- 1Department of Mental Health, Salerno, Italy
- 2European Biomedical Research Institute of Salerno (EBRIS), Salerno, Italy
- 3Dipartimento di Scienze Aziendali—Managment e Innovation Systems, Università di Salerno, Salerno, Italy
- 4Innovation Tecnology e Sviluppo (I.T. Svil), Salerno, Italy
- 5Department of Pediatrics, Yonsei University College of Medicine, Seoul, Republic of Korea
- 6Severance Underwood Meta-Research Center, Institute of Convergence Science, Yonsei University, Seoul, Republic of Korea
- 7Department of Psychiatry, University of Ottawa, Ontario, ON, Canada
- 8On Track: The Champlain First Episode Psychosis Program, Department of Mental Health, The Ottawa Hospital, Ontario, ON, Canada
- 9Clinical Epidemiology Program, Ottawa Hospital Research Institute, University of Ottawa, Ottawa, ON, Canada
- 10School of Epidemiology and Public Health, Faculty of Medicine, University of Ottawa, Ottawa, ON, Canada
- 11Department of Child and Adolescent Psychiatry, Charité—Universitätsmedizin, Berlin, Germany
Introduction: Depression is the leading cause of worldwide disability, until now only 3% of patients with major depressive disorder (MDD) experiences full recovery or remission. Different studies have tried to better understand MDD pathophysiology and its resistant forms (TRD), focusing on the identification of candidate biomarkers that would be able to reflect the patients’ state and the effects of therapy. Development of digital technologies can generate useful digital biomarkers in a real-world setting. This review aims to focus on the use of digital technologies measuring symptom severity and predicting treatment outcomes for individuals with mood disorders.
Methods: Two databases (PubMed and APA PsycINFO) were searched to retrieve papers published from January 1, 2013, to July 30, 2023, on the use of digital devices in persons with MDD. All papers had to meet specific inclusion criteria, which resulted in the inclusion of 12 articles.
Results: Research on digital biomarkers confronts four core aspects: (I) predicting diagnostic status, (II) assessing symptom severity and progression, (III) identifying treatment response and (IV) monitoring real-word and ecological validity. Different wearable technologies have been applied to collect physiological, activity/sleep, or subjective data to explore their relationships with depression.
Discussion: Depression’s stable rates and high relapse risk necessitate innovative approaches. Wearable devices hold promise for continuous monitoring and data collection in real world setting.
Conclusion: More studies are needed to translate these digital biomarkers into actionable interventions to improve depression diagnosis, monitoring and management. Future challenges will be the applications of wearable devices routinely in personalized medicine.
Highlights
• Digital biomarkers show promise in predicting and assessing mood disorders.
• Smartphone data aids in tracking depression severity and treatment responses.
• Wearable devices enhance real-world monitoring of mood disorders.
• Artificial intelligence advances offer new diagnostic and therapeutic possibilities.
• Integration of technology improves major depressive disorder (MDD) diagnosis and personalized treatment.
1 Introduction
Globally, depression is estimated to affect 300 million individuals and is the leading cause of disability worldwide (1) and will become the leading cause of disability globally by 2030 (1). The prevalence of depressive disorders is highest among young adults aged 18 years (2–4). Onset during adolescence poses a particularly elevated risk of recurrence and long-term impairment in real-life functioning (5, 6). Despite increased accessibility of treatment over the last four decades prevalence rates have remained static (7). Even the best available treatments are largely unsuccessful at producing lasting outcomes, as approximately 40%–50% of patients relapse within 1–2 years of receiving treatment (8, 9). Symptoms of depression may manifest on multiple levels, including subjective emotional, cognitive, behavioral, and physical. In the depression field there is a strong need for monitoring clinical evolution and treatment responses in a more efficient manner to identify the treatment-resistant depression (TRD) forms. TRD is a condition characterized by persistent or recurrent depressive symptoms despite adequate treatment with one or more antidepressant medications (10). Approximately one-third of individuals with major depressive disorder (MDD) do not achieve full remission of symptoms even after trying two suitable trials of antidepressants without adequate response (11, 12). Despite its medical importance MDD is poorly defined and diagnosed since its diagnosis is mostly based on data subjectively reported by the patients themselves (13–15) and lack of objective, clinically relevant outcome measure. It is still debated whether mental disorders should be conceptualized as discrete entities (categorical approach) such as DSM or ICD or as phenomena along a continuum of severity (dimensional approach). The US National Institute of Mental Health proposed a new approach for research on mental disorders, the research domain criteria (RDoC) (16), a project aimed at re-orienting research on etiology and pathophysiology of psychopathological phenomena from category-based to dimension-based and at incorporating genetics, neuroimaging, and cognitive features into diagnostic schemes. The focus of research in mood disorders has shifted to more quantifiable metrics, while behavioral aspects have diminished markedly in importance (17). Digital biomarkers have significant value in psychiatric conditions like schizophrenia, autism, and PTSD. In schizophrenia, they assist in early diagnosis, symptom tracking, and treatment optimization, enhancing patient care. For autism, digital biomarkers are crucial for monitoring social interactions and enabling early diagnosis and personalized interventions. In PTSD, these biomarkers aid in monitoring physical and behavioral responses, supporting early intervention and symptom assessment. Digital biomarkers are defined as objective, quantifiable physiological and behavioral data that are collected and measured by means of digital devices such as portables, wearables, implantables, or ingestibles. Digital technologies offer promising tools for detecting MDD and depression-related symptoms objectively and precisely (18, 19). These technologies enable the remote collection of large volumes of clinically relevant data, which may be less burdensome than traditional in-clinic visits and more reflective of clinically relevant changes (20, 21). Wearable technologies, such as smartwatches and novel sensors, can generate valuable digital biomarkers of depression in real-world settings (22) building a digital phenotyping, defined as the moment-by-moment quantification of the individual-level human phenotypein its own environment using apps from smartphones or other personal devices (19, 23). In the recent years, several digital biomarkers have been investigated for MDD characterization and diagnosis such as measure patterns of physical activity (24, 25) features from voice samples (26, 27), light exposure measurements (25), mobile phone global positioning systems (GPS) and normal usage of smartphones such as usage duration and frequency (22).
2 Aims of this review
This review investigates how digital technologies, such as wearables and smartphone apps, are revolutionizing the assessment and treatment of major depressive disorder (MDD) and treatment-resistant depression (TRD). It anticipates that as digital mental health assessment advances and precision medicine is applied, the quality of life for individuals with MDD and TRD will improve. The review also identifies research gaps and recommends further investigation.
3 Methods
3.1 Search strategy and study eligibility criteria
To identify studies on the use of wearable devices in depression research, a literature search was performed to two major health-related databases: PubMed and APA PsycINFO, focusing on articles published from 1st of January 2013 to 30 July 2023. We searched for papers in which abstracts included the terms: Depression AND Device OR Depression AND digital tools OR Depression AND digital biomarkers OR Depression AND smartwatch OR Resistant depression AND digital biomarker OR Resistant depression AND digital biomarker OR Resistant depression AND actigraphy OR Resistant depression AND smartwatch.
Studies were chosen based on these inclusion criteria: randomized controlled trial, retrospective study, cohort study, open study, expert opinion, concerning conceptualization, diagnosis of major depressive disorder (MDD) according to DSM-5 or ICD-10, studies published in English, studies carried out in humans and studies published in journals indexed in Embase or Medline.
The exclusion criteria were meta-analysis, review, duplicates, comments, editorials, case reports/case series, theses, proceedings, letters, short surveys and notes, studies irrelevant for the topic, unavailable full-text and studies that do not meet inclusion criteria.
The PRISMA search process is presented in Figure 1.
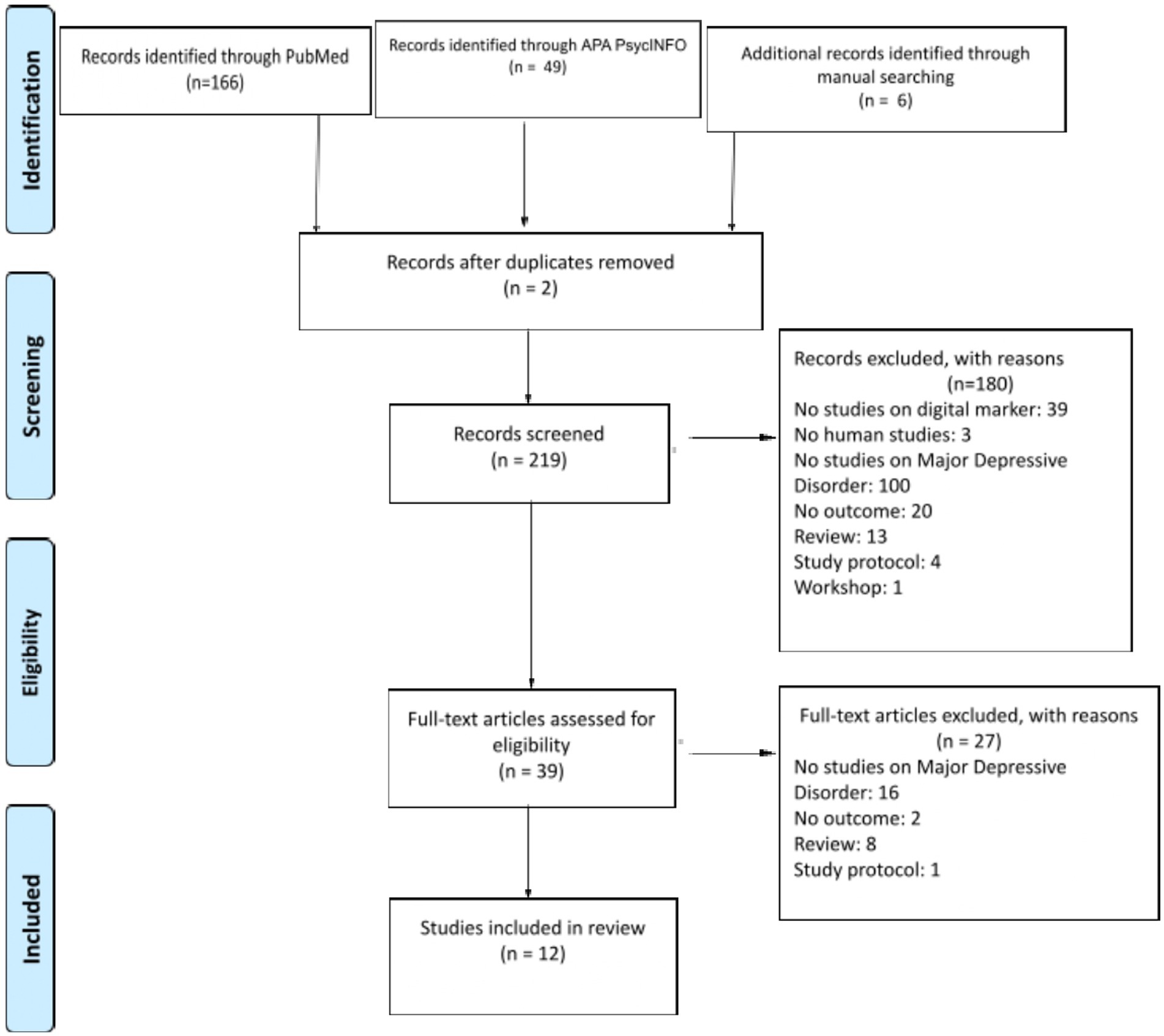
Figure 1. Flowchart of the study selection process. Adapted from Moher D, Liberati A, Tetzlaff J, Altman DG, PRISMA Group (2009). Preferred reporting items for systematic reviews and meta-analyses: the PRISMA statement. PLoS Med. 21. e1000097. doi: 10.1371/journal.pmed.1000097.
We collected a total of 12 studies that made use of wearable devices to assess or monitor depressive symptoms and TRD or to predict MDD (Table 1).
3.2 Study selection
The selection of studies for this review occurred in a two-stage process. Initially, two independent reviewers assessed the titles and abstracts of all the retrieved papers. In the subsequent stage, these same reviewers individually examined the full texts of the papers identified in the first phase. Any discrepancies between the two reviewers were resolved by involving a third reviewer.
3.3 Data extraction and data synthesis
Data extraction for each included study was carried out by two independent researchers, namely AV and FM, utilizing a standardized data extraction sheet in Microsoft Excel. The focus of this extraction encompassed several key subjects, including study design, participant characteristics, diagnosis of MDD, and digital device details derived from the original research.
In the event of any disparities in data entry between these two researchers, such discrepancies were thoroughly deliberated with two additional independent reviewers when deemed necessary.
4 Results
4.1 Literature search
The search across PubMed and APA PsycINFO bibliographic database produced a total of 215 records. Additionally, by conducting backward reference list checking and forward reference list checking, we discovered 6 new studies. After initial screening based on their titles and abstracts, 180 records were excluded. Out of the initial 39 references, the synthesis now comprises a total of 12 articles.
4.2 General description of included studies
Among the various studies examining wearable devices for individuals with MDD, approximately one- third employed actigraph units, while the rest utilized commercial wearable devices not originally designed for medical purposes. These included devices such as the Fitbit® (Fitbit®, Inc., San Francisco, CA, United States), the Apple Watch (Apple Inc., Cupertino, CA, United States), and the Mi Smartwatch (Xiaomi Corporation, China). In addition, some studies relied on mobile applications (Apps).
Through a narrative synthesis of various reviews and in agreement with several authors’ perspectives, we have pinpointed specific domains where the integration of tools and digital markers significantly enhances clinicians’ capabilities in predicting, diagnosing, and providing care and treatment for individuals grappling with MDD and TRD.
The reviewed studies focused on gathering specific physiological, activity/sleep, or subjective data from individuals through digital devices, with the aim of exploring the relationship between these parameters and depression.
4.2.1 Predictive modeling of diagnostic status
Multiple studies have showcased the promise of digital biomarkers, encompassing factors like movement intensity, light exposure, and smartphone usage patterns, in forecasting the diagnostic status of individuals grappling with mood disorders (19, 22, 38). Jacobson et al. (28) conducted a study to identify digital biomarkers for MDD and bipolar disorder (BD) and track symptom changes over 2 weeks. By analyzing movement patterns, they used extreme gradient boosting to achieve an 89% accuracy in predicting diagnostic groups and monitoring symptom changes. Combining MDD and BD data revealed potential transdiagnostic traits. Movement and light data were found relevant for detecting mood disorders and correlated with behaviors like energy levels, psychomotor activity, and sleep disturbances during mood episodes. Two studies focus on data analysis to identify important predictors in different contexts (35, 39). In their study Mahendran et al. (35), researchers used cardiac monitoring data, which included questionnaire responses and smartwatch sensor data. They then trained machine learning models such as logistic regression and random forest, and the results showed that the ensemble of models performed better than individual implementations, with random forest standing out. McNamara et al. (39), used a wide range of data, including demographic data, biobehavioral measurements, and self-report questionnaires to identify predictors of depression. The results revealed that psychosocial predictors such as negative self-referential thinking, rumination, self- reported sleep quality, and functional distress were important in predicting depression.
4.2.2 Assessment of symptom severity and progression
Digital phenotyping has emerged as a valuable tool for evaluating the severity and trajectory of mood disorder symptoms. In the realm of assessing depression severity and treatment response in individuals with MDD, researchers have explored the utilization of digital biomarkers and technologies. Four notable studies shed light on this area: in the study conducted by Jacobson et al. (24), passive movement and light exposure data were analyzed in 15 medicated outpatients with MDD over a week. The study demonstrated that passive movement and light data could effectively gauge depression severity, even in cases of high severity. However, while modern lifestyles often drive the development of technology tailored for personal fitness, such as Fitbit® and various apps that monitor vital signs like heart rate and body temperature, many devices used in research are repurposed for the advancement of mental health applications. Abbas et al. (31), used the AiCure smartphone app to track digital biomarkers associated with MDD under antidepressant therapy (ADT). These markers included voice, facial expressions, and movement indicators. The study found that monoamine ADTs, such as SSRIs and SNRIs, had a significant impact on digital biomarker with a reduction in symptom severity as assessed by the MADRS evaluation, indicating an improvement in motor functioning and a decrease in depression severity due to these treatments. Indeed, in Sverdlov et al. (30), the authors points out that common efficacy scales like HAM-D and MADRS are subjective and prone to bias. Digital technologies offer objective tools for depression symptom detection. Mobile apps and wearables can generate digital biomarkers in real-world settings. The study assessed seven digital technologies in individuals with unipolar depression and healthy controls, aiming to distinguish between them, build accurate classifiers, and explain variation in MADRS scores. Technologies were evaluated to identify digital biomarkers revealing correlations between different digital Importantly, selected digital biomarker features demonstrated predictive capabilities for individual. Therefore, Siddi et al. (29) in their study with up to 2 years follow up, based on data from the RADAR-MDD study involving 600 individuals with MDD, examined the relationship between heart rate (HR) parameters using a Fitbit® device and the severity of depression. The findings showed that individuals with higher depression severity tended to have a lower resting HR variation throughout the day, and this association remained significant even after accounting for individual characteristics. The research indicates that passive behaviors, which are indicative of depression, are more common in individuals with greater depression severity, particularly during the nighttime when HR may be elevated due to the sleep problems often seen in those with MDD.
4.2.3 Treatment response monitoring
Assessing treatment responses in the context of mood disorders constitutes a pivotal research domain. Abbas et al. (31) showcased the capacity of digital biomarkers, encompassing motor function, to accurately monitor shifts in depression severity throughout the course of antidepressant therapy. In a parallel attempt, Kim et al. (33) used smartphone data to prognosticate treatment outcomes among adolescents grappling with MDD, shedding light on the prospect of personalized treatment strategies through the avenue of digital phenotyping. In their research, Winkler et al. (36) investigated the impact of electroconvulsive therapy (ECT) on rest-activity patterns in patients with TRD. They studied 15 individuals with TRD who received ECT and used wrist actigraphy to measure their activity levels before and after treatment. They observed that Individuals who reached remission experienced notable enhancements in light activity, overall activity, and circadian amplitude and ECT had a limited impact on the timing of peak activity or actigraphic sleep measurements. In 2016, Nishida et al. (37) conducted an open-label pilot study on 14 medication-resistant MDD patients to assess the impact of rTMS on their rest-activity cycle and sleep disturbances. They administered 10 rTMS sessions targeting the bilateral dorsolateral prefrontal cortex and used waist actigraphy to measure changes in the rest- activity cycle. The results showed significant improvements in depression symptoms and sleep quality measured by rating scales, but actigraphy-based sleep measures did not exhibit substantial changes. Digital therapeutics are under study and represent a potential future clinical vista in this population (18). These findings, coupled with advancements in the realm of digital biomarkers and the refinement of neurostimulation parameters, hold potential for improving overall health results and the cost efficiency of MDD and TRD treatment.
4.2.4 Real-world monitoring and ecological validity
Incorporating wearable devices into depression research offers several benefits. Wearable technology allows for ongoing and unbiased observation of individuals in their everyday environments. This enables the objective tracking of real-time changes and enhances the precision of monitoring treatment outcomes. Cormack et al. (32), involving individuals with MDD, explored the use of wearable technology for high-frequency cognitive and mood assessments over 6 weeks. The study found that daily assessments were practical and showed meaningful correlations with established measures of mood and cognition. While there was some improvement in mood, it varied among participants, highlighting the complexity of depression. In Kim et al. (33) paper, data from a smartphone app called “STAR-DS” was used to predict depressive symptoms and treatment responses in adolescents. The study found that call-related features, smartphone usage duration, and movement distance were important predictors of MDD. Call duration was especially significant in predicting treatment responses. Adolescents with MDD had different smartphone usage patterns compared to controls. This study emphasized the potential of smartphone behaviors in forecasting depression outcomes. Remote measurement technologies were used to monitor individuals with MDD in real-world settings. The study of Zhang et al. (34), found that Bluetooth device count (NBDC) data was correlated with depressive symptoms. Lower PHQ-8 scores were associated with increased social activities. Changes in NBDC data were linked to fluctuations in depressive manifestations and behaviors, including reduced social engagement, impaired work, or study performance, and disrupted circadian rhythms. This research highlighted the feasibility of using NBDC for monitoring individuals with MDD in real-life contexts.
5 Discussion
The review of the selected studies on predictive modeling, assessment of symptom severity and progression, treatment response monitoring, and real-world monitoring with ecological validity showcases the remarkable potential of digital biomarkers and technologies in advancing our understanding and management of MDD and TRD. The ability to predict diagnostic status with a high degree of accuracy using digital biomarkers is may be a transformative breakthrough. The integration of movement, light exposure, and smartphone data has not only enabled accurate predictions but has also revealed common features across mood disorders, highlighting the existence of transdiagnostic traits. This is in line with the research domain criteria (RDoC) criteria and opens new prospective for understanding the underlying mechanisms of mood disorders (35, 39). Assessing symptom severity and progression may be significantly enhanced by digital phenotyping, although this kind of technology is rapidly improving and the validity of these measurements need more strong confirmations. The use of passive movement and light exposure data, often repurposed from personal fitness technology, demonstrates the adaptability and versatility of digital biomarkers in assessing depression severity. Moreover, the impact of antidepressant therapies on digital biomarkers related to motor function may provides valuable insights into the mechanisms of action of these treatments. The ability to predict individual depression severity scores are groundbreaking advancements in the field, digital biomarkers potential role in predicting individual depression severity may be a groundbreaking advancement, once confirmed and replicated in larger populations studies, providing clinicians with even more personalized assessment instruments (29, 30). Monitoring treatment responses, especially in cases of treatment-resistant depression (TRD), is crucial for improving patient outcomes. These advancements, coupled with the refinement of neurostimulation parameters, hold the potential to enhance overall health outcomes and the cost-effectiveness of TRD care. This suggests that approaches, such as electroconvulsive therapy (ECT) and repetitive transcranial magnetic stimulation (rTMS), have the potential to positively impact the rest-activity cycle in TRD patients who achieve remission. The demonstrated effectiveness of digital biomarkers, including motor function, in tracking shifts in depression severity during treatment is a significant step forward (33, 36). Additionally, the potential for personalized treatment strategies through smartphone data analysis among adolescents with MDD holds promise for tailoring interventions to individual needs. Real-world monitoring with ecological validity using wearable technology and smartphone apps represents a paradigm shift in depression research. The ability to conduct daily assessments in natural environments provides a more comprehensive understanding of mood fluctuations and cognitive changes. Smartphone behaviors and Bluetooth device count data offer exciting prospects for predicting MDD and treatment responses. These findings underscore the dynamic nature of depression and the importance of considering real-world factors in assessment and treatment planning (33, 34).
6 Conclusion
This narrative review highlights the diverse research areas that underscore the versatility and potential of digital biomarkers and technologies in the diagnosis, assessment, and treatment of mood disorders. In this review, we delve into the technologies, available research findings, and implementation challenges most pertinent to the integration of digital psychiatry within MDD and its resistant forms. The integration of wearable devices with smart devices, such as mobile phones, has gained widespread acceptance due to their convenience and style (20). Notably, our review is the first in the literature to focus on wearable devices targeting depression assessed using DSM-5 or ICD-10 criteria. Although the different wearable device technologies were examined, the review falls short of reporting the effectiveness measure values, and therefore does not assess performance. This review underscores the potential for remote diagnosis and prediction using these devices. Future trends are anticipated with the emergence of new wearable devices that will introduce innovative diagnostic and therapeutic approaches like motion capture, speech analysis, and portable light therapy. These developments hold the promise of fundamental changes in the diagnosis and treatment of depression, potentially enabling early and precise diagnosis, personalized treatment for depression patients, and preventive measures for at-risk groups (40, 41). Digital psychiatry encompasses various aspects of healthcare, including delivery, illness surveillance, disease management, and treatment. Advances in artificial intelligence and machine learning are expected to serve as a crucial bridge for translating new data into clinically relevant digital biomarkers (22, 38).
Wearable devices are poised to play a critical role in medicine, particularly in the context of personalized telemedicine. Future research endeavors should continue to explore these areas, enhancing the precision and efficacy of digital phenotyping in mental healthcare, ultimately leading to an improved quality of life for individuals affected by MDD.
Author contributions
AV: Conceptualization, Data curation, Methodology, Project administration, Resources, Writing – original draft. FM: Conceptualization, Methodology, Project administration, Writing – original draft. CP: Writing – review & editing. MP: Writing – review & editing. FF: Writing – review & editing. GP: Writing – review & editing. RS: Writing – review & editing. AM: Writing – review & editing. JS: Writing – review & editing. MS: Writing – review & editing. GC: Writing – review & editing.
Funding
The author(s) declare financial support was received for the research, authorship, and/or publication of this article. This study was funded by POR CAMPANIA FESR 2014–2020 ASSE PRIORITARIO 3 (Grant No. 2022.108).
Conflict of interest
The authors declare that the research was conducted in the absence of any commercial or financial relationships that could be construed as a potential conflict of interest.
Publisher’s note
All claims expressed in this article are solely those of the authors and do not necessarily represent those of their affiliated organizations, or those of the publisher, the editors and the reviewers. Any product that may be evaluated in this article, or claim that may be made by its manufacturer, is not guaranteed or endorsed by the publisher.
References
1. World Health Organization. World mental health report: transforming mental health for all World Health Organization (2022).
2. Maj, M. Validity and clinical utility of the current operational characterization of major depression. Int Rev Psychiatry. (2012) 24:530–7. doi: 10.3109/09540261.2012.712952
3. Maj, M. Development and validation of the current concept of major depression. Psychopathology. (2012) 45:135–46. doi: 10.1159/000329100
4. Solmi, M, Radua, J, Olivola, M, Croce, E, Soardo, L, Salazar de Pablo, G, et al. Age at onset of mental disorders worldwide: large-scale meta-analysis of 192 epidemiological studies. Mol Psychiatry. (2022) 27:281–95. doi: 10.1038/s41380-021-01161-7
6. Yalin, N, and Young, AH. Pharmacological treatment of bipolar depression: what are the current and emerging options? Neuropsychiatr Dis Treat. (2020) 16:1459–72. doi: 10.2147/NDT.S245166
7. Ormel, J, Kessler, RC, and Schoevers, R. Depression: more treatment but no drop in prevalence: how effective is treatment? And can we do better? Curr Opin Psychiatry. (2019) 32:348–54. doi: 10.1097/YCO.0000000000000505
8. Dobson, KS, Hollon, SD, Dimidjian, S, Schmaling, KB, Kohlenberg, RJ, Gallop, RJ, et al. Randomized trial of behavioral activation, cognitive therapy, and antidepressant medication in the prevention of relapse and recurrence in major depression. J Consult Clin Psychol. (2008) 76:468–77. doi: 10.1037/0022-006X.76.3.468
9. Vittengl, JR, Clark, LA, Dunn, TW, and Jarrett, RB. Reducing relapse and recurrence in unipolar depression: a comparative meta-analysis of cognitive-behavioral therapy’s effects. J Consult Clin Psychol. (2007) 75:475–88. doi: 10.1037/0022-006X.75.3.475
10. McIntyre, RS, Alsuwaidan, M, Baune, BT, Berk, M, Demyttenaere, K, Goldberg, JF, et al. Treatment- resistant depression: definition, prevalence, detection, management, and investigational interventions. World Psychiatry. (2023) 22:394–412. doi: 10.1002/wps.21120
11. Rush, AJ, Trivedi, MH, Wisniewski, SR, Nierenberg, AA, Stewart, JW, Warden, D, et al. Acute and longer-term outcomes in depressed outpatients requiring one or several treatment steps: a STAR*D report. Am J Psychiatry. (2006) 163:1905–17. doi: 10.1176/ajp.2006.163.11.1905
12. Voineskos, D, Daskalakis, ZJ, and Blumberger, DM. Management of treatment-resistant depression: challenges and strategies. Neuropsychiatr Dis Treat. (2020) 16:221–34. doi: 10.2147/NDT.S198774
13. Fried, EI. Moving forward: how depression heterogeneity hinders progress in treatment and research. Expert Rev Neurother. (2017) 17:423–5. doi: 10.1080/14737175.2017.1307737
14. Fried, EI. The 52 symptoms of major depression: lack of content overlap among seven common depression scales. J Affect Disord. (2017) 208:191–7. doi: 10.1016/j.jad.2016.10.019
15. Taliaz, D, Spinrad, A, Barzilay, R, Barnett-Itzhaki, Z, Averbuch, D, Teltsh, O, et al. Optimizing prediction of response to antidepressant medications using machine learning and integrated genetic, clinical, and demographic data. Transl Psychiatry. (2021) 11:381. doi: 10.1038/s41398-021-01488-3
16. Insel, TR. The NIMH research domain criteria (RDoC) project: precision medicine for psychiatry. Am J Psychiatry. (2014) 171:395–7. doi: 10.1176/appi.ajp.2014.14020138
17. Insel, TR. Digital phenotyping: technology for a new science of behavior. JAMA. (2017) 318:1215–6. doi: 10.1001/jama.2017.11295
18. McIntyre, RS, Greenleaf, W, Bulaj, G, Taylor, ST, Mitsi, G, Saliu, D, et al. Digital health technologies and major depressive disorder. CNS Spectr. (2023) 1-12:1–12. doi: 10.1017/S1092852923002225
19. Lee, S, Kim, H, Park, MJ, and Jeon, HJ. Current advances in wearable devices and their sensors in patients with depression. Front Psychiatry. (2021) 12:672347. doi: 10.3389/fpsyt.2021.672347
20. Ahmed, A, Aziz, S, Alzubaidi, M, Schneider, J, Irshaidat, S, Abu Serhan, H, et al. Wearable devices for anxiety & depression: a scoping review. Comput Methods Programs Biomed Update. (2023) 3:100095. doi: 10.1016/j.cmpbup.2023.100095
21. Ahmed, A, Hassan, A, Aziz, S, Abd-Alrazaq, AA, Ali, N, Alzubaidi, M, et al. Chatbot features for anxiety and depression: a scoping review. Health Informatics J. (2023) 29:14604582221146719. doi: 10.1177/14604582221146719
22. Torous, J, Wisniewski, H, Liu, G, and Keshavan, M. Mental health mobile phone app usage, concerns, and benefits among psychiatric outpatients: comparative survey study. JMIR Ment Health. (2018) 5:e11715. doi: 10.2196/11715
23. Rajagopalan, A, Shah, P, Zhang, MW, and Ho, RC. Digital platforms in the assessment and monitoring of patients with bipolar disorder. Brain Sci. (2017) 7:150. doi: 10.3390/brainsci7110150
24. Jacobson, NC, Weingarden, H, and Wilhelm, S. Using digital phenotyping to accurately detect depression severity. J Nerv Ment Dis. (2019) 207:893–6. doi: 10.1097/NMD.0000000000001042
25. Tonon, AC, Fuchs, DFP, Barbosa Gomes, W, Levandovski, R, de Almeida, P, Fleck, M, et al. Nocturnal motor activity and light exposure: objective actigraphy-based marks of melancholic and non-melancholic depressive disorder. Brief report. Psychiatry Res. (2017) 258:587–90. doi: 10.1016/j.psychres.2017.08.025
26. Mundt, JC, Snyder, PJ, Cannizzaro, MS, Chappie, K, and Geralts, DS. Voice acoustic measures of depression severity and treatment response collected via interactive voice response (IVR) technology. J Neurolinguistics. (2007) 20:50–64. doi: 10.1016/j.jneuroling.2006.04.001
27. Zhang, L, Duvvuri, R, Chandra, KKL, Nguyen, T, and Ghomi, RH. Automated voice biomarkers for depression symptoms using an online cross-sectional data collection initiative. Depress Anxiety. (2020) 37:657–69. doi: 10.1002/da.23020
28. Jacobson, NC, Weingarden, H, and Wilhelm, S. Digital biomarkers of mood disorders and symptom change. NPJ Digit Med. (2019) 2:3. doi: 10.1038/s41746-019-0078-0
29. Siddi, S, Bailon, R, Gine-Vazquez, I, Matcham, F, Lamers, F, Kontaxis, S, et al. The usability of daytime and night-time heart rate dynamics as digital biomarkers of depression severity. Psychol Med. (2023) 53:3249–60. doi: 10.1017/S0033291723001034
30. Sverdlov, O, Curcic, J, Hannesdottir, K, Gou, L, De Luca, V, Ambrosetti, F, et al. A study of novel exploratory tools, digital technologies, and central nervous system biomarkers to characterize unipolar depression. Front Psychiatry. (2021) 12:640741. doi: 10.3389/fpsyt.2021.640741
31. Abbas, A, Sauder, C, Yadav, V, Koesmahargyo, V, Aghjayan, A, Marecki, S, et al. Remote digital measurement of facial and vocal markers of major depressive disorder severity and treatment response: a pilot study. Front Digit Health. (2021) 3:610006. doi: 10.3389/fdgth.2021.610006
32. Cormack, F, McCue, M, Taptiklis, N, Skirrow, C, Glazer, E, Panagopoulos, E, et al. Wearable technology for high-frequency cognitive and mood assessment in major depressive disorder: longitudinal observational study. JMIR Ment Health. (2019) 6:e12814. doi: 10.2196/12814
33. Kim, JS, Wang, B, Kim, M, Lee, J, Kim, H, Roh, D, et al. Prediction of diagnosis and treatment response in adolescents with depression by using a smartphone app and deep learning approaches: usability study. JMIR Form Res. (2023) 7:e45991. doi: 10.2196/45991
34. Zhang, Y, Folarin, AA, Sun, S, Cummins, N, Ranjan, Y, Rashid, Z, et al. Predicting depressive symptom severity through individuals’ nearby Bluetooth device count data collected by mobile phones: preliminary longitudinal study. JMIR Mhealth Uhealth. (2021) 9:e29840. doi: 10.2196/29840
35. Mahendran, N, Vincent, DR, Srinivasan, K, Chang, CY, Garg, A, Gao, L, et al. Sensor-assisted weighted average ensemble model for detecting major depressive disorder. Sensors. (2019) 19:4822. doi: 10.3390/s19224822
36. Winkler, D, Pjrek, E, Lanzenberger, R, Baldinger, P, Eitel, D, Kasper, S, et al. Actigraphy in patients with treatment-resistant depression undergoing electroconvulsive therapy. J Psychiatr Res. (2014) 57:96–100. doi: 10.1016/j.jpsychires.2014.06.006
37. Nishida, M, Kikuchi, S, Nisijima, K, and Suda, S. Actigraphy in patients with major depressive disorder undergoing repetitive transcranial magnetic stimulation: an open label pilot study. J ECT. (2017) 33:36–42. doi: 10.1097/YCT.0000000000000352
38. Torous, J, Lipschitz, J, Ng, M, and Firth, J. Dropout rates in clinical trials of smartphone apps for depressive symptoms: a systematic review and meta-analysis. J Affect Disord. (2020) 263:413–9. doi: 10.1016/j.jad.2019.11.167
39. McNamara, ME, Shumake, J, Stewart, RA, Labrada, J, Alario, A, Allen, JJB, et al. Multifactorial prediction of depression diagnosis and symptom dimensions. Psychiatry Res. (2021) 298:113805. doi: 10.1016/j.psychres.2021.113805
40. Moshe, I, Terhorst, Y, Philippi, P, Domhardt, M, Cuijpers, P, Cristea, I, et al. Digital interventions for the treatment of depression: a meta-analytic review. Psychol Bull. (2021) 147:749–86. doi: 10.1037/bul0000334
Keywords: major depressive disorder, digital biomarkers, wearable devices, artificial intelligence, personalized treatment, mental healthcare
Citation: Vignapiano A, Monaco F, Pagano C, Piacente M, Farina F, Petrillo G, Sica R, Marenna A, Shin JI, Solmi M and Corrivetti G (2023) A narrative review of digital biomarkers in the management of major depressive disorder and treatment-resistant forms. Front. Psychiatry. 14:1321345. doi: 10.3389/fpsyt.2023.1321345
Edited by:
Vassilis Martiadis, Asl Napoli 1 Centro, ItalyReviewed by:
Luca Steardo, University Magna Graecia of Catanzaro, ItalyPaolo Meneguzzo, University of Padua, Italy
Copyright © 2023 Vignapiano, Monaco, Pagano, Piacente, Farina, Petrillo, Sica, Marenna, Shin, Solmi and Corrivetti. This is an open-access article distributed under the terms of the Creative Commons Attribution License (CC BY). The use, distribution or reproduction in other forums is permitted, provided the original author(s) and the copyright owner(s) are credited and that the original publication in this journal is cited, in accordance with accepted academic practice. No use, distribution or reproduction is permitted which does not comply with these terms.
*Correspondence: Annarita Vignapiano, annarita.vignapiano@gmail.com
†These authors have contributed equally to this work and share first authorship