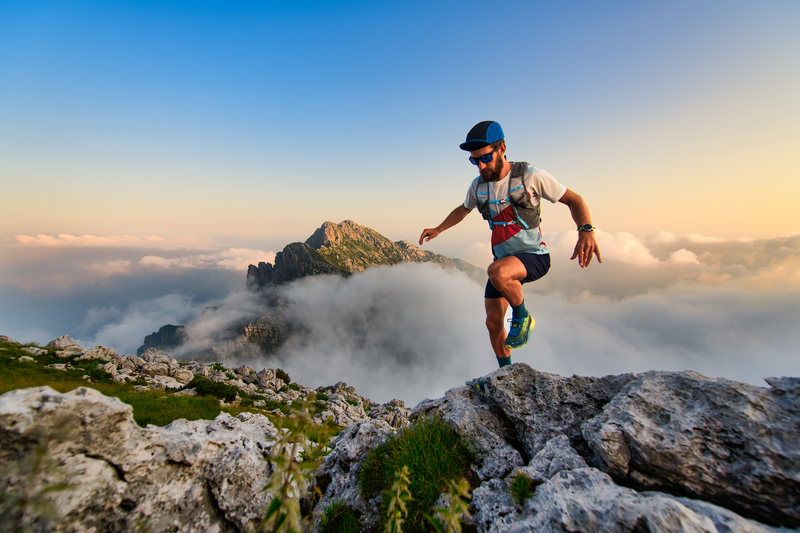
94% of researchers rate our articles as excellent or good
Learn more about the work of our research integrity team to safeguard the quality of each article we publish.
Find out more
SYSTEMATIC REVIEW article
Front. Public Health , 10 January 2023
Sec. Infectious Diseases: Epidemiology and Prevention
Volume 10 - 2022 | https://doi.org/10.3389/fpubh.2022.1022055
The coronavirus disease (COVID-19) outbreak has turned the world upside down bringing about a massive impact on society due to enforced measures such as the curtailment of personal travel and limitations on economic activities. The global pandemic resulted in numerous people spending their time at home, working, and learning from home hence exposing them to air contaminants of outdoor and indoor origins. COVID-19 is caused by the Severe Acute Respiratory Syndrome Coronavirus 2 (SARS-CoV-2), which spreads by airborne transmission. The viruses found indoors are linked to the building's ventilation system quality. The ventilation flow in an indoor environment controls the movement and advection of any aerosols, pollutants, and Carbon Dioxide (CO2) created by indoor sources/occupants; the quantity of CO2 can be measured by sensors. Indoor CO2 monitoring is a technique used to track a person's COVID-19 risk, but high or low CO2 levels do not necessarily mean that the COVID-19 virus is present in the air. CO2 monitors, in short, can help inform an individual whether they are breathing in clean air. In terms of COVID-19 risk mitigation strategies, intelligent indoor monitoring systems use various sensors that are available in the marketplace. This work presents a review of scientific articles that influence intelligent monitoring development and indoor environmental quality management system. The paper underlines that the non-dispersive infrared (NDIR) sensor and ESP8266 microcontroller support the development of low-cost indoor air monitoring at learning facilities.
The COVID-19 outbreak has had a significant impact on daily lives affecting work, school, the healthcare system, and the pursuit of pleasure. Since the outbreak became an altering global health crisis, the pandemic is nearing its end. Nevertheless, the public faces the threat of COVID-19 common symptoms such as coughs, headaches, eye irritation, dizziness, fatigue, and death. The rapid transmission of the deadly virus led to a strong emphasis on health precautions and prevention techniques by government officials and health institutions worldwide to curb its spread. Several situations can exacerbate the number of COVID-19 cases, particularly from a socio-demographic level, including lack of essential services, slums and impoverished settlements, the density of public transportation, the duration of a journey, and the state of a country's infrastructure. Furthermore, climatic factors such as temperature, airflow, air quality, and humidity may also contribute to increased COVID-19 cases. In Spain's capital city of Madrid, the mortality rate was 312 deaths per 100,000 inhabitants on 5 May 2020 (1). Individuals suffering from respiratory disease and people aged 60 years and above who were exposed to poor air quality (2) had a higher mortality rate. During Spain's first outbreak, the lifestyle of its residents underwent a drastic change because the entire Spanish population was placed under a 24/7 lockdown for 45 days. As such, people were mandated to stay at home and could only leave to perform essential activities (1). In the education sector, the pandemic affected class attendance, academic performance, and children's health. Consequentially, numerous countries had to grapple with a health crisis as well as economic shocks that affected human capital, productivity, and society.
According to the World Health Organization, the primary mechanism of COVID-19 transmission is between people, particularly in situations where there is extended and unprotected virus exposure (3). The SARS-CoV-2 virus—which causes COVID-19—is emitted from a contagious person's respiratory system and transmitted to a responsive host. There are three major routes for the spread of COVID-19, namely droplet transmission, close contact, and airborne transmission (4–8). Particulate matter (PM) is also known as particle pollution in the air that comes in solid and/or liquid form. The size of the virus particles ranges from sub-micrometers to a few micrometers whilst the pathogens are contained in fluid-based particles that are aerosolized from respiratory tract sites during activities such as coughing, breathing, sneezing, and speaking (9). Previous studies found that indoor air circulation is a crucial factor behind virus viability, therefore, poor ventilation, loud vocalization, density occupancy, and stay duration (7, 10, 11) increase environmental risk factors in transmitting the virus. The research conducted by Baboli revealed that the essential condition which harbors the presence of the virus indoors includes low levels of humidity and temperature, a high PM level, and the absence of any air filtration (12).
In addition, outdoor temperatures are hotter than usual due to global warming which can influence people's choices to spend time indoors working, learning, and relaxing. Therefore, it is important to measure indoor air quality as it is one of the most significant real-time indicators for today's urban environment given how it significantly influences human health, safety, and comfort (13); the Air Quality Index (AQI) represents a quantitative level of air pollution. Indoor air pollution is influenced by how building ventilation systems are planned, managed, and maintained thus when rooms are not well-ventilated, there is a risk of cross-infection. Minimizing indoor air pollution is the first and best measure to ensure indoor environmental quality whereas providing sufficient ventilation supports mitigation strategies of airborne disease transmissions. Adequate ventilation helps to control the movement and advection of any aerosols, pollutants, and CO2 created by indoor sources/occupants (14). One of the essential parameters of indoor CO2 level scales quite accurately with the number of individuals in a room and their activity level (8, 15). As such, measuring CO2 levels can indicate the presence of sufficient ventilation which in turn, aids in the prevention of respiratory virus infections in humans. Indoor CO2 monitoring is, thus, an effective technique to assess an individual's COVID-19 risk. However, high, or low CO2 levels cannot directly detect COVID-19 whilst close contact poses the highest risk for COVID-19 transmission.
The SARS-CoV-2 virus will not disappear given that it has anchored itself as part of our lives. Even though people have received COVID-19 vaccinations and normal activities resumed by wearing face masks, the risk of reinfection still lingers. The SARS-CoV-2 virus infects the upper respiratory system and is dangerous and potentially lethal given that infected persons could develop severe symptoms and illnesses while those who have recovered may suffer from “Long COVID”. A COVID-19 infection could lead to hospitalizations which can be particularly expensive in some countries. The elderly, unvaccinated people and persons with underlying medical conditions are the most at-risk groups due to a weakened immune system. The SARS-CoV-2 virus is known for its rapid transmission, yet no person is naturally immune to the virus underscoring the extreme danger of the illness. Essentially, maintaining a safe and healthy environment is crucial to breaking the COVID-19 chain of infection. In terms of COVID-19 risk mitigation, zoning can be implemented by remote sensing and Geographic Information System (GIS) with several parameters such as hazard data, socioeconomic data, and biophysical data enabled to categorized of five zones that are representative of colors, beginning with a very high-risk zone (red), the high-risk (orange), the moderate zone (blue), the low-risk zone (green), until the very low zone (pink) (16–18). Certain countries such as Germany, India, the USA, and South Africa have experienced more than two COVID-19 waves (19). Meanwhile, other countries have reported mutations of the SARS-CoV-2 virus in addition to cases of the monkeypox disease that also spreads through respiratory droplets (20). Most disease transmissions occur indoors thus the development of intelligent air monitoring and management system—boosted by cutting-edge technologies, like cloud computing and the Internet of Things (IoT)—is vital. Intelligent indoor air monitoring comprises many sensors that are sold at various prices in the marketplace. Low-cost sensors based on Electrochemical Cell (EC), Metal-oxide Semiconductor (MOS), Non-Dispersive Infrared (NDIR), nephelometry, and Optical Particle Counters (OPC) promise the indoor air-monitoring as user-friendly, easy deployment portable devices (15, 21, 22). This study aims to analyze the potential of indoor air monitoring and alternative solutions to minimize disease transmissions. In this paper, the self-assembly of an indoor monitoring system using inexpensive sensors is aimed to support the realization of a healthy indoor environment, particularly in reducing COVID-19 risks.
The structure of this paper is as follows: the first section reviews the background and previous research, the method is explained in methods and result section presents the research results. To deepen the understanding of other objective studies, features a discussion section. The conclusion is outlined in conclusion section along with future research directions.
To accomplish this study, several electronic databases inclusive of Scopus, Web of Science (WoS), ScienceDirect, IEEE Xplore, Google Scholar, and EBSCO databases as well as the keywords “indoor ventilation transmission COVID19”, “indoor room monitoring COVID19”, “air quality index COVID19”, “cloud computing COVID19”, “internet of things COVID19” were used in systematic searching. The Preferred Reporting Items for Systematic Reviews and Meta-Analyses (PRISMA) approach is an evidence-based minimum set of items to conduct a Systematic Review for the identification, screening, evaluation, and analysis of the eligibility of all published studies relevant to a certain Research Topic (23).
There were 1,980 extracted papers from the electronic databases mentioned above which have been peer-reviewed and were published between 2019 and 28th February 2022. After the extraction process, 1,550 duplicate records were removed, and 430 papers were screened based on the title and the abstract. In total, there were 158 eligible articles, but 104 were excluded for a few reasons: not available in English, it was a conference abstract, or the focus was not on indoor monitoring. A file (“.ris” format) in the Mendeley Reference Manager contained 54 full-text electronic English references. Figure 1 shows the working of PRISMA method in the flow diagram.
The corpus contained five databases in which Google Scholar had the highest percentage and the WoS had the lowest, as shown in Table 1. Figure 2 shows the distribution of the examined publications which has increased since 2019 and reached a peak in publications in 2021.
The journals from Elsevier had the most publications and the most frequently identified was the journal Sensors from MDPI publishers. Table 2 shows the classification of study types conducted by the researchers which consist of 45 experimental study papers and 9 review papers from various interdisciplinary studies.
Table 3 represents the classification data references present in the corpus. The Building and Environment journal in the building domain focuses on building science, urban physics, and human interaction with the indoor and outdoor built environment (67). The Sensors journal accommodates the original contribution submission in science and technology of sensors and their application (68). The Journal of Sustainable Cities and Society specializes in environmentally sustainable and socially resilient cities (69). The Environmental Research journal welcomes a multi-disciplinary approach aimed at anthropogenic issues of global relevance and applicability in a wide range of environmental disciplines and demonstrates environmental application in the real-world context (70).
Chinese academics and researchers have contributed toward COVID-19 mitigation, meanwhile, academics and researchers from Italy and the Republic of Korea have demonstrated a strong interest in deepening the study concerning COVID-19 indoor monitoring topics. Table 4 represents the classification data based on the Author's country. The experimental study on indoor monitoring used a wide range of locations comprising university classrooms, university laboratories, university offices, classrooms in daycare centers, hospital rooms, and houses.
Researchers expanded their focus beyond measuring CO2 by also measuring temperature and humidity (27, 35, 65, 66). To simplify the monitoring process, wireless technologies such as ZigBee were additionally utilized (52, 65, 71). Although researchers deployed their low-cost indoor monitoring system, no explanation has been provided about the significance of monitoring measures in reducing COVID-19 cases. Regardless, indoor monitoring data can be used to predict COVID-19 risks.
Table 5 shows the potential area of indoor air monitoring, the duration of observation, and the sensors/technologies used in building non-commercial indoor air monitoring monitors. Kim and the team evaluated indoor air quality based on the national guidance centers in South Korea and discovered that most indoor air contaminants were found during the opening hours of daycare centers (a public area) even though the centers operated per the Regulations for Building Systems (Ministry of Land, Infrastructure, and Transport) and the Indoor Air Quality Control Act (Ministry of Environment) in South Korea (72). They found that CO2, PM10, and PM2.5 possessed the capability of becoming a media for infectious viruses and that the density and activity of indoor occupants are the driving factors of indoor air contaminants.
Most of the research found a correlation between seasonality and increased COVID-19 cases. The researchers from our study concluded that SARS-CoV-2 spreads slower in the summer than in the winter demonstrating the significant role of humidity and temperature. Elsaid (25) provided guidelines and recommendations to achieve indoor air quality in air-conditioned areas. It includes ensuring the height of an exhaust air line is at least 5 meters from the end of a building's ceiling and increasing the exhaust fan size. Another suggestion is to reach an indoor temperature of 25–27°C and relative humidity of 50–70% which can suppress the SARS-CoV-2 virus from spreading (25, 73). However, Meraj and his teams (74) found that the spreading of COVID-19 was not influenced by temperature significantly when they investigated three areas with distinct climatic (subtropical, desertic, coastal) in India.
Agarwal (2) found that there was a positive correlation between air pollution (NO2 and PM2.5) and COVID-19 contamination in the air quality index. Natural ventilation uses vents, louvers, windows, or mechanical ventilation systems. In addition, an effective non-medical action that can considerably reduce infection risk is social distancing in indoor areas.
Marques (52) introduced a solution to CO2 real-time monitoring using an IoT system and called it the iAirCO2 platform. There are some sophisticated technological features of the iAirCO2 as it consists of Web as well as smartphone software for data consulting in addition to a hardware mock-up for the collection of latent data. Ultimately, the objective is for doctors to be able to access these data in the future to help them make a medical diagnosis. Compared to other systems, the iAirCO2 relies on open-source technology and offers a comprehensive wireless system with benefits such as scalability, flexibility, affordable pricing, and a simple installation. These findings demonstrate that the production of a system can serve as a credible indoor air quality assessment and facilitates the prediction of technical adjustments that lead to a better living environment.
Salman et al. (71) described the design and implementation of wireless sensor units to examine indoor air quality for real-time data capture. This is accomplished using infrared sensors that detect humidity, CO2, and temperature as well as low-power wireless networking and geographical prediction using geostatistical approaches. The platform contains an MBED LPC 1768 board that collects the sensor unit data and delivers it to a central base station through a ZigBee module where it is analyzed and stored.
Gilio et al. (27) conducted experiments, split into two evaluation stages, concerning CO2 levels real-time monitoring during the reopening of schools in the Apulia Region of southern Italy. The first evaluation was the preliminary procedure where classroom activities in nine schools (11 classrooms) were assessed. A detailed air ventilation operating protocol was also implemented to determine whether there would be an improvement. Even though the windows and doors were opened for air circulation during the first assessment stage, six classrooms (54%) reached CO2 concentration levels of more than 1,000 ppm. It means all the classrooms exceeded the prescribed CO2 levels of 700 ppm. The second evaluation stage sought to improve the conditions from the first phase by applying a detailed air ventilation operating protocol. NDIR sensors were deployed to simultaneously visualize real-time CO2 levels as part of the CO2 monitoring process.
Ng et al. (53) tested NDIR CO2 gas sensing by applying pyroelectric detectors that are CMOS compatible and MEMS ScAlN-based. The researchers used an eight-inch wafer level technology and 12 percent Sc-doped AlN stored at 200°C to create their ScAlN pyroelectric detectors. Using a blackbody thermal emitter, a 10-centimeter (cm) long enclosed gas channel with inlet and outlet holes connected to some tubing, and carrying out tests utilizing two types of reference gases (synthetic air and N2), the experiment resulted in a voltage signal drop. The drop is believed to be caused by the CO2 gas absorption—specifically at the 4.26 μm wavelength—at CO2 gas concentrations that ranged from 25 parts per million (ppm) to 5,000 ppm. The findings suggest that affordable, monolithic, wafer-level NDIR gas sensors with a minimal footprint that is merged with CMOS circuits are possible to achieve by using pyroelectric detectors.
Chojer investigated the development of low-cost indoor air monitoring devices and found that most devices were lack of assessment. Using the standard factory calibration setting and ML models such as Multiple Linear Regression, Support Vector Regression, and Gradient Boosting Regression as sensor calibrations were needed for data reliability, especially measuring the response time that played an essential role in real-time monitoring (15, 22).
The COVID-19 outbreak in the Hubei province, specifically Wuhan, China had been initially considered pneumonia back in December 2019. The quick-spreading nature of this coronavirus disease became apparent as global case numbers rose and nations reported cases of the SARS-CoV-2 virus in their respective countries. This demonstrates how infectious diseases are frequently caused by viruses. Characteristics of diseases include being highly pathogenic and contagious as well as being easily transmissible in congested or poorly ventilated indoor spaces, such as health facilities and public locations. People spend nearly three-quarters of their day indoors hence exposing them to a variety of external and internal air contaminants.
For indoor environments, the quality of the ventilation construction system affects airborne transmission which is a crucial route for the spread of contagious viruses. The CO2 concentration of indoor spaces possesses a significant impact on airborne transmission by way of indoor air and it can impact mental activity, increase reading errors, reduce cognitive and behavioral responses, increase end-tidal CO2 levels, decrease heart rate, spur breathing problems, and cause unconsciousness (38). As such, an increase in CO2 concentration signals inadequate ventilation and, if an infected person is nearby, would boost the risk of a COVID-19 infection (1, 8, 72). In addition, humidity level and temperature have been found to exacerbate the transmission of the virus. Most research found a correlation between seasonality and increased COVID-19 cases where the spread of SARS-CoV-2 in the winter is faster than in summer (25).
Learning activities in educational buildings, such as classrooms were primarily observed as part of the monitoring system (27, 28, 30, 31, 39, 62, 65). The prevention of SARS-CoV-2 transmission inside such infrastructures and the safe reopening of some public spaces and educational facilities were implemented through mechanical or non-mechanical means. The mechanical strategy adopts intelligent air ventilation made up of sensors and the sensor data will be transmitted to the cloud computing system thus easing users in terms of monitoring and controlling (28, 65, 66). Nowadays, CO2 monitors are available in the marketplace and moreover, the Federation of European Heating, Ventilation and Air Conditioning Associations (REHVA) recommends installing CO2 sensors to alert against poor ventilation in indoor areas (27). Eleven of the fifty four conducted studies examined the use of sensors (31, 35, 36, 39, 43, 44, 49, 53, 56, 62, 63, 65, 66), and indicated that the NDIR sensor is the simplest and most common technology (8, 15). The mechanism of the NDIR sensor is to evaluate the value of light that is absorbed across wavelengths. Because CO2 absorbs light at wavelengths, other gases present provide minimal interference although temperature and humidity react with readout. Other than the NDIR sensor, the microcontroller ESP8266 can also support the creation of low-cost indoor air monitoring (31, 50). Powering the ESP8266 with a wireless module allows for the supervision of ambient temperature in real time. The results from the monitoring system are stored and projected on a screen that users can view every 30 min. Ensuring data reliability by calibrating the devices of the low-cost indoor air monitoring before they were used.
Constructing a building with a low-cost physical barrier of at least 60 cm in height above the desk surface and arranging the distance of personnel working areas (within 4 m of the outlet) (6) are some preventive measures to maintain a safe and healthy environment for open offices. Initiatives including lockdown restrictions, using facemasks, hand washing, practicing physical distancing of more than 2 m, and using disinfectants on surfaces in crowded places are supplementary, but also effective means.
By observing the indoor air quality of public places like health facilities, education facilities, offices, and other buildings, decision-makers can move forward with shaping and enforcing policies as well as programs to prevent the spread of COVID-19. Enhancing building ventilation systems and using low-cost assembly sensors are some of the ways to improve indoor air quality. The NDIR sensor and ESP8266 microcontroller are used to monitor CO2 which has a significant part in airborne transmission. Intelligent indoor monitoring mitigates the SARS-CoV-2 spread and the risks of other common symptoms such as coughs, headaches, eye irritation, dizziness, and fatigue. Innovative technologies may help alleviate the ongoing COVID-19 pandemic and help prevent similar global crises in the future. From an engineering perspective, sensors equipped with a cloud computing architecture can be used to build hygienic ventilation systems with low energy consumption. Other uncomplicated ways include wearing a face mask and maintaining a physical distance. Although there have been many contributions regarding this multidisciplinary subject, most are still in the early stages of development and require further refinement, providing an abundance of opportunities for researchers.
The raw data supporting the conclusions of this article will be made available by the authors, without undue reservation.
Conceptualization: C-TY and P-YL. Methodology: RW, P-YL, and EK. Software: RW and C-TY. Validation: C-TY, T-KY, and P-YL. Formal analysis: C-TY. Investigation: P-YL. Resources: RW, EK, and C-TY. Data curation and visualization: RW. Writing: RW, C-TY, and P-YL. Supervision: C-YH, P-YL, and C-TY. All authors have read and agreed to the published version of the manuscript.
This work was funded in part by the National Science and Technology Council (NTSC), Taiwan under (Grant Nos. 110-2221-E-029-020-MY3, 111-2621-M-029-004, and 111-2622-E-029-003) and also by Taichung Veterans General Hospital (TCVGH), Taiwan under (Grant Nos. TCVGH-1123901C and TCVGH-1123901D).
The authors declare that the research was conducted in the absence of any commercial or financial relationships that could be construed as a potential conflict of interest.
All claims expressed in this article are solely those of the authors and do not necessarily represent those of their affiliated organizations, or those of the publisher, the editors and the reviewers. Any product that may be evaluated in this article, or claim that may be made by its manufacturer, is not guaranteed or endorsed by the publisher.
1. Domínguez-amarillo S, Fernández-agüera J, Cesteros-garcía S, González-lezcano RA. Bad air can also kill: Residential indoor air quality and pollutant exposure risk during the covid-19 crisis. Int J Environ Res Public Health. (2020) 17:1–34. doi: 10.3390/ijerph17197183
2. Agarwal N, Meena CS, Raj BP, Saini L, Kumar A, Gopalakrishnan N, et al. Indoor air quality improvement in COVID-19 pandemic: review. Sustain Cities Soc. (2021) 70:102942. doi: 10.1016/j.scs.2021.102942
3. Noorimotlagh Z, Jaafarzadeh N, Martínez SS, Mirzaee SA, A. systematic review of possible airborne transmission of the COVID-19 virus (SARS-CoV-2) in the indoor air environment. Environ Res. (2021) 193:110612. doi: 10.1016/j.envres.2020.110612
4. Burridge HC, Bhagat RK, Stettler MEJ, Kumar P, de Mel I, Demis P, et al. The ventilation of buildings and other mitigating measures for COVID-19: a focus on wintertime. Proc Royal Soc Math Phys Eng Sci. (2021) 477:20200855. doi: 10.1098/rspa.2020.0855
5. Adam MG, Tran PTM, Balasubramanian R. Air quality changes in cities during the COVID-19 lockdown: a critical review. Atmos Res. (2021) 264:105823. doi: 10.1016/j.atmosres.2021.105823
6. Ren C, Xi C, Wang J, Feng Z, Nasiri F, Cao SJ, et al. Mitigating COVID-19 infection disease transmission in indoor environment using physical barriers. Sustain Cities Soc. (2021) 74:103175. doi: 10.1016/j.scs.2021.103175
7. Hou D, Katal A, Wang L, Professor A. Bayesian Calibration of CO2, Ventilation and COVID-19 Airborne Risk 1 Bayesian Calibration of Using CO2 Sensors to Assess Ventilation Conditions and Associated 1 COVID-19 Airborne Aerosol Transmission Risk in Schools 2.
8. Eykelbosh A. Indoor CO2 Sensors for COVID-19 risk Mitigation: Current Guidance and Limitations. Available online at: ncceh.ca/documents/field-inquiry/indoor-co2-sensors-covid-19-risk-mitigation-current-guidance-and.2021 (accessed 2021).
9. Morawska L, Milton DK. It Is Time to Address Airborne Transmission of Coronavirus Disease 2019 (COVID-19). Vol. 71, Clinical Infectious Diseases. Oxford: Oxford University Press (2020). p. 2311–3. doi: 10.1093/cid/ciaa939
10. Miller SL, Nazaroff WW, Jimenez JL, Boerstra A, Buonanno G, Dancer SJ, et al. Transmission of SARS-CoV-2 by inhalation of respiratory aerosol in the Skagit Valley Chorale superspreading event. Indoor Air. (2021) 31:314–23. doi: 10.1111/ina.12751
11. Amnuaylojaroen T, Parasin N. The association between COVID-19, air pollution, and climate change. Front Public Health Front Media SA. (2021) 9:918. doi: 10.3389/fpubh.2021.662499
12. Baboli Z, Neisi N, Babaei AA, Ahmadi M, Sorooshian A, Birgani YT, et al. On the airborne transmission of SARS-CoV-2 and relationship with indoor conditions at a hospital. Atmos Environ. (2021) 261:118563. doi: 10.1016/j.atmosenv.2021.118563
13. Idrees Z, Zou Z, Zheng L. Edge computing based IoT architecture for low cost air pollution monitoring systems: a comprehensive system analysis, design considerations & development. Sensors. (2018) 18:3021. doi: 10.3390/s18093021
14. Piscitelli P, Miani A, Setti L, de Gennaro G, Rodo X, Artinano B, et al. The Role of Outdoor and Indoor Air Quality in the Spread of SARS-CoV-2: Overview and Recommendations by the Research Group on Covid-19 and Particulate Matter (Rescop Commission). Cambridge: Environmental Research Academic Press Inc. (2022). doi: 10.1016/j.envres.2022.113038
15. Chojer H, Branco PTBS, Martins FG, Alvim-Ferraz MCM, Sousa SIV. Development of low-cost indoor air quality monitoring devices: recent advancements. Sci Total Environ. (2020) 727:138385. doi: 10.1016/j.scitotenv.2020.138385
16. Chandel RS, Kanga S, Singh SK. Impact of COVID-19 on tourism sector: a case study of Rajasthan, India. AIMS Geosci. (2021) 7:224–43. doi: 10.3934/geosci.2021014
17. Kanga S, Sudhanshu, Meraj G, Farooq M, Nathawat MS, Singh SK. Reporting the management of COVID-19 threat in India using remote sensing and GIS based approach. Geocarto Int. (2020) 37:1–8. doi: 10.1080/10106049.2020.1778106
18. Kanga S, Meraj G. Sudhanshu, Farooq M, Nathawat MS, Singh SK. Analyzing the risk to COVID-19 infection using remote sensing and GIS. Risk Anal. (2021) 41:801–13. doi: 10.1111/risa.13724
19. Rajeshbhai SP, Dhar SS, Shalabh. Fourth Wave of COVID-19 in India : Statistical Forecasting. medRxiv (2022). doi: 10.1101/2022.02.23.22271382
20. WHO. Multi-Country Monkeypox Outbreak: Situation Update. (2022). Available online at: https://www.who.int/emergencies/disease-outbreak-news/item/2022-DON393
21. Zheng H, Krishnan V, Walker S, Loomans M, Zeiler W. Laboratory evaluation of low-cost air quality monitors and single sensors for monitoring typical indoor emission events in Dutch daycare centers. Environ Int. (2022) 166:107372. doi: 10.1016/j.envint.2022.107372
22. Chojer H, Branco PTBS, Martins FG, Alvim-Ferraz MCM, Sousa SIV. Can data reliability of low-cost sensor devices for indoor air particulate matter monitoring be improved?—An approach using machine learning. Atmos Environ. (2022) 286:119251. doi: 10.1016/j.atmosenv.2022.119251
23. Hajjaji Y, Boulila W, Farah IR, Romdhani I, Hussain A. Big data and IoT-based applications in smart environments: a systematic review. Comput Sci Rev. (2021) 39:100318. doi: 10.1016/j.cosrev.2020.100318
24. Dinoi A, Feltracco M, Chirizzi D, Trabucco S, Conte M, Gregoris E, et al. A review on measurements of SARS-CoV-2 genetic material in air in outdoor and indoor environments: Implication for airborne transmission. Sci Total Environ. (2022) 809:151137. doi: 10.1016/j.scitotenv.2021.151137
25. Elsaid AM, Ahmed MS. Indoor air quality strategies for air-conditioning and ventilation systems with the spread of the global coronavirus (COVID-19) epidemic: improvements and recommendations. Environ Res. (2021) 199:111314. doi: 10.1016/j.envres.2021.111314
26. Singh RP, Javaid M, Haleem A, Suman R. Internet of things (IoT) applications to fight against COVID-19 pandemic. Diabetes Metabolic Syndrome Clin Res Rev. (2020) 14:521–4. doi: 10.1016/j.dsx.2020.04.041
27. Aguilar AJ. de la Hoz-Torres ML, Costa N, Arezes P, Martínez-Aires MD, Ruiz DP. Assessment of ventilation rates inside educational buildings in Southwestern Europe: analysis of implemented strategic measures. J Build Eng. (2022) 51:104204. doi: 10.1016/j.jobe.2022.104204
28. Assante D, Fornaro C. “An Educational IoT-based Indoor Environment Monitoring System; An Educational IoT-based Indoor Environment Monitoring System,” In: 2019 IEEE Global Engineering Education Conference (EDUCON). (2019). doi: 10.1109/EDUCON.2019.8725262
29. Asthana P, Mishra S. “IoT Enabled Real Time Bolt based Indoor Air Quality Monitoring System,” In: 2018 International Conference on Computational and Characterization Techniques in Engineering and Sciences, CCTES 2018. (2019). p. 36–9. doi: 10.1109/CCTES.2018.8674076
30. Monge-Barrio A, Bes-Rastrollo M, Dorregaray-Oyaregui S, González-Martínez P, Martin-Calvo N, López-Hernández D, et al. Encouraging natural ventilation to improve indoor environmental conditions at schools. Case studies in the north of Spain before and during COVID. Energy Build. (2022) 254:111567. doi: 10.1016/j.enbuild.2021.111567
31. Bidila T, Pietraru RN, Ionita AD, Olteanu A. “Monitor indoor air quality to assess the risk of COVID-19 transmission,” In: Proceedings - 2021 23rd International Conference on Control Systems and Computer Science Technologies, CSCS 2021. Piscataway, NJ: Institute of Electrical and Electronics Engineers Inc. (2021). p. 356–61. doi: 10.1109/CSCS52396.2021.00065
32. Carlotti P, Massoulié B, Morez A, Villaret A, Jing L, Vrignaud T, et al. Respiratory pandemic and indoor aeraulics of classrooms. Build Environ. (2022) 212:108756. doi: 10.1016/j.buildenv.2022.108756
33. Chen CY, Chen PH, Chen JK, Su TC. Recommendations for ventilation of indoor spaces to reduce COVID-19 transmission. J Formosan Med Assoc. (2021) 120:2055–60. doi: 10.1016/j.jfma.2021.08.007
34. Chen H, Li S, Zhang J, Peng X. “Indoor formaldehyde monitoring system based on fog computing,” In: IOP Conference Series: Materials Science and Engineering Institute of Physics Publishing. (2019). doi: 10.1088/1757-899X/569/4/042048
35. Choe Y, Shin J. shup, Park J, Kim E, Oh N, Min K, et al. Inadequacy of air purifier for indoor air quality improvement in classrooms without external ventilation. Build Environ. (2022) 207:108450. doi: 10.1016/j.buildenv.2021.108450
36. Cicceri G, Scaffidi C, Benomar Z, Distefano S, Puliafito A, Tricomi G, et al. “Smart healthy intelligent room: headcount through air quality monitoring,” In: Proceedings - 2020 IEEE International Conference on Smart Computing, SMARTCOMP 2020. Piscataway, NJ: Institute of Electrical and Electronics Engineers Inc. (2020). p. 320–5. doi: 10.1109/SMARTCOMP50058.2020.00071
37. Dhanalakshmi S, Poongothai M, Sharma K. IoT based indoor air quality and smart energy management for HVAC system. Procedia Comput Sci. (2020) 171:1800–9. doi: 10.1016/j.procs.2020.04.193
38. Dinh TV, Lee JY, Ahn JW, Kim JC. Development of a wide-range non-dispersive infrared analyzer for the continuous measurement of CO2 in indoor environments. Atmosphere. (2020) 11:1024. doi: 10.3390/atmos11101024
39. di Gilio A, Palmisani J, Pulimeno M, Cerino F, Cacace M, Miani A, et al. CO2 concentration monitoring inside educational buildings as a strategic tool to reduce the risk of SARS-CoV-2 airborne transmission. Environ Res. (2021) 202:111560. doi: 10.1016/j.envres.2021.111560
40. Goyal P, Prit G, Bharti M. “Smart indoor weather monitoring framework using IoT devices and cloud computing,” In: 2020 6th International Conference on Signal Processing and Communication, ICSC 2020. (2020). p. 216–21. doi: 10.1109/ICSC48311.2020.9182769
41. Guo Y, Zhang N, Hu T, Wang Z, Zhang Y. Optimization of energy efficiency and COVID-19 pandemic control in different indoor environments. Energy Build. (2022) 261:111954. doi: 10.1016/j.enbuild.2022.111954
42. Hoang ML, Carratù M, Paciello V, Pietrosanto A. Body temperature—indoor condition monitor and activity recognition by mems accelerometer based on IoT-alert system for people in quarantine due to COVID-19. Sensors. (2021) 21:2313. doi: 10.3390/s21072313
43. Huang X, Li Q, Wei X, Yang X, Luo D, Zeng H, et al. Indoor test system for liquid CO2 phase change shock wave pressure with PVDF sensors. Sensors. (2020) 20:2395. doi: 10.3390/s20082395
44. Jo J, Jo B, Kim J, Kim S, Han W. Development of an IoT-based indoor air quality monitoring platform. J Sens. (2020) 2020:13–5. doi: 10.1155/2020/8749764
45. Kaliszewski M, Włodarski M, Młyńczak J, Kopczyński K. Comparison of low-cost particulate matter sensors for indoor air monitoring during covid-19 lockdown. Sensors. (2020) 20:1–17. doi: 10.3390/s20247290
46. Kenarkoohi A, Noorimotlagh Z, Falahi S, Amarloei A, Mirzaee SA, Pakzad I, et al. Hospital indoor air quality monitoring for the detection of SARS-CoV-2 (COVID-19) virus. Sci Total Environ. (2020) 748:141324. doi: 10.1016/j.scitotenv.2020.141324
47. Khan MTR, Saad MM, Tariq MA, Akram J, Kim D, SPICE-IT. Smart COVID-19 pandemic controlled eradication over NDN-IoT. Inform Fusion. (2021) 74:50–64. doi: 10.1016/j.inffus.2021.03.005
48. Kim J, Go T, Lee SJ. Volumetric monitoring of airborne particulate matter concentration using smartphone-based digital holographic microscopy and deep learning. J Hazard Mater. (2021) 418:126351. doi: 10.1016/j.jhazmat.2021.126351
49. Ko K, Lee J. yeon, Chung H. Highly efficient colorimetric CO2 sensors for monitoring CO2 leakage from carbon capture and storage sites. Sci Total Environ. (2020) 729:138786. doi: 10.1016/j.scitotenv.2020.138786
50. Ladekar MTech V, Daruwala Professor D. “Indoor Air Quality Monitoring on AWS Using MQTT Protocol; Indoor Air Quality Monitoring on AWS Using MQTT Protocol,” In: 2019 10th International Conference on Computing, Communication and Networking Technologies (ICCCNT). (2019). doi: 10.1109/ICCCNT45670.2019.8944892
51. Li J, Xu J. “Air quality monitoring system for indoor sports venues,” In: IEEE Advanced Information Technology, Electronic and Automation Control Conference (IAEAC). (2021). p. 715–8. doi: 10.1109/IAEAC50856.2021.9390881
52. Marques G, Ferreira CR, Pitarma R. Indoor air quality assessment using a CO2 monitoring system based on internet of things. J Med Syst. (2019) 43:1–10. doi: 10.1007/s10916-019-1184-x
53. Ng DKT, Ho CP, Xu L, Chen W, Fu YH, Zhang T, et al. NDIR CO2 gas sensing using CMOS compatible MEMS ScAlN-based pyroelectric detector. Sens Actuators B Chem. (2021) 346:130437. doi: 10.1016/j.snb.2021.130437
54. Palanisamy D, Ayalur BK. Development and testing of condensate assisted pre-cooling unit for improved indoor air quality in a computer laboratory. Build Environ. (2019) 163:106321. doi: 10.1016/j.buildenv.2019.106321
56. Peng Z, Jimenez JL. Exhaled CO2as a COVID-19 infection risk proxy for different indoor environments and activities. Environ Sci Technol Lett. (2021) 8:392–7. doi: 10.1021/acs.estlett.1c00183
57. Petrovic N, Kocic D. IoT-based System for COVID-19 Indoor Safety Monitoring. IcETRAN 2020. (2020). Available online at: https://www.researchgate.net/publication/343231422_IoT-based_System_for_COVID-19_Indoor_Safety_Monitoring
58. Pietrogrande MC, Casari L, Demaria G, Russo M. Indoor air quality in domestic environments during periods close to italian covid-19 lockdown. Int J Environ Res Public Health. (2021) 18:4060. doi: 10.3390/ijerph18084060
59. Rantas J, Wang D, Jarrard W, Sterchi J, Wang A, Varnosfaderani MP, et al. “A user interface informing medical staff on continuous indoor environmental quality to support patient care and airborne disease mitigation,” In: 2021 IEEE Systems and Information Engineering Design Symposium, SIEDS 2021. Piscataway, NJ: Institute of Electrical and Electronics Engineers Inc. (2021). doi: 10.1109/SIEDS52267.2021.9483774
60. Rivas E, Santiago JL, Martín F, Martilli A. Impact of natural ventilation on exposure to SARS-CoV 2 in indoor/semi-indoor terraces using CO2 concentrations as a proxy. J Build Eng. (2022) 46:103725. doi: 10.1016/j.jobe.2021.103725
61. Stufano A, Lisco S, Bartolomeo N, Marsico A, Lucchese G, Jahantigh H, et al. COVID19 outbreak in Lombardy, Italy: an analysis on the short-term relationship between air pollution, climatic factors and the susceptibility to SARS-CoV-2 infection. Environ Res. (2021) 198:111197. doi: 10.1016/j.envres.2021.111197
62. Vanus J, Kubicek J, Gorjani OM, Koziorek J. Using the IBM SPSS SW tool with wavelet transformation for CO2 prediction within IoT in smart home care. Sensors. (2019) 19:1407. doi: 10.3390/s19061407
63. Wall D, McCullagh P, Cleland I, Bond R. Development of an Internet of Things solution to monitor and analyse indoor air quality. Internet Things. (2021) 14:100392. doi: 10.1016/j.iot.2021.100392
64. Wang J, Huang J, Fu Q, Gao E, Chen J. Metabolism-based ventilation monitoring and control method for COVID-19 risk mitigation in gymnasiums and alike places. Sustain Cities Soc. (2022) 80:103719. doi: 10.1016/j.scs.2022.103719
65. Yang CT, Chen ST Den W, Wang YT, Kristiani E. Implementation of an Intelligent Indoor Environmental Monitoring and management system in cloud. Future Generat Comput Syst. (2019) 96:731–49. doi: 10.1016/j.future.2018.02.041
66. Yang CT, Chen HW, Chang EJ, Kristiani E, Nguyen KLP, Chang JS. Current advances and future challenges of AIoT applications in particulate matters (PM) monitoring and control. J Hazard Mater. (2021) 419:126442. doi: 10.1016/j.jhazmat.2021.126442
67. Environment B and Building and Environment. Available online at: www.elsevier.com/locate/buildenv (accessed Mar 30, 2022).
68. Sensors. Available online at: http://www.mdpi.com/journal/sensors%0A (accessed Mar 30, 2022).
69. Sustainable Cities and Society. Available online at: https://www.journals.elsevier.com/sustainable-cities-and-society (accessed Mar 10, 2022).
70. Environmental Research. Available online at: www.elsevier.com/locate/envres (accessed Mar 10, 2022).
71. Salman N, Kemp AH, Khan A, Noakes CJ. “Real time wireless sensor network (WSN) based Indoor air quality monitoring system,” In: IFAC-PapersOnLine. Amsterdam: Elsevier (2019). p. 324–7. doi: 10.1016/j.ifacol.2019.12.430
72. Kim C, Choi D, Lee YG, Kim K. Diagnosis of indoor air contaminants in a daycare center using a long-term monitoring. Build Environ. (2021) 204:108124. doi: 10.1016/j.buildenv.2021.108124
73. Spena A, Palombi L, Corcione M, Carestia M, Spena VA. On the optimal indoor air conditions for SARS-CoV-2 inactivation. An enthalpy-based approach. Int J Environ Res Public Health. (2020) 17:1–15. doi: 10.3390/ijerph17176083
Keywords: building ventilation, COVID-19, indoor environmental quality, intelligent monitoring, SARS-CoV-2
Citation: Wiryasaputra R, Huang C-Y, Kristiani E, Liu P-Y, Yeh T-K and Yang C-T (2023) Review of an intelligent indoor environment monitoring and management system for COVID-19 risk mitigation. Front. Public Health 10:1022055. doi: 10.3389/fpubh.2022.1022055
Received: 18 August 2022; Accepted: 23 December 2022;
Published: 10 January 2023.
Edited by:
Frank Scholle, North Carolina State University, United StatesReviewed by:
Giacomo Viccione, University of Salerno, ItalyCopyright © 2023 Wiryasaputra, Huang, Kristiani, Liu, Yeh and Yang. This is an open-access article distributed under the terms of the Creative Commons Attribution License (CC BY). The use, distribution or reproduction in other forums is permitted, provided the original author(s) and the copyright owner(s) are credited and that the original publication in this journal is cited, in accordance with accepted academic practice. No use, distribution or reproduction is permitted which does not comply with these terms.
*Correspondence: Ting-Kuang Yeh, tingkuangyeh@gmail.com; Chao-Tung Yang,
ctyang@thu.edu.tw
Disclaimer: All claims expressed in this article are solely those of the authors and do not necessarily represent those of their affiliated organizations, or those of the publisher, the editors and the reviewers. Any product that may be evaluated in this article or claim that may be made by its manufacturer is not guaranteed or endorsed by the publisher.
Research integrity at Frontiers
Learn more about the work of our research integrity team to safeguard the quality of each article we publish.