi: 10.1056/NEJMoa2113017
67. Verbeke, R, Lentacker, I, De Smedt, SC, and Dewitte, H. The dawn of mRNA vaccines: the COVID-19 case. J Control Release. (2021) 333:511–20. doi: 10.1016/j.jconrel.2021.03.043
68. Rana, R, Kant, R, Kumra, T, Gupta, S, Rana, DS, and Ganguly, NK. An update on SARS-CoV-2 immunization and future directions. Front Pharmacol. (2023) 14:1125305. doi: 10.3389/fphar.2023.1125305
69. Fatihi, S, Rathore, S, Pathak, AK, Gahlot, D, Mukerji, M, Jatana, N, et al. A rigorous framework for detecting SARS-CoV-2 spike protein mutational ensemble from genomic and structural features. Curr Res Struct Biol. (2021) 3:290–300. doi: 10.1016/j.crstbi.2021.11.002
Keywords: SARS-CoV-2, mutations, proteome, vaccine, conservation
Citation: Ulzurrun E, Grande-Pérez A, del Hoyo D, Guevara C, Gil C, Sorzano CO and Campillo NE (2024) Unlocking the puzzle: non-defining mutations in SARS-CoV-2 proteome may affect vaccine effectiveness. Front. Public Health. 12:1386596. doi: 10.3389/fpubh.2024.1386596
Edited by:
Dharmendra Kumar Yadav, Gachon University, Republic of KoreaReviewed by:
Amir Elalouf, Bar-Ilan University, IsraelMd. Aminul Islam, President Abdul Hamid Medical College, Bangladesh
Copyright © 2024 Ulzurrun, Grande-Pérez, del Hoyo, Guevara, Gil, Sorzano and Campillo. This is an open-access article distributed under the terms of the Creative Commons Attribution License (CC BY). The use, distribution or reproduction in other forums is permitted, provided the original author(s) and the copyright owner(s) are credited and that the original publication in this journal is cited, in accordance with accepted academic practice. No use, distribution or reproduction is permitted which does not comply with these terms.
*Correspondence: Nuria E. Campillo, bnVyaWEuY2FtcGlsbG9AY3NpYy5lcw==