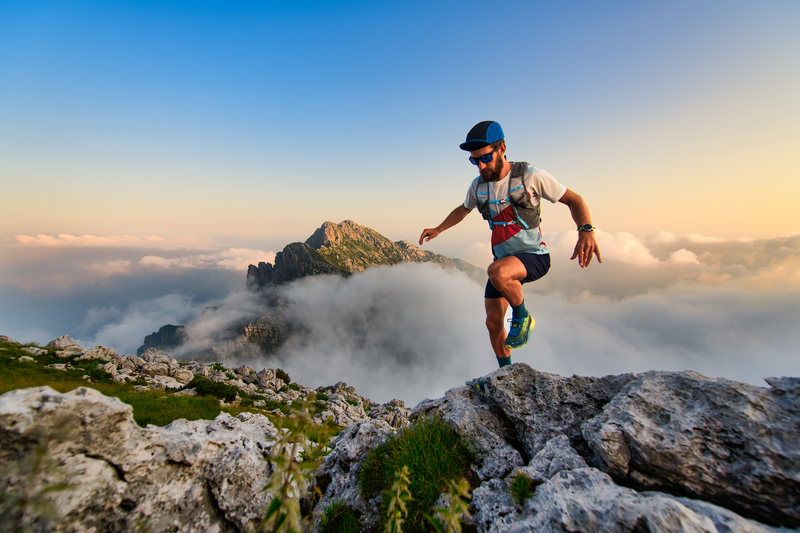
94% of researchers rate our articles as excellent or good
Learn more about the work of our research integrity team to safeguard the quality of each article we publish.
Find out more
BRIEF RESEARCH REPORT article
Front. Hum. Neurosci. , 31 October 2024
Sec. Brain Imaging and Stimulation
Volume 18 - 2024 | https://doi.org/10.3389/fnhum.2024.1501310
Resting-state functional magnetic resonance imaging (RS-fMRI) has been extensively utilized for noninvasive investigation of human brain activity. While studies employing simultaneous recordings of fMRI and electrophysiology have established a connection between the low-frequency fluctuation (< 0.1 Hz) observed in RS-fMRI and the local field potential (LFP), it remains unclear whether the RS-fMRI signal exhibits frequency-dependent modulation, which is a well-documented phenomenon in LFP. The present study concurrently recorded resting-state functional magnetic resonance imaging (RS-fMRI) and local field potentials (LFP) in the striatum of 8 rats before and after a pharmacological manipulation. We observed a highly similar frequency-dependent pattern of amplitude changes in both RS-fMRI and LFP following the manipulation, specifically an increase in high-frequency band amplitudes accompanied by a decrease in low-frequency band amplitudes. These findings provide direct evidence that the enhanced high-frequency fluctuations and reduced low-frequency fluctuations observed in RS-fMRI may reflect heightened neuronal activity.
Blood oxygenation level dependent (BOLD) functional magnetic resonance imaging (fMRI) has been extensively utilized for mapping human brain function. Resting-state fMRI (RS-fMRI) is a technique that measures brain activity without requiring participants to perform specific tasks, making it particularly useful for populations with difficulty engaging in tasks, such as young children and patients with severe psychiatric conditions (Biswal et al., 1995). To date, thousands of RS-fMRI studies have been published, shedding light on abnormal spontaneous brain activity across a range of neurological and psychiatric disorders (Bandettini, 2012). Despite these advancements, the precise neurophysiological mechanisms underlying RS-fMRI signals remain largely unknown (Logothetis, 2008).
The BOLD signal serves as an indirect marker of neuronal activity. Approximately two decades ago, Logothetis and colleagues conducted a pioneering study involving simultaneous intracortical recordings of fMRI and electrophysiological signals. Their findings indicated that local field potential (LFP) signals were more predictive of BOLD signal fluctuations compared to multiple unit or single unit activity during visual stimulation, suggesting that the BOLD signal primarily reflects synaptic inputs and local processing rather than neuronal outputs (Logothetis et al., 2001). Since this seminal work, numerous studies have explored the relationship between RS-fMRI signals and electrophysiological activity through simultaneous recordings. Most of these studies have investigated correlations between RS-fMRI time series and LFP power fluctuations (Pan et al., 2011; Pan et al., 2013; Shi et al., 2017; Chan et al., 2017; Liu et al., 2018; Jaime et al., 2019). Notably, significant correlations have been observed between low-frequency components of the RS-fMRI signal (typically 0.01–0.08 Hz) and band-limited power (BLP) time series across broad LFP frequency bands (1–100 Hz) (Pan et al., 2011; Pan et al., 2013; Jaime et al., 2019), with evidence that these correlations are modulated by factors such as anesthesia or pharmacological agents like alpha-amino-3-hydroxy-5-methyl-4-isoxazole propionic acid (AMPA) (Jaime et al., 2019).
It is well-established that increases in neuronal activity are accompanied by elevated LFP amplitudes in high-frequency bands and reduced amplitudes in low-frequency bands (Leopold et al., 2003). Most RS-fMRI studies have focused on conventional low-frequency signals (0.01–0.08 Hz), as higher frequency components (≥ 0.08 Hz) are considered more susceptible to physiological noise from sources such as cardiac and respiratory fluctuations (Birn, 2012). Nevertheless, accumulating evidence suggests that higher frequency RS-fMRI signals may carry important physiological (Boubela et al., 2013; Yuan et al., 2014) or pathophysiological information (Malinen et al., 2010; Otti et al., 2013; Hong et al., 2013; Hong et al., 2018; Ries et al., 2019; Wang et al., 2020). Despite these indications, few studies have examined the neuronal significance of higher frequency RS-fMRI components using simultaneous recordings. Thus, it remains critical to explore whether BOLD signals exhibit a similar frequency-dependent modulation as observed in LFP, characterized by decreased low-frequency and increased high-frequency amplitudes with enhanced neuronal activity. In the present study, we sought to demonstrate a highly similar frequency-dependent pattern of RS-fMRI and LFP amplitudes in response to pharmacological manipulation.
Eight male Sprague-Dawley rats (275–450g; Charles River) were used in the current study. Each rat was implanted with microelectrode arrays and guide cannulas for pharmacological manipulations. The rats were housed individually in a colony room with ad libitum access to water and food, under a 12-hour light/dark cycle. All experiments were conducted during the light phase. The study procedures were approved by the NIDA-IRP Animal Care and Use Committee.
An MRI-compatible linear microelectrode array was implanted to cover the dorsolateral to ventromedial striatum. A guide cannula was also implanted in the VTA. One week after surgery, MRI and electrophysiological recordings were performed to ensure sufficient surgical recovery. Resting-state scans were initially performed as the baseline session. Subsequently, AMPA was infused into the VTA to enhance dopamine release in the nucleus accumbens, a major target of VTA dopamine neurons (Wang et al., 2015). Special care was taken to minimize MRI-induced electrical artifacts in the local field potential (LFP) recordings, and to ensure the AMPA solution was precisely limited to 1 μl.
To record from the dorsolateral to ventromedial striatal domain, a silicon-based MRI-compatible 16-channel linear electrode array was implanted using aseptic procedures. The electrodes were 15 μm thick, 10 mm long, with 16 gold contacts (30 μm diameter) spaced 200 μm apart (Neuronexus, Ann Arbor, MI) (Figure 1). Rats were initially anesthetized with 2% isoflurane, and their core body temperature, respiration, and arterial oxygenation were continuously monitored and maintained within normal physiological ranges throughout surgery. A 1 mm2 craniotomy was made above the nucleus accumbens (NAc) (AP: ++1.2 mm, ML: 3.9 mm, DV: −7.0 mm). The dura was carefully removed using a 27-gauge needle and a #10 scalpel blade. The electrode array was lowered into the brain using a motorized microdriver at a 20° angle (medial-lateral). Dental cement was used to secure the electrode following a 5-minute rest period to allow the tissue to stabilize. A 22-gauge plastic guide cannula (7 mm below pedestal; Plastics One, Roanoke, VA), with a cannula dummy, was implanted into the VTA (AP: −5.0 mm, ML: 0.6–0.8 mm). A ground/reference brass screw, soldered to the ground/reference wires from the electrode using silver solder, was placed over the cerebellum. Rats were given at least one week to recover from surgery before further manipulations. Gentamicin and buprenorphine were administered intraperitoneally to reduce infection risk and alleviate pain during recovery.
Figure 1. The electrode position. Simultaneous recording of local field potential and resting-state functional magnetic resonance imaging (RS-fMRI) in the striatum of a rat before and after AMPA infusion in the ventral tegmental area (VTA). The time series of the RS-fMRI signal in a region of interest (ROI) at tip (in red, 2 slices, 2 voxels, 0.5 × 0.5 × 1 mm3) at the tip of the electrode was extracted for further analysis (L: left. R: right).
Rats were initially anesthetized with 2% isoflurane until the hind limb reflex was absent. Then, anesthesia was maintained with a continuous loading dose (0.01 mg/kg, i.p.) of dexmedetomidine (Dexdomitor), an α2-adrenergic receptor agonist. Rats were placed in an MRI-compatible holder, and their heads were stabilized using a customized bite bar and ear holders. Body temperature was maintained at approximately 37 ± 0.5°C using a water heating pad and monitored with a rectal temperature probe. A pad was placed between the rat and the heating pad to monitor respiration rate concurrently (SA Instrument Inc., NY, USA). Oxygen saturation was monitored using a noninvasive MRI-compatible pulse oximeter (Starr Life Sciences Corp., PA, USA) attached to a hind paw, with O2 saturation maintained at > 96% by adjusting the inhaled oxygen concentration. Isoflurane levels were gradually lowered to 0.5–0.75% depending on the rat’s condition. This anesthesia protocol has been tested in previous studies and shown to have minimal effects on spontaneous brain activity.
MRI data were acquired using a Bruker Biospin 9.4T scanner equipped with an actively shielded gradient coil, running ParaVision 6.0.1 (Bruker Medizintechnik, Karlsruhe, Germany). An 86 mm volume transmitter coil was used for RF excitation, and a 2 cm OD surface coil was placed directly over the skull to encompass the electrode and cannula placement sites for MR signal reception. Tuning and matching of the coils were performed for each subject. T2-weighted anatomical images were acquired using a rapid acquisition with relaxation enhancement (RARE) sequence. Scan parameters were as follows: TR = 2500 ms, TE = 40 ms, RARE factor = 8, field of view (FOV) = 3.5 × 3.5 cm2, matrix size = 256 × 256. Functional images were acquired using a gradient echo echo-planar imaging (EPI) sequence with the following parameters: FOV = 3 × 3 cm2, matrix size = 64 × 64, TR = 1500 ms, TE = 13 ms, four 1 mm thick slices. The data acquisition bandwidth was 250 kHz. The first three slices covered the striatal region, and the fourth slice was positioned in the VTA. The midsagittal view of the anterior commissure decussation (−0.36 mm from bregma) was used as a fiducial marker to standardize slice localization. fMRI scanning commenced 90 minutes after the dexmedetomidine loading dose, ensuring stable animal physiology and robust BOLD signal (Lu et al., 2012; Jaime et al., 2019; Brynildsen et al., 2016; Hsu et al., 2016). Pre- and post-AMPA infusion scans were conducted sequentially, with each session lasting 7 minutes and 34 seconds, comprising 300 TRs with four dummy scans.
A customized jumper cable (1.5 feet in length; Plexon Inc., Dallas, TX) was attached directly to the probe implant (Neuronexus, Ann Arbor, MI) when the animal was set well inside the MRI holder. This jumper cable allowed for the headstage to be placed outside of the gradient and RF coils to reduce the MRI- induced artifacts. An MRI compatible headstage (gain = 1) was attached to the jumper cable and the amplifier. A multichannel data acquisition system (Plexon Inc., Dallas, TX) was used for recorded the LFP signals and amplified at a gain of 1000, notch filtered at 60 Hz, and digitally sampled at 5 kHz. Another two separate channels were used to record the respiratory waveform and MRI trigger signal besides the electrode array’s 16 channels. We need to use the trigger signal to remove LFPs’ MRI artifacts. However, in this experiment, we cannot get any recordings at the amplifier for the 15th channel in our system all the time; this channel’s data was abandoned.
The striatum is a major projection of the midbrain dopamine neurons. Previous studies had shown that the ventral striatum (nucleus accumbens, NAc) receives projections mainly from the VTA, while the dorsal striatum receives mainly substantia nigra and pars compacta (Ikemoto, 2007). By using the opto-fMRI, recent studies have demonstrated that the dorsal striatum becomes activated in response to optogenetic stimulation of VTA dopaminergic neurons (Beier et al., 2015; Ferenczi et al., 2016; Decot et al., 2017; Lohani et al., 2017). An early study demonstrated that the dopamine release in the ventral striatum could be enhanced by the microinjection of AMPA (RS-alpha-amino-3-hydroxy-5-methyl-4-isoxazolepropionic acid), an ionotropic glutamate receptor agonist (Karreman et al., 1996). Its mechanism is that VTA glutamate neurons synapse on VTA DA neurons and these DA neurons project to the ventral striatum (nucleus accumbens core), and both NMDA and AMPA receptors are involved in this circuit (Wang et al., 2015). This inspired us to manipulate the ventral striatal neuronal activity via similar approach, AMPA injections (Bristol, UK, 1μl, 100μM in saline vehicle) into the VTA.
Specifically, a fused silica needle (Plastics One, Roanoke, VA) was lowered into the implanted guide cannula and connected to a micro-infusion pump via 1.5m long polyethylene tubing (PE 10). We kept the pump outside and the rat inside the scanner on a plane level to minimize solution backflow or leakage. Also, air bubbles inside the infusion line were avoided.
In this study, we implanted a guide cannula into the VTA for AMPA infusion and an electrode in the ventral striatum for LFP recording. A total of 12 sessions of simultaneous fMRI and LFP recordings were conducted before and after AMPA infusion across 8 rats. AMPA infusion into the VTA has been well-documented to enhance neuronal activity in the striatum (Jaime et al., 2019; Karreman et al., 1996; Wang et al., 2015). Therefore, we anticipated that both BOLD and LFP signals would exhibit similar patterns of change following the infusion.
LFP data analysis was performed using EEGLAB (Delorme and Makeig, 2004) in Matlab (MathWorks, MA, USA). The analysis included MRI artifact removal, filtering, and downsampling. The preprocessed signals were then divided into conventional delta (1–4 Hz), theta (5–8 Hz), alpha (9–14 Hz), beta (15–30 Hz), and gamma (30–50 Hz) frequency bands for subsequent fractional amplitude calculations.
The MR environment induces significant artifacts in LFP signals, which is a common issue in simultaneous recordings. To address this, we applied the following algorithm to remove MR artifacts: LFP signals were segmented using the concurrently recorded trigger signal. Each 60 ms segment immediately following the trigger signal contained high amplitude (up to ± 5 mV) rapidly changing signals, which were replaced by linear interpolation. An eighth-order Butterworth filter was then applied to low-pass filter the resulting signal to 400 Hz using Matlab’s filtfilt() function, which prevents phase nonlinearity and group delays. The filtered data were subsequently downsampled to 1 kHz. The linear interpolation minimized ringing artifacts during filtering. The linearly interpolated segments were further refined using cubic-spline interpolation of the data from 35 ms before and after the artifact segments. Finally, the Butterworth filter and filtfilt() function were applied again to low-pass filter the LFP data to 100 Hz, followed by downsampling to 250 Hz.
The preprocessed LFP signals were analyzed using fast Fourier transformation (FFT) for frequencies between 1 Hz and 50 Hz. After denoising, the amplitude of the LFP at the electrode tip channel (Figure 1) was calculated for the following sub-frequency bands: delta (1–4 Hz), theta (5–8 Hz), alpha (9–14 Hz), beta (15–30 Hz), and gamma (30–50 Hz). The fractional amplitude for each above conventional LFP sub-frequency band was calculated by first determining the mean amplitude for each band after FFT, and then dividing each of these mean values by the mean amplitude of the entire 1–50 Hz range.
Preprocessing of resting-state MRI data was conducted using the AFNI software package (Cox and Hyde, 1997). Spatial independent component analysis (ICA) from the Melodic package (FSL software, Oxford University, UK) was used on each scan separately to identify and remove noisy components related to respiration and cardiac pulsation. Images from each session were coregistered to a common 3D anatomical space (Lu et al., 2010). The registered data were then subjected to slice-timing correction, linear and quadratic trend removal, and spatial smoothing with a Gaussian kernel (full width at half maximum (FWHM) = 0.6 mm). After preprocessing, wavelet transform (Luo et al., 2020) was used to calculate the fractional amplitude of low-frequency fluctuations (fALFF) (0.01–0.333 Hz) in four different frequency bands (0.01–0.027 Hz, 0.027–0.073 Hz, 0.073–0.198 Hz, and 0.198–0.333 Hz) (Zou et al., 2008; Zuo et al., 2010). The selected voxels (Figure 1) corresponded to the approximate location of the electrode tip, allowing direct comparison of the LFP and BOLD signals. Moreover, the LFP probe did not significantly interfere with the BOLD signal, as evidenced by intact contralateral connectivity from the affected striatum (Supplementary Figure 1).
Each rat underwent 6 pre- and 6 post-AMPA infusion sessions, resulting in 48 pairs (8 rats × 6 sessions) for the paired t-test analysis. To minimize selection bias, we permuted the pre- and post-infusion sessions across all rats and repeated the paired t-test 1000 times to generate average.
Two-way repeated measures ANOVA (frequency bands × pre- and post-infusion) was used to test the interaction effects of both LFP and BOLD signals.
After AMPA infusion, we observed a decrease in amplitude within the low frequency bands and an increase in amplitude within the high frequency range for both LFP and RS-fMRI signals (Figure 2). Specifically, the amplitude of the BOLD signal exhibited a reduction in the low frequency band (0.027–0.073 Hz) but an elevation in the high frequency band (0.198–0.333 Hz) following AMPA infusion (Figure 2 and Tables 1, 2).
Figure 2. Frequency-dependent pharmacological manipulation effects (mean amplitude of post- minus pre-AMPA infusion). (a), Local field potential (LFP) in 5 conventional frequency bands (Delta: 1–4 Hz; Theta: 5–8 Hz; Alpha: 9–14 Hz; Beta: 15–30 Hz; Gamma: 30–50 Hz). (b), Resting-state functional magnetic resonance imaging (RS-fMRI) in 4 frequency bands (0.01–0.027 Hz; 0.027–0.073 Hz; 0.073–0.198 Hz; 0.198–0.333 Hz). Paired t-tests showed that the high frequency bands of both LFP (Beta and Gamma) and RS-fMRI (0.198–0.333 Hz) were significantly increased after AMPA infusion (*P < 0.05; **P < 0.01; ***P < 0.001. More details were shown in Tables 1, 2).
This experiment was a 2 × 2 repeated measures design. Repeated measures ANOVA showed that LFP and BOLD signal were significantly different in sub-frequency band, and the interaction effect of frequency band and AMPA modulation was significant (Tables 3, 4 and Figure 3).
Figure 3. LFP and BOLD sub-frequency bands interaction. (a) LFP interaction pattern. For better comparison, the figure here were using Z-transform along each rat, increased or decreased sub-frequency bands were using different colors. (b) BOLD interaction pattern. Same as the LFP interaction, this figure was using Z-transform along each rat, increased or decreased sub-frequency bands were using different colors.
The majority of RS-fMRI studies have predominantly focused on low-frequency (< 0.08 Hz) signals in the context of brain disorders. However, a few RS-fMRI studies have reported abnormally increased amplitudes in the high-frequency band of the BOLD signal, accompanied by decreased amplitudes in the low-frequency band, in certain brain disorders (Malinen et al., 2010; Otti et al., 2013; Hong et al., 2013; Hong et al., 2018; Ries et al., 2019; Wang et al., 2020). For instance, two independent studies have shown increased RS-fMRI fluctuation amplitude in the high-frequency band along with decreased low-frequency amplitude in the anterior cingulate cortex and anterior insula of patients with chronic pain (Malinen et al., 2010; Otti et al., 2013). In the present study, we found that the increased amplitude in the high-frequency band, combined with a decreased amplitude in the low-frequency band of RS-fMRI, mirrors the frequency-dependent changes observed in LFP amplitudes (Figure 2). These findings suggest that increased high-frequency RS-fMRI amplitudes in brain disorders reflect abnormally heightened neuronal activity in high-frequency bands, which may be linked to underlying pathophysiology.
It is important to highlight the analytical approach used in the current study. Most existing studies of simultaneous LFP-fMRI recordings have focused on correlations between LFP power time series and RS-fMRI time series. In contrast, our study analyzed the amplitude of RS-fMRI signals (Zou et al., 2008; Zang et al., 2007) in a manner similar to the analysis of LFP amplitude (Birn, 2012). The amplitude of low-frequency fluctuation (ALFF) is one of the most widely used methods to depict local RS-fMRI activity (Di Martino et al., 2014). ALFF analysis involves examining the single time series within a specific brain region, which allows for precise localization of abnormal activity. The fALFF index is normalized in the frequency domain based on ALFF, thereby mitigating the impact of noise and enhancing the stability and comparability of the results (Zou et al., 2008). In this study, we analyzed not only the lower-frequency components but also the higher-frequency components of the RS-fMRI signal (Malinen et al., 2010; Otti et al., 2013; Hong et al., 2013; Hong et al., 2018; Ries et al., 2019; Wang et al., 2020).
The choice of the striatum for LFP recordings was informed by its central role in motor and cognitive functions, as well as its involvement in a wide range of neuropsychiatric disorders. This makes the striatum a relevant region for understanding frequency-dependent neuronal dynamics in the context of brain disorders. However, it remains unclear to what extent the findings can be generalized to other brain regions. Future studies should investigate whether similar frequency-dependent modulation patterns are present in other key brain areas, such as the prefrontal cortex, to better understand the broader applicability of these findings.
Several limitations should be acknowledged. First, the sample size in this study (n = 8) is relatively small. While the results are robust, this limitation may impact the statistical power and generalizability of the findings. Future studies should aim to include larger sample sizes to enhance the reliability of the conclusions. Additionally, we used a TR of 1.5 seconds, which limits the ability to analyze RS-fMRI signals above 0.34 Hz. Future studies should use faster scanning protocols to capture higher-frequency signals. Another limitation is that, due to magnetic susceptibility and subsequent signal loss at the thicker parts of the electrode (Figure 1), only the electrophysiological signal from the tip channel was analyzed. This constraint may have reduced the overall richness of the LFP data obtained.
Finally, the frequency-dependent modulation pattern observed in our study points to an intriguing mechanism of neuronal activity regulation. The increased high-frequency amplitude, along with decreased low-frequency amplitude, may reflect a shift towards heightened excitatory activity, potentially contributing to dysregulation in brain disorders. This could represent a compensatory mechanism or a maladaptive process linked to disease pathology. Future studies should aim to elucidate the underlying cellular and network mechanisms of this modulation, which may provide insights into the functional significance of these changes in the context of both health and disease.
In this study, we demonstrated a highly similar frequency-dependent pattern in the amplitude of RS-fMRI and LFP following pharmacological manipulation. We observed that the amplitudes of RS-fMRI and LFP signals covaried in a similar manner, with decreased low-frequency and increased high-frequency amplitudes. This finding reveals, for the first time, a potential direct coupling between high-frequency RS-fMRI signals and LFP activity. Furthermore, our results suggest that high-frequency RS-fMRI components, rather than low-frequency ones, may have significant physiological and pathophysiological relevance.
The raw data supporting the conclusions of this article will be made available by the authors, without undue reservation.
The animal study was approved by the NIDA-IRP Animal Care and Use Committee. The study was conducted in accordance with the local legislation and institutional requirements.
YQ: Writing – original draft, Writing – review and editing. HL: Writing – review & editing. YY: Writing – review and editing. YZ: Writing – review and editing, Writing – original draft.
The author(s) declare financial support was received for the research, authorship, and/or publication of this article. This work is supported by the Key Project of the Department of Science and Technology of Zhejiang Province, Key Medical Discipline of Hangzhou (2015C03037) and NSFC (81520108016, 81661148045, and 31471084). HL and YY were supported by the Intramural Research Program of the National Institute on Drug Abuse, the National Institutes of Health.
The authors declare that the research was conducted in the absence of any commercial or financial relationships that could be construed as a potential conflict of interest.
The author(s) declared that they were an editorial board member of Frontiers, at the time of submission. This had no impact on the peer review process and the final decision.
All claims expressed in this article are solely those of the authors and do not necessarily represent those of their affiliated organizations, or those of the publisher, the editors and the reviewers. Any product that may be evaluated in this article, or claim that may be made by its manufacturer, is not guaranteed or endorsed by the publisher.
The Supplementary Material for this article can be found online at: https://www.frontiersin.org/articles/10.3389/fnhum.2024.1501310/full#supplementary-material
Bandettini, P. A. (2012). Twenty years of functional MRI: The science and the stories. Neuroimage 62, 575–588. doi: 10.1016/j.neuroimage.2012.04.026
Beier, K., Elizabeth, E., DeLoach, K., Xie, S., Miyamichi, K., Schwarz, L., et al. (2015). Circuit architecture of VTA dopamine neurons revealed by systematic input-output mapping. Cell 162, 622–634.
Birn, R. (2012). The role of physiological noise in resting-state functional connectivity. Neuroimage 62, 864–870.
Biswal, B., Yetkin, F., Haughton, V., and Hyde, J. (1995). Functional connectivity in the motor cortex of resting human brain using echo-planar MRI. Magn. Reson. Med. 34, 537–541.
Boubela, R., Kalcher, K., Huf, W., Kronnerwetter, C., Filzmoser, P., and Moser, E. (2013). Beyond noise: Using temporal ICA to extract meaningful information from high-frequency fMRI signal fluctuations during rest. Front. Hum. Neurosci. 7:168. doi: 10.3389/fnhum.2013.00168
Brynildsen, J., Hsu, L., Ross, T., Stein, E., Yang, Y., and Lu, H. (2016). Physiological characterization of a robust survival rodent fMRI method. Magn. Reson. Imaging 35, 54–60. doi: 10.1016/j.mri.2016.08.010
Chan, R., Leong, A., Ho, L., Gao, P., Wong, E., Dong, C., et al. (2017). Low-frequency hippocampal-cortical activity drives brain-wide resting-state functional MRI connectivity. Proc. Natl. Acad. Sci. U.S.A. 114, E6972–E6981. doi: 10.1073/pnas.1703309114
Cox, R., and Hyde, J. (1997). Software tools for analysis and visualization of fMRI data. NMR Biomed. 10, 171–178.
Decot, H., Namboodiri, V., Gao, W., McHenry, J., Jennings, J., Lee, S., et al. (2017). Coordination of brain-wide activity dynamics by dopaminergic neurons. Neuropsychopharmacology 42, 615–627.
Delorme, A., and Makeig, S. (2004). EEGLAB: An open source toolbox for analysis of single-trial EEG dynamics including independent component analysis. J. Neurosci. Methods 134, 9–21.
Di Martino, A., Fair, D., Kelly, C., Satterthwaite, T., Castellanos, F., Thomason, M., et al. (2014). Unraveling the miswired connectome: A developmental perspective. Neuron 83, 1335–1353. doi: 10.1016/j.neuron.2014.08.050
Ferenczi, E., Zalocusky, K., Liston, C., Grosenick, L., Warden, M., Amatya, D., et al. (2016). Prefrontal cortical regulation of brainwide circuit dynamics and reward-related behavior. Science 351:aac9698. doi: 10.1126/science.aac9698
Hong, J., Kilpatrick, L., Labus, J., Gupta, A., Jiang, Z., Ashe-McNalley, C., et al. (2013). Patients with chronic visceral pain show sex-related alterations in intrinsic oscillations of the resting brain. J. Neurosci. 33, 11994–12002. doi: 10.1523/JNEUROSCI.5733-12.2013
Hong, J., Müller-Oehring, E., Pfefferbaum, A., Sullivan, E., Kwon, D., and Schulte, T. (2018). Aberrant blood-oxygen-level-dependent signal oscillations across frequency bands characterize the alcoholic brain. Addict. Biol. 23, 824–835. doi: 10.1111/adb.12532
Hsu, L., Liang, X., Gu, H., Brynildsen, J., Stark, J., Ash, J., et al. (2016). Constituents and functional implications of the rat default mode network. Proc. Natl. Acad. Sci. U.S.A. 113, E4541–E4547. doi: 10.1073/pnas.1601485113
Ikemoto, S. (2007). Dopamine reward circuitry: Two projection systems from the ventral midbrain to the nucleus accumbens- olfactory tubercle complex. Brain Res. Rev. 56, 27–78. doi: 10.1016/j.brainresrev.2007.05.004
Jaime, S., Gu, H., Sadacca, B. F., Stein, E. A., Cavazos, J. E., Yang, Y., et al. (2019). Delta rhythm orchestrates the neural activity underlying the resting state BOLD signal via phase-amplitude coupling. Cereb. Cortex 29, 119–133. doi: 10.1093/cercor/bhx310
Karreman, M., Westerink, B., and Moghaddam, B. (1996). Excitatory amino acid receptors in the ventral tegmental area regulate dopamine release in the ventral striatum. J. Neurochem. 67, 601–607.
Leopold, D., Murayama, Y., and Logothetis, N. (2003). Very slow activity fluctuations in monkey visual cortex: Implications for functional brain imaging. Cereb. Cortex 13, 422–433.
Liu, X., de Zwart, J., Schölvinck, M., Chang, C., Ye, F., Leopold, D., et al. (2018). Subcortical evidence for a contribution of arousal to fMRI studies of brain activity. Nat. Commun. 9:395.
Logothetis, N., Pauls, J., Augath, M., Trinath, T., and Oeltermann, A. (2001). Neurophysiological investigation of the basis of the fMRI signal. Nature 412, 150–157.
Lohani, S., Poplawsky, A., Kim, S., and Moghaddam, B. (2017). Unexpected global impact of VTA dopamine neuron activation as measured by opto-fMRI. Mol. Psychiatry 22, 585–594. doi: 10.1038/mp.2016.102
Lu, H., Scholl, C., Zuo, Y., Demny, S., Rea, W., Stein, E., et al. (2010). Registering and analyzing rat fMRI data in the stereo- taxic framework by exploiting intrinsic anatomical features. Magn. Reson. Imaging 28, 146–152. doi: 10.1016/j.mri.2009.05.019
Lu, H., Zou, Q., Gu, H., Raichle, M., Stein, E., and Yang, Y. (2012). Rat brains also have a default mode network. Proc. Natl. Acad. Sci. U.S.A. 109, 3979–3984.
Luo, F. F., Wang, J. B., Yuan, L. X., Zhou, Z. W., Xu, H., Ma, S. H., et al. (2020). Higher Sensitivity and Reproducibility of Wavelet-Based Amplitude of Resting-State fMRI. Front. Neurosci. 14:224. doi: 10.3389/fnins.2020.00224
Malinen, S., Vartiainen, N., Hlushchuk, Y., Koskinen, M., Ramkumar, P., Forss, N., et al. (2010). Aberrant temporal and spatial brain activity during rest in patients with chronic pain. Proc. Natl. Acad. Sci. U.S.A. 107, 6493–6497. doi: 10.1073/pnas.1001504107
Otti, A., Guendel, H., Wohlschläger, A., Zimmer, C., and Noll-Hussong, M. (2013). Frequency shifts in the anterior default mode network and the salience network in chronic pain disorder. BMC Psychiatry 13:84. doi: 10.1186/1471-244X-13-84
Pan, W., Thompson, G., Magnuson, M., Jaeger, D., and Keilholz, S. (2013). Infraslow LFP correlates to resting-state fMRI BOLD signals. Neuroimage 74, 288–297.
Pan, W., Thompson, G., Magnuson, M., Majeed, W., Jaeger, D., and Keilholz, S. (2011). Broadband local field potentials correlate with spontaneous fluctuations in functional magnetic resonance imaging signals in the rat somatosensory cortex under isoflurane anesthesia. Brain Connect. 1, 119–131. doi: 10.1089/brain.2011.0014
Ries, A., Hollander, M., Glim, S., Meng, C., Sorg, C., and Wohlschläger, A. (2019). Frequency-dependent spatial distribution of functional hubs in the human brain and alterations in major depressive disorder. Front. Hum. Neurosci. 13:146. doi: 10.3389/fnhum.2019.00146
Shi, Z., Wu, R., Yang, P., Wang, F., Wu, T., Mishra, A., et al. (2017). High spatial correspondence at a columnar level between activation and resting state fMRI signals and local field potentials. Proc. Natl. Acad. Sci. U.S.A. 114, 5253–5258. doi: 10.1073/pnas.1620520114
Wang, B., Wang, J., Cen, Z., Wei, W., Xie, F., Chen, Y., et al. (2020). Altered cerebello-motor network in familial cortical myoclonic tremor with epilepsy type 1. Mov. Disord. 35, 1012–1020. doi: 10.1002/mds.28014
Wang, H., Qi, J., Zhang, S., Wang, H., and Morales, M. (2015). Rewarding effects of optical stimulation of ventral tegmental area glutamatergic neurons. J. Neurosci. 35, 15948–15954.
Yuan, B., Wang, J., Zang, Y., and Liu, D. (2014). Amplitude differences in high-frequency fMRI signals between eyes open and eyes closed resting states. Front. Hum. Neurosci. 8:503. doi: 10.3389/fnhum.2014.00503
Zang, Y., He, Y., Zhu, C., Cao, Q., Sui, M., Liang, M., et al. (2007). Altered baseline brain activity in children with ADHD revealed by resting-state functional MRI. Brain Dev. 29, 83–91.
Zou, Q., Zhu, C., Yang, Y., Zuo, X., Long, X., Cao, Q., et al. (2008). An improved approach to detection of amplitude of low-frequency fluctuation (ALFF) for resting-state fMRI: Fractional ALFF. J. Neurosci. Methods 172, 137–141. doi: 10.1016/j.jneumeth.2008.04.012
Keywords: resting state fMRI, local field potential, simultaneous recording, drug manipulation, frequency-dependent pattern
Citation: Qiao Y, Lu H, Yang Y and Zang Y (2024) Neuronal basis of high frequency fMRI fluctuation: direct evidence from simultaneous recording. Front. Hum. Neurosci. 18:1501310. doi: 10.3389/fnhum.2024.1501310
Received: 24 September 2024; Accepted: 17 October 2024;
Published: 31 October 2024.
Edited by:
Zhen Yuan, University of Macau, ChinaReviewed by:
Meng-Yun Wang, University of Bergen, NorwayCopyright © 2024 Qiao, Lu, Yang and Zang. This is an open-access article distributed under the terms of the Creative Commons Attribution License (CC BY). The use, distribution or reproduction in other forums is permitted, provided the original author(s) and the copyright owner(s) are credited and that the original publication in this journal is cited, in accordance with accepted academic practice. No use, distribution or reproduction is permitted which does not comply with these terms.
*Correspondence: Yihong Yang, eWlob25neWFuZ0BpbnRyYS5uaWRhLm5paC5nb3Y=; Yufeng Zang, emFuZ3lmQGh6bnUuZWR1LmNu
Disclaimer: All claims expressed in this article are solely those of the authors and do not necessarily represent those of their affiliated organizations, or those of the publisher, the editors and the reviewers. Any product that may be evaluated in this article or claim that may be made by its manufacturer is not guaranteed or endorsed by the publisher.
Research integrity at Frontiers
Learn more about the work of our research integrity team to safeguard the quality of each article we publish.