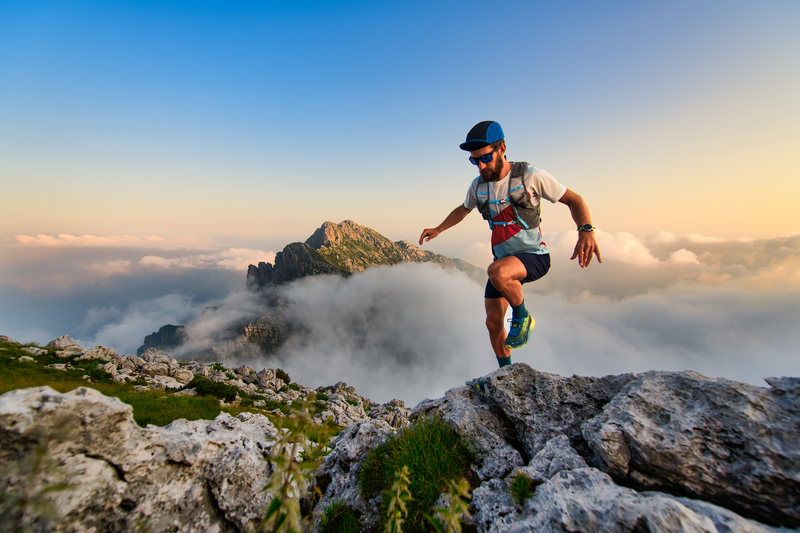
95% of researchers rate our articles as excellent or good
Learn more about the work of our research integrity team to safeguard the quality of each article we publish.
Find out more
BRIEF RESEARCH REPORT article
Front. Psychiatry , 17 March 2025
Sec. ADHD
Volume 16 - 2025 | https://doi.org/10.3389/fpsyt.2025.1466107
Attention-deficit hyperactivity disorder (ADHD) occurs in 5.9% of youth, impacting their health and social conditions often across their lifespan. Currently, early diagnosis is constrained by clinical complexity and limited resources of professionals to conduct evaluations. Scalable methods for ADHD screening are thus needed. Recently, digital epidemiology and biometry, such as the visual, emotional, or digit pathway, have examined brain dysfunction in ADHD individuals. However, whether biometry can support screening for ADHD symptoms using a multimodal tech system is still unknown. The EPIDIA4Kids study aims to create objective measures, i.e., biometrics, that will provide a comprehensive transdiagnostic picture of individuals with ADHD, aligning with current evidence for comorbid presentations. Twenty-four children aged 7 to 12 years performed gamified tasks on an unmodified tablet using the XAI4Kids® multimodal system, which allows extraction of biometrics (eye-, digit-, and emotion-tracking) from video and touch events using machine learning. Neuropsychological assessments and questionnaires were administered to provide ADHD-related measures. Each ADHD-related measure was evaluated with each biometric using linear mixed-effects models. In contrast to neuro-assessments, only two digit-tracking features had age and sex effects (p < 0.001) among the biometrics. Biometric constructs were predictors of working memory (p < 0.0001) and processing speed (p < 0.0001) and, to a lower extent, visuo-spatial skills (p = 0.003), inattention (p = 0.04), or achievement (p = 0.04), where multimodalities are crucial to capture several symptomatology dimensions. These results illustrate the potential of multimodality biometry gathered from a tablet as a viable and scalable transdiagnostic approach for screening ADHD symptomatology and improving accessibility to specialized professionals. Larger populations including clinically diagnosed ADHD will be needed for further validation.
Attention-deficit hyperactivity disorder (ADHD) is one of the most common neurodevelopmental disorders, affecting approximately 5% [95% confidence interval (CI) = 5.0, 5.6] and 7.2% (95% CI = 6.7, 7.8) of children and adolescents across the world (1, 2). This disorder is characterized by developmental impairments of inattention, hyperactivity, or a combination of both. Various genetic and environmental risk factors contribute to ADHD (e.g., prenatal factors and early life adversities) (3), but the pathomechanism of such a condition is not yet deciphered (4).
Youth with ADHD are affected in their daily cognitive, behavioral, and social functions (5) and often require support in and beyond school in many trans-nosographic dimensions such as emotion dysregulation, irritability, and impairment in executive functions (6). Longitudinal studies have shown that persistent symptoms in childhood and adolescence lead to chronic conditions that may persist into adulthood (7). Youth with ADHD have a higher risk for somatic, medical, and psychiatric conditions (4, 6) with a decreased life expectancy of 10 to 15 years compared to the general population (1, 8). Therefore, ADHD has become a public health challenge with important socio-economic burdens to children, families, and the whole society.
The early detection and diagnosis of ADHD is reliable (4) when evaluated by well-trained practitioners following the standard diagnostic criteria (9). Adequate early detection of ADHD and its associated conditions appears crucial to reduce the risk of comorbidities, alter the course of the disorder, and hereby improve several health and well-being outcomes of children and adolescents (10–12). However, access to ADHD evaluation and diagnosis is limited by a lack of consultation time, trained specialists in the current care system (1, 13, 14), and stigmatization for both patients and families (15, 16). A further complementary approach based on technology (17) has emerged to reach screening at a large scale while improving diagnosis accuracy and lowering stigma and cost (18). Objective screening methods are thus increasingly needed; however, they have to be translated into clinical practice after rigorous scientific validation.
Digital epidemiology relies on the data acquired from devices to advance the understanding of health and disorders related the population dynamics (19). In the meantime, lab-scale evidence showed that biometry such as eye-tracking (20–23), digit-tracking (24, 25), or emotion dysregulation (26–28) has at the potential to provide high accuracy classification of ADHD through digital assessments (29). Thus, biometry can measure at once multiple traits (iris, fingerprints, face, retina, hand geometry, and voice) of an individual, which will allow the identification of various dimensions and comorbid presentations as symptoms of ADHD. In contrast to neuroimaging modalities (30, 31) or physiological signals (i.e., electroencephalogram and electrocardiograms) (32, 33), biometry markers or biometrics can easily be used in real-life practice or even remotely during ADHD assessments in children and adolescents. However, very few diagnostic biomarkers for ADHD have been validated clinically (34, 35) based on criteria set by the World Federation of ADHD and the World Federation of Societies of Biological Psychiatry (4). Nevertheless, these results have no attempts to screen for ADHD symptomatology and its comorbidities in real-life practice. An alternative transdiagnostic approach using digital epidemiology may support a better understanding of brain function by providing a screening tool to the general population.
In this paper, we present the preliminary results of the EPIDIA4Kids study (36) based on a multimodality biometry system combining digit-, eye-, and emotion-tracking measurements to examine brain function in children aged 7 to 12 years. Using machine learning, we investigated whether multimodal biometry could serve as an objective measurement for ADHD-related symptomatology in a pediatric population.
EPIDIA4Kids is an observational uncontrolled multi-center study (several cities in France) approved by the Committee for the Protection of Individuals Sud-Est II under the national French register number 2022-A00766-37 and the Commission Nationale de l’Informatique et des Libertés (CNIL). This study was registered in October 2022 on ClinicalTrials.gov under the number NCT05577533. The study is designed to create a brain function normative database relying on digital epidemiology in children aged 7 to 12 years using multimodality biometry (36).
The present study is a preliminary investigation aimed at exploring the feasibility and potential of using multimodal biometry for ADHD symptomatology screening. As such, it is part of a larger ongoing study that aims at recruiting 400 children for a power analysis of 0.8, a confidence level of 0.05, and a type I error, based on three dimensions of executive skills, processing abilities, and processing speed, previously reported in the literature (36). Twenty-four children aged 7 to 12 years were enrolled in the EPIDIA4kids study from March 2023 to December 2023. Details on recruitment and participants’ characteristics were reported previously. Informed consent was obtained from parent/legal representatives with child assent. Children were screened to ensure that they have no parent-reported history of major psychiatric or neurological disorders, brain injury, or other medical conditions that would affect their brain development. Children born prematurely (<32 weeks gestational age) with significant prenatal drug or alcohol exposure were also excluded.
All questionnaires and neuro-assessments are detailed in Table 1. Children performed the digitalized Wechsler Intelligence Scale for Children® Fifth Edition (WISC-V) battery (1.5 hours; 37). Parents or legal representatives answered standardized questionnaires digitally.
XAI4Kids® is a multimodality biometry tool that combines data acquisition and an AI pipeline to model heterogeneous and dynamic data/signals in relation to the target variables. Data were collected from an unmodified touch-screen tablet with an intrinsic camera (video, ~28 frames per second; digit, ~100 Hz) while participants were playing the O-Games battery. In the present paper, the two games, named Rocket and Connect, were included in the analysis. Each game was divided into challenges (11 for Rocket and six for Connect). Time-series data were analyzed for each challenge, which themselves represent a higher-order time series for each game.
Seven emotion features (Table 1) were predicted per frame from video processing using a single neural network that was pre-trained on face identification and fine-tuned for facial expression recognition on static images from AffectNet (38). Four eye-tracking features (Table 1) were extracted with a velocity-threshold identification (I-VT), a method that separates fixation and saccade points based on their point-to-point velocities (39). Using the touch data and kinetic information collected from the tablet, a set of five digit-tracking features (touch events, Table 1) were computed representing the participant’s motor behaviors (40).
Databasing, statistical software, and analysis implementation were programmed in Python using the Pandas library (41). Statistical analysis used the Statsmodel and Pingouin packages (42). Graphs presented in this paper were plotted using the matplotlib (43) and seaborn packages (44). The Holm–Bonferroni sequential correction (45) was used to correct for multiple comparisons (46).
Extracted biometric features (emotion-, digit-, or eye-tracking) were statistically modeled as time series using a moving-average (MA) model that uses past values of the series itself and relies on a series of past errors. Indeed, since biometric features were collected as a function of the behavior of each child and their related performances at the psychometric tasks, the assumption is that the past values of the biometric values have a linear relationship with the current values. Extracted biometric features have a very high-dimensionality vector representing the data for one participant: n features, by 100 bins, for each ci challenge. Biometric features and bins were merged into a single dimension, accounting for a time series, consisting of a vector of n * 100 second-order features per participant.
Principal component analysis (PCA) was then performed on every single dimension to identify for each gamified task the three most informative components that were called “biometric construct” as an objective time-series measurement of biometric features that cannot be directly observed.
To reduce selection bias and improve representativeness and statistical power (overfitting), PCAs were fitted on an internal dataset of 63 children who underwent the same study protocol. The obtained eigenvectors were then applied to biometric constructs of the EPIDIA4Kids cohort to ensure the same dimensionality reduction.
Statistical relationships between biometric constructs and multidimensional assessment measures were examined using linear mixed models that allow for fixed and random effects to account for the effect of each O-Games challenge as well as the effects of biometric constructs on multidimensional assessment measures. O-Games challenges were set as a random effect, and each biometric construct had a fixed effect. Regression model fitting was performed for each ADHD-related symptomatology measure across challenges to account for individual variability in gaming performance. Targeted variables and explanatory variables are listed in Table 1. Parameter estimation was conducted using restricted maximum likelihood (REML). The model’s goodness of fit was assessed using R-squared and log-likelihood metrics. Residual diagnostics confirmed the normality, homoscedasticity, and independence of residuals. The fixed effects of the biometric constructs showed significant predictive power, with effect sizes (Cohen D between 0.4 and 1.8 for each significant PC).
To assess multimodal over unimodal biometry, model performance was examined using the mean absolute error. The multimodal biometric construct model was considered the baseline model and referred to as 1. Values for the model for each unimodal biometric construct (emotion, digit-, or eye-tracking) were calculated as percentage increases relative to the baseline model (Table 2).
Table 2. Multimodal biometry allowed capture of more multidimensional measures of ADHD-related symptomatology.
Twenty-four children (11 girls and 13 boys; mean age 10.55 years ± 1.69) were enrolled in the study as the database in December 2023. No sex difference was observed in socio-economic status or manual laterality (Table 3). Among neuro-assessments and standardized questionnaires, girls scored lower than boys only on the Inattention score of the ADHD scale (p = 0.04; F = 4.7), while boys tended to perform worse on the Cancellation subscale of the WISC-V battery than girls (p = 0.05; F = 4.26). These suggest that the children were well-matched in sex, age, and ADHD-related symptomatology.
Age- and sex-based differences are crucial covariates in brain function and psychopathology research (47). To account for them and minimize further bias, we examined whether these two variables alter biometric measurement. We showed that biometric features exhibited no age or sex effect in both gamified tasks. We found a sex effect only in touch area (p(adjusted for Holm–Bonferroni correction) = 0.001) and touch duration (p(adjusted for Holm–Bonferroni correction) = 0.001), i.e., digit-tracking features, in one of the challenges of the Rocket task and Connect task, respectively. All biometric features were then not adjusted for age and sex in contrast to neuro-assessments and standardized questionnaires.
Sixteen biometric features (Table 1) were collected from video and touchscreen events while children were playing on gamified tasks. They were extracted for the eye-, emotion-, and digit-tracking analyses to capture simultaneously behavioral, emotional, and cognitive states to obtain a multidimensional clinical picture of each child. Since trajectories, i.e., time-series data, reflect better abilities of children than just aggregated point scores, these biometric features were structured as a time-series construct per type of biometry modalities (eye-, emotion-, and digit-tracking) and called biometric constructs.
A PCA with all biometric constructs was performed per gamified task to extract the most informative components, named multimodal biometric construct component (MBC). Effects of the MBC were assessed on neuro-assessments and standardized questionnaires using both linear model and robust linear models (Supplementary Table 1). Both models showed that for Connect, MBC1 was a predictor of Digit span (p < 0.003) and Letter–number sequencing (p = 0.0001–0.04) as measures of the working memory, of Symbol search (p < 0.002), and to a lower extent of Coding (p = 0.02) and Cancellation (p = 0.01–0.04) as measures of processing speed. It was also found that the MBC1 has an effect on the Inattention score (ADHD scale Inattention, p = 0.04) and the Achievement score [Grit Scale for Children and Adults (GSCA); p = 0.04]. A strong effect on Block design (p = 0.003) was found only with the linear model, while MBC3 had an effect only on Social problems (CBCL, p = 0.007) using the robust linear model. Altogether, these results strongly suggest that the multimodal biometric constructs are objective measurements capturing ADHD symptomatology.
Cognitive performance and rating scales, such as the WISC-V (37) alone, cannot lead to an ADHD diagnosis (4, 48), but they are commonly used in clinical practice (49). They can also provide a comprehensive assessment of ADHD-related symptomatology to align with a transdiagnostic approach. Gamified tasks were then designed to trigger several behavioral, cognitive, and emotional states in a reduced time. Using linear model regression (Table 4), it was found that the Connect task allowed predictions for working memory scores (p ≤ 0.001), for Symbol search (p < 0.0001), and “at lower extent” for Inattention (p = 0.04) and Achievement (p = 0.04) abilities. The Rocket task allowed specific predictions for processing speed as measured by Coding and Cancellation (p ≤ 0.001), Manual laterality [Edinburgh Handedness Inventory (EHI); p = 0.002], and Vocabulary (p = 0.003). Independently of the gamified tasks, each MBC1 predicted only Block design (p ≤ 0.003) that reflects visuo-spatial reasoning. These indicated that biometric constructs could predict several neuropsychopathological measures related to ADHD symptomatology that were triggered by the proprieties of each gamified task.
Table 4. Linear Model regression for significance of each multimodal biometric construct component (MBC) on neuro-assessments and standardized questionnaires for both Rocket and Connect gamified tasks.
The goal is to achieve the optimal biometric construct model to predict a multidimensional screening for ADHD-related symptomatology in children and adolescents. The mean absolute error (MAE) is a critical indicator for comparing the performance of each biometric model. Table 2 presents a comparative analysis of performances between the multimodal and unimodal biometric models in which the multimodal model was set as the baseline model.
First, a multimodal biometric model could enhance performance to predict each of the neuropsychopathological measures but not systematically. In the Rocket task, unimodal models for digit- and eye-tracking had similar performances to multimodal biometric models independently of the neuropsychopathological measures, while the unimodal model for emotion (−13% to 0%) had systematically equal or better performance than multimodal biometric model for each measure. In the Connect task, the multimodal biometric model had better performance overall than the unimodal digit-tracking model (−1% to 3%) but worse performances over the unimodal model for emotion (−9% to 3%) and eye-tracking (−4% to 3%) dependently to the neuropsychopathological measures.
Altogether, these results suggest that multimodal biometry is required for capturing multidimensional measures of ADHD-related symptomatology in children and adolescents. However, the performance of multimodal biometric models can be lower than that obtained from unimodal biometric models.
These preliminary results from the EPIDIA4Kids study showed that multimodality biometry provides insights into brain function in children aged 7 to 12 years old. Biometrics may ultimately serve as a viable candidate for an objective measurement to screen for multidimensional ADHD symptomatology in children.
ADHD has a complex clinical presentation whose symptoms are highly variable including age and sex. Indeed, sex- and gender-specific differences in health exist largely due to genetic and hormonal influences of biological sex, hereby influencing physiology and disease and thus biometrics. Here, we showed that most biometric features extracted using machine learning models are not influenced by age and sex during this period of age. Further validation will be needed on a larger cohort to examine whether this statement stands. In line with previous research (24, 50), touch duration and touch area were correlated with age, suggesting that biometric constructs have a higher potential to assess neurodevelopmental symptomatology while accounting for fewer cofounders than the current assessments within this age range (neuropsychological tests or clinical questionnaires).
Furthermore, we found that biometric constructs predict performances of specific tasks that were shown mainly impaired in children and adolescents with ADHD, i.e., working memory (51, 52) and processing speed (53, 54). More specifically, several studies have reported that ADHD children have lower scores on working memory and processing speed indexes among the whole subtest battery of the WISC using its different versions (55). Additionally, we showed a specific prediction of the biometric construct for the Digit span subtest: performances were lower in ADHD children compared to healthy children (55). Interestingly, working memory was predicted by biometric construct mainly with the Connect task and processing speed with the Rocket task, while visuo-spatial abilities were predicted specifically in both gamified tasks. Verbal comprehension and fluid reasoning were not predicted by any of the biometric constructs. These findings suggest that biometric constructs have potential value as biomarkers for ADHD-related symptomatology where the design of gamified tasks plays a crucial role in triggering specific brain functions. However, predictions for subtest scores and indexes should be interpreted with caution since they assess symptoms at one time-point rather than clinical deficits, and they cannot reflect causality but correlations. For instance, we found that a biometric construct predicts processing speed and the Vocabulary subtest in the same Rocket task. Since verbal comprehension is highly correlated with processing speed in ADHD children (56), we cannot rule out that prediction for processing speed in Rocket tasks is not mediated by deficits in vocabulary performance. Altogether, biometric constructs can serve as a screening method at a large scale in children, but longitudinal studies will be needed to understand clinical causality among those biometric construct predictions.
Finally, we showed that multimodality biometry is required to capture multi-dimensional measurements of ADHD symptomatology in a limited time. Previously, eye-tracking features were reported to provide information on higher-order brain processes including memory and attention in ADHD individuals as measured by changes in gaze fixation, saccadic movements (23), or longer reaction times and errors in the direction of anti-saccadic movements (21). Digit-tracking using touchscreen events such as touch duration and the number of clicks was described as a reliable tool for quantifying attention (25) or visual motor skills (24) in ADHD children. Likewise, evidence revealed that facial emotion recognition can be used in the evaluation of children with ADHD (26–28). However, to our knowledge, none has attempted to examine whether these biometric features all collected from an unmodified tablet can serve as objective measures for a multidimensional assessment of ADHD-related symptomatology.
We found that multimodal biometric measures predicted specifically working memory and processing speed abilities that are mainly impaired in ADHD children. We should point out that unimodal biometric models outperformed sometimes the multimodal models for a specific psychopathological score. However, multimodality is required to obtain a comprehensive multidimensional picture of children. Biometric feature identification and selection will help optimize the prediction of multidimensional psychopathological measurements, but they are out of the scope of the present study.
The preliminary results represent thus a notable step toward objective measurements based on multimodality biometry for screening multiple dimensions of ADHD symptomatology in children. However, most participants were healthy children with no history of neurological disorders. The preliminary aim of the present study was to first test our hypothesis on the potential of multimodality biometry as a viable and scalable transdiagnostic approach for screening ADHD symptomatology. A strong tension exists between balancing the desire to minimize heterogeneity (“noise”), which can mask the effect of interest, and the desire to generate data that are generalizable to a broader population. We chose to narrow eligibility criteria to limit the variability in a study population and control for confounding factors. Narrowing eligibility criteria will most probably diminish the understanding and applicability of the findings in real-world ADHD populations, but this step is crucial to move forward. Increasing the number of participants diagnosed with ADHD is required to establish generalizability with rigorous clinical validation.
As a secondary judgment criterion, multimodality approaches bring high potential for improving access to trained specialists as well as diagnosis support at cost-efficiency (57–59). Recent machine learning techniques could handle heterogeneous data from multimodal sources for prediction (60–62), but combining data from multiple sources can hurt model performance (63) and increase the risk of incurring biases (64). Given this, we applied PCA technology, which appears the appropriate approach to minimize data dimensionality, thereby stabilizing subsequent modeling (65) to mitigate biases.
Another limitation is to account for the inherent complexity of health data that encompass many domains (social, biological, environmental, and genetic) that influence health. Multimodal machine learning can leverage different types of data and find patterns in and between modalities to improve prediction performance (66) using transformer architectures. However, training of supervised machine learning model relied on existing samples, and no database with images of children with ADHD is available. A promising aspect of transformers is the ability to learn meaningful representation with unlabeled data, which is paramount in biomedical artificial intelligence given the limited and expensive resources needed to obtain high-quality labels. Diagnostic testing tools such as those based on digital technology, i.e., multimodal biometry, would then provide for global mental and medical states of individuals in a shorter time but will not replace the professionals’ experience and observation time (29, 67). Such scales or tools are complementary to them, as they will be helpful in diagnosing and predicting the full spectrum of ADHD symptoms by accelerating and improving medical information and history retrievals. Knowledge of clinical expertise and technologies is required to gather clinical evidence for such digital tools. Diagnosis of ADHD will stay primarily a clinical one where clinicians/professionals are at the core of the whole process. However, there is a shortage of medical professionals in which 40% of medical doctors are retiring or will retire by 2030. Therefore, remote and digital assessments would be valuable tools in routine care, but it is essential to account for patients and relatives who are digitally disadvantaged and cannot access remote technologies (68). Low- and middle-income families or households have often lower digital literacy and are less likely to access digital psychiatric services (69). To minimize such social inequities, the XAI4Kids® system was developed for ADHD assessments at a large scale in a natural setting without the need for additional cost-limiting equipment.
Altogether, evidence strongly suggests that the XAI4Kids® system combining biometric measures and transdiagnostic approaches may provide compelling alternatives in capturing the multiple dimensions of ADHD symptomatology in the pediatric population and at a large scale. Multimodality data and modeling have the goal of addressing urgent issues such as easing access to child specialists due to limited resources.
Future work focuses on conducting a comprehensive and rigorous validation with clinically diagnosed ADHD participants to ensure reliability in clinical settings but also apprehend differences in ADHD presentation and comorbidities. Lastly, the integration of XAI4Kids® and its efficiency along the existing clinical workflow will be examined to tailor screening and diagnosis in primary care.
The datasets presented in this article are not readily available because it was not included in the ethics approval to submit the datasets.
The appropriate ethics committee (Comité de Protection des Personnes Sud-Est II) approved the protocol, under the reference 2022-A00766-37 in compliance with the French law “Jardé” (n°2012-300 of March 5th, 2012) after reviewing the informed consent forms and all information notice dedicated to participants. The participants have provided their written informed consent to participate in this study. The study is conducted in accordance with the principles of the Declaration of Helsinki (1964) as reviewed in Edinburgh (2000). Data collection and processing is complying with General Data Protection Regulation (GDPR). The studies were conducted in accordance with the local legislation and institutional requirements. Written informed consent for participation in this study was provided by the participants’ legal guardians/next of kin.
YG: Writing – review & editing, Data curation, Formal Analysis, Visualization. AH: Data curation, Formal Analysis, Writing – review & editing. TM: Data curation, Writing – review & editing. MC: Data curation, Writing – review & editing. LP: Methodology, Project administration, Validation, Writing – review & editing. PS: Conceptualization, Investigation, Methodology, Supervision, Validation, Writing – review & editing. VDV: Writing – review & editing, Conceptualization, Methodology, Project administration, Investigation, Supervision.
The author(s) declare that financial support was received for the research and/or publication of this article. The study was funded partially by the Bank of Innovation (grant: DOS0180634/00) to O-Kidia. The funding institution had no role in study design, data collection, data analysis and interpretation, writing the manuscripts, or the decision about publication.
We thank the caregivers, parents, and children for their participation in the study without which this research would not have been possible. Special thanks to the Fuso France non-profit organization. We gratefully acknowledge the collaboration of physicians in Côte d’Azur University and Centre Hospitalier de Nice as well as several clinical research specialists.
AH, YG, TM, MC, and LP are employees of O-Kidia, and VDV has stock and ownership interest in O-Kidia. As part of the study, AH, YG, TM, MC, and LP report to the Principal Investigator/Investigator of the present study, VDV, under the supervision of the Comité de Protection des Personnes Sud-Est II CPP 69.
The remaining author declares that the research was conducted in the absence of any commercial or financial relationships that could be construed as a potential conflict of interest.
All claims expressed in this article are solely those of the authors and do not necessarily represent those of their affiliated organizations, or those of the publisher, the editors and the reviewers. Any product that may be evaluated in this article, or claim that may be made by its manufacturer, is not guaranteed or endorsed by the publisher.
The Supplementary Material for this article can be found online at: https://www.frontiersin.org/articles/10.3389/fpsyt.2025.1466107/full#supplementary-material
1. Cortese S, Song M, Farhat LC, Yon DK, Lee SW, Kim MS, et al. Incidence, prevalence, and global burden of ADHD from 1990 to 2019 across 204 countries: data, with critical re-analysis, from the global burden of disease study. Mol Psychiatry. (2023) 28:4823–30. doi: 10.1038/s41380-023-02228-3
2. Francés L, Ruiz A, Virgínia Soler C, Francés J, Caules J, Hervás A, et al. Prevalence, comorbidities, and profiles of neurodevelopmental disorders according to the DSM-5-TR in children aged 6 years old in a european region. Front Psychiatry. (2023) 14:1260747. doi: 10.3389/fpsyt.2023.1260747
3. Faraone SV, Asherson P, Banaschewski T, Biederman J, Buitelaar JK, Ramos-Quiroga JA, et al. Attention-deficit/hyperactivity disorder. Nat Rev Dis Primers. (2015) 1:150205. doi: 10.1038/nrdp.2015.20
4. Faraone SV, Banaschewski T, Coghill D, Zheng Y, Biederman J, Bellgrove MA, et al. The world federation of ADHD international consensus statement: 208 evidence-based conclusions about the disorder. Neurosci Biobehav Rev. (2021) 128:789–818. doi: 10.1016/j.neubiorev.2021.01.022
5. Usami M. Functional consequences of attention-deficit hyperactivity disorder on children and their families. Psychiatry Clin Neurosci. (2016) 70(8):303–17. doi: 10.1111/pcn.12393
6. Franke B, Michelini G, Asherson P, Banaschewski T, Bilbow A, Buitelaar JK, et al. Live fast, die young? A review on the developmental trajectories of ADHD across the lifespan. Eur Neuropsychopharmacol. (2018) 28(10):1059–88. doi: 10.1016/j.euroneuro.2018.08.001
7. Meter ARV, Sibley MH, Vandana P, Birmaher B, Fristad MA, Horwitz S, et al. The stability and persistence of symptoms in childhood-onset ADHD. Eur Child Adolesc Psychiatry. (2024) 33(4):1163–70. doi: 10.1007/s00787-023-02235-3
8. Cherkasova MV, Roy A, Molina BSG, Scott G, Weiss G, Barkley RA, et al. Review: adult outcome as seen through controlled prospective follow-up studies of children with attention-deficit/hyperactivity disorder followed into adulthood. J Am Acad Child Adolesc Psychiatry. (2022) 61(3):378–91. doi: 10.1016/j.jaac.2021.05.019
9. American Psychiatric Association. Diagnostic and statistical manual of mental disorders. Fifth Edition, Text Revision. Washington, DC: American Psychiatric Association (2022).
10. Coghill D, Banaschewski T, Cortese S, Asherson P, Brandeis D, Buitelaar J, et al. The management of ADHD in children and adolescents: bringing evidence to the clinic: perspective from the european ADHD guidelines group (EAGG). Eur Child Adolesc Psychiatry. (2021) 32(8):1337–61. doi: 10.1007/s00787-021-01871-x
11. Daley D, Oord Svd, Ferrin M, Cortese S, Danckaerts M, Doepfner M, et al. Practitioner review: current best practice in the use of parent training and other behavioural interventions in the treatment of children and adolescents with attention deficit hyperactivity disorder. J Child Psychol Psychiatry Allied Disciplines. (2017) 59(9):932–47. doi: 10.1111/jcpp.12825
12. National Collaborating Centre for Mental Health. Attention deficit hyperactivity disorder: diagnosis and management. Nice guideline no 87. Natl Institute Health Clin Excellence. (2018).
13. Willig TN, Dajon M, Assathiany R, Brun L, Fourneret P, Massé M, et al. Healthcare pathways and practitioners’ Knowledge about ADHD in children. Encephale. (2023) 50(4):363–72. doi: 10.1016/j.encep.2023.07.005
14. Sapiets SJ, Hastings RP, Totsika V. Predictors of access to early support in families of children with suspected or diagnosed developmental disabilities in the United Kingdom. J Autism Dev Disord. (2024) 54(4):1628–41. doi: 10.1007/s10803-023-05996-7
15. Chang CC, Chen YM, Hsiao RC, Chou WJ, Yen CF. Did affiliate stigma predict affective and behavioral outcomes in caregivers and their children with attention-deficit/hyperactivity disorder? Int J Environ Res Public Health. (2021) 18(14):7532. doi: 10.3390/ijerph18147532
16. Leitch S, Sciberras E, Post B, Gerner B, Rinehart N, Nicholson JM, et al. Experience of stress in parents of children with ADHD: A qualitative study. Int J Qual Stud Health Well-Being. (2019) 14(1):1690091. doi: 10.1080/17482631.2019.1690091
17. Previously Marzena Szkodo MOR, Micai M, Caruso A, Fulceri F, Fazio M, Scattoni ML. Technologies to support the diagnosis and/or treatment of neurodevelopmental disorders: A systematic review. Neurosci Biobehav Rev. (2023) 145:105021. doi: 10.1016/j.neubiorev.2022.105021
18. Valentine AZ, Brown BJ, Groom MJ, Young E, Hollis C, Hall CL. A systematic review evaluating the implementation of technologies to assess, monitor and treat neurodevelopmental disorders: A map of the current evidence. Clin Psychol Rev. (2020) 80:101870. doi: 10.1016/j.cpr.2020.101870
19. Salathé M. Digital epidemiology: what is it, and where is it going? Life Sciences Soc Policy. (2018) 14(1):1. doi: 10.1186/s40504-017-0065-7
20. Das W, Khanna S. A robust machine learning based framework for the automated detection of ADHD using pupillometric biomarkers and time series analysis. Sci Rep. (2021) 11(1):16370. doi: 10.1038/s41598-021-95673-5
21. Caldani S, Razuk M, Septier M, Barela JA, Delorme R, Acquaviva E, et al. The effect of dual task on attentional performance in children with ADHD. Front Integr Neurosci. (2019) 12:67. doi: 10.3389/fnint.2018.00067
22. Liu Z, Li J, Zhang Y, Wu D, Huo Y, Yang J, et al. Auxiliary diagnosis of children with attention-deficit/hyperactivity disorder using eye-tracking and digital biomarkers: case-control study. JMIR MHealth UHealth. (2024) 12:e58927. doi: 10.2196/58927
23. Fernandez-Ruiz J, Schwerdtfeger RMH, Alahyane N, Brien DC, Coe BC, Munoz DP. Dorsolateral prefrontal cortex hyperactivity during inhibitory control in children with ADHD in the antisaccade task. Brain Imaging Behav. (2020) 14(6):2450–63. doi: 10.1007/s11682-019-00196-3
24. Perochon S, Martino JMD, Carpenter KLH, Compton S, Davis N, Espinosa S, et al. A tablet-based game for the assessment of visual motor skills in autistic children. NPJ Digital Med. (2023) 6(1):17. doi: 10.1038/s41746-023-00762-6
25. Teruel MA, Sanchis J, Ruiz-Robledillo N, Albaladejo-Blázquez N, Ferrer-Cascales R, Trujillo J. Measuring attention of ADHD patients by means of a computer game featuring biometrical data gathering. Heliyon. (2024) 10(5):e26555. doi: 10.1016/j.heliyon.2024.e26555
26. Yildirim Demİrdöğen E, Turan B, Gülşen M, Çubukçu HC, Dursun OB. Voluntary imitation of dynamic facial expressions in attention deficit hyperactivity disorder: A facial-Behavior analysis. J Clin Exp Neuropsychol. (2023) 45:915–275. doi: 10.1080/13803395.2024.2320464
27. Kühle HJ, Kinkelbur J, Andes K, Heidorn FM, Zeyer S, Rautzenberg P, et al. Self-Regulation of visual attention and facial expression of emotions in ADHD children. J Attention Disord. (2007) 10:350–585. doi: 10.1177/1087054706289944
28. Staff AI, Luman M, Oord Svd, Bergwerff CE, Hoofdakker BJvd, Oosterlaan J. Facial emotion recognition impairment predicts social and emotional problems in children with (Subthreshold) ADHD. Eur Child Adolesc Psychiatry. (2022) 31:715–275. doi: 10.1007/s00787-020-01709-y
29. Cibrian FL, Monteiro EM, Lakes KD. Digital assessments for children and adolescents with ADHD: A scoping review. Front Digital Health. (2024) 6:1440701. doi: 10.3389/FDGTH.2024.1440701
30. Bohland JW, Saperstein S, Pereira F, Rapin J, Grady L. Network, anatomical, and non-imaging measures for the prediction of ADHD diagnosis in individual subjects. Front Syst Neurosci. (2012) 6:78/ABSTRACT. doi: 10.3389/FNSYS.2012.00078/ABSTRACT
31. Tang Y, Sun J, Wang C, Zhong Y, Jiang A, Liu G, et al. ADHD classification using auto-encoding neural network and binary hypothesis testing. Artif Intell Med. (2022) 123:102209. doi: 10.1016/J.ARTMED.2021.102209
32. Öztoprak H, Toycan M, Alp YK, Arıkan O, Doğutepe E, Karakaş S. Machine-Based classification of ADHD and nonADHD participants using time/Frequency features of event-Related neuroelectric activity. Clin Neurophysiol. (2017) 128:2400–24105. doi: 10.1016/J.CLINPH.2017.09.105
33. Koh JEW, Ooi CP, Lim-Ashworth NSJ, Vicnesh J, Tor HT, Lih OS, et al. Automated classification of attention deficit hyperactivity disorder and conduct disorder using entropy features with ECG signals. Comput Biol Med. (2022) 140:105120. doi: 10.1016/J.COMPBIOMED.2021.105120
34. Cortese S, Solmi M, Michelini G, Bellato A, Blanner C, Canozzi A, et al. Candidate diagnostic biomarkers for neurodevelopmental disorders in children and adolescents: A systematic review. World Psychiatry. (2023) 22:129–49. doi: 10.1002/wps.21037
35. Parlatini V, Bellato A, Gabellone A, Margari L, Marzulli L, Matera E, et al. A state-of-the-art overview of candidate diagnostic biomarkers for attention-deficit/hyperactivity disorder (ADHD). Expert Rev Mol Diagnostics. (2024) 24:259–715. doi: 10.1080/14737159.2024.2333277
36. Douet Vannucci V, Marchand T, Hennequin A, Caci H, Staccini P. The EPIDIA4Kids protocol for a digital epidemiology study on brain functioning in children, based on a multimodality biometry tool running on an unmodified tablet. Front Public Health. (2023) 11:1185565. doi: 10.3389/fpubh.2023.1185565
37. The Wechsler Intelligence Scale for Children–Fifth Edition Integrated. In Flanagan DP, McDonough EM. Eds. Contemporary intellectual assessment: Theories, tests, and issues. 4th ed. The Guilford Press. (2014) 303–32.
38. Savchenko AV, Savchenko LV, Makarov I. Classifying emotions and engagement in online learning based on a single facial expression recognition neural network. IEEE Trans Affect Computing. (2022) 13(4):2132–43. doi: 10.1109/TAFFC.2022.3188390
39. Salvucci DD, Goldberg JH. Identifying fixations and saccades in eye-tracking protocols. Eye Tracking Research & Application (2000) 71–78. doi: 10.1145/355017.355028
40. Anzulewicz A, Sobota K, Delafield-Butt JT. Toward the autism motor signature: gesture patterns during smart tablet gameplay identify children with autism. Sci Rep. (2016) 6:31107. doi: 10.1038/srep31107
41. Mckinney W. Data structures for statistical computing in python. Proceedings of the 9th Python in Science Conference. Austin. (2010). doi: 10.25080/Majora-92bf1922-00a
42. Vallat R. Pingouin: statistics in python. J Open Source Software. (2018) 3:1026. doi: 10.21105/joss.01026
43. Hunter JD. Matplotlib: A 2D graphics environment. In. Computing Sci Eng. (2007) 9:90–5. doi: 10.1109/MCSE.2007.55
44. Waskom ML. Seaborn: statistical data visualization. J Open Source Software. (2021) 6:3021. doi: 10.21105/joss.03021
45. Holm S. A simple sequentially rejective multiple test procedure. Scandinavian J Stat. (1979) 6:65–70.
46. Aickin M, Gensler H. Adjusting for multiple testing when reporting research results: the bonferroni vs holm methods. Am J Public Health. (1996) 86:726–28. doi: 10.2105/AJPH.86.5.726
47. Hyatt CS, Owens MM, Crowe ML, Carter NT, Lynam DR, Miller JD. The quandary of covarying: A brief review and empirical examination of covariate use in structural neuroimaging studies on psychological variables. NeuroImage. (2020) 205:116225. doi: 10.1016/j.neuroimage.2019.116225
48. Aiello R, Ruble L, Esler A. National study of school psychologists’ Use of evidence-based assessment in autism spectrum disorder. J Appl School Psychol. (2017) 33(1):67–88. doi: 10.1080/15377903.2016.1236307
49. Wilson AC. Cognitive profile in autism and ADHD: A meta-analysis of performance on the WAIS-IV and WISC-V. Arch Clin Neuropsychol. (2024) 39(4):498–515. doi: 10.1093/arclin/acad073
50. Lio G, Fadda R, Doneddu G, Duhamel JR, Sirigu A. Digit-tracking as a new tactile interface for visual perception analysis. Nat Commun. (2019) 10(1):5392. doi: 10.1038/s41467-019-13285-0
51. Kasper LJ, Alderson RM, Hudec KL. Moderators of working memory deficits in children with attention-Deficit/Hyperactivity disorder (ADHD): A meta-Analytic review. Clin Psychol Review. (2012) 32(7):605–17. doi: 10.1016/j.cpr.2012.07.001
52. Martinussen R, Hayden J, Hogg-Johnson S, Tannock R. A meta-analysis of working memory impairments in children with attention-deficit/hyperactivity disorder. J Am Acad Child Adolesc Psychiatry. (2005) 44(4):377–84. doi: 10.1097/01.chi.0000153228.72591.73
53. Salum GA, Sergeant J, Sonuga-Barke E, Vandekerckhove J, Gadelha A, Pan PM, et al. Specificity of basic information processing and inhibitory control in attention deficit hyperactivity disorder. Psychol Med. (2014) 44(3):617–31. doi: 10.1017/S0033291713000639
54. Shanahan MA, Pennington BF, Yerys BE, Scott A, Boada R, Willcutt EG, et al. Processing speed deficits in attention deficit/hyperactivity disorder and reading disability. J Abnormal Child Psychol. (2006) 34(5):585–602. doi: 10.1007/s10802-006-9037-8
55. Becker A, Christiane B, Maurer J, Daseking M, Pauls F. Measurement invariance of the WISC-V across a clinical sample of children and adolescents with ADHD and a matched control group. J Intell. (2024) 12(1):6. doi: 10.3390/jintelligence12010006
56. Pineda DA, Puerta IC, Aguirre DC, García-Barrera MA, Kamphaus RW. The role of neuropsychologic tests in the diagnosis of attention deficit hyperactivity disorder. Pediatr Neurol. (2007) 36(6):373–81. doi: 10.1016/j.pediatrneurol.2007.02.002
57. Kingston D, Austin MP, Veldhuyzen van Zanten S, Harvalik P, Giallo R, McDonald SD, et al. Pregnant Women’s Views on the Feasibility and Acceptability of Web-Based Mental Health e-Screening versus Paper-Based Screening: A Randomized Controlled Trial. J Med Internet Res. (2017) 19(4):e88. doi: 10.2196/jmir.6866
58. Ness SL, Bangerter A, Manyakov NV, Lewin D, Boice M, Skalkin A, et al. An observational study with the janssen autism knowledge engine (JAKE®) in individuals with autism spectrum disorder. Front Neurosci. (2019) 13:111. doi: 10.3389/fnins.2019.00111
59. Volzhenin K, Changeux JP, Dumas G. Multilevel development of cognitive abilities in an artificial neural network. Proc Natl Acad Sci United States America. (2022) 119(39):e2201304119. doi: 10.1073/pnas.2201304119
60. Tornero-Costa R, Martinez-Millana A, Azzopardi-Muscat N, Lazeri L, Traver V, Novillo-Ortiz D. Methodological and quality flaws in the use of artificial intelligence in mental health research: systematic review. JMIR Ment Health. (2023) 10:e42045. doi: 10.2196/42045
61. Mhasawade V, Zhao Y, Chunara R. Machine learning and algorithmic fairness in public and population health. Nat Mach Intelligence. (2021) 3:659–66. doi: 10.1038/s42256-021-00373-4
62. Bowe AK, Lightbody G, Staines A, Murray DM. Big data, machine learning, and population health: predicting cognitive outcomes in childhood. Pediatr Res. (2023) 93(2):300–7. doi: 10.1038/s41390-022-02137-1
63. Berisha V, Krantsevich C, Richard Hahn P, Hahn S, Dasarathy G, Turaga P, et al. Digital medicine and the curse of dimensionality. Npj digital medicine. Nat Res. (2021) 4:153. doi: 10.1038/s41746-021-00521-5
64. Vokinger KN, Feuerriegel S, Kesselheim AS. Mitigating bias in machine learning for medicine. Commun Med. (2021) 1:25. doi: 10.1038/s43856-021-00028-w
65. Acosta JN, Falcone GJ, Rajpurkar P, Topol EJ. Multimodal biomedical AI. Nat Med. (2022) 28:1773–84. doi: 10.1038/s41591-022-01981-2
66. Akbari H, Yuan L, Qian R, Chuang W, Chang S, et al. VATT: transformers for multimodal self-supervised learning from raw video, audio and text. ArXiv. (2021) doi: 10.48550/arXiv.2104.11178
67. Loh HW, Ooi CP, Barua PD, Palmer EE, Molinari F, Acharya UR. Automated detection of ADHD: current trends and future perspective. Comput Biol Med. (2022) 146:105525. doi: 10.1016/J.COMPBIOMED.2022.105525
68. Santosh P, Cortese S, Hollis C, Bölte S, Daley D, Coghill D, et al. Remote assessment of ADHD in children and adolescents: recommendations from the european ADHD guidelines group following the clinical experience during the COVID-19 pandemic. Eur Child Adolesc Psychiatry. (2023) 32(6):921–35. doi: 10.1007/s00787-023-02148-1
Keywords: digital epidemiology, multidimensional assessment, biometry, multimodality, child development, ADHD, Cognitive and behavioral performances
Citation: Guigou Y, Hennequin A, Marchand T, Chebli M, Pisella LI, Staccini P and Douet Vannucci V (2025) Preliminary results of the EPIDIA4Kids study on brain function in children: multidimensional ADHD-related symptomatology screening using multimodality biometry. Front. Psychiatry 16:1466107. doi: 10.3389/fpsyt.2025.1466107
Received: 17 September 2024; Accepted: 18 February 2025;
Published: 17 March 2025.
Edited by:
Jeff Bolles, Francis Marion University, United StatesReviewed by:
Larry R. Price, Texas State University, United StatesCopyright © 2025 Guigou, Hennequin, Marchand, Chebli, Pisella, Staccini and Douet Vannucci. This is an open-access article distributed under the terms of the Creative Commons Attribution License (CC BY). The use, distribution or reproduction in other forums is permitted, provided the original author(s) and the copyright owner(s) are credited and that the original publication in this journal is cited, in accordance with accepted academic practice. No use, distribution or reproduction is permitted which does not comply with these terms.
*Correspondence: Vanessa Douet Vannucci, dmFuZXNzYS5kb3VldEBvLWtpZGlhLmNvbQ==
†These authors contributed equally to this work and share first authorship
Disclaimer: All claims expressed in this article are solely those of the authors and do not necessarily represent those of their affiliated organizations, or those of the publisher, the editors and the reviewers. Any product that may be evaluated in this article or claim that may be made by its manufacturer is not guaranteed or endorsed by the publisher.
Research integrity at Frontiers
Learn more about the work of our research integrity team to safeguard the quality of each article we publish.