- 1Department of Medical Statistics, School of Public Health & Center for Health Information Research & Sun Yat-sen Global Health Institute, Sun Yat-sen University, Guangzhou, China
- 2Peking University Center for Public Health and Epidemic Preparedness and Response, Peking, China
Background: Although the association between short-term air pollution exposure and certain hospitalizations has been well documented, evidence on the effect of longer-term (e. g., monthly) air pollution on a comprehensive set of outcomes is still limited.
Method: A total of 68,416 people in South China were enrolled and followed up during 2019–2020. Monthly air pollution level was estimated using a validated ordinary Kriging method and assigned to individuals. Time-dependent Cox models were developed to estimate the relationship between monthly PM10 and O3 exposures and the all-cause and cause-specific hospitalizations after adjusting for confounders. The interaction between air pollution and individual factors was also investigated.
Results: Overall, each 10 μg/m3 increase in PM10 concentration was associated with a 3.1% (95%CI: 1.3%−4.9%) increment in the risk of all-cause hospitalization. The estimate was even greater following O3 exposure (6.8%, 5.5%−8.2%). Furthermore, each 10 μg/m3 increase in PM10 was associated with a 2.3%-9.1% elevation in all the cause-specific hospitalizations except for those related to respiratory and digestive diseases. The same increment in O3 was relevant to a 4.7%−22.8% elevation in the risk except for respiratory diseases. Additionally, the older individuals tended to be more vulnerable to PM10 exposure (Pinteraction: 0.002), while the alcohol abused and those with an abnormal BMI were more vulnerable to the impact of O3 (Pinteraction: 0.052 and 0.011). However, the heavy smokers were less vulnerable to O3 exposure (Pinteraction: 0.032).
Conclusion: We provide comprehensive evidence on the hospitalization hazard of monthly PM10 and O3 exposure and their interaction with individual factors.
1. Introduction
The association between air pollution and human health has been well documented. For example, a meta-analysis on top of 21 studies worldwide suggested a 1.0% and 0.4% increase in hospitalization or emergency room visit for pneumonia for every 10 μg/m3 increment in the short-term exposure to PM2.5 and PM10 (1). Another meta-analysis also reported that each 10 μg/m3 increase in the 6-day cumulative PM2.5 concentration was associated with a 2.5% rise in chronic obstructive pulmonary disease hospitalization (2). Similar adverse health impact of other pollutants such as NO2, O3, and NO were also reported previously (3).
As suggested by previous studies, people of certain characteristics tended to be more vulnerable to the health impact of air pollution. For example, Michelle et al. pooled the results of 108 studies and concluded that the older individuals suffered from a greater mortality risk than the younger ones following the same exposure to particulate matters, with a 0.64% vs. 0.34% increase in mortality per 10 μg/m3 increment in PM10 concentration (4). They also observed that women had a slightly higher mortality risk of 0.55% compared with 0.50% among men (4). Compared with basic demographic variables such as age and sex, lifestyle information (e.g., smoking, drinking and physical activities), existing health status (e.g., overweight) and preexisting health conditions may be of a greater public health significance as they usually are more feasible to be modified to improve human wellbeing. The modification effect of these individual factors on the health impact of air pollution was biologically plausible (5–12). For example, smoking, alcohol consumption and air pollution were considered to increase the oxidative stress and dysfunction within cell mitochondria (5, 6). It is likely that smoking and drinking may strengthen the adverse health impact of air pollution in a synergistic way.
Although a lot of efforts have been taken to investigate the potential individual modifiers underlying the health impact of air pollution, existing evidence was challenged by several issues. First, most of existing works focused on the acute health effect of air pollution which usually occurred within one week following exposure, however, the evidence for exposures over a longer-term may be of a greater significance in policy making. Moreover, current studies only involved limited air pollutants or outcomes, systematic evidence for multiple pollutants and a comprehensive set of health outcomes is still limited. Additionally, the modifiers on the health effect of air pollution in existing studies generally were basic demographics (e.g., gender, age) or common lifestyle variables (e.g., smoking, drinking), evidence on a more comprehensive set of individual variables would be needed.
To address these research gaps, we investigated the effect of longer-term (i.e., monthly) exposure to main air pollutants on all-cause and cause-specific hospitalizations. Stratified effects and interactions were further investigated to identify potential individual effect modifiers. Our work provides a comprehensive insight into the health effect of air pollution and its modification by unhealthy lifestyles, demographics and preexisting health conditions, etc.
2. Methods
2.1. Study design and participants
We did a retrospective cohort study in Guangzhou area from 1/1/2019 through 12/31/2020 based on the primary medical and health service facilities. A multi-stage sampling was used to recruit participants from Community Healthcare Center-based population. In the first stage, 39 Community Healthcare Centers (183 in total) were randomly selected in Guangzhou. In the second stage, the residents who registered at these centers and met our inclusion criteria were invited to participate in our study voluntarily. The participants were followed up from the day entering the cohort to the first hospital admission, death or end of the study period, whichever came first. Participants followed up for less than 28 days were excluded to ensure sufficient time for exposure. Hospitalization records were retrieved from Guangzhou Health Commission. We obtained basic demographics (i.e., gender, age, ethnicity, education, retirement status, and marital status), lifestyle variables (smoking, alcohol intake, and activity level), and preexisting health conditions (BMI and disease history) through a questionnaire survey upon enrollment.
Individuals who were suffering from malignant tumor (591, 0.77%) or followed up for <28 days (9041, 11.7%) were excluded, leaving 68,416 participants in the final analysis. All-cause hospitalization was selected as the primary outcome. In addition, according to the number of patients, we also selected the top four subtypes as secondary outcomes including the hospitalizations due to (1) circulatory system disease; (2) respiratory system diseases; (3) digestive system disease; and (4) endocrine, nutritional and metabolic diseases with ICD-10 (International Statistical Classification of Diseases and Related Health Problems, 10th Revision) codes of I00-I99, J00-J99, K00-K93 and E00-E90, respectively. This study was approved by the School of Public Health, Sun Yat-sen University. All individuals consented to be included in the study.
2.2. Exposure assessment
Data on the concentration of four main air pollutants (i.e., PM10, PM2.5, NO2, and O3) were retrieved from the China National Environmental Monitoring Centre (https://air.cnemc.cn:18007). The monthly concentration of air pollution in Guangzhou during the study period was generated based on data collected from 98 monitoring stations in Guangdong through the ordinary Kriging interpolation method at a 0.01° × 0.01° resolution. The 10-fold cross-validation was performed to ensure the accuracy of the estimates, with the mean absolute percentage error (MAPE), root mean square error (RMSE) and determination coefficient (R-square) being computed and described in Supplementary Table S1, which showed similar accuracy with existing studies (13, 14).
As the residential address for each participant was not available while a community was relatively small, we used the coordinates (i.e., longitude and latitude) of the health service center in each community as a surrogate of the residential location. The coordinates were requested through the AutoNavi Map web service API (15, 16) (https://lbs.amap.com/). The monthly air pollution concentrations at each coordinate were assigned to the participants in the corresponding community and were further incorporated as a time-varying variable in the analysis.
2.3. Statistical analysis
We performed time-dependent Cox proportional hazard models to estimate the effect of long-term air pollution on both all-cause and specific-cause hospitalizations. Compared with the traditional Cox models, the time-dependent Cox models are more reliable against the proportional hazard assumption. Specifically, we established four models with an increased number of covariates being adjusted. Model 1 was a crude model which only included two air pollutants (i.e., PM10 and O3) without strong correlations (Pearson's rho < |0.8|, Supplementary Table S2). Other pollutants were excluded due to the collinearity issue. In model 2, we additionally adjusted for all basic demographics including age, gender, education, marital status, and retirement status. In model 3, we additionally included three lifestyle variables and BMI, and in model 4 we additionally adjusted for preexisting health conditions (i.e., hypertension, diabetes, and hyperlipidemia).
To evaluate whether effects of PM10 and O3 were modified by individual factors and to identify the most susceptible subgroups, we performed a comprehensive set of stratified analyses. Smoking status was measured by the smoking index (BI, BI = the number of cigarettes smoked per day × the duration of smoking in years), and was classified as never, light (BI < 400) and heavy (BI ≥ 400) according to previous studies (17–19). We defined the alcohol intake into never, under limit and over limit according to the Chinese Dietary Guidelines 2016 with limits of 25 g/day and 15 g/day for men and women, respectively (20). The activity level was categorized with the same method as literature, and comprised of 3 types (i.e., light, moderate, and heavy) (21). Participants were also grouped according to BMI (i.e., underweight: <18.5 kg/m2; normal: 18.5–23.9 kg/m2; overweight: 24–27.9 kg/m2; obese: >28 kg/m2). P for interaction was computed by including an interaction term of a certain pair of air pollution and individual factor. We also conducted a sensitive analysis by using PM2.5 instead of PM10. All hazard ratios (HR) were reported for each 10 μg/m3 increase in concentrations of air pollution. We used R software version 4.1.3 for our analyses.
3. Results
3.1. Descriptive statistics
A total of 68,416 participants were included in our study. The characteristics of population including basic demographics, lifestyle variables, preexisting health conditions are presented in Table 1. Over the study period, 5,278 hospital admissions were reported which accounted for 7.72% of the total population. Among the whole group, 40,212 (58.80%) were older individuals, 40,682 (59.50%) were female, 67,563 (98.80%) were Han Chinese, 59,259 (86.60%) never smoked, 61,580 (90.00%) never drank and 28,636 (41.80%) were with moderate level of physical activities. Across the whole study period, the daily average concentrations of PM10 and O3 in Guangzhou were 49.28 μg/m3 and 99.46 μg/m3, respectively.
Supplementary Table S3 shows the result of univariate analysis. The association between the risk of all-cause hospital admission and O3, PM10, age, gender, education level, marital status, retirement status, alcohol intake, activity level, BMI and disease history were statistically significant (P < 0.05).
3.2. Association between air pollution and hospital admissions
Table 2 shows the association between air pollution and all-cause hospital admissions in single-pollutant and two-pollutant models. Effect estimates were generally consistent between the two sets of models as well as across the models with sequential adjustment of covariates. According to the final two-pollutant models, each 10 μg/m3 increase in the concentration of O3 and PM10 was associated with a 6.8% higher hazard (HR, 1.068; 95% CI, 1.055, 1.082) and a 3.1% higher hazard of the all-cause hospital admissions (HR, 1.031; 95% CI, 1.013, 1.049), respectively. When we used PM2.5 instead of PM10, we found the changes in the results for O3 were subtle, and we did not observe any significant effect for PM2.5 (Supplementary Table S4).
When the results were stratified by the cause of hospital admissions (Table 3), we found that each 10 μg/m3 increase in the concentration of O3 was associated with a 4.7%-22.8% increase in the risk of hospital admissions due to one of the multiple outcomes except for the respiratory diseases for which no significant signals were detected. Similarly, we found that each 10 μg/m3 increase in the concentration of PM10 was related to a 2.3%−8.1% increase in the risk of hospital admissions due to reasons other than respiratory and digestive diseases. Results were also consistent between the single-pollutant and two-pollutant models.
3.3. Moderating effect
Figure 1 shows the moderating effect of covariates on the association between air pollution (i.e., O3 and PM10) and all-cause hospital admissions, respectively. For O3, we observed significant moderating effect of alcohol intake, BMI, and tobacco abuse. More specifically, participants who drank over limit (HR = 1.103, 95% CI: 1.006–1.211) were more susceptible to the impact of O3 than those who never drank (HR = 1.070, 95% CI: 1.056–1.084) or drank under limit (HR = 1.016, 95% CI: 0.960–1.076) with Pinteraction being 0.052. In contrast, participants with normal BMI (HR = 1.072, 95% CI: 1.054–1.091) tended to be less susceptible than those who were underweight (HR = 1.105, 95% CI: 1.047–1.167), overweight (HR = 1.069, 95% CI: 1.045–1.094) or obese (HR = 1.017, 95% CI: 0.976–1.059) with Pinteraction being 0.011. Similarly, participants who smoked heavily (HR = 1.071, 95% CI: 1.033–1.111) tended to be less susceptible than others (Pinteraction = 0.032). As for PM10, we observed a significant effect of pollution among the older individuals (HR = 1.058, 95% CI: 1.033–1.083) but not among the others (HR = 0.998, 95% CI: 0.971–1.025) with Pinteraction being 0.002.
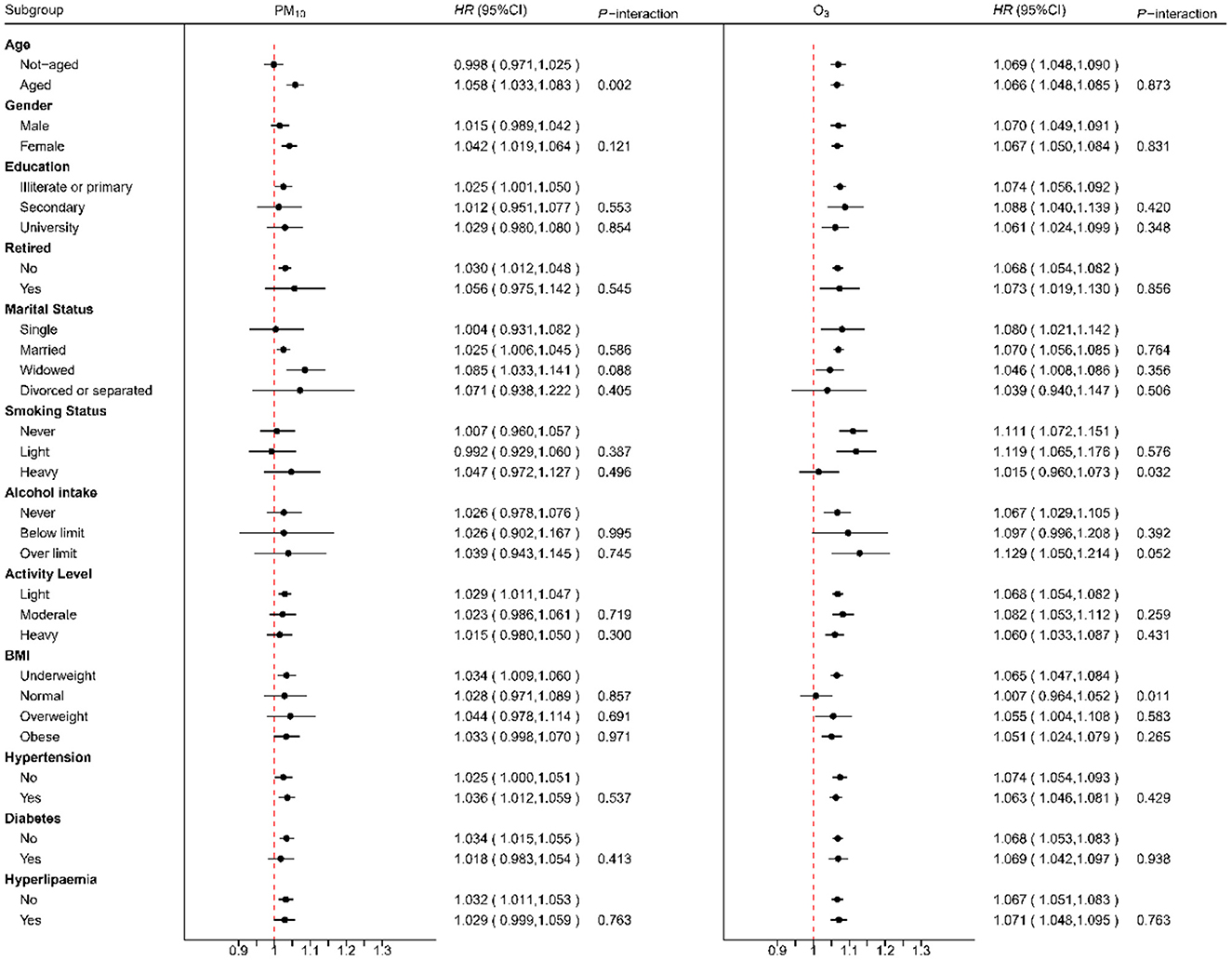
Figure 1. The modification effect on the association between air pollution (O3 and PM10) and all-cause hospitalization by different individual factors.
Supplementary Figures S1–S4 show the moderating effect of individual factors on the associations between air pollution and cause-specific hospital admissions. For O3, non-aged people suffered from a higher risk of hospitalizations due to endocrine, nutritional and metabolic diseases (HR, 95%CI: non-aged, 1.158, 1.069–1.254 vs. aged, 1.069, 0.991–1.123; Pinteraction = 0.154), digestive system diseases (HR, 95%CI: non-aged, 1.125, 1.060–1.194 vs. aged, 1.074, 1.014–1.137; Pinteraction = 0.271) and circulatory system diseases (HR, 95%CI: non-aged, 1.093, 1.038–1.150 vs. aged, 1.020, 0.984–1.058; Pinteraction = 0.060). In addition, underweight people were also more sensitive to the hospitalization due to these causes, HRs of underweight group and normal group for endocrine, nutritional & metabolic diseases were 1.114 (95% CI: 1.012–1.227) vs. 0.945 (95% CI: 0.752–1.186), for digestive system diseases were 1.086 (95% CI: 1.022–1.154) vs. 0.874 (95% CI: 0.748–1.020), for circulatory system diseases were 1.053 (95% CI: 1.014–1.094) vs. 1.012 (95% CI: 0.926–1.105), with Pinteraction being 0.184, 0.004, and 0.412. For PM10, age and smoking status could modify its effect on the hospitalization due to some specific causes. Specifically, aged people had a higher risk to be admitted into hospital due to digestive system diseases with HR being 1.149 (95% CI: 1.063–1.242) compared with non-aged people with Pinteraction being 0.004; people who smoked heavily had a significant higher risk for the hospital admission due to circulatory system diseases with HR being 1.291 (95% CI: 1.080–1.544) compared with lightly smoking people and non-smokers with Pinteraction being 0.013.
4. Discussion
4.1. The association between air pollution and hospitalization
We found that the monthly concentration of PM10 and O3 exposures were positively associated with the risk of all-cause hospitalization. According to our estimates, increased O3 exposure was associated with an increased hospitalization due to each one of the multiple diseases except for respiratory system diseases. In contrast, increased PM10 exposure was only positively associated with the hospital admissions due to respiratory and digestive system diseases. Although few previous studies focused on the health effect of monthly exposure of air pollution, our results were in line with most of the evidence on the acute health effect. For example, a study conducted in southwestern France indicated that every 5 μg/m3 increment in the current-day exposure to O3 associated with a 5% increase in the risk of acute myocardial infarction hospitalization (22). Another study from Southern Europe suggested that each 14.4 μg/m3 elevation in PM10 at lag 0–5 days was associated with a 1.36% increase in the risk of respiratory hospitalization (23).
Air pollution may cause oxidative stress, inflammation, DNA damage and epigenetic changes (24), thereby triggering acute diseases or contributing to the increased rate of exacerbation of preexisting conditions, and leading to a greater risk of inpatient treatment. For instance, Li et al. found that each 10 μg/m3 increase in the 28-day moving average of O3 was correlated with a rise of 3.9% in total cholesterol, 8.2% in low-density lipoprotein cholesterol, 4.8% in Castelli risk indexes I and 7.0% in Castelli risk indexes II (25), and these markers are important risk factors of cardiovascular diseases (26). In addition, it has been widely reported that exposure to O3 elevated the level of systemic oxidative stress (27), which is one of the major contributors to the development of stomach diseases (28). The increase in short-term exposure to O3 was also observed to associate with the elevation of blood glucose and insulin level, and the occurrence of insulin resistance, which plays a vital role in the onset of type 2 diabetes (29). The mechanisms underlying the health effect of PM10 may be similar to that of O3 (30, 31).
4.2. The effect modification of individual factors on the association
We found people who drank over limit were more susceptible to the effect of air pollution on hospitalization. Although few evidence showed over drinking could strengthen the effect of air pollution for hospitalization, Dai et al. indicated that each 7% increase in the prevalence of heavy drinkers at county-level was associated with an increment of 40% in the estimated mortality effect of fine particles (32). The defense function of immune system is the main response to air pollution-induced inflammation for organism (33). However, a high alcohol intake is an essential risk factor for health which adversely affect the functioning of immune system (34, 35).
Surprisingly, we found people who smoked heavily were less vulnerable compared with those who smoked lightly and non-smokers. However, the result was supported by some existing findings. For example, Puett et al. suggested that current smokers had a lower risk of lung cancer associated with particulate matters compared with those who had never smoked or stopped smoking for more than 10 years (36). Tobacco smoke contains several chemical compounds and particulate matters, and was considered one of the major sources of indoor air pollution (37). Therefore, these heavy smokers might get used to air pollution already due to the years of tobacco-stimulation. Another possible reason is the “healthy smoker effect” which is similar to the well-known “healthy worker effect” (38), where the heavy smokers may be healthier than others because the unhealthy ones were more likely to have died or suffered from severe diseases, making them less likely to be included in this study.
We also found that people with abnormal body weight (i.e., underweight, overweight and obese) were more sensitive to the hospital admission associated with air pollution. For underweight people, evidence on their prolonged recovery duration after diseases were well documented (39), and underlying malnutrition in underweight people was a significant threat to their functional recovery (40). So, we speculated that the insufficient recovery capability from air pollution-induced damage may be a possible explanation for the greater susceptibility of underweight people to the effects of air pollution. For overweight and obese people, they had already been in the state of systemic oxidative stress. Air pollution-induced oxidative stress may aggravate their abnormal lipids metabolism (41) and thus attributes to the progression of diseases.
4.3. Limitations
We acknowledge that this study has some limitations, including the potential exposure misclassification by using the interpolation data of air pollution although the data was well validated against the observational data from monitoring stations. Another limitation is that the proportion of the elderly included in our study tended to be higher than the actual proportion reported for the area probably due to the fact the elderly were more likely to register at the Community Healthcare Centers and attended surveys on workdays. Our conclusions need to be extrapolated with cautions and validated with future studies based on more representative samples. Additionally, this is a single-city study which may limit the generalizing our findings. However, Guangzhou is the largest city in South China with a population of ~19 million from all over the country. It's of great significance to investigate the effect of long-term air pollution and identify corresponding modifiers in this area.
5. Conclusion
We found positive association of all-cause and cause-specific hospitalizations with monthly exposure to PM10 and O3. We also observed vulnerable populations to these pollution, such as people who drank over limit and those with abnormal body weight. Our results provided comprehensive evidence for the health effect of monthly exposure to air pollution. Clean-air Policies might be an effective way to reduced hospitalizations and specific measures should be taken to protect the most susceptible groups.
Data availability statement
The raw data supporting the conclusions of this article will be made available by the authors, without undue reservation.
Author contributions
WY, XJ, ZD, WZ, and YH contributed to conception and design of the study. WY, XJ, ZD, XW, and HC organized the database. WY, XJ, SC, YZ, GW, and WW performed the statistical analysis. WY, XJ, ZD, and WZ wrote the first draft of the manuscript. XL, YW, JJ, and WH wrote sections of the manuscript. All authors contributed to manuscript revision, read, and approved the submitted version.
Funding
This work was supported by the National Science and Technology Major Project of the Ministry of Science and Technology of China (grant number 2018ZX10715004), the National Natural Science Foundation of China (grant numbers 82204162 and 82204154), and the Natural Science Foundation of Guangdong Province (grant number 2022A1515010823).
Acknowledgments
The authors gratefully acknowledge the support of K. C. Wong Education Foundation.
Conflict of interest
The authors declare that the research was conducted in the absence of any commercial or financial relationships that could be construed as a potential conflict of interest.
Publisher's note
All claims expressed in this article are solely those of the authors and do not necessarily represent those of their affiliated organizations, or those of the publisher, the editors and the reviewers. Any product that may be evaluated in this article, or claim that may be made by its manufacturer, is not guaranteed or endorsed by the publisher.
Supplementary material
The Supplementary Material for this article can be found online at: https://www.frontiersin.org/articles/10.3389/fpubh.2023.1137196/full#supplementary-material
References
1. Yee J, Cho YA, Yoo HJ, Yun H, Gwak HS. Short-term exposure to air pollution and hospital admission for pneumonia: a systematic review and meta-analysis. Environ Health. (2021) 20:6. doi: 10.1186/s12940-020-00687-7
2. Zhu RX, Nie XH, Chen YH, Chen J, Wu SW, Zhao LH. Relationship between particulate matter (PM(2. 5)) and hospitalizations and mortality of chronic obstructive pulmonary disease patients: a meta-analysis. Am J Med Sci. (2020) 359:354–64. doi: 10.1016/j.amjms.2020.03.016
3. Rajak R, Chattopadhyay A. Short and long term exposure to ambient air pollution and impact on health in India: a systematic review. Int J Environ Health Res. (2020) 30:593–617. doi: 10.1080/09603123.2019.1612042
4. Bell ML, Zanobetti A, Dominici F. Evidence on vulnerability and susceptibility to health risks associated with short-term exposure to particulate matter: a systematic review and meta-analysis. Am J Epidemiol. (2013) 178:865–76. doi: 10.1093/aje/kwt090
5. Cakir Y, Yang Z, Knight CA, Pompilius M, Westbrook D, Bailey SM, et al. Effect of alcohol and tobacco smoke on mtDNA damage and atherogenesis. Free Radic Biol Med. (2007) 43:1279–88. doi: 10.1016/j.freeradbiomed.2007.07.015
6. Zhong J, Karlsson O, Wang G, Li J, Guo Y, Lin X, et al. B vitamins attenuate the epigenetic effects of ambient fine particles in a pilot human intervention trial. Proc Natl Acad Sci USA. (2017) 114:3503–8. doi: 10.1073/pnas.1618545114
7. Shah AS, Langrish JP, Nair H, McAllister DA, Hunter AL, Donaldson K, et al. Global association of air pollution and heart failure: a systematic review and meta-analysis. Lancet. (2013) 382:1039–48. doi: 10.1016/S0140-6736(13)60898-3
8. Jayes L, Haslam PL, Gratziou CG, Powell P, Britton J, Vardavas C, et al. SmokeHaz: Systematic reviews and meta-analyses of the effects of smoking on respiratory health. Chest. (2016) 150:164–79. doi: 10.1016/j.chest.2016.03.060
9. Mostofsky E, Chahal HS, Mukamal KJ, Rimm EB, Mittleman MA. Alcohol and immediate risk of cardiovascular events: a systematic review and dose-response meta-analysis. Circulation. (2016) 133:979–87. doi: 10.1161/CIRCULATIONAHA.115.019743
10. Zhang W, Gao M, Xiao X, Xu SL, Lin S, Wu QZ, et al. Long-term PM(0.1) exposure and human blood lipid metabolism: new insight from the 33-community study in China. Environ Pollut. (2022) 303:119171. doi: 10.1016/j.envpol.2022.119171
11. Kim H-J, Min J-y, Seo Y-S, Min K-b. Association of ambient air pollution with increased liver enzymes in Korean adults. Int J Environ Res Public Health. (2019) 16:1213. doi: 10.3390/ijerph16071213
12. Kim SR, Choi S, Kim K, Chang J, Kim SM, Cho Y, et al. Association of the combined effects of air pollution and changes in physical activity with cardiovascular disease in young adults. Eur Heart J. (2021) 42:2487–97. doi: 10.1093/eurheartj/ehab139
13. Zhang L, An J, Liu M, Li Z, Liu Y, Tao L, et al. Spatiotemporal variations and influencing factors of PM(2.5) concentrations in Beijing, China. Environ Pollut. (2020) 262:114276. doi: 10.1016/j.envpol.2020.114276
14. Ouyang W, Gao B, Cheng H, Hao Z, Wu N. Exposure inequality assessment for PM(2.5) and the potential association with environmental health in Beijing. Sci Total Environ. (2018) 635:769–78. doi: 10.1016/j.scitotenv.2018.04.190
15. Sun Y, Ji M, Jin F, Wang H. Public responses to air pollution in Shandong Province using the online complaint data. ISPRS Int J Geo-Inform. (2021) 10:126. doi: 10.3390/ijgi10030126
16. Sun Y, Jin F, Zheng Y, Ji M, Wang H. A new indicator to assess public perception of air pollution based on complaint data. Appl Sci. (2021) 11:1894. doi: 10.3390/app11041894
17. Liu L, Huang C, Liao W, Chen S, Cai S. Smoking behavior and smoking index as prognostic indicators for patients with esophageal squamous cell carcinoma who underwent surgery: a large cohort study in Guangzhou, China. Tob Induc Dis. (2020) 18:9. doi: 10.18332/tid/117428
18. Saito T, Miyatake N, Sakano N, Oda K, Katayama A, Nishii K, et al. Relationship between cigarette smoking and muscle strength in Japanese men. J Prev Med Public Health. (2012) 45:381–6. doi: 10.3961/jpmph.2012.45.6.381
19. Li HM, Azhati B, Rexiati M, Wang WG Li XD, Liu Q, et al. Impact of smoking status and cumulative smoking exposure on tumor recurrence of non-muscle-invasive bladder cancer. Int Urol Nephrol. (2017) 49:69–76. doi: 10.1007/s11255-016-1441-6
21. Tu R, Li Y, Shen L, Yuan H, Mao Z, Liu X, et al. The prevalence and influencing factors of physical activity and sedentary behaviour in the rural population in China: the Henan Rural Cohort Study. BMJ Open. (2019) 9:e029590. doi: 10.1136/bmjopen-2019-029590
22. Ruidavets J-B, Cournot M, Cassadou S, Giroux M, Meybeck M, Ferrières J. Ozone air pollution is associated with acute myocardial infarction. Circulation. (2005) 111:563–9. doi: 10.1161/01.CIR.0000154546.32135.6E
23. Stafoggia M, Samoli E, Alessandrini E, Cadum E, Ostro B, Berti G, et al. Short-term associations between fine and coarse particulate matter and hospitalizations in Southern Europe: results from the MED-PARTICLES project. Environ Health Perspect. (2013) 121:1026–33. doi: 10.1289/ehp.1206151
24. Brucker N, do Nascimento SN, Bernardini L, Charão MF, Garcia SC. Biomarkers of exposure, effect, and susceptibility in occupational exposure to traffic-related air pollution: a review. J Appl Toxicol. (2020) 40:722–36. doi: 10.1002/jat.3940
25. Li A, Pei L, Zhao M, Xu J, Mei Y, Li R, et al. Investigating potential associations between O3 exposure and lipid profiles: a longitudinal study of older adults in Beijing. Environ Int. (2019) 133(Pt A):105135. doi: 10.1016/j.envint.2019.105135
26. Kopin L, Lowenstein C. Dyslipidemia. Ann Intern Med. (2017)167:Itc81–itc96. doi: 10.7326/AITC201712050
27. Jiang Y, Wang C, Lin Z, Niu Y, Xia Y, Liu C, et al. Alleviated systemic oxidative stress effects of combined atmospheric oxidant capacity by fish oil supplementation: a randomized, double-blinded, placebo-controlled trial. Ecotoxicol Environ Saf. (2019) 184:109598. doi: 10.1016/j.ecoenv.2019.109598
28. Suzuki H, Nishizawa T, Tsugawa H, Mogami S, Hibi T. Roles of oxidative stress in stomach disorders. J Clin Biochem Nutr. (2012) 50:35–9. doi: 10.3164/jcbn.11-115SR
29. Kim JH, Hong YC. GSTM1, GSTT1, and GSTP1 polymorphisms and associations between air pollutants and markers of insulin resistance in elderly Koreans. Environ Health Perspect. (2012) 120:1378–84. doi: 10.1289/ehp.1104406
30. Donaldson K, Stone V, Borm PJ, Jimenez LA, Gilmour PS, Schins RP, et al. Oxidative stress and calcium signaling in the adverse effects of environmental particles (PM10). Free Radic Biol Med. (2003) 34:1369–82. doi: 10.1016/S0891-5849(03)00150-3
31. Wang X, Guo B, Yang X, Li J, Baima Y, Yin J, et al. Role of liver enzymes in the relationship between particulate matter exposure and diabetes risk: a longitudinal cohort study. J Clin Endocrinol Metab. (2022) 107:e4086–e97. doi: 10.1210/clinem/dgac438
32. Dai L, Zanobetti A, Koutrakis P, Schwartz JD. Associations of fine particulate matter species with mortality in the United States: a multicity time-series analysis. Environ Health Perspect. (2014) 122:837–42. doi: 10.1289/ehp.1307568
34. Fernández-Solà J. Cardiovascular risks and benefits of moderate and heavy alcohol consumption. Nature reviews Cardiology. (2015) 12:576–87. doi: 10.1038/nrcardio.2015.91
35. Grønbaek M. The positive and negative health effects of alcohol- and the public health implications. J Intern Med. (2009) 265:407–20. doi: 10.1111/j.1365-2796.2009.02082.x
36. Puett RC, Hart JE, Yanosky JD, Spiegelman D, Wang M, Fisher JA, et al. Particulate matter air pollution exposure, distance to road, and incident lung cancer in the nurses' health study cohort. Environ Health Perspect. (2014) 122:926–32. doi: 10.1289/ehp.1307490
37. Vardoulakis S, Giagloglou E, Steinle S, Davis A, Sleeuwenhoek A, Galea KS, et al. Indoor exposure to selected air pollutants in the home environment: a systematic review. Int J Environ Res Public Health. (2020) 17:8972. doi: 10.3390/ijerph17238972
38. Eisner MD. Smoking and adult asthma: a healthy smoker effect? Am J Respir Crit Care Med. (2002) 165:1566; author reply–7. doi: 10.1164/ajrccm.165.11.165112
39. Weidman M, MacDonald SL. The impact of body mass index classification on outcomes after stroke rehabilitation: a retrospective study. Am J Phys Med Rehabil. (2022) 101:714–7. doi: 10.1097/PHM.0000000000001966
40. Nii M, Maeda K, Wakabayashi H, Nishioka S, Tanaka A. Nutritional improvement and energy intake are associated with functional recovery in patients after cerebrovascular disorders. J Stroke Cerebrovasc Dis. (2016) 25:57–62. doi: 10.1016/j.jstrokecerebrovasdis.2015.08.033
Keywords: hospitalizations, air pollution, effect modification, time-dependent Cox proportional model, particulate matter
Citation: Ju X, Yimaer W, Du Z, Wang X, Cai H, Chen S, Zhang Y, Wu G, Wu W, Lin X, Wang Y, Jiang J, Hu W, Zhang W and Hao Y (2023) The impact of monthly air pollution exposure and its interaction with individual factors: Insight from a large cohort study of comprehensive hospitalizations in Guangzhou area. Front. Public Health 11:1137196. doi: 10.3389/fpubh.2023.1137196
Received: 04 January 2023; Accepted: 01 March 2023;
Published: 21 March 2023.
Edited by:
Jun Yang, Guangzhou Medical University, ChinaReviewed by:
Zhongjun Shao, Air Force Medical University, ChinaQi Zhao, Shandong University, China
Jianxiong Hu, Guangdong Provincial Center for Disease Control and Prevention, China
Copyright © 2023 Ju, Yimaer, Du, Wang, Cai, Chen, Zhang, Wu, Wu, Lin, Wang, Jiang, Hu, Zhang and Hao. This is an open-access article distributed under the terms of the Creative Commons Attribution License (CC BY). The use, distribution or reproduction in other forums is permitted, provided the original author(s) and the copyright owner(s) are credited and that the original publication in this journal is cited, in accordance with accepted academic practice. No use, distribution or reproduction is permitted which does not comply with these terms.
*Correspondence: Wangjian Zhang, emhhbmd3ajIyN0BtYWlsLnN5c3UuZWR1LmNu; Yuantao Hao, aGFveXRAYmptdS5lZHUuY24=
†These authors have contributed equally to this work