- 1Department of Psychiatry and Behavioral Neurosciences, Wayne State University School of Medicine, Detroit, MI, United States
- 2Department of Psychiatry, University of Michigan, Ann Arbor, MI, United States
Abnormal function of the thalamo-cortical relay is considered a hallmark of obsessive-compulsive disorder (OCD) and aberrant network interactions may underpin many of the clinical and cognitive symptoms that characterize the disorder. Several statistical approaches have been applied to in vivo fMRI data to support the general loss of thalamo-cortical connectivity in OCD. However, (a) few studies have assessed the contextual constraints under which abnormal network interactions arise or (b) have used methods of effective connectivity to understand abnormal network interactions. Effective connectivity is a particularly valuable method as it describes the putative causal influences that brain regions exert over each other, as opposed to the largely statistical consistencies captured in functional connectivity techniques. Here, using dynamic causal modeling (DCM), we evaluated how attention demand induced inter-group differences (HC ≠ OCD) in effective connectivity within a motivated thalamo-cortical network. Of interest was whether these effects were observed on the ascending thalamo-cortical relay, essential for the sensory innervation of the cortex. fMRI time series data from sixty-two participants (OCD, 30; HC, 32) collected using an established sustained attention task were submitted to a space of 162 competing models. Across the space, models distinguished between competing hypotheses of thalamo-cortical interactions. Bayesian model selection (BMS) identified marginally differing likely generative model architectures in OCD and HC groups. Bayesian model averaging (BMA), was used to weight connectivity parameter estimates across all models, with each parameter weighted by each model’s posterior probability, thus providing more stable estimates of effective connectivity. Inferential statistical analyses of estimated parameters revealed two principal results: (1) Significantly reduced intrinsic connectivity of the V1 → SPC pathway in OCD, suggested connective weakness in the early constituents of the dorsal visual pathway; (2) More pertinent with the discovery possibilities afforded by DCM, sustained attention in OCD patients induced significantly reduced contextual modulation of the ascending relay from the thalamus to the prefrontal cortex. These results form an important complement to our understanding of the contextual bases of thalamo-cortical network deficits in OCD, emphasizing vulnerability of the ascending relay.
Introduction
Obsessive-compulsive disorder (OCD) is a common neuropsychiatric disorder characterized by excessive anxiety-inducing thoughts (obsessions) that lead to repetitive anxiety-reducing behaviors (compulsions). In youth, incidence rates of OCD are high, and sub-clinical OCD symptoms are highly prevalent (1). Elucidating brain network dysfunction is of significant importance in clinical neuroscience because network neuroscience provides the best hope of identifying mechanistic pathways in the brain (2), and because the relative stability of OCD symptoms into adulthood suggests that trait-related network dysfunction may underpin this condition (3). The goal of identifying plausible mechanistic pathways has been central to all of medicine (2, 4), because an understanding of such mechanisms can result in better outcomes. Moreover, such understanding can serve as targets for assessing the efficacy of pharmacological or other interventions in OCD. This may be particularly important as many first-line treatments such as cognitive behavioral therapy (CBT) and selective serotonin reuptake inhibitors (SSRIs) remain ineffective in a substantial number of patients (5). Of particular interest is the characterization of causal or directional interactions between brain network constituents (4). Recovering causal relationships within the brain is a central challenge in neuroscience, largely because the activity of network constituents is generally not driven by single causes, and network constituents do not exert mono-causal effects on each other (6, 7). Rather, network interactions emerge from partitions of neuronal states over progressively coarser scales and that retain a measure of directionality (8). It is acknowledged that largely correlative measures for functional connectivity, while valuable in capturing time ordered statistical regularities between the activity of network constituents, do not provide an understanding of how network constituents impact each other (4). However, such interactions can be successfully recovered from fMRI times series data using dynamic causal modeling (DCM), the central method for effective connectivity analyses (9). In contra-position to functional connectivity, effective connectivity seeks to mechanistically model network interactions by recovering experimental and time-dependent, observed timing relationships between recorded neurophysiological signals (9). Thus, in the context of conditions like OCD the application of DCM can elucidate the functionally-evoked signatures of network dysfunction, and understanding task-evoked network dysfunction is key to understanding brain network dysfunction in neuropsychiatric disorders (10). Indeed, DCM has been used to study dysfunctional effective connectivity in OCD youth in domains including emotional processing (11, 12) and cognitive control (13). Herein, we provide the first extension of DCM to understand if and how task-processing during the more basic domain of sustained attention, evokes disordered effective connectivity in OCD youth (14).
Sustained attention involves the maintenance of vigilance, or tonic alertness, in the service of task persistence over sustained periods of time (15). Unsurprisingly, sustained attention is seen as a quantitative biological trait that is reliable and reasonably heritable (16), and therefore an intermediate phenotype across many psychiatric conditions including OCD (17). Impairments in sustained attention are “upstream” from other critical higher cognitive domains such as learning and memory (18). In real world settings, a loss in cognitive competence in the classroom in patients with OCD has been associated with stigmatizing attitudes amongst poorly informed teachers (19). Thus, a focus on sustained attention in OCD is not simply an issue of scientific curiosity, but one of direct clinical relevance.
Sustained attention is sub served by critical thalamo-cortical interactions (20), with the ascending thalamo-cortical relay to the dorsal prefrontal cortex (dPFC) being particularly important in mediating cortical innervation and network synchronization (21). Notably, effective connectivity of thalamo-cortical networks in healthy youth (22), reveals an intricate interplay between sustained attention processing and the impact of such processing on changes in the effective connectivity of thalamo-cortical pathways. However, such interactions have not been assessed in OCD. This is a critical lacuna because understanding altered thalamo-cortical network function in OCD may elucidate how the antecedents of higher-order behavioral dysfunction in OCD lies in functional impairments in more rudimentary brain pathways (23). Here we applied DCM to discover whether sustained attention evoked differences in the effective connectivity of frontal-striatal-thalamic networks in OCD youth and typical controls. Sustained attention was manipulated using a specifically tailored version of the continuous performance task (CPT) (14). The task required participants to remain vigilant for extended periods (∼120 s) during which time, numbers were presented in rapid sequence. Participants were required to detect repeated instances of numbers. A simple parametric manipulation of attention demand was evoked by changing numerical magnitude: thus, alternating epochs consisted of either only two- (low demand) or three- (high demand) digit numbers. This parametric manipulation typical of sustained attention paradigms was intended to vary the behavioral demands placed on brain networks (22, 24, 25), wherein any demand-induced changes would be reflected in estimates of effective connectivity discovered with DCM (26).
Sustained attention, thalamo-cortical networks and relevance for obsessive-compulsive disorder
Why are thalamo-cortical networks central to sustained attention? All incoming sensory inputs (except olfactory) pass through the thalamus, and the structure has been viewed as a “relay station” and “gateway” to subcortical and cortical regions (27, 28). Anatomically, several distinct thalamic sub-nuclei synapse onto pertinent attention-related brain cortices (29, 30) including the dorsal anterior cingulate cortex (dACC), dPFC, primary visual cortex (V1), and the superior parietal cortex (SPC). In this regard, ascending relays from the thalamus assume importance.
Thalamic transmission of sensory information can be subdivided into first-order and higher-order relays. In first-order relays, thalamic nuclei convey the initial sensory inputs from sensory organs (i.e., eyes) to the cortex. For instance, retinal inputs travel to the lateral geniculate nucleus (LGN) of the thalamus before being relayed to the V1. In higher-order relays, thalamic nuclei receive descending inputs from cortical regions before relaying and controlling the flow of information back to the cortex (31–33). These descending (cortico-thalamic) and ascending (thalamo-cortical) pathways cumulatively underpin communication in brain networks (34), and suggest an important role for thalamic engagement and dynamic regulation of attentional, decision-making, executive control processes in the cortex (35).
Extant studies suggest that networks associated with sustained attention are subsets of the overall thalamo-cortical system (36). The reliably identified constituent regions of the attention network include frontal-striatal regions such as the dACC, the dPFC and the basal ganglia (BG)—all important for executive or supervisory mechanisms of attention (37, 38). The dACC functions as a “control center” organizing and recruiting executive brain regions like the dPFC (39–42). Additionally, the dACC shares bilateral connections to the dPFC, with the latter region known to be involved in the critical functioning of vigilance, executive control, working memory, and selective and divided attention (43). The BG are a varied group of subcortical nuclei involved in the functioning of eye movement (44), working memory (45), decision making (46), and in the organization of motivations that lead to the execution of goal-directed behaviors (e.g., organizing task-driven responses) (47). In addition to these “heteromodal” regions, multiple regions have been associated with spatial attention and orientation and sensory gating including the SPC, involved in the allocation of spatial attention, which is innervated by the V1 via the dorsal stream (48, 49) as well as direct thalamic connections (27, 40, 50).
Is there evidence of disordered brain function in OCD patients during attention processing? Utilizing a cued task-switching paradigm, Gu et al. found significantly reduced levels of brain activation in cortical (dPFC, dACC, and orbitofrontal cortex) and subcortical (caudate nucleus) regions, replicating prior studies on impaired executive functioning and cognitive flexibility (51); the task-switching paradigm required subjects to disengage attention from a prior task, resolve interference, and configure to a new cognitive task-set (52). Attention-related mechanisms in OCD have also been probed using the Stroop task, that measures functions related to selective attention, interference control, and cognitive flexibility (53). Exploring inhibition control in OCD, Page et al., used three different motor and cognitive control tasks (Go/No-go, motor Stroop, Switch tasks) to assess dysfunctional brain activations. The authors found reduced activation in OCD in multiple cortical (DLPFC, OFC), striatal, and thalamic regions (54). In a motor task with substantial attention demands, Meram and colleagues demonstrated disordered network-based mechanisms of frontal control in OCD (55), a pattern that replicates prior studies using working memory (56). Finally, Woolley et al. used an inhibitory (‘stop’) task, to show reduced activation of frontal, striatal and thalamic regions in pediatric OCD, evidence of dysregulation of fronto-striatal-thalamic (FST) regions during motor inhibition (57). Given that thalamic inputs are highly salient in driving cortical networks, and given the loss of cortical network tone in OCD, Del Casale and colleagues have suggested that altered thalamic gating in OCD, results in alterations in cortical activity in the disorder, leading to the subsequent emergence of core clinical characteristics including intrusive thoughts and repetitive and ritualistic behaviors (58). Is there any evidence of altered effective connectivity of ascending and/or descending relays of the thalamo-cortical network in OCD using task-based fMRI? To our knowledge this specific issue has not been addressed with DCM.
Functional connectivity studies in obsessive-compulsive disorder
What insights in OCD have we gained from functional connectivity studies? In the past 20 years, task-positive and resting-state functional connectivity studies have expanded our understanding of brain circuit abnormalities in OCD (59). Chiefly, our understanding of dysfunctional cortical, striatal, and thalamic (CSTC) circuits and resting-state networks [i.e., default-mode network (DMN) circuits] has been greatly expanded. Task-based fMRI studies have revealed abnormal patterns of hypo- and hyper-activation in domains such as executive functioning, emotional processing, and symptom provocation (59) with commonly implicated brain regions including: the prefrontal cortex (PFC) (54, 60), BG and thalamus (61), orbitofrontal cortex (OFC) (62), dACC (63), amygdala (64).
What is effective connectivity and how can it expand our understanding of dysfunctional brain networks in OCD? While functional connectivity describes statistical dependencies between times series, effective connectivity rests on a mechanistic model of the neurophysiological processes that generated the observed data (65). Thus, it is plausible to arrive at an understanding of the casual interactions one neuronal unit exerts over another; the elucidation of these causal influences via effective connectivity studies may prove pivotal in the characterization of aberrant pathways in OCD. Indeed, attempts to understand directed connectivity have been conducted using approaches such as psychophysiological interaction (PPI) (66) or structural equation modeling (SEM) (67). However, these approaches rely on observed fMRI signals (65). We relied on DCM as we were specifically motivated to recover causal effects between brain regions in OCD patients and controls.
Effective connectivity using dynamic causal modeling
Dynamic causal modeling allows for the interpretation of causal interactions between hidden state variables (9, 26) by modeling the brain as a bilinear input-output system. In this framework, inputs to the system are the experimental conditions and stimuli, and the outputs from the system are fMRI measured hemodynamic responses. DCM evaluates and assesses competing neurobiologically plausible models to determine any model’s posterior evidence for predicting the observed fMRI data. The competing models constitute a motivated a priori hypothesis space. Posterior probabilities are used to weight parameter estimates and differences between estimated parameters can be investigated using standard inferential statistics (26, 68). The following state differential equation is implemented to measure changes in neural responses:
Where, “A” represents the task-independent intrinsic (endogenous) coupling that exists between brain regions of interest, “B(j)” represents the task-dependent modulation of intrinsic connections via experimental manipulations, and variable “C” represents the sensorimotor driving inputs on cortical regions. We surmise that characterizing ascending thalamic-cortical relays in OCD is essential and that DCM applied to fMRI data collected during sustained attention, provides that ideal combination of method and task (4, 22, 69). Therefore, DCM allowed us to specifically assess the effects of task conditions on the ascending and descending relay and how (and why) any contextual modulation on thalamo-cortical relays might differ in OCD participants relative to controls.
Materials and methods
Subjects (participants)
Thirty OCD subjects (Age: 10.1–21.9 years; Mean Age: 16.2 years.; 10 males) and 32 healthy controls (Age: 10.9–21.1 years; Mean Age: 16.7 years; 12 males) provided informed consent or assent to participate in the fMRI study. For assenting participants, parental consent was also obtained. Participants’ IQ (89 < IQ < 143; Mean: 109.8; SD: 11.3) was assessed using either the Wechsler Intelligence Scale for Children (WISC) or the Wechsler Adult Intelligence Scale (WAIS). Healthy controls were evaluated for, and cleared of, any psychiatric illness. OCD participants were evaluated by a psychiatrist. Demographic and clinical characteristics of study participants can be viewed in Table 1.
The Schedule for Obsessive-Compulsive and Other Behavioral Syndromes and Schedule for Schizophrenia and Affective Disorders for School-Aged Children-Present and Lifetime Version were used to interview participants and their parents (70, 71). Furthermore, a modified version of the Children’s Yale-Brown Obsessive-Compulsive Disorder Scale (CY-BOCS) was used to measure the lifetime (maximum) and current severity of OCD participants (72, 73). Using DSM-5 criteria, clinicians independently confirmed the lifetime and current Axis I diagnosis. Participants with the following medical or psychiatric criteria were excluded: lifetime history of schizophrenia, bipolar disorder, substance abuse/dependence, anorexia nervosa, bulimia nervosa, epilepsy, head injuries resulting in chronic loss of consciousness, Huntington’s disease, dyskinesia, autism, IQ ≤ 80 or ≥ 15 on the lifetime version of the Social Communication Questionnaire (74, 75). The Wayne State University School of Medicine (WSUSOM) and the UM Human Investigation Committee approved our study.
fMRI task and data collection
All participants were administered a modified version of the Continuous Performance Task, Identical Pairs (CPT-IP) (Figure 1) deployed in previous studies (14, 22, 76), and required subjects to detect repeated numbers within a rapidly presented sequence. In separate blocks, two- or three-digit numbers were presented (50 ms, 250 ms SOA) over extended block lengths (120 s). The extended blocks were specifically designed to induce sustained (as opposed to transient) attention. Titrating demand in sustained attention has typically been implemented by changing the onsets between successively presented stimuli (77) or by experimentally manipulating stimulus characteristics (as undertaken here). Previous studies that have analyzed behavioral performance have demonstrated that the numerosity of the targets does evoke a lucid parameterization of task difficulty associated with attention-demand (Low vs. High); detection sensitivity (d’) for targets (repeated numbers) during three-digit epochs is lower than detection sensitivity for targets during two-digit epochs (14). Thus, attention demand constitutes a potentially critical modulator of the effective connectivity of ascending and/or descending thalamo-cortical relays (22, 78). In addition, 20 s rest epochs interspersed the experiment allowing participants some recovery between task-active epochs.
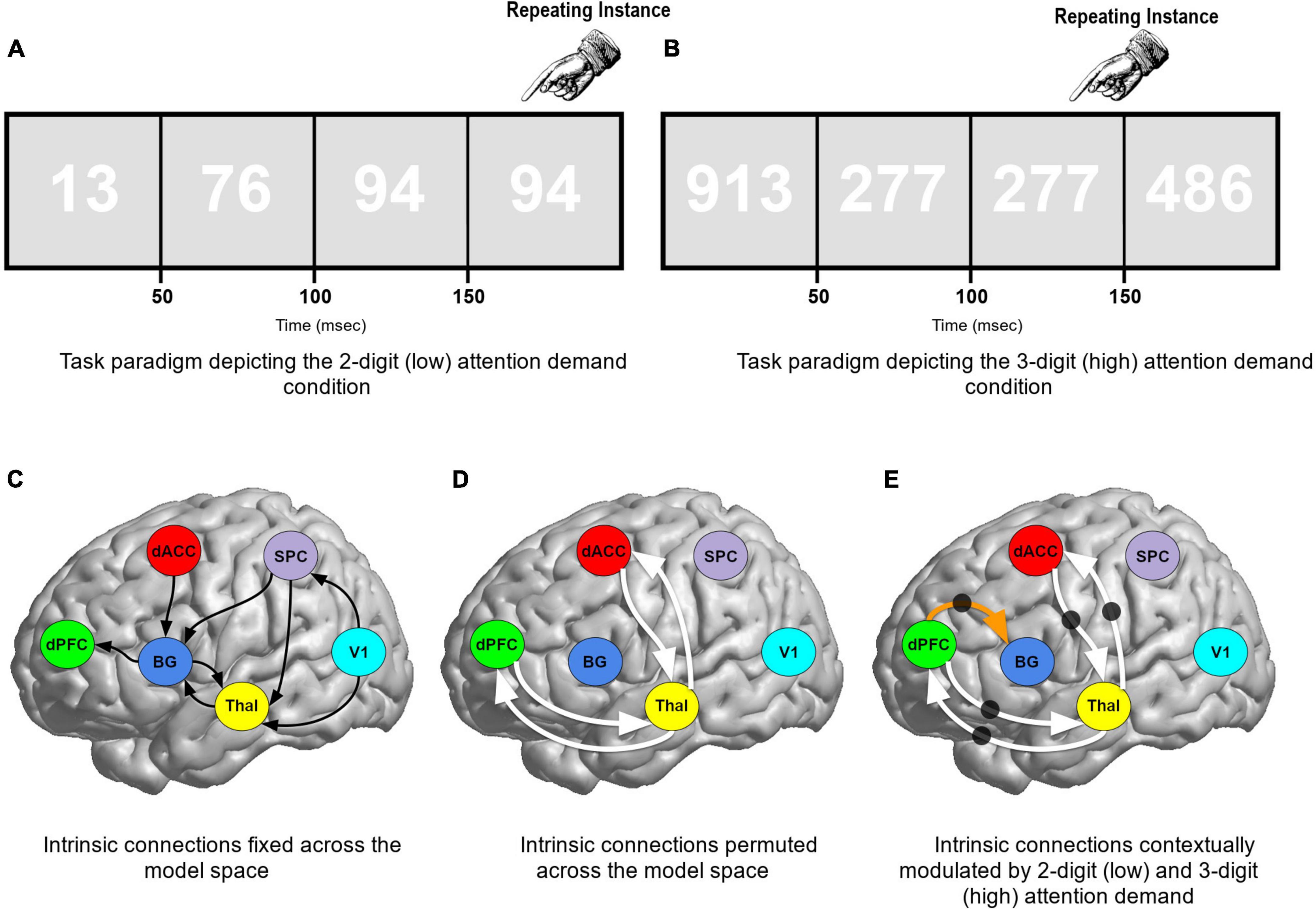
Figure 1. (A,B) The paradigm employed and the low and high demand conditions are schematically depicted. Participants performed a continuous performance task, identical pairs version (CPT-IP), and identified repeating instances of either 2- or 3-digit numbers (blocked in 120 s epochs). The magnitude of targets constitutes a parametric manipulation of attention demands: low (2-digit) or high (3-digit) attention demand. The remainder of the figure reveals the model space used for DCM. (C) Solid (curved) black lines represent the intrinsic connections fixed across the model space. (D) Solid white lines represent cortical-thalamic intrinsic connections that were permuted across the model space. The black circles (E) denote connections which were contextually modulated by both low (2-digit) and high (3-digit) attention demand conditions. Thus, the solid white lines were permuted for both intrinsic connectivity AND contextual modulation. The dPFC → BG connection (orange arrow) was contextually modulated when the intrinsic connection was present.
480 stimuli were shown in each block (25% were targets). To diminish figure-ground contrast (and increase attention commitments), numbers were presented in white numerical text (RGB: 255,255,255) against a grey background (RGB: 225,225,225) (79). Furthermore, to prevent selection of consecutive digits based on low-level features such as the lack of a flicker between repeated numbers, participants were presented with alternating fonts for successive digits in the sequence (78) thus ensuring a constant level of flicker throughout each task-active epoch.
Data acquisition
Gradient echo echo-planar imaging (EPI) fMRI data were obtained using a 3 Tesla Siemens Verio system (12-channel volume coil head) at the Vaitkevicius Magnetic Resonance Center. The following parameters were used for the T2* fMRI acquisition: TR: 2.6 s, echo time; TE: 29 ms; matrix dimensions: 128 × 128; voxel dimensions: 2 mm × 2 mm × 2 mm; field of view (FOV): 256 mm × 256 mm; 36 axial slices. The 3D matrix (128 × 128 × 36) provided very high resolution coverage of the cortex for a conventional EPI T2* sequence. The 36 axial slices were positioned parallel to the anterior commissure/posterior commissure (AC-PC) line and provided comprehensive coverage of the cerebrum. A T1-weighted structural image was obtained for preprocessing and co-registration using a 3D Magnetization Prepared Rapid Gradient Echo (MPRAGE) sequence with the following parameters: TR: 2,200 ms; TI: 778 ms; TE: 3 ms; FOV: 256 mm × 256 mm; matrix dimensions: 256 × 256; flip-angle: 13°; 256 axial slices, 1.0 mm thickness. All scans were reviewed by a neuroradiologist to exclude clinically significant irregularities.
fMRI processing (image preprocessing)
Image processing was undertaken in SPM121 using established methods for temporal (slice timing correction) and spatial preprocessing. First, EPI images were manually oriented to the AC-PC line with the reorientation vector applied across the EPI image set, realigned to a reference image to correct for head movement, and co-registered to the anatomical high resolution T1 image. The T1 image was normalized to the Montreal Neurological Institute (MNI) template, with the resultant deformations applied to the co-registered EPI images. Low frequency components were removed with a lenient filter (1/256 as opposed to 1/128) and this filter accommodated longer block lengths (120 s) that were used to induce sustained, as opposed to transient attention (14, 22). Images were resliced to (2 mm3) and smoothed (8 mm FWHM). At the first level, epochs were modeled with boxcar stimulus functions convolved with a canonical hemodynamic response function to form regressors of interest, with the six motion parameters (3 for translation and 3 for rotation) from the co-registration modeled as covariates of no interest. We did not model phasic or event-related responses to targets because we were interested in the responses specifically associated with sustained attention. Images exceeding 4 mm of movement (<1% of all images) were excised from analyses. This approach to image processing and modeling is consistent with recent publications (55, 68, 80–84).
Defining the dynamic causal modeling model space
The regressors of interest representing each of the levels of demand (Low:2-digit; High:3-digit) were submitted to a second-level mixed random effects model (85), with condition and group respectively modeled as repeated and independent factors. To identify an appropriate conjunction of co-activated clusters across groups and conditions, we used Nichols et al. version of the minimum-inference statistic (86)(minimum statistic compared to the conjunction null). These co-activations (depicted in Figure 2) conform to activation profiles identified in previous studies using identical and related paradigms (14, 22, 87). Clearly identifiable peaks are noted in regions including the V1, SPC, thalamus, BG, dACC, and the dPFC. These regions of interest provided a thalamo-cortical network of interest wherein inter-group differences in effective connectivity could be investigated without the confound of activation-differences between groups (41).
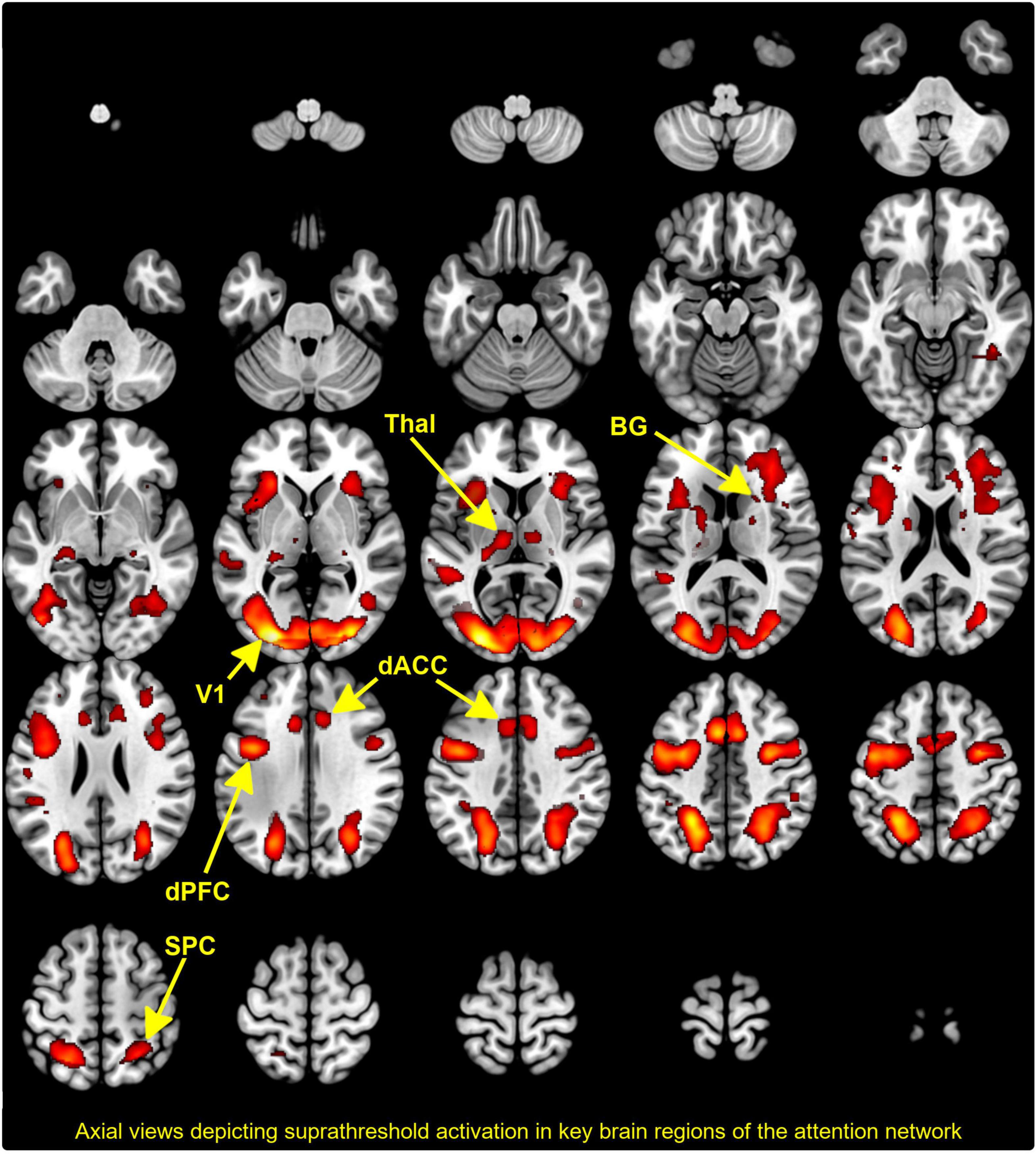
Figure 2. The activation map depicts the results of conjunction analyses across group and condition with co-activated clusters projected to a mosaic of axial views. Supra threshold clusters are clearly seen in the thalamus, basal ganglia (BG), dorsal anterior cingulate cortex (dACC), dorsal prefrontal cortex (dPFC), superior parietal cortex (SPC), and visual cortex (V1). As we note, these sites are consistent with previously published evidence of the engagement of the attention network.
A total of 162 competing network models were configured in the hypothesis/model space. Hypotheses of interest heavily focused on evaluating the contextual modulation of specific pathways during sustained attention with varying levels of demand. Across models eight hypothesis-neutral intrinsic connections were fixed, depicted in Figure 1C by a solid black (curved) line. Four cortical-thalamic intrinsic connections were permuted across the model space represented by solid white lines (Figure 1D): (1) Thal → dPFC; (2) dPFC → Thal; (3) Thal → dACC; and (4) dACC → Thal. We tested whether these four cortical-thalamic connections when present, were contextually modulated by the task (represented by black circles in Figure 1E). Finally, the dPFC → BG connection was permuted across models and when present we tested if it was contextually modulated by the task. This 162-model space was therefore a combination of (a) three possible contextual modulation effects on four permuted intrinsic connections plus, (b) two possible effects on the dPFC → BG connection (34 × 2 = 162 models).
Model estimation
Across each the 62 participants, time series were extracted using spheres (5 mm radius) centered on the peak of the “effects of interest” F-contrast (p < 0.05, adjusted for “effects of no interest”) within each region. These time series were submitted to model estimation, and the cumulative estimated model space (10,044 models, i.e., 162 models across 62 participants) was then submitted to random effects (RFX) Bayesian model selection (BMS), with an initial goal of determining the most likely generative model in each of the OCD and HC groups (Figure 3). A variational Bayes method using the RFX procedure was employed to estimate the posterior probabilities of competing models, resulting in posterior likelihoods associated with each model in the space in each group. RFX is an ideal approach for identifying model likelihood under conditions of sensorimotor or cognitive tasks (14, 26, 41).
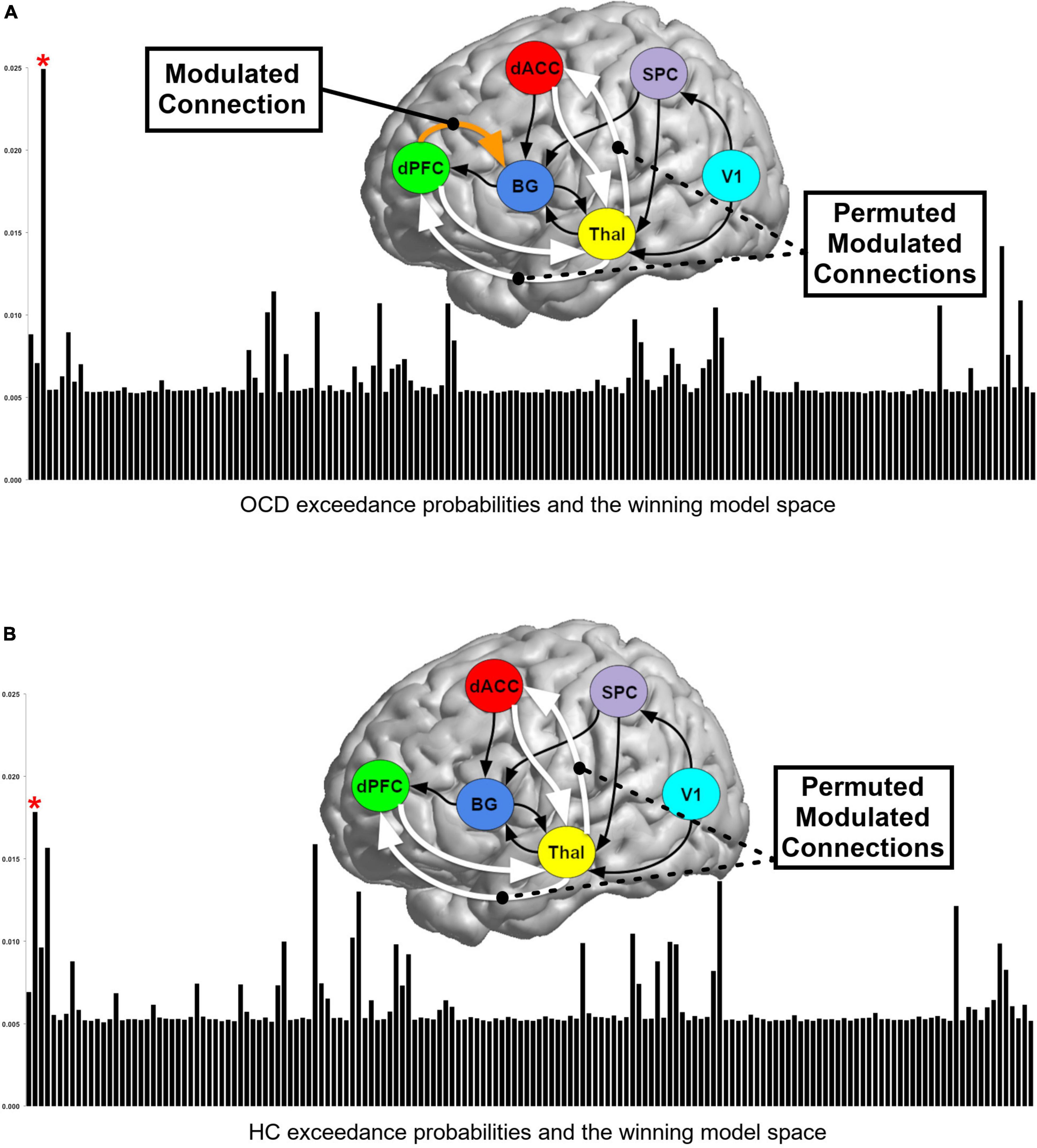
Figure 3. Bayesian model selection (BMS) results for (A) OCD and (B) HC. Exceedance probabilities (relative likelihood) for each of the 162 models generated are depicted in the skyscraper plots for each of the (A) OCD and (B) HC groups. Red asterisks denote models with the highest exceedance probability. BMS revealed slightly different winning models for OCD and HC groups and these differing model structures are depicted above each plot (see text for discussion).
Parameter estimation (for values associated with intrinsic connectivity and contextual modulation) was conducted using Bayesian model averaging (BMA) which generates a weighted average of the parameter estimates of each model within a group, where the weight is determined by the posterior probability of each individual model. BMA is well-optimized for reliably estimating parameter values in relatively large model spaces (88), and in a human psychological context has been hypothesized as being a generally rational approach to decision making (89).
Results
We organize our results as follows: (1) We first present exceedance probabilities across the 162-model space observed for each of the OCD and HC groups (Figure 3); (2) Heat maps are used to depict intrinsic connectivity values (following BMA) for pathways in each of the OCD and HC groups (Figure 4); (3) In Figures 5, 6, we provide histograms of parameters (generated from multiple simulations) associated with the contextual modulation of multiple pathways by each level of attention demand. These distributions motivate speculative inferences about within and inter-group differences in parameter estimates; (4) These speculations are codified in Figure 7 where we identify statistically significant inter-group differences in the contextual modulation of the ascending relay (thalamus during each of the low and high demand task conditions).
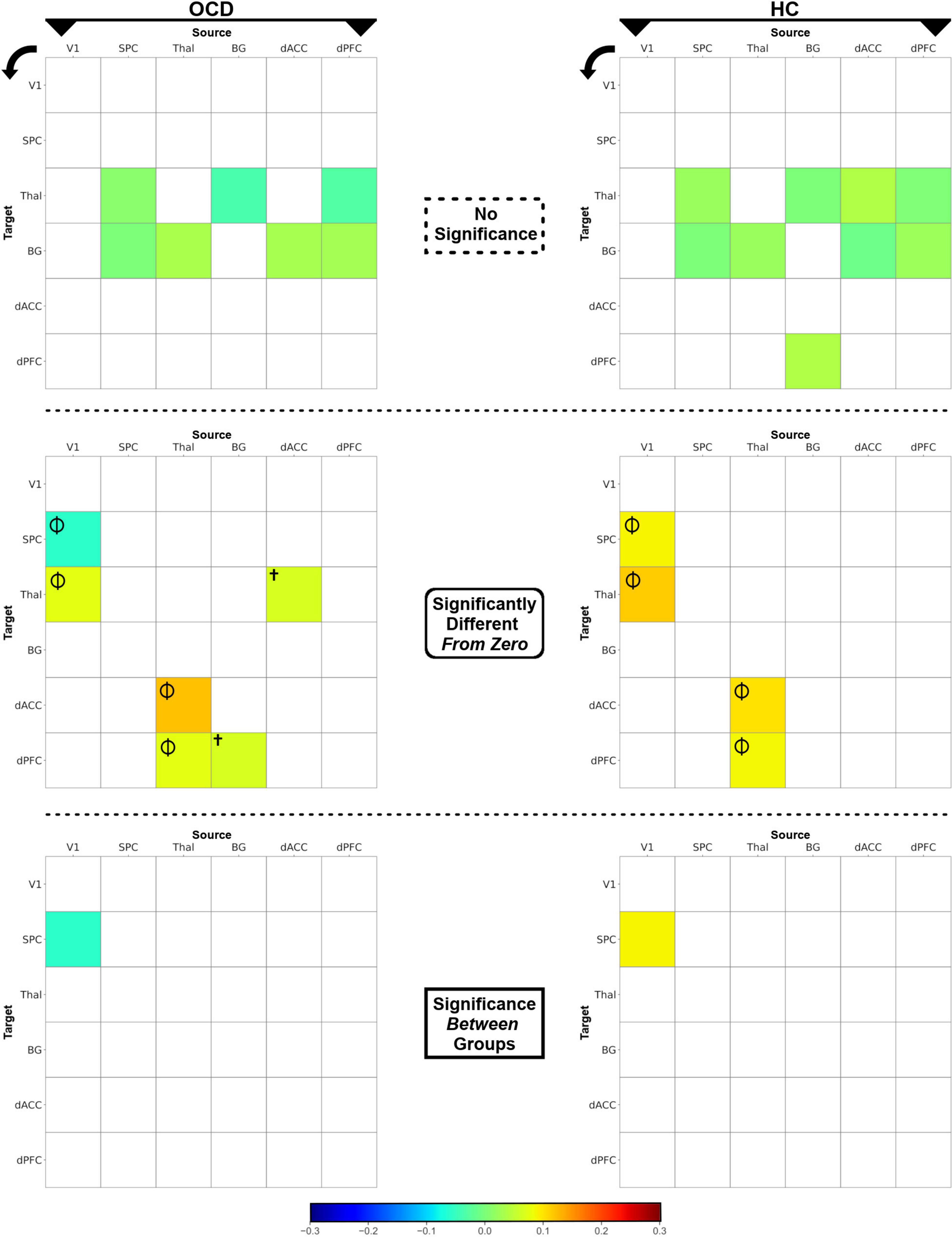
Figure 4. The heat maps depict posterior intrinsic connectivity means for OCD (left) and HC (right) groups. The posterior means are displayed using a blue-to-red color bar and the maps are divided by the nature of the effects (non-significant, different from zero, different between groups. (Top row) Non-significant posterior means are depicted for each of the OCD (left) and HC (right) groups. (Middle row) Posterior means which were significantly different from zero (p < 0.05, one-sample t-tests, i.e., there was significant intrinsic connectivity) are depicted. Effects observed in both groups (Φ) or only in OCD (†) are marked. (Bottom row) The intrinsic connectivity of the V1 → SPC pathway was significantly reduced in OCD, revealed in an independent-samples t-test.
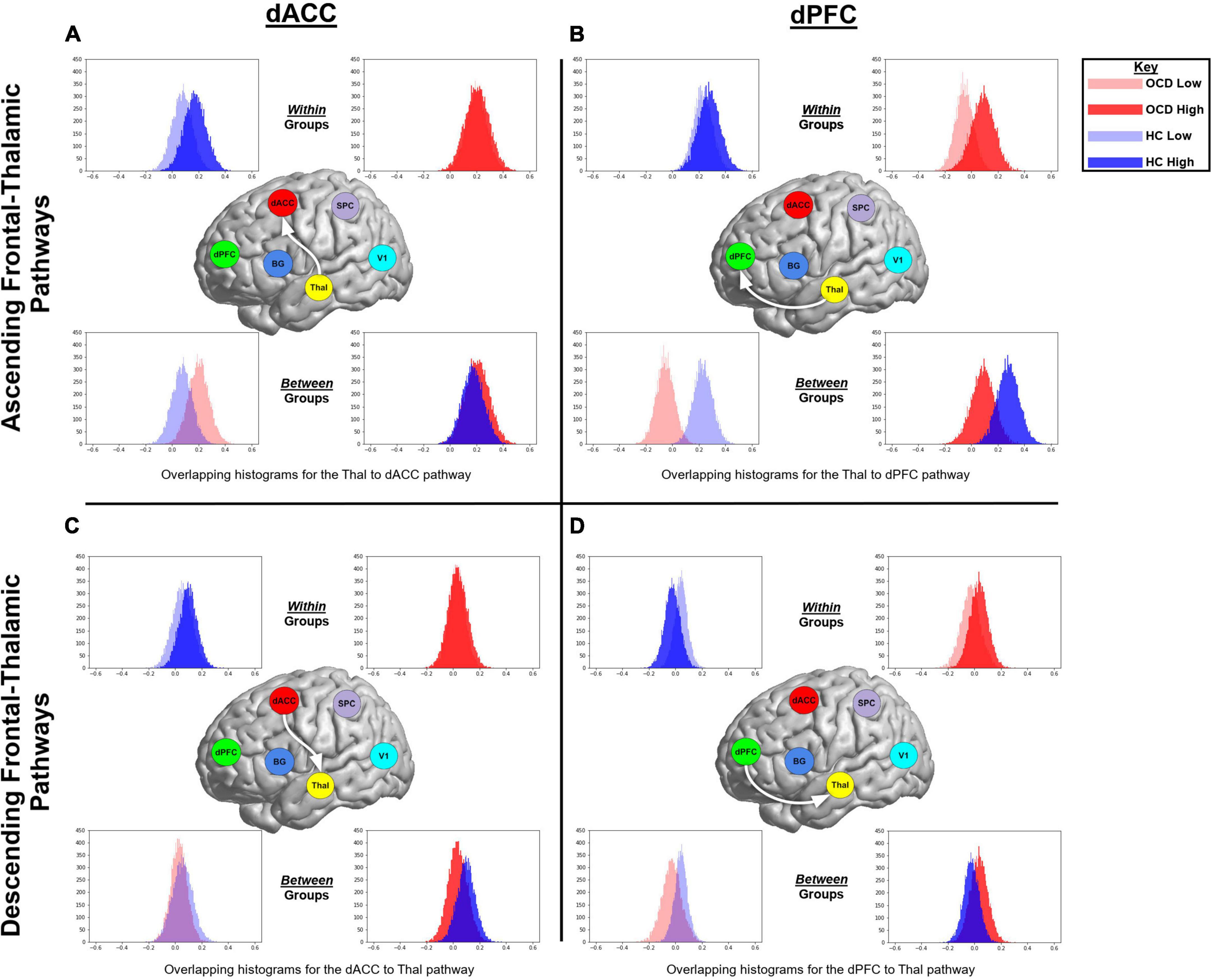
Figure 5. The histograms were generated from extracting 20,000 BMA samples in Occam’s window, and highlight speculative trends associated with within or between group parameter estimates of contextual modulation. Data from OCD are represented in red, while data from HC are in blue. Data from the more demanding attention condition are represented by opaque colors, while data from the less demanding condition in transparent colors. The figure represents ascending and descending fronto-thalamic pathways and is organized in four panels: each column represents pathways involving either the dACC or the dPFC, and each row represents the ascending (from the thalamus) or descending (to the thalamus) pathway. In each panel, histograms are presented twice; initially to depict within group trends in each of the task conditions (top row) and then to depict between group trends in each of the conditions (bottom row).
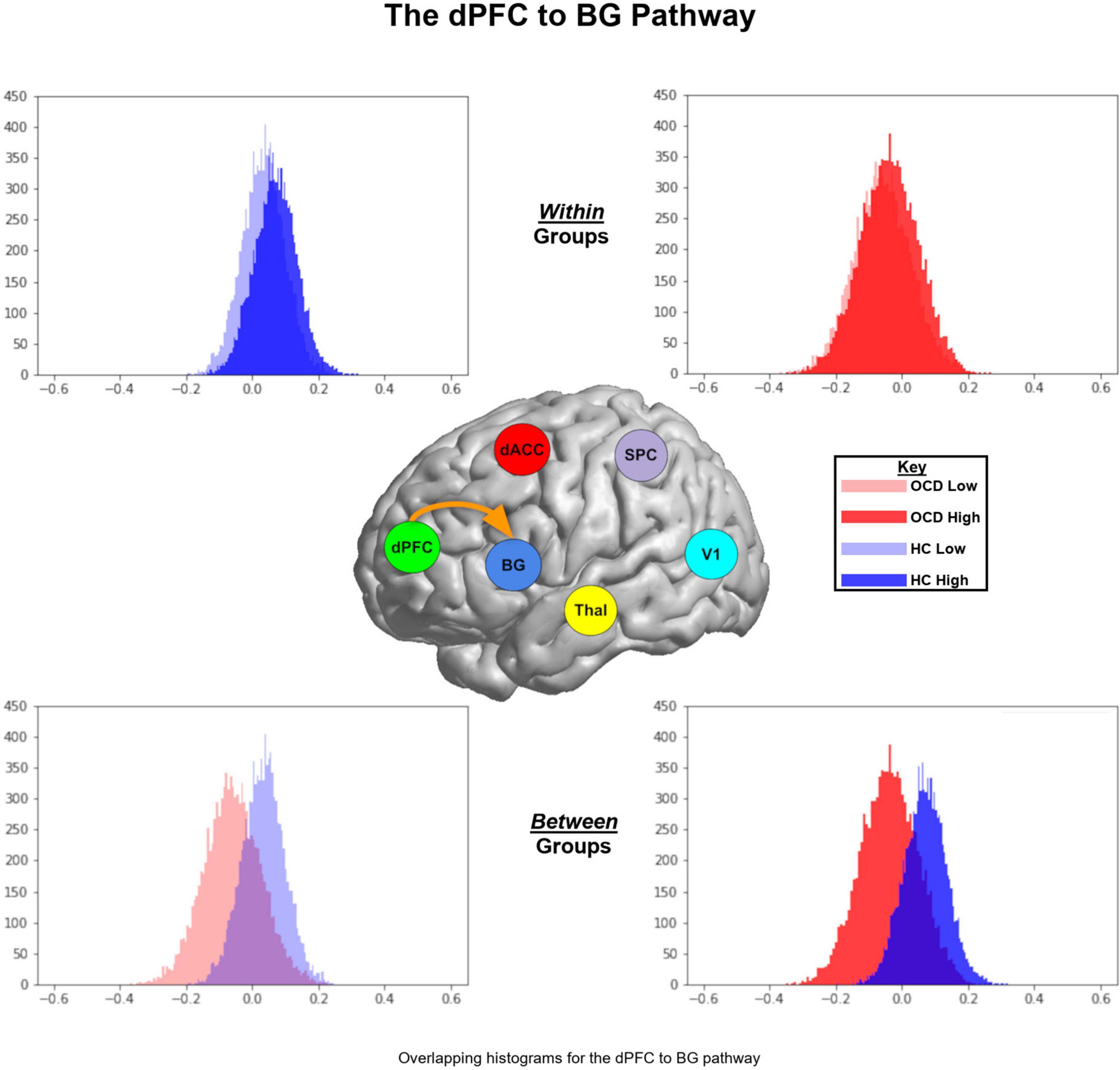
Figure 6. Parameter histograms for the dPFC → BG pathway are presented in similar format as Figure 5. For both low and high levels of demand, the parameter histograms for the HC group are shifted to the right of the OCD group.
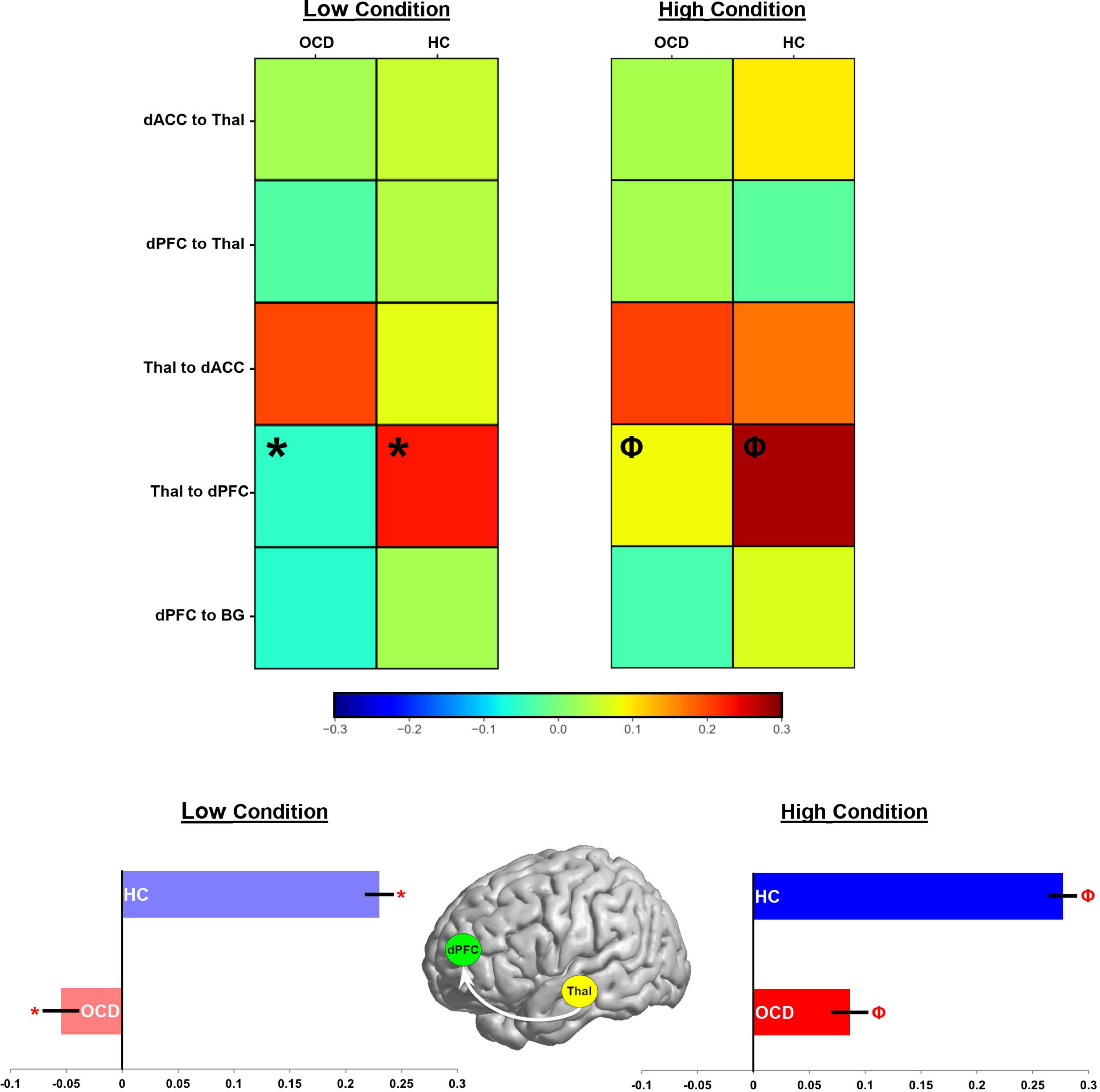
Figure 7. Parameter estimates for the contextual modulation of network pathways are present in heat maps (see color bar for values). Values for the low demand condition are on the left, while those for the high demand condition are on the right. In each map, groups are presented in separate columns (OCD, HC). Independent samples t-tests revealed statistically significant differences between OCD and HC in the Thal → dPFC pathway for both task conditions (Low demand: *; High Demand: Φ). These data are depicted in bar graphs below to accentuate the effect (±sem).
Model selection in DCM
A perusal of the exceedance probabilities (relative likelihood) for each of the 162 competing models in Figure 3 is illustrative. In each group, no single model was characterized by substantially larger likelihood relative to its competitors and the likeliest generative models in the OCD and the HC groups differed slightly. This difference suggests that the effective network structure of task implementation in OCD differed from HC, but the relative proximity of posterior likelihoods motivate the subsequent reliance on BMA for inference (26).
Statistical assessment of intrinsic connectivity parameters
The statistical significance of intrinsic connectivity parameters was comprehensively evaluated using a combination of one-sample t-tests (to identify pathways where parameters in either group were significantly different from zero), and independent samples t-tests (to identify pathways with significant inter-group differences in intrinsic connectivity). For ease of access, the single full matrix in each group is decomposed into a) no significant effects (top row), significantly different (from zero) connectivity parameters (middle row) and significant inter-group differences (bottom row) in Figure 4. Each asymmetric connectivity matrix represents intrinsic connectivity parameters from a source region (column) to a target region (row) with the colors calibrated by the estimated mean value (see color bar). One-sample t-tests indicated that in both OCD and HC groups, four intrinsic connectivity parameters were significantly different from zero: (1) V1 → Thal, (2) Thal → dACC, (3) Thal → dPFC, and (4) V1 → SPC (Φ in Figure 4). Two other intrinsic connectivity parameters were also significantly different from zero, but only in the OCD group: (1) BG → dPFC and (2) dACC → Thal (†in Figure 4). Finally, the results of the independent samples t-tests revealed significant differences in the parameters for the V1 → SPC pathway, indicating that V1 → SPC connectivity was lower in OCD. Statistical information is provided in Table 2.
Statistical assessment of contextual modulation parameters
BMA was used to weight parameters over the 162 generative model architectures based on the posterior probability of models across a group. Thus, the likelihood of each hypothesis is represented in a quantitative estimate of the parameters for variables associated with that hypothesis. For an initial assessment, histograms were generated from extracting 2 × 104 BMA samples in Occam’s window. These were used to highlight speculative trends associated with within or between group parameter estimates of contextual modulation of the relevant pathways by each of the attention conditions. These histograms are presented in Figures 5, 6. In each figure, data from the OCD group are represented in red, while data from the HC group are in blue. Data from the more demanding attention condition are represented by opaque colors, while data from the less demanding condition in transparent colors. Figure 5 represents ascending and descending fronto-thalamic pathways and is organized in four panels: each column represents pathways involving either the dACC or the dPFC, and each row represents the ascending (from the thalamus) or descending (to the thalamus) pathway. In each panel, histograms are presented twice; initially to depict within group trends in each of the task conditions (top row) and then to depict between group trends in each of the conditions (bottom row).
Figure 5A (top left panel) depicts two interesting trends associated with the ascending Thal → dACC pathway: (1) As seen, within the HC (but not OCD) group, the parameter histogram associated with high demand is shifted to the right. This suggests that in healthy controls, an increase in attention demand effects an increase in connectivity of this ascending pathway; (2) Between groups, the histogram for HC is shifted to the left of the OCD group. This effect is a between-group extension of the previously noted within-group effect. In Figure 5B (top right panel), two interesting trends characterize the Thal → dPFC pathway: (1) Within OCD, the histogram of values for the high demand attention condition is shifted to the right; (2) When inspecting data between groups, we observe that for both the low and the high demand conditions, the parameter histograms in HC are shifted to the right of those in OCD. This effect suggests that there is a general increase in the contextual modulation of this ascending relay in HC compared to OCD (or a decrease in OCD compared to HC). In Figure 5C (bottom left), the histograms for the dACC → Thal descending relay suggests that the high demand condition induces an overall larger, positive contextual modulation in the sample means in HC. Finally, in Figure 5D (bottom right panel), parameter histograms for the dPFC → Thal descending relay suggest that for the low demand condition, the parameter histogram for HC is shifted to the right of that for the OCD group. However, for the high demand condition, we observed a reversal of this effect. Figure 6 depicts parameter histograms for the dPFC → BG pathway revealing that for both low and high levels of demand, the parameter histograms for the HC group are shifted to the right of the OCD group.
The effects depicted in Figures 5, 6 are suggestive, but motivated more robust parametric analyses in the service of inference. Accordingly, parameter estimates across participants and groups were submitted to independent sample t-tests to identify pathways with significant inter-group differences. Figure 7 (top) uses a heat map to visualize parameter means in each of the OCD and HC groups. Data are separated into each of the low and high demand conditions. Cells marked by symbols constitute a member of a pair with significant differences in contextual modulation (see Table 3 for details). Two effects were significant, both observed on the ascending Thal → dPFC relay. Both the low and the high attention demand conditions induced greater contextual modulation in HC compared to OCD. These significant effects were pulled from the heat maps with the group means visualized in the bar graphs in Figure 7 (bottom) (±sem).
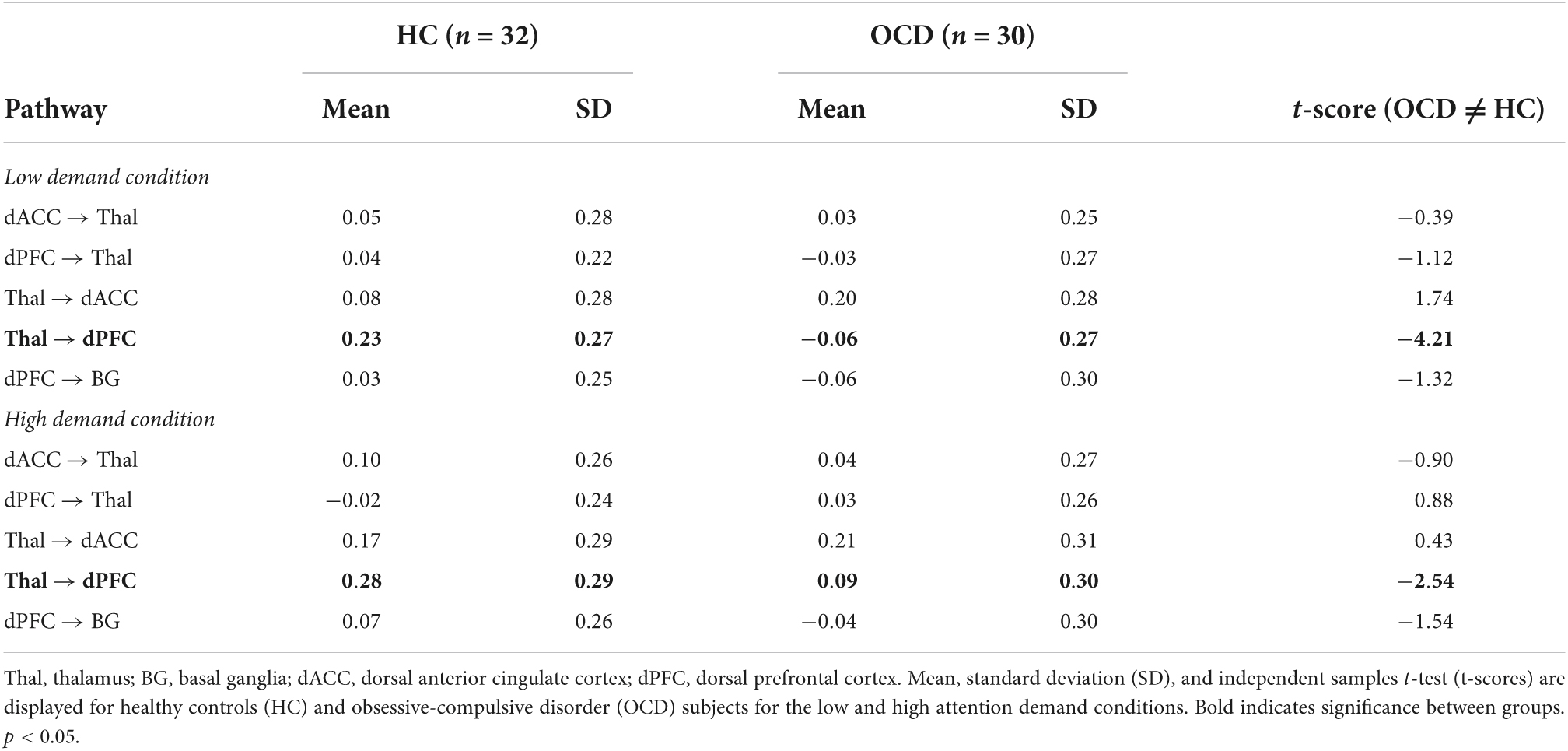
Table 3. Parameters for contextual modulation and statistical analyses (significant effects in bold).
Discussion
In this study, we utilized DCM to assess the impact of sustained attention at varying levels of demand on the effective connectivity of cortical-striatal-thalamic pathways in OCD and HC. The DCM investigations were underpinned by a complex hypothesis-driven model space with 162 competing models/hypotheses. Across the space, we evaluated (a) the likelihood of specific intrinsic connections being present, and (b) whether task conditions modulated the connectivity of ascending and descending frontal-thalamic pathways. Bayesian model selection (BMS) was employed to sift between the predictive models for each group, with a focus of the analyses relying on Bayesian model averaging (BMA) to evaluate group differences in weighted posterior parameter estimates. This approach provides a more inclusive, albeit weighted approach toward the contributions of the different models to our results (88).
From these investigations, we discuss two principal results: (1) Analyses of parameter estimates revealed that the V1 → SPC pathway showed significantly weaker intrinsic connectivity in OCD (Figure 4, bottom row); (2) Most compellingly, task conditions during sustained attention evoked statistically significant differences (OCD < HC) for the ascending Thal → dPFC relay (Figure 7), a central pathway through which thalamic inputs innervate the cortex (90). These highly circumscribed results reaffirm the contextual bases of sensorimotor processes in brain network function (6, 91). Moreover, they motivate two sets of inferences that are related to relatively early sensory and visual pathways. The first is that in OCD, contextual modulation of the ascending Thal → dPFC pathway during sustained attention is greatly reduced. This constitutes evidence for task-induced alterations in the functionality of this ascending relay. This deficit is observed even as contextual modulation of other pathways across the CST connective circuitry remained largely intact. Secondly, portions of the dorsal visual pathway show reduced intrinsic connectivity in OCD. This reduction is tentative evidence in support of the hypothesis that early sensory processing might be impaired in OCD (92, 93). The approach and results highlight the value of DCM in the discovery of intact and dysfunctional network structure (94), and our observations of functional loss provide previously unknown insights on how behavioral context evokes circumscribed pathology in the OCD brain. In the remainder of the paper, we discuss the putative import of the observed effects and their relevance for our understanding of the brain network expressions of OCD.
A loss of intrinsic connectivity of the dorsal pathway and its relevance for obsessive-compulsive disorder
The dorsal visual pathway has long been presumed to play a key role in the transmission of spatial aspects of visual processing in the service of higher sensori-motor function (49). The V1 receives visual sensory information via the lateral geniculate nucleus (LGN) of the thalamus before sending projections to subsequent brain regions (95). Likewise, the SPC is also innervated by thalamic connections (27, 40, 50) and functions in the allocation of spatial attention. How does visual information interface between occipital and parietal cortices? As noted, current understanding rests on the two-streams (dorsal and ventral) as being the purported mechanism of action (96). The dorsal visual stream (i.e., V1→ SPC) functions in computing spatial relationships and orienting of visuospatial attention, often referred to as the “where” pathway (48, 97, 98). In contrast, the ventral visual stream functions in the recognition of objects and faces, often described as the “what” pathway (48, 97, 98).
Evidence from in vivo MRI studies tends to be consistent with these theoretical motivations. Resting state functional connectivity studies have shown that connectivity between the V1 and superior parietal lobe (SPL) is predictive of visual search efficiency which relies heavily on the spatial components of vision (99). Thus, visual search tasks induce greater engagement of brain regions that are part of the dorsal visual stream including the occipital cortex, the SPL and occipital cortex, and beyond including the dACC, and inferior frontal cortex. Moreover, participants who are more effective in visual search show stronger functional connectivity between the SPL and visual cortex. Thus, a finding of weaker intrinsic connectivity of the V1 → SPC pathway implies that in OCD there may be reduced ability of the dorsal visual stream to effectively transmit spatial information from the V1. Is there evidence for visuo-spatial processing deficits in OCD?
Indeed, existing studies have frequently described problems with visuo-spatial processing in OCD patients (100, 101). For example, poor fine-motor and visuospatial skills in pediatric OCD appear to predict the presence of the disorder well into adulthood (102). In fact, deficits in visual memory, especially when complex visual stimuli are involved, is one of the most commonly reported dysfunctional cognitive domains in OCD (103–105). One meta-analysis of cognitive functioning in OCD showed that OCD patients experience impairments in visuospatial memory, visual organizational skill, and executive functions such as planning ability (105). Other studies on OCD have also shown deficits in spatial working memory and verbal/nonverbal recall (104, 106, 107). A few early studies on youth with Tourette’s syndrome and/or ADHD disorders revealed slowed reaction times on continuous performance tasks, implying a reduced ability of subjects to remain vigilant compared to controls (108, 109). Employing numerous neurocognitive tasks (e.g., Finger Windows task), Chang et al showed spatial attention deficits in OCD youth (110); this result was consistent with prior adult OCD studies on memory, spatial attention, and other executive functions (106, 111, 112). Our observations of reduced intrinsic connectivity of the V1→ SPC are indeed consistent with these results.
A loss of contextual modulation of the ascending relay in obsessive-compulsive disorder
Sensory inputs and outputs to the thalamic nuclei can be subdivided into first-order relays and higher-order relays. In first-order relays, thalamic nuclei receive input from sensory organs (i.e., the eye) before relaying information to the cortex. For instance, visual information from the retina is relayed from the LGN of thalamus to the V1 before further processing of visual information can occur. In higher-order relays, thalamic nuclei receive descending inputs from cortical regions before relaying and controlling the flow of information back to the cortex, forming cortico-thalamo-cortical pathways (31–33). Studies on the lateral pulvinar nucleus’ role in cortical processing of visual information further supports the expanded modulatory role of some thalamic nuclei; removing lateral pulvinar input to the V1 nearly extinguishes “visual responses in the primary visual cortex,” suggesting that the pulvinar nucleus plays a critical and integral role in the functioning of the visual cortex (31). Due to relaying more “processed” inputs flowing back to the cortex, higher-order relays have been viewed as taking a more active and modulatory role in routing inputs (113).
Because groups of thalamic nuclei are involved in relaying and controlling the flow of sensory and motor information to the cortex, the thalamus has been dubbed the “relay station” or “gatekeeper” of cortical-bound information (27, 28, 114, 115). Thalamic inputs to the dPFC assume particular importance, given this heteromodal structure’s role in a multiplicity of processing domains including working memory, selective, divided, and sustained attention, and executive function (43, 116). Far from being a passive relay in the bottom-up gating of attentional (and other sensory) information to the cortex, the thalamus is now thought to exert more constructive influences on the dynamic upward flow of sensory information. In addition to a vast array of cellular studies (117–120), this framework has also been confirmed by DCM studies showing that ascending relays from the thalamus to the dPFC are contextually modulated by sustained attention (22). Such DCM based studies affirm the importance of ascending relays in the functional innervation of cortical networks at the macroscopic scale. Our observations of reduced modulation of this ascending relay in OCD is suggestive of a contextually induced thalamo-cortical deficit in network function. What might the impact of such a deficit be? Before addressing this question, we briefly review extant understanding of the functional role of thalamo-cortical relays.
Both ascending and descending thalamo-cortical relays are necessary to balance the flow of ascending sensory inputs to the cortex against the descending modulation of these inputs. Ascending relays regulate the flow of sensory information to the cortex, whereas descending relays (dPFC → Thal) can directly excite or inhibit thalamic relay neurons via GABAergic neurons of the thalamic reticular nucleus (TRN), thus dynamically shaping the ascending inputs (121–123). The TRN provides inhibitory innervation to fellow thalamic nuclei while modulating the flow of sensory information to the cortex, as well as “influencing ongoing cortical activity by modulating cortico-thalamo-cortical transmission” (124, 125). As early as 1984, Francis Crick hypothesized an attentional role for the TRN, suggesting “if the thalamus is the gateway to the cortex, the reticular complex might be described as the guardian of the gateway”(126). Later, lesion studies on the TRN have shown impairments in the cognitive domain of attention (127, 128). Moreover, attention modulates visual processing via a reciprocal relationship between increasing LGN responses and decreasing neuronal TRN responses (30), consistent with Crick’s proposals. Thus, a vast connectivity network and polysynaptic receptors allowing tonic and burst modes of firing (129), regulate how the intrinsic circuitry dynamically modifies cortico-thalamic synchronization. This synchronization in turn is seen as a major functional basis for multiple cognitive functions (130). Unsurprisingly, a loss of this functional synchronization, particularly between cortical, striatal and thalamic circuits has been proposed to underlie OCD (131, 132) and has been documented in studies of resting state and task-based functional connectivity (55, 133, 134). Moreover, numerous studies implicate a broad range of neurocognitive deficits in attention, working memory, inhibition, and executive functions deficits in OCD (135). These have been observed during attention-related task paradigms such as the Trail-Making and Stroop Tasks and measurements of attentional set shifting, reversal learning, and cued tasked switching (136, 137). OCD patients also show hypo-activity in the dPFC as well as other PFC structures such as the OFC during reversal learning and task switching paradigms (51, 62, 138). From these results, Ahmari & Rauch have suggested that OCD subjects performing tasks with changing conditions “display less recruitment of multiple PFC structures” compared to controls. Furthermore, studies using the Tower of London task, a test which assesses goal-directed planning have also found hypo activation and slowed reaction times of the dPFC, suggesting a decrease in “dorsal prefrontal-striatal responsiveness” (138, 139).
Evidence of a loss of contextual modulation of the ascending relay falls firmly within this emerging framework. As we have noted, effective connectivity methods provide particular insight on the “causative” bases of inter-network effects (4, 9). They offer the possibility of identifying task-induced specificity in evoked network deficits. Such evidence is informative about how disease-related traits are manifested in functional brain states that are themselves transient and frequently task specific (91, 140). Such an approach may illuminate understanding of the mechanistic basis for pathology in neuropsychiatric conditions (2). We suggest that the reduced modulation of the ascending relay regardless of attention demand (Figure 7) reflects a basic loss of network tone evoked by sustained attention (22). It is plausible that this deficit reflects a relative loss of cortical innervation in OCD that subsequently impairs CST function (56), and that it provides functional evidence for increasingly clear impairments of thalamic structure in OCD (141). While circuit hyper-activity may be a trait emergent aspect of OCD (142), this may impact state-related network interactions that underlie basic processes such as attention. Thus, these deficits may affect how sensory information propagates to the cortex, where maladaptive inputs impact the fidelity of cortical network function in the disorder. This loss of network fidelity leads to a degradation of internal signals in the OCD brain, results in the emergence of erroneous perceptions (“something is wrong”), and eventually drives the anxieties that are characteristic of the disorder (143) and that drive trait-related hyperactivity.
The current study was partly motivated by prior investigations on the contextual modulation of cortico-thalamic pathways by attention demand (22), and which utilized a similar approach albeit only in healthy adolescents. That study used a winner-take-all approach from Bayesian model selection (26, 41), subsequently using Bayesian Parameter Averaging to estimate connectivity parameters from the likeliest generative model architecture. By comparison, BMA as employed in the current investigation is used to weight connectivity parameter estimates across all models, based on each model’s posterior probability. BMA is suitable for estimating parameters values for large model spaces (88), avoids uncertainties of model selection and mitigates any brittleness associated with parameter estimation (144). These differences in DCM-related methodology, as well as the focus on inter-group comparisons (as opposed to intra-group effects) may account for some differences between the sets of results from the two studies. For instance, a critical difference was that Jagtap and Diwadkar (22) noted differential effects of demand on the contextual modulation of the descending dPFC → Thal pathway (High Demand > Low Demand), suggestive of the role of frontally driven attention gain on the thalamus when demand for attention-resources was high. This effect was not replicated in the current study (see Figure 5), though our results on the ascending relay (significant contextual modulation for both low and high attention demand) in healthy controls (Figures 5, 7) was consistent with observations from the previous work.
Effective connectivity studies are beginning to illuminate understanding about casual brain network interactions in OCD. For example, using a reward learning task, Alves-Pinto et al., showed alterations in intrinsic connectivity and contextual modulation of fronto-striatal circuits, mainly from the ventromedial PFC (vmPFC) to the left and right orbitofrontal cortex (OFC) (145). In another study, Schlösser and colleagues used a Stroop color-word task to elicit aberrant effective connectivity pathways between OCD and HC in the fronto-cingulate system, consistent with an over-active error control system during decision-making observed in OCD (13). Han et al., showed that negative emotional distraction (working memory task) induced significantly reduced contextual modulation in the dorsolateral PFC (DLPFC) to OFC pathway in OCD compared to HC (146). Finally, van Velzen and colleagues have documented altered directionality of the connectivity from the amygdala to the OFC in OCD participants during a stop signal task (12).
Limitations and conclusion
Understanding the relationship between behavior and brain network dynamics is perhaps the central challenge in human neuroscience (147) and the thalamo-cortical network appears to play a central role in mediating this relationship (148). Our focus on thalamo-cortical networks in OCD (1) reflects understandable interest in the role of the thalamus in the disorder (149), and (2) acknowledges the value of methods like DCM in revealing task-induced “mechanistic” deficits in brain networks (68). However, several limitations to our intriguing results should be acknowledged. Firstly, task-based fMRI results provide crucial information about demand-induced modulation of network connectivity and its relevance for psychiatric conditions in ways that resting state studies cannot (10). However, understanding specifically how impairments in micro or macro-circuits in the brain explain mental disorders remains a significant open problem in clinical neuroscience (150) and one that limits interpretations from a majority of fMRI studies including our own. Secondly, an absence of longitudinal data limits our ability to comment on the long-term impact of our observed connectivity deficits on the course of OCD symptomatology. Finally, as an exploration on putative mechanisms (see Introduction), we are not in a position to comment on whether treatment (or time) results in an amelioration of our observed connectivity impairments. This brief list of limitations is far from exhaustive, but serves as a road map for potential future investigations in the clinical neuroscience of the disorder.
In conclusion, large scale meta-analyses of network function are challenging given that network interactions are contextually tuned by task characteristics (151). However, we suggest that a growing corpus of studies on the effective (dys)connectivity of brain networks in OCD can contribute to understanding of the task conditions under which network dysfunction is evoked in the illness. Moreover, mechanism-based markers of dysfunction can also serve as therapeutic targets for long term intervention.
Data availability statement
The original contributions presented in this study are included in the article/supplementary material, further inquiries can be directed to the corresponding author.
Ethics statement
The studies involving human participants were reviewed and approved by Wayne State University IRB. Written informed consent to participate in this study was provided by the participants or their legal guardian/next of kin.
Author contributions
MY conducted the analyses, created the figures, and wrote the manuscript. AC assisted in analyses and statistical methods. PE assisted in data collection and clinical analyses. GH assisted in clinical characterization and data collection. DR assisted in clinical characterization, data collection, and study design. VD oversaw all aspects of the analyses, writing, and presentation. All authors contributed to the article and approved the submitted version.
Funding
This work was supported by the National Institute of Mental Health (MH059299), the Children’s Foundation of Michigan, Cohen Neuroscience Endowment, the Prechter World Bipolar Foundation, the Lykacki-Young Fund from the State of Michigan, the Miriam Hamburger Endowed Chair of Psychiatry, the Paul and Anita Strauss Endowment, the Donald and Mary Kosch Foundation, the Elliott Luby Endowed Professorship, and the Detroit Wayne County Authority. The funding agencies played no role in the analyses or reporting of the data.
Conflict of interest
The authors declare that the research was conducted in the absence of any commercial or financial relationships that could be construed as a potential conflict of interest.
Publisher’s note
All claims expressed in this article are solely those of the authors and do not necessarily represent those of their affiliated organizations, or those of the publisher, the editors and the reviewers. Any product that may be evaluated in this article, or claim that may be made by its manufacturer, is not guaranteed or endorsed by the publisher.
Footnotes
References
1. Valleni-Basile LA, Garrison CZ, Waller JL, Addy CL, McKeown RE, Jackson KL, et al. Incidence of obsessive-compulsive disorder in a community sample of young adolescents. J Am Acad Child Adolesc Psychiatry. (1996) 35:898–906. doi: 10.1097/00004583-199607000-00015
2. Diwadkar VA, Eickhoff SB. Brain network dysfunction in neuropsychiatric illness: Methods, applications and implications. New York, NY: Springer Nature (2021).
3. Tottenham N, Sheridan MA. A review of adversity, the amygdala and the hippocampus: A consideration of developmental timing. Front Hum Neurosci. (2009) 3:68. doi: 10.3389/neuro.09.068.2009
4. Silverstein B, Bressler S, Diwadkar VA. Inferring the dysconnection syndrome in schizophrenia: Interpretational considerations on methods for the network analyses of fMRI data. Front Psychiatry. (2016) 7:132. doi: 10.3389/fpsyt.2016.00132
5. Albert U, Marazziti D, Di Salvo G, Solia F, Rosso G, Maina G. A systematic review of evidence-based treatment strategies for obsessive- compulsive disorder resistant to first-line pharmacotherapy. Curr Med Chem. (2018) 25:5647–61. doi: 10.2174/0929867325666171222163645
6. Mannino M, Bressler SL. Foundational perspectives on causality in large-scale brain networks. Phys Life Rev. (2015) 15:107–23.
7. Hameroff S. Consciousness, cognition and the neuronal cytoskeleton – A new paradigm needed in neuroscience. Front Mol Neurosci. (2022) 15:869935. doi: 10.3389/fnmol.2022.8699
8. Friston KJ, Fagerholm ED, Zarghami TS, Parr T, Hipolito I, Magrou L, et al. Parcels and particles: Markov blankets in the brain. Netw Neurosci. (2021) 5:211–51. doi: 10.1162/netn_a_00175
10. Diwadkar VA, Eickhoff SB. Brain network dysfunction in neuropsychiatric disorders: The practice of “normal science”. In: Diwadkar VA, Eickhoff SB, editors. Brain network dysfunction in neuropsychiatric illness: Methods, applications, implications. New York, NY: Springer International Publishing (2021). p. 1–15.
11. Xu FF, Han L, He HJ, Zhu YH, Zhong JH. [Effective connectivity within the default mode network modulated by methylphenidate using dynamic causal modeling on resting-state functional magnetic resonance imaging]. Sheng Li Xue Bao. (2016) 68:255–64.
12. van Velzen LS, de Wit SJ, Curcic-Blake B, Cath DC, de Vries FE, Veltman DJ, et al. Altered inhibition-related frontolimbic connectivity in obsessive-compulsive disorder. Hum Brain Mapp. (2015) 36:4064–75.
13. Schlosser RG, Wagner G, Schachtzabel C, Peikert G, Koch K, Reichenbach JR, et al. Fronto-cingulate effective connectivity in obsessive compulsive disorder: A study with fMRI and dynamic causal modeling. Hum Brain Mapp. (2010) 31:1834–50. doi: 10.1002/hbm.20980
14. Diwadkar VA, Bakshi N, Gupta G, Pruitt P, White R, Eickhoff SB. Dysfunction and dysconnection in cortical-striatal networks during sustained attention: Genetic risk for schizophrenia or bipolar disorder and its impact on brain network function. Front Psychiatry. (2014) 5:50. doi: 10.3389/fpsyt.2014.00050
16. Diwadkar VA. Adolescent risk pathways toward schizophrenia: Sustained attention and the brain. Curr Top Med Chem. (2012) 12:2339–47. doi: 10.2174/156802612805289962
17. Lucke IM, Lin C, Conteh F, Federline A, Sung H, Specht M, et al. Continuous performance test in pediatric obsessive-compulsive disorder and tic disorders: The role of sustained attention. CNS Spectr. (2015) 20:479–89. doi: 10.1017/S1092852914000467
18. Fortenbaugh FC, DeGutis J, Esterman M. Recent theoretical, neural, and clinical advances in sustained attention research. Ann N Y Acad Sci. (2017) 1396:70–91. doi: 10.1111/nyas.13318
19. Chaves A, Arnaez S, Roncero M, Garcia-Soriano G. Teachers’ knowledge and stigmatizing attitudes associated with obsessive-compulsive disorder: Effectiveness of a brief educational intervention. Front Psychiatry. (2021) 12:677567. doi: 10.3389/fpsyt.2021.677567
20. Langner R, Eickhoff SB. Sustaining attention to simple tasks: A meta-analytic review of the neural mechanisms of vigilant attention. Psychol Bull. (2013) 139:870–900. doi: 10.1037/a0030694
21. Mitchell AS, Sherman SM, Sommer MA, Mair RG, Vertes RP, Chudasama Y. Advances in understanding mechanisms of thalamic relays in cognition and behavior. J Neurosci. (2014) 34:15340–6.
22. Jagtap P, Diwadkar VA. Effective connectivity of ascending and descending frontalthalamic pathways during sustained attention: Complex brain network interactions in adolescence. Hum Brain Mapp. (2016) 37:2557–70. doi: 10.1002/hbm.23196
23. Fisher AV. Selective sustained attention: A developmental foundation for cognition. Curr Opin Psychol. (2019) 29:248–53.
24. Butterworth B. The development of arithmetical abilities. J Child Psychol Psychiatry Allied Discipl. (2005) 46:3–18.
25. Diwadkar VA, Sweeney JA, Boarts D, Montrose DM, Keshavan MS. Oculomotor delayed response abnormalities in young offspring and siblings at risk for schizophrenia. CNS Spectr. (2001) 6:899–903. doi: 10.1017/s109285290000095x
26. Stephan KE, Penny WD, Moran RJ, den Ouden HE, Daunizeau J, Friston KJ. Ten simple rules for dynamic causal modeling. Neuroimage. (2010) 49:3099–109.
27. Jang SH, Lim HW, Yeo SS. The neural connectivity of the intralaminar thalamic nuclei in the human brain: A diffusion tensor tractography study. Neurosci Lett. (2014) 579:140–4. doi: 10.1016/j.neulet.2014.07.024
28. Wolff M, Vann SD. The cognitive thalamus as a gateway to mental representations. J Neurosci. (2019) 39:3–14.
29. Brunia CH. Waiting in readiness: Gating in attention and motor preparation. Psychophysiology. (1993) 30:327–39. doi: 10.1111/j.1469-8986.1993.tb02054.x
30. McAlonan K, Cavanaugh J, Wurtz RH. Guarding the gateway to cortex with attention in visual thalamus. Nature. (2008) 456:391–4. doi: 10.1038/nature07382
31. Purushothaman G, Marion R, Li K, Casagrande VA. Gating and control of primary visual cortex by pulvinar. Nat Neurosci. (2012) 15:905–12. doi: 10.1038/nn.3106
32. Saalmann YB, Pinsk MA, Wang L, Li X, Kastner S. The pulvinar regulates information transmission between cortical areas based on attention demands. Science. (2012) 337:753–6. doi: 10.1126/science.1223082
33. Theyel BB, Llano DA, Sherman SM. The corticothalamocortical circuit drives higher-order cortex in the mouse. Nat Neurosci. (2010) 13:84–8. doi: 10.1038/nn.2449
35. Halassa MM, Sherman SM. Thalamocortical circuit motifs: A general framework. Neuron. (2019) 103:762–70. doi: 10.1016/j.neuron.2019.06.005
36. Petersen SE, Posner MI. The attention system of the human brain: 20 years after. Annu Rev Neurosci. (2012) 35:73–89.
37. Fan J, Hof PR, Guise KG, Fossella JA, Posner MI. The functional integration of the anterior cingulate cortex during conflict processing. Cereb Cortex. (2008) 18:796–805.
38. Posner MI, Rothbart MK, Sheese BE, Tang Y. The anterior cingulate gyrus and the mechanism of self-regulation. Cogn Affect Behav Neurosci. (2007) 7: 391–5.
39. Bakshi N, Pruitt P, Radwan J, Keshavan MS, Rajan U, Zajac-Benitez C, et al. Inefficiently increased anterior cingulate modulation of cortical systems during working memory in young offspring of schizophrenia patients. J Psychiatr Res. (2011) 45:1067–76. doi: 10.1016/j.jpsychires.2011.01.002
40. Bush G. Attention-deficit/hyperactivity disorder and attention networks. Neuropsychopharmacology. (2010) 35:278–300.
41. Diwadkar VA, Wadehra S, Pruitt P, Keshavan MS, Rajan U, Zajac-Benitez C, et al. Disordered corticolimbic interactions during affective processing in children and adolescents at risk for schizophrenia revealed by functional magnetic resonance imaging and dynamic causal modeling. Arch Gen Psychiatry. (2012) 69:231–42. doi: 10.1001/archgenpsychiatry.2011.1349
42. Paus T. Primate anterior cingulate cortex: Where motor control, drive and cognition interface. Nat Rev Neurosci. (2001) 2:417–24. doi: 10.1038/35077500
43. Duncan J, Owen AM. Common regions of the human frontal lobe recruited by diverse cognitive demands. Trends Neurosci. (2000) 23:475–83.
44. Hikosaka O, Takikawa Y, Kawagoe R. Role of the basal ganglia in the control of purposive saccadic eye movements. Physiol Rev. (2000) 80:953–78. doi: 10.1152/physrev.2000.80.3.953
45. Schroll H, Hamker FH. Computational models of basal-ganglia pathway functions: Focus on functional neuroanatomy. Front Syst Neurosci. (2013) 7:122. doi: 10.3389/fnsys.2013.00122
46. Maia TV, Frank MJ. From reinforcement learning models to psychiatric and neurological disorders. Nat Neurosci. (2011) 14:154–62.
47. Haber SN. The primate basal ganglia: Parallel and integrative networks. J Chem Neuroanat. (2003) 26:317–30.
48. Creem SH, Proffitt DR. Defining the cortical visual systems: “What”, “where”, and “how”. Acta Psychol (Amst). (2001) 107:43–68.
49. Mishkin M, Ungerleider LG, Macko KA. Object vision and spatial vision: Two cortical pathways. Trends Neurosci. (1983) 6:414–7.
50. Modha DS, Singh R. Network architecture of the long-distance pathways in the macaque brain. Proc Natl Acad Sci U.S.A. (2010) 107:13485–90.
51. Gu BM, Park JY, Kang DH, Lee SJ, Yoo SY, Jo HJ, et al. Neural correlates of cognitive inflexibility during task-switching in obsessive-compulsive disorder. Brain. (2008) 131(Pt 1):155–64.
53. Strauss E, Sherman EMS, Spreen O. A compendium of neuropsychological tests : Administration, norms, and commentary. 3rd ed. Oxford: Oxford University Press (2006).
54. Page LA, Rubia K, Deeley Q, Daly E, Toal F, Mataix-Cols D, et al. A functional magnetic resonance imaging study of inhibitory control in obsessive-compulsive disorder. Psychiatry Res. (2009) 174:202–9.
55. Meram TD, Chowdury A, Easter P, Attisha T, Kallabat E, Hanna GL, et al. Evoking network profiles of the dorsal anterior cingulate in youth with obsessive-compulsive disorder during motor control and working memory. J Psychiatr Res. (2021) 132:72–83. doi: 10.1016/j.jpsychires.2020.09.028
56. Diwadkar VA, Burgess A, Hong E, Rix C, Arnold PD, Hanna GL, et al. Dysfunctional activation and brain network profiles in youth with obsessive-compulsive disorder: A focus on the dorsal anterior cingulate during working memory. Front Hum Neurosci. (2015) 9:149. doi: 10.3389/fnhum.2015.00149
57. Woolley J, Heyman I, Brammer M, Frampton I, McGuire PK, Rubia K. Brain activation in paediatric obsessive compulsive disorder during tasks of inhibitory control. Br J Psychiatry. (2008) 192:25–31. doi: 10.1192/bjp.bp.107.036558
58. Del Casale A, Kotzalidis GD, Rapinesi C, Serata D, Ambrosi E, Simonetti A, et al. Functional neuroimaging in obsessive-compulsive disorder. Neuropsychobiology. (2011) 64:61–85.
60. Roth RM, Saykin AJ, Flashman LA, Pixley HS, West JD, Mamourian AC. Event-related functional magnetic resonance imaging of response inhibition in obsessive-compulsive disorder. Biol Psychiatry. (2007) 62:901–9.
61. Morein-Zamir S, Voon V, Dodds CM, Sule A, van Niekerk J, Sahakian BJ, et al. Divergent subcortical activity for distinct executive functions: Stopping and shifting in obsessive compulsive disorder. Psychol Med. (2016) 46:829–40. doi: 10.1017/S0033291715002330
62. Remijnse PL, Nielen MM, van Balkom AJ, Cath DC, van Oppen P, Uylings HB, et al. Reduced orbitofrontal-striatal activity on a reversal learning task in obsessive-compulsive disorder. Arch Gen Psychiatry. (2006) 63:1225–36. doi: 10.1001/archpsyc.63.11.1225
63. Grutzmann R, Endrass T, Kaufmann C, Allen E, Eichele T, Kathmann N. Presupplementary motor area contributes to altered error monitoring in obsessive-compulsive disorder. Biol Psychiatry. (2016) 80:562–71. doi: 10.1016/j.biopsych.2014.12.010
64. Etkin A, Wager TD. Functional neuroimaging of anxiety: A meta-analysis of emotional processing in PTSD, social anxiety disorder, and specific phobia. Am J Psychiatry. (2007) 164:1476–88. doi: 10.1176/appi.ajp.2007.07030504
65. Stephan KE, Friston KJ. Analyzing effective connectivity with functional magnetic resonance imaging. Wiley Interdiscip Rev Cogn Sci. (2010) 1:446–59.
66. Friston KJ, Buechel C, Fink GR, Morris J, Rolls E, Dolan RJ. Psychophysiological and modulatory interactions in neuroimaging. Neuroimage. (1997) 6:218–29.
67. McLntosh AR, Gonzalez-Lima F. Structural equation modeling and its application to network analysis in functional brain imaging. Hum Brain Mapp. (1994) 2:2–22.
68. Muzik O, Baajour S, Chowdury A, Diwadkar VA. Effective connectivity of brain networks controlling human thermoregulation. Brain Struct Funct. (2022). 227:299–312. doi: 10.1007/s00429-021-02401-w
69. Friston KJ, Li B, Daunizeau J, Stephan KE. Network discovery with DCM. Neuroimage. (2011) 56:1202–21.
70. Kaufman J, Birmaher B, Brent D, Rao U, Flynn C, Moreci P, et al. Schedule for affective disorders and schizophrenia for school-age children-present and lifetime version (K-SADS-PL): Initial reliability and validity data. J Am Acad Child Adolesc Psychiatry. (1997) 36:980–8. doi: 10.1097/00004583-199707000-00021
71. Wolff RP, Wolff LS. Assessment and treatment of obsessive-compulsive disorder in children. Behav Modif. (1991) 15:372–93.
72. Goodman WK, Price LH, Rasmussen SA, Mazure C, Fleischmann RL, Hill CL, et al. The Yale-Brown obsessive compulsive scale. I. Development, use, and reliability. Arch Gen Psychiatry. (1989) 46:1006–11.
73. Scahill L, Riddle MA, McSwiggin-Hardin M, Ort SI, King RA, Goodman WK, et al. Children’s Yale-Brown obsessive compulsive scale: Reliability and validity. J Am Acad Child Adolesc Psychiatry. (1997) 36:844–52.
74. Moody EJ, Reyes N, Ledbetter C, Wiggins L, DiGuiseppi C, Alexander A, et al. Screening for autism with the SRS and SCQ: Variations across demographic, developmental and behavioral factors in preschool children. J Autism Dev Disord. (2017) 47:3550–61. doi: 10.1007/s10803-017-3255-5
75. Mulligan A, Richardson T, Anney RJ, Gill M. The social communication questionnaire in a sample of the general population of school-going children. Ir J Med Sci. (2009) 178:193–9. doi: 10.1007/s11845-008-0184-5
76. Diwadkar VA, Goradia D, Hosanagar A, Mermon D, Montrose DM, Birmaher B, et al. Working memory and attention deficits in adolescent offspring of schizophrenia or bipolar patients: Comparing vulnerability markers. Prog Neuro Psychopharmacol Biol Psychiatry. (2011) 35:1349–54.
77. Christakou A, Murphy CM, Chantiluke K, Cubillo AI, Smith AB, Giampietro V, et al. Disorder-specific functional abnormalities during sustained attention in youth with attention deficit hyperactivity disorder (ADHD) and with autism. Mol Psychiatry. (2013) 18:236–44.
78. Diwadkar VA, Segel J, Pruitt P, Murphy ER, Keshavan MS, Radwan J, et al. Hypo-activation in the executive core of the sustained attention network in adolescent offspring of schizophrenia patients mediated by premorbid functional deficits. Psychiatry Res. (2011) 192:91–9. doi: 10.1016/j.pscychresns.2010.12.005
79. Dresp B, Grossberg S. Spatial facilitation by color and luminance edges: Boundary, surface, and attentional factors. Vision Res. (1999) 39:3431–43. doi: 10.1016/s0042-6989(99)00026-7
80. Muzik O, Baajour S, Bressler S, Diwadkar VA. Directional interactions between constituents of the human large-scale thermoregulatory network. Brain Topogr. (2020) 33:489–503. doi: 10.1007/s10548-020-00778-1
81. Szczepaniak M, Chowdury A, Soloff PH, Diwadkar VA. Stimulus valence, episodic memory, and the priming of brain activation profiles in borderline personality disorder. Psychol Med. (2021). doi: 10.1017/S0033291721001136 [Epub ahead of print].
82. Baajour SJ, Chowdury A, Thomas P, Rajan U, Khatib D, Zajac-Benitez C, et al. Disordered directional brain network interactions during learning dynamics in schizophrenia revealed by multivariate autoregressive models. Hum Brain Mapp. (2020) 41:3594–607. doi: 10.1002/hbm.25032
83. Ravishankar M, Morris A, Burgess A, Khatib D, Stanley JA, Diwadkar VA. Cortical-hippocampal functional connectivity during covert consolidation sub-serves associative learning: Evidence for an active “rest” state. Brain Cogn. (2019) 131:45–55. doi: 10.1016/j.bandc.2017.10.003
84. Tso IF, Angstadt M, Rutherford S, Peltier S, Diwadkar VA, Taylor SF. Dynamic causal modeling of eye gaze processing in schizophrenia. Schizophr Res. (2021) 229:112–21. doi: 10.1016/j.schres.2020.11.012
86. Nichols T, Brett M, Andersson J, Wager T, Poline JB. Valid conjunction inference with the minimum statistic. Neuroimage. (2005) 25:653–60.
87. Fan J, McCandliss BD, Fossella J, Flombaum JI, Posner MI. The activation of attentional networks. Neuroimage. (2005) 26:471–9.
88. Penny WD, Stephan KE, Daunizeau J, Rosa MJ, Friston KJ, Schofield TM, et al. Comparing families of dynamic causal models. PLoS Comput Biol. (2010) 6:e1000709. doi: 10.1371/journal.pcbi.1000709
89. FitzGerald TH, Dolan RJ, Friston KJ. Model averaging, optimal inference, and habit formation. Front Hum Neurosci. (2014) 8:457. doi: 10.3389/fnhum.2014.00457
90. Herrero MT, Barcia C, Navarro JM. Functional anatomy of thalamus and basal ganglia. Childs Nerv Syst. (2002) 18:386–404.
91. Park HJ, Friston K. Structural and functional brain networks: From connections to cognition. Science. (2013) 342:1238411.
92. Geffen T, Smallwood J, Finke C, Olbrich S, Sjoerds Z, Schlagenhauf F. Functional connectivity alterations between default mode network and occipital cortex in patients with obsessive-compulsive disorder (OCD). Neuroimage Clin. (2021) 33:102915.
93. Goncalves OF, Marques TR, Lori NF, Sampaio A, Branco MC. Obsessive-compulsive disorder as a visual processing impairment. Med Hypotheses. (2010) 74:107–9.
95. Kastner S, Schneider KA, Wunderlich K. Beyond a relay nucleus: Neuroimaging views on the human LGN. Prog Brain Res. (2006) 155:125–43. doi: 10.1016/S0079-6123(06)55008-3
96. Goodale MA, Milner AD. Separate visual pathways for perception and action. Trends Neurosci. (1992) 15:20–5.
97. Atkinson J, Braddick O. From genes to brain development to phenotypic behavior: “Dorsal-stream vulnerability” in relation to spatial cognition, attention, and planning of actions in Williams syndrome (WS) and other developmental disorders. Prog Brain Res. (2011) 189:261–83. doi: 10.1016/B978-0-444-53884-0.00029-4
98. Corbetta M, Shulman GL. Control of goal-directed and stimulus-driven attention in the brain. Nat Rev Neurosci. (2002) 3:201–15.
99. Bueicheku E, Ventura-Campos N, Palomar-Garcia MA, Miro-Padilla A, Parcet MA, Avila C. Functional connectivity between superior parietal lobule and primary visual cortex “at rest” predicts visual search efficiency. Brain Connect. (2015) 5:517–26. doi: 10.1089/brain.2015.0352
100. Moritz S, Rietschel L, Jelinek L, Bauml KH. Are patients with obsessive-compulsive disorder generally more doubtful? Doubt is warranted! Psychiatry Res. (2011) 189:265–9. doi: 10.1016/j.psychres.2011.07.023
101. Savage CR, Keuthen NJ, Jenike MA, Brown HD, Baer L, Kendrick AD, et al. Recall and recognition memory in obsessive-compulsive disorder. J Neuropsychiatry Clin Neurosci. (1996) 8:99–103.
102. Bloch MH, Sukhodolsky DG, Dombrowski PA, Panza KE, Craiglow BG, Landeros-Weisenberger A, et al. Poor fine-motor and visuospatial skills predict persistence of pediatric-onset obsessive-compulsive disorder into adulthood. J Child Psychol Psychiatry. (2011) 52:974–83. doi: 10.1111/j.1469-7610.2010.02366.x
103. Muller J, Roberts JE. Memory and attention in obsessive-compulsive disorder: A review. J Anxiety Disord. (2005) 19:1–28.
104. Savage CR, Baer L, Keuthen NJ, Brown HD, Rauch SL, Jenike MA. Organizational strategies mediate nonverbal memory impairment in obsessive-compulsive disorder. Biol Psychiatry. (1999) 45:905–16.
105. Shin NY, Lee TY, Kim E, Kwon JS. Cognitive functioning in obsessive-compulsive disorder: A meta-analysis. Psychol Med. (2014) 44:1121–30.
106. Purcell R, Maruff P, Kyrios M, Pantelis C. Cognitive deficits in obsessive-compulsive disorder on tests of frontal-striatal function. Biol Psychiatry. (1998) 43:348–57.
107. Savage CR, Rauch SL. Cognitive deficits in obsessive-compulsive disorder. Am J Psychiatry. (2000) 157:1182–3.
108. Harris EL, Schuerholz LJ, Singer HS, Reader MJ, Brown JE, Cox C, et al. Executive function in children with Tourette syndrome and/or attention deficit hyperactivity disorder. J Int Neuropsychol Soc. (1995) 1:511–6.
109. Shucard DW, Benedict RH, Tekok-Kilic A, Lichter DG. Slowed reaction time during a continuous performance test in children with Tourette’s syndrome. Neuropsychology. (1997) 11:147–55. doi: 10.1037//0894-4105.11.1.147
110. Chang SW, McCracken JT, Piacentini JC. Neurocognitive correlates of child obsessive compulsive disorder and Tourette syndrome. J Clin Exp Neuropsychol. (2007) 29:724–33.
111. Christensen KJ, Kim SW, Dysken MW, Hoover KM. Neuropsychological performance in obsessive-compulsive disorder. Biol Psychiatry. (1992) 31:4–18.
112. Head D, Bolton D, Hymas N. Deficit in cognitive shifting ability in patients with obsessive-compulsive disorder. Biol Psychiatry. (1989) 25:929–37.
113. Sherman SM, Guillery RW. Exploring the thalamus and its role in cortical function. 2nd ed. Cambridge, MA: MIT Press (2006).
114. Ab Aziz CB, Ahmad AH. The role of the thalamus in modulating pain. Malays J Med Sci. (2006) 13:11–8.
115. Saalmann YB, Kastner S. Cognitive and perceptual functions of the visual thalamus. Neuron. (2011) 71:209–23.
116. Kane MJ, Engle RW. The role of prefrontal cortex in working-memory capacity, executive attention, and general fluid intelligence: An individual-differences perspective. Psychon Bull Rev. (2002) 9:637–71. doi: 10.3758/bf03196323
117. Basso MA, Uhlrich D, Bickford ME. Cortical function: A view from the thalamus. Neuron. (2005) 45:485–8.
118. Briggs F, Usrey WM. Emerging views of corticothalamic function. Curr Opin Neurobiol. (2008) 18:403–7. doi: 10.1016/j.conb.2008.09.002
119. McCormick DA, Bal T. Sensory gating mechanisms of the thalamus. Curr Opin Neurobiol. (1994) 4:550–6.
121. Guillery RW, Harting JK. Structure and connections of the thalamic reticular nucleus: Advancing views over half a century. J Comp Neurol. (2003) 463:360–71. doi: 10.1002/cne.10738
122. Jagirdar R, Chin J. Corticothalamic network dysfunction and Alzheimer’s disease. Brain Res. (2019) 1702:38–45.
123. Pinault D. The thalamic reticular nucleus: Structure, function and concept. Brain Res Brain Res Rev. (2004) 46:1–31.
124. Takata N. Thalamic reticular nucleus in the thalamocortical loop. Neurosci Res. (2020) 156:32–40.
125. Young A, Wimmer RD. Implications for the thalamic reticular nucleus in impaired attention and sleep in schizophrenia. Schizophr Res. (2017) 180:44–7. doi: 10.1016/j.schres.2016.07.011
126. Crick F. Function of the thalamic reticular complex: The searchlight hypothesis. Proc Natl Acad Sci U.S.A. (1984) 81:4586–90.
127. Bucherelli C, Tassoni G, Bures J. Differential effect of functional ablation of thalamic reticular nucleus on the acquisition of passive and active avoidance. Int J Neurosci. (1993) 73:77–84. doi: 10.3109/00207459308987213
128. Weese GD, Phillips JM, Brown VJ. Attentional orienting is impaired by unilateral lesions of the thalamic reticular nucleus in the rat. J Neurosci. (1999) 19:10135–9. doi: 10.1523/JNEUROSCI.19-22-10135.1999
129. Sherman SM. Tonic and burst firing: Dual modes of thalamocortical relay. Trends Neurosci. (2001) 24:122–6.
130. Haber SN, Calzavara R. The cortico-basal ganglia integrative network: The role of the thalamus. Brain Res Bull. (2009) 78:69–74. doi: 10.1016/j.brainresbull.2008.09.013
131. Chamberlain BL, Ahmari SE. Animal models for OCD research. Curr Top Behav Neurosci. (2021) 49:55–96.
132. MacMaster FP, O’Neill J, Rosenberg DR. Brain imaging in pediatric obsessive-compulsive disorder. J Am Acad Child Adolesc Psychiatry. (2008) 47:1262–72.
133. Sha Z, Versace A, Edmiston EK, Fournier J, Graur S, Greenberg T, et al. Functional disruption in prefrontal-striatal network in obsessive-compulsive disorder. Psychiatry Res Neuroimaging. (2020) 300:111081.
134. Li K, Zhang H, Yang Y, Zhu J, Wang B, Shi Y, et al. Abnormal functional network of the thalamic subregions in adult patients with obsessive-compulsive disorder. Behav Brain Res. (2019) 371:111982. doi: 10.1016/j.bbr.2019.111982
135. Dajani DR, Uddin LQ. Demystifying cognitive flexibility: Implications for clinical and developmental neuroscience. Trends Neurosci. (2015) 38:571–8. doi: 10.1016/j.tins.2015.07.003
136. Ahmari SE, Rauch S. The prefrontal cortex and OCD. Neuropsychopharmacology. (2022). 47:211–224. doi: 10.1038/s41386-021-01130-2
137. Gruner P, Pittenger C. Cognitive inflexibility in obsessive-compulsive disorder. Neuroscience. (2017) 345:243–55.
138. Vaghi MM, Hampshire A, Fineberg NA, Kaser M, Bruhl AB, Sahakian BJ, et al. Hypoactivation and dysconnectivity of a frontostriatal circuit during goal-directed planning as an endophenotype for obsessive-compulsive disorder. Biol Psychiatry Cogn Neurosci Neuroimaging. (2017) 2:655–63. doi: 10.1016/j.bpsc.2017.05.005
139. van den Heuvel OA, Veltman DJ, Groenewegen HJ, Cath DC, van Balkom AJ, van Hartskamp J, et al. Frontal-striatal dysfunction during planning in obsessive-compulsive disorder. Arch Gen Psychiatry. (2005) 62: 301–9.
140. Singh KD. Which “neural activity” do you mean? fMRI, MEG, oscillations and neurotransmitters. Neuroimage. (2012) 62:1121–30. doi: 10.1016/j.neuroimage.2012.01.028
141. Weeland CJ, Vriend C, van der Werf Y, Huyser C, Hillegers M, Tiemeier H, et al. Thalamic Subregions and Obsessive-Compulsive Symptoms in 2,500 Children From the General Population. J Am Acad Child Adolesc Psychiatry. (2022) 61:321–330. doi: 10.1016/j.jaac.2021.05.024
142. Monteiro P, Feng G. Learning from animal models of obsessive-compulsive disorder. Biol Psychiatry. (2016) 79:7–16.
143. Aouizerate B, Guehl D, Cuny E, Rougier A, Bioulac B, Tignol J, et al. Pathophysiology of obsessive-compulsive disorder: A necessary link between phenomenology, neuropsychology, imagery and physiology. Prog Neurobiol. (2004) 72:195–221. doi: 10.1016/j.pneurobio.2004.02.004
144. Mu Y, See I, Edwards JR. Bayesian model averaging: Improved variable selection for matched case-control studies. Epidemiol Biostat Public Health. (2019) 16:e13048.
145. Alves-Pinto A, Rus OG, Reess TJ, Wohlschlager A, Wagner G, Berberich G, et al. Altered reward-related effective connectivity in obsessive-compulsive disorder: An fMRI study. J Psychiatry Neurosci. (2019) 44:395–406. doi: 10.1503/jpn.180195
146. Han HJ, Jung WH, Yun JY, Park JW, Cho KK, Hur JW, et al. Disruption of effective connectivity from the dorsolateral prefrontal cortex to the orbitofrontal cortex by negative emotional distraction in obsessive-compulsive disorder. Psychol Med. (2016) 46:921–32. doi: 10.1017/S0033291715002391
147. Blanken TF, Bathelt J, Deserno MK, Voge L, Borsboom D, Douw L. Connecting brain and behavior in clinical neuroscience: A network approach. Neurosci Biobehav Rev. (2021) 130:81–90.
148. Brandt T, Dieterich M. Thalamocortical network: A core structure for integrative multimodal vestibular functions. Curr Opin Neurol. (2019) 32:154–64. doi: 10.1097/WCO.0000000000000638
150. Stephan KE, Binder EB, Breakspear M, Dayan P, Johnstone EC, Meyer-Lindenberg A, et al. Charting the landscape of priority problems in psychiatry, part 2: Pathogenesis and aetiology. Lancet Psychiatry. (2016) 3:84–90. doi: 10.1016/S2215-0366(15)00360-0
Keywords: dynamic causal modeling, obsessive-compulsive disorder, sustained attention, fMRI, thalamo-cortical relay
Citation: Yacou MA, Chowdury A, Easter P, Hanna GL, Rosenberg DR and Diwadkar VA (2022) Sustained attention induces altered effective connectivity of the ascending thalamo-cortical relay in obsessive-compulsive disorder. Front. Psychiatry 13:869106. doi: 10.3389/fpsyt.2022.869106
Received: 03 February 2022; Accepted: 18 July 2022;
Published: 10 August 2022.
Edited by:
Stefan Borgwardt, University of Lübeck, GermanyReviewed by:
Jennifer Barredo, Providence VA Medical Center, United StatesMeng-Yun Wang, University of Bergen, Norway
Zhen Yuan, University of Macau, Macao SAR, China
Copyright © 2022 Yacou, Chowdury, Easter, Hanna, Rosenberg and Diwadkar. This is an open-access article distributed under the terms of the Creative Commons Attribution License (CC BY). The use, distribution or reproduction in other forums is permitted, provided the original author(s) and the copyright owner(s) are credited and that the original publication in this journal is cited, in accordance with accepted academic practice. No use, distribution or reproduction is permitted which does not comply with these terms.
*Correspondence: Vaibhav A. Diwadkar, dmFpYmhhdi5kaXdhZGthckB3YXluZS5lZHU=