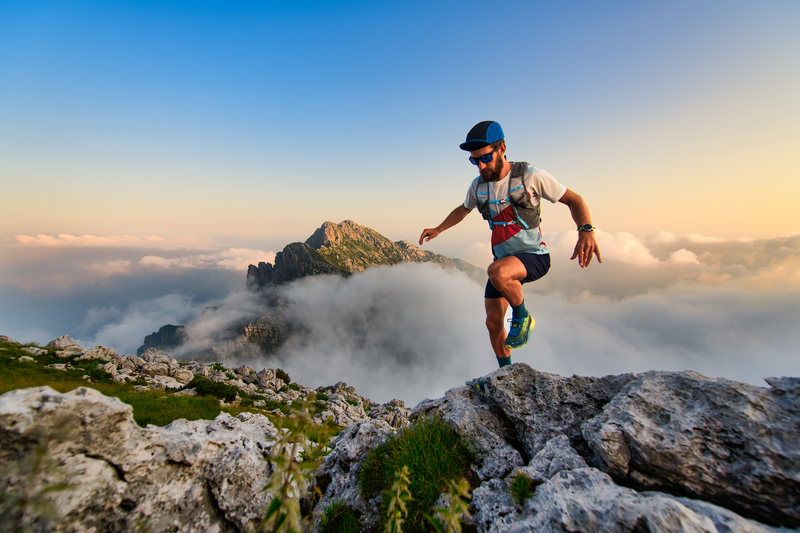
95% of researchers rate our articles as excellent or good
Learn more about the work of our research integrity team to safeguard the quality of each article we publish.
Find out more
ORIGINAL RESEARCH article
Front. Psychol. , 02 April 2025
Sec. Evolutionary Psychology
Volume 16 - 2025 | https://doi.org/10.3389/fpsyg.2025.1531021
This article is part of the Research Topic Hormones and Person Perception View all 7 articles
Introduction: Hormone fluctuations during the menstrual cycle are known to influence a wide variety of cognitive-emotional processes and behavior. Mate choice and changes in attractiveness ratings for faces and voices are often investigated in this context, but research on changes in voice-gender perception independent of attractiveness ratings is rare even though the voice is an essential element in social interactions. For this reason, we investigated the influence of cycle phase and levels of estrogen and progesterone on performance in a voice-gender categorization task. Our expectation was to find a more pronounced other-sex effect, so faster and more accurate reactions for masculine voices, in the follicular (fertile) phase than in the luteal phase.
Methods: We measured 65 healthy, naturally-cycling women, half of them in the follicular phase and the other half in the luteal phase. For the analyses, we used signal detection theory (SDT) measures in addition to reaction times and percent of correct reactions. The study was preregistered after measuring the first 33 participants and prior to any data analyses (https://osf.io/dteyn).
Results: Cycle phase and hormone levels showed no significant effect on reaction time or SDT measures. This was the case both using frequentist analyses and Bayesian statistics. Reaction time was influenced by voice-gender, with faster reactions for feminine voices compared to masculine voices in both cycle phases.
Discussion: Taken together, our results add to the increasing number of studies that do not find an interaction of menstrual cycle phase and reaction to gendered stimuli.
The menstrual cycle, and associated changes in hormonal levels, have been shown to influence a variety of human functions, including cognition, emotion, physiology, and brain activity (Albert et al., 2015; Derntl et al., 2008; Derntl et al., 2013; Haraguchi et al., 2021; Hidalgo-Lopez et al., 2020; Pletzer et al., 2019). The primary hormonal fluctuations throughout the menstrual cycle involve changes in estradiol and progesterone levels. Both hormone levels are low during menses and the early follicular phase. In the later follicular phase, estradiol rises, peaking before ovulation which characterizes high fertility. After ovulation, the luteal phase begins, characterized by a rise in progesterone, which peaks mid-luteal phase, accompanied by a second estradiol increase. Finally, concentrations of both hormones begin to decline to their lowest levels during menses (Farage et al., 2008).
Associated with these hormonal fluctuations, changes in social behavior (Maner and Miller, 2014; Anderl et al., 2015) and mate selection (Puts et al., 2012) can be observed. For example, during the fertile phase of the cycle, women tend to prefer more masculine partners (Penton-Voak et al., 1999; Penton-Voak and Perrett, 2000) whereas during the nonfertile phase of the cycle, masculinity seems to be less important. Especially for faces, studies suggest a menstrual cycle related change in women’s preference with a higher preference for more masculine faces during the late follicular phase and during days prior to and directly after ovulation (Penton-Voak and Perrett, 2000; Johnston et al., 2001; Little et al., 2008). Evolutionary explanations suggest that higher levels of testosterone, which are associated with greater virility (Penton-Voak and Chen, 2004), may be associated with healthier offspring (Jones et al., 2008). This idea is supported by studies finding robust cycle shift effects for evaluation of potential short-term partners as opposed to only small or nul effects on choice of ponential long-term partners (Little et al., 2002; for an extensive review on cycle-shifts for attraction ratings see e.g., Gildersleeve et al., 2014 and Jones et al., 2008). Overall, the gender-categorization of human faces seems to be influenced by a variety of biological factors such as the viewers own gender role and sexual orientation (Luther et al., 2021), their age and experience with human faces (Hillairet de Boisferon et al., 2019) as well as previously activated categorial knowledge (Macrae et al., 2002).
Challenging the idea of a menstrual cycle-shift, a growing body of literature does not find a clear association between cycle phase and preferences for masculinized faces (Jones et al., 2018; Marcinkowska et al., 2016; Peters et al., 2009). Inconsistent findings may be related to methodological shortcomings such as inconsistent methods even within the same lab (Harris et al., 2013), low statistical power, and a lack of objective measures of cycle phase (Jones et al., 2019; Lewis, 2020). Another explanation for inconsistent findings is changes in participants’ visual processing (Garza and Byrd-Craven, 2019) and their visual discrimination abilities over the cycle. These changes have been demonstrated in increased visual sensitivity (Lewis, 2020; Parlee, 1983) and an increased ability to identify facial symmetry during fertile cycle phases (Lewis, 2017). Facial symmetry is generally interpreted as a sign of advantageous genetic traits and health (Fink and Penton-Voak, 2002; Foo et al., 2017), associated with a strong preference for symmetrical faces, independent of conscious detection (Little and Jones, 2006) thus substantiating the idea of evolutionary mating strategies exerting a strong influence on face perception and preference. Subconscious changes in the ability to identify facial symmetry or asymmetry may contribute to variations in face preference over the cycle. These hormonal variations not only affect preference and perception of faces, but also the appearance and attractiveness of women’s own faces. Through subtle changes in shape and skin structure, female faces are perceived as more attractive during the fertile phase (Bobst and Lobmaier, 2012; Puts et al., 2013).
While much of the literature focuses on changes in face preference during the menstrual cycle, less attention has been paid to other mating cues. One such cue is the human voice, which, like facial features, plays a key role for both mating (Hughes and Puts, 2021; Pisanski et al., 2018) and other social interactions (Guldner et al., 2020; Hellbernd and Sammler, 2016; McGettigan, 2015). The voice conveys pertinent characteristics which allow us not only to identify known voices, but also to characterize a stranger’s age, gender (Gallup and Frederick, 2010) or even health status (Arnocky et al., 2018). To do so, people rely mainly on two properties of the voice: the fundamental frequency (F0) and formant frequencies (Hillenbrand and Clark, 2009). The F0 is the average rate of vibration of the vocal folds per second and is closely related to the perceived overall pitch of a voice, while the formant frequencies are the result of the movement of the vocal apparatus during formatting vowels and consonants (Goldstein, 2014).
For women’s own voices, a robust association between menstrual cycle phase and voice quality can be seen. During phases of lower estradiol, the voice quality decreases in naturally cycling women, showing higher tension, roughness and instability (Arruda et al., 2019; Raj et al., 2010). Additionally, changes in F0 have been reported across the cycle, though with inconsistent direction (Bryant and Haselton, 2009; Fischer et al., 2011; Karthikeyan and Locke, 2015; Lã and Polo, 2020). However, research findings on changes in voice preference for male voices associated with the menstrual cycle are mixed. Whereas some studies find a clear inclination of women to more masculine voices during fertile cycle phases (Feinberg et al., 2006; Puts, 2005), other studies fail to find an effect of hormonal fluctuations on voice preferences (Jünger et al., 2018). These mixed results on the influence of the menstrual cycle on voice preference parallel the mixed results on face preference and perception.
However, studies on changes in voice perception over the cycle are still scarce. Nonetheless, this is an important factor in understanding the underlying mechanisms of potential preference changes, as illustrated by the previously described influences on face perception. Therefore, the goal of this study is to shed light on potential differences between cycle phases in voice-gender categorization as an important part of social interactions, where not only visual facial cues but also vocal information has to be integrated into a multisensory perception that guides behavior.
According to previous studies, similar to the perceived gender of faces, voice-gender categorization is influenced by both listeners’ gender (Junger et al., 2013; Smith et al., 2018) and sexual orientation (Smith et al., 2019). One way to study voice-gender perception is via a voice-gender categorization paradigm which uses words spoken by both natural male and female speakers alongside with voices morphed toward the opposite sex to investigate a person’s reaction to increasingly ambiguous stimuli. This kind of paradigm allows for the analysis of both the accuracy of responses and the response bias, meaning the inclination to a certain response in ambiguous situations. Overall, the aforementioned studies found an opposite sex effect for response accuracy in highly ambiguous trials together with a tendency for a response bias toward the opposite sex in those trials both heterosexual men and women (Smith et al., 2018; Junger et al., 2013; Junger et al., 2014). For both homosexual men and women however, response bias in ambiguous trials show a pattern more similar to heterosexual men, underlining an association of sexual orientation (Smith et al., 2019). Strikingly, the effect of listeners’ own sex on voice-gender categorization seems to be less robust in women than in men, as response patterns across studies show greater variance for women than they do for men. This may be related to hormonal fluctuations associated with the menstrual cycle. In fact, previous studies on voice-gender categorization have not taken the menstrual cycle phase into account (Smith et al., 2018).
Due to the high relevance of voices as cues for social interaction and mating and because of the influence of female sex hormones on mating cues, we first expected to find a behavioral difference between women in different cycle phases. Because the late follicular phase is associated with higher fertility and a greater preference for masculinity in heterosexual women, we expected a stronger other-sex effect in that cycle phase for response accuracy and reaction time. Secondly, we expected a difference in response bias, meaning the inclination to categorize a voice rather as masculine or feminine when the categorization is unclear. Specifically, we expected the response bias to be influenced by the estimated cost of a wrong decision. According to error management theory (Haselton and Buss, 2000), the response bias can be expected to be influenced by the estimated costs of incorrect decisions. The favored decision should be the one that results in the less costly error, so the smallest loss of resources, if the decision was wrong. Applied to female mate choice, it is unclear if the cost is higher for mistaking a male for a female and thereby missing a potential mate or if it is higher for mistaking a female for a male and thereby investing in a non-reproductive mate (Johnston et al., 2008). Thus, this is a more exploratory question, and we do not have prior assumptions for the direction of the difference. The influence of choice costs is expected to be more pronounced in the follicular phase since mating is more likely to result in offspring. Hence, we expect a stronger response bias in the follicular compared to the luteal phase.
All procedures were in accordance with the Declaration of Helsinki and were approved by the Independent Ethics Committee of the RWTH Aachen Faculty of Medicine. All participants gave written informed consent and received financial compensation of 10 €.
A total of 78 naturally cycling cisgender heterosexual women between 18 and 35 years (M = 25.48, SD = 4.11) participated in the study. Thirty-three of these datasets were collected in the context of an earlier study (unpublished data) but have not been analyzed before. The required sample size was 62 as calculated a priori using G*Power 3.1 (Faul et al., 2007). Based on the mixed results on associations of voice perception and cycle phase, as described in the introduction, we expected a small effect (Cohen’s f = 0.15) with a power of β = 0.80 and an α error probability of 0.05. In the context of mixed results and varying degrees of uncertainty, sample sizes in studies on the influence of cycle phase on perception and reaction to cues with mate value vary quite substantially, ranging from 50 or less (Oinonen and Mazmanian, 2007; Sanders and Wenmoth, 1998; Rosenberg and Park, 2002) to 200 or more (Jones et al., 2018; Jünger et al., 2018; Stern et al., 2021) and yield mixed results independent of sample size. Therefore, we decided to base our sample on the basic power calculation described above.
Participants were recruited using public flyers and online postings. All participants reported a regular menstrual cycle and did not take any contraceptives. Participants were recruited to be either in the follicular (N = 31) or the luteal (N = 34) phase (as determined by self-reports and hormonal profiles; see below) at the time of measurement. Only women whose reported menstrual cycle phase matched the cycle phase measured by blood samples were included. Consequently, 13 participants were be excluded from all analyses, because their self-reported cycle phase differed from the cycle phase determined, making a clear classification impossible. Both progesterone (t(63) = −8.25, p < 0.001) and estradiol (t(63) = −6.91, p = < 0.001) levels were significantly different between the two groups. There was no significant difference in age (t(63) = −1.45 p = 0.152) or years of education (t(62) = −1.29, p = 0.202) between groups. Demographic information and hormonal levels (M ± SD) are presented in Table 1.
Prior to enrolment, each woman took part in a telephone interview to assess eligibility for the study and to assess the current day of the cycle. Exclusion criteria were hearing or speech impairment, use of oral contraceptives or other hormones, diseases or medications known to affect the endocrine system, pregnancy, or breastfeeding, and neurological or mental disorders. Physical illness, medication and pregnancy were assessed by self-report. The absence of mental disorders was assessed using the clinical version of the structured clinical interview for DSM-5 (SCID-5 CV; First et al., 2016). One participant had to be excluded due to an assumed presence of a mental illness based on the SCID interview. To control for a potential influence of sexual orientation, only heterosexual women were included in this study. Sexual orientation was assessed via self-report.
To determine the cycle phase, participants were asked for the first day of their last menses during the telephone interview. To schedule the measurement date, they had to inform the study team via email as soon as their next menses started. If the time frame between both menses fell into a regular cycle (23–35 days), participants were randomly assigned to either the follicular phase group (7–11 days after onset of the current menses) or the luteal phase group (17–34 days after onset of the current menses). Since the cycle phase is often inaccurately self-reported (Farrar et al., 2015), we confirmed the estimated cycle phase by assessing levels of progesterone (P) and estradiol (E) via blood serum samples, assessed using ElektroChemiLumineszenz-ImmunoAssays (ECLIAs). The reference range for estradiol was 20.5–233 pg./mL for the follicular and 30.2–305 pg./mL for the luteal phase. For progesterone, the reference range for the follicular phase was <0.05–0.323 ng/mL and for the luteal phase 0.537–20.9 ng/mL. Progesterone levels below the detection limit (0.05 ng/mL) were entered as half the detection limit (0.025 ng/mL). In our sample, this was the case for 4 women, all of them in the follicular phase. For higher reliability of cycle phase determination, people not involved in data collection or analyses rated the cycle phase for each woman based on hormonal levels according to reference ranges. Additional information about the procedure can be found in the Supplementary information 1. This combined approach of forward counting of cycle days and assessing the level of reproductive hormones allows us to substantially reduce the uncertainty of true cycle phase for each participant. This procedure enables detecting effects with a much smaller sample size than usually required in studies using counting methods alone (Gangestad et al., 2016; Jonge et al., 2019; Maki et al., 2002).
At the beginning of the session, each woman completed a short interview including questions on demographic data, current cycle phase and exclusion of hormone intake. This interview was followed by a screening version of the SCID-5 to exclude mental disorders, and a blood withdrawal (ca. 7 mL) to assess blood serum hormone levels. Due to practical reasons, we could not control for time of day for the blood withdrawal. Subsequently, participants completed a voice-gender categorization paradigm. An extensive description of the paradigm can be found in Junger et al. (2013). The stimuli consisted of 6 trisyllabic, neutral nouns, each spoken by 5 male and 5 female speakers. The resulting 60 words were each morphed 2, 4 and 6 semitones (st) toward the speaker’s other sex by adjusting the pitch contour and the formant structure as a reflection of vocal tract length accordingly using the “change gender” function implemented in the software Praat Version 5.2.03 (Boersma and Weenink, 2010). These final 240 words were presented pseudorandomized in a way that no speaker and no word was presented consecutively. The presentation was divided into 80 blocks, each consisting of 3 words spoken by the same sex and morphed to the same degree. The stimuli were delivered via headphones using the software Presentation Version 21.1 (Neurobehavioral Systems, Inc., 2019). Participants were instructed to categorize the speaker’s sex for each stimulus as male or female as fast as possible by pressing the number key “7” for male speakers and number key “8” for female speakers on a laptop keyboard.
Behavioral data were analyzed using Matlab2019a (MathWorks, Inc., 2019). Sociodemographics, group differences and correlations were analyzed using the software R version 4.1.2 (RStudio Team, 2021). The study has been preregistered after measuring the first 33 participants and prior to any data analyses.1
Reaction time differences were calculated using three mixed-model ANOVAs, with overall reaction time, reaction time for correct trials and reaction time for incorrect trials as dependent variables, respectively. The models contained the between-subjects factor cycle phase (follicular vs. luteal phase) and the two within-subject factors voice-gender (masculine vs. feminine) and morphing level (0, 2, 4, or 6 st morphing) as independent variables. For significant effects, post-hoc pairwise comparisons were calculated. All post-hoc comparisons were Bonferroni-corrected to account for multiple testing. Effect sizes were calculated using generalized eta squared.
The frequentist approach to statistics has been increasingly criticized (Jarosz and Wiley, 2014). Major points of criticism have been the arbitrariness of a p-value of 0.05 as a cut-off as well the influence of sampling and sample size on the p-value, which can lead to significant results, that are only valid within the given sample (for an extensive overview, refer to Wagenmakers, 2007). Therefore, we decided to validate effects of cycle phase using Bayesian ANOVAs to assess the likelihood for H0 (cycle phase does not influence task performance) over H1 (there is a significant difference between cycle phases) using the Bayes factor (BF01). Conventions for interpreting the resulting BF are provided by Raftery (1995) and define a BF between 1 and 3 as weak, between 3 and 20 as positive, between 20 and 150 as strong and larger than 150 as very strong. Bayesian analyses were conducted using the BayesFactor package for R. For all models 10,000 iterations were run, participants were included as a random factor.
To allow for a more detailed investigation of the underlying mechanisms of potential performance differences, we employed signal detection theory (SDT) measures. The SDT is a well-established model for decision making processes (Lynn and Barrett, 2014; Stanislaw and Todorov, 1999). It was originally developed for signal vs. noise psychophysical perception tasks (Green and Swets, 1974) and differentiates between discriminability (i.e., ability to detect a target stimulus from background events) and a response bias (i.e., a tendency toward a certain response independent of the stimulus) (Stanislaw and Todorov, 1999). SDT measures have been employed in previous studies using the same voice-gender categorization paradigm and proved to be suitable for detecting differences in gender categorization ability (Junger et al., 2013; Smith et al., 2018; Smith et al., 2019; Junger et al., 2014). To calculate SDT measures for our paradigm, male voices were defined as target and female voices were defined as noise. This definition is arbitrary and chosen to match previous studies ability (Junger et al., 2013; Smith et al., 2018; Smith et al., 2019; Junger et al., 2014). This results in a definition of correct reactions to male voices as hits and incorrect reactions to male voices as misses. For female voices, correct reactions are defined as correct rejections, while incorrect reactions are defined as false alarms.
Since the Shapiro–Wilk test revealed deviances from normality of the data, we used non-parametrical measures. These measures were A′ for discriminability (Equation 1) and B″D for response bias (Equation 2) and can be calculated using the following formulas (Pallier, 2002):
A′ ranges from 0 to 1 with higher values indicating better discriminability (i.e., a high rate of correct reactions to both male and female speakers) and values near 0.5 indicating performance on chance level (Pallier, 2002). B″D ranges from −1 to 1 with a value of 0 indicating no response bias, positive values indicating in this case a tendency to categorize a voice as feminine and negative values indicating a tendency to categorize a voice as masculine. As each trial must be categorized as either a hit/correct rejection or a miss/false alarm for SDT analysis, non-response trials were not considered.
To test for differences between groups and conditions, three mixed-model ANOVAs were performed with cycle phase (follicular vs. luteal phase) as between-subjects factor and morphing level (0, 2, 4, 6) as within-subject factor. A′ was the dependent variable for the first ANOVA, whereas B″D was the dependent variable for the second. Additional to the SDT measures, a mixed-model ANOVA with percent of correct responses as dependent variable was calculated. While the normality assumption was not fulfilled, homoscedasticity was given, therefore the results of a mixed-model ANOVA can still be assumed to be robust despite the non-parametric distribution (Harwell et al., 1992). For all variables where Mauchly test indicated a lack of sphericity, degrees of freedom were corrected using Greenhouse–Geisser adjustment (Greenhouse and Geisser, 1959).
One of the major drawbacks of the SDT is that it does not take RT into account. Therefore, we assessed potential associations between SDT values and RT in both cycle phases using correlations. The Shapiro–Wilk-Test indicated a lack of normal distribution for SDT values (p > 0.05), so Kendall’s τ was chosen as a measure of correlation. SDT measures and mean reaction times for male and female speakers were correlated over all morphing levels. To control for multiple testing, Holm correction was used (Holm, 1979).
To allow us to look at hormonal influences and their interactions with morphing level and the voice-gender regardless of cycle phase, we ran multiple regressions with response bias B″D and with RT as dependent variables and hormone levels (P and E) as independent variables. This approach gave us the possibility to include hormone levels as a continuous variable as regression analyses have a higher statistical power than ANOVAs. To identify influential predictor variables, we compared increasingly complex models by stepwise adding predictors and interactions. For both B″D and RT we started with simple models including only variables as determined by the design of the paradigm: we started with morphing level, followed by voice-gender as a predictor for RT. Finally, hormone levels were added as further predictors. Additive models as well as interactions were tested. Since our previous analyses did not yield a significant difference between the two cycle groups, we did not include cycle phase as a factor. Model fits were compared based on Akaike information criterion (AIC) and Bayesian information criterion (BIC) values. AIC and BIC are both calculated using a model’s maximum likelihood estimate and correcting for number of model parameters (Vrieze, 2012). A major difference between both criteria is that BIC is growing more restrictive with an increasing number of parameters. Thus, BIC is more consistent, as long as the true model has a finite number of parameters and is one of the models, that are tested. In cases, where the model is more complex, the AIC is preferred (Vrieze, 2012). Due to the different calculations, we included both measures in our model selection. Additionally, we tested for significant differences between the models using Chi-Square test.
For overall RT, we found significant main effects of voice-gender (F(1, 63) = 44.18, p < 0.0001, η2 = 0.023) and morphing level (F(3, 189) = 206.38, p < 0.0001, η2 = 0.193) as well as an interaction for both variables (F(3, 189) = 39.96, p < 0.0001, η2 = 0.022), but no main effect for cycle phase (F(1, 64) = 2.15, p = 0.148). Post-hoc comparisons showed lower reaction times for feminine voices compared to male voices (t(259) = −8.20, p < 0.001). For morphing level, we found significant RT differences for each morphing level compared to another (all p < 0.001). Post-hoc tests for interaction effects of voice-gender and morphing level showed a significant influence of voice-gender on RT for each morphing level with faster RTs for feminine voices for 4 st (F(1, 64) = 52.32, p < 0.0001, η2 = 0.065) and 6 st morphing (F(1, 64) = 100.94, p < 0.0001, η2 = 0.085).
Comparable to overall RT, RT for correct trials also showed significant effects of voice-gender (F(1, 63) = 38.67, p < 0.0001, η2 = 0.027) and morphing level (F(3, 189) = 231.88, p < 0.0001, η2 = 0.204) as well as an interaction for both variables (F(3, 189) = 27.1, p < 0.0001, η2 = 0.018). Again, post-hoc pairwise comparisons showed faster RT for feminine voices over all morphing levels (t(259) = −8.21, p < 0.001), significant differences between each morphing level (all p < 0.001) and a significant interaction of voice-gender and morphing level for 4 st (F(1, 64) = 69.33, p < 0.001, η2 = 0.082) and 6 st (F(1, 64) = 33.28, p < 0.001, η2 = 0.061) morphing (see Figure 1) with faster RT for feminine voices. We did not find any significant RT effects of incorrect reaction trials (all p > 0.05).
Figure 1. Reaction time as a function of morphing level and voice gender for all trials (left) and for correct reactions (right) across cycle phase. f = female speaker, m = male speaker. Reaction time is depicted in ms. The asterisk on the upper line indicates significant differences between all morphing levels. Results of the pairwise comparisons are listed in the Supplementary material.
To substantiate the null effect for menstrual cycle phase, we conducted a Bayesian ANOVA. Results for overall RT (BF01 = 1.975), RT for correct responses (BF01 = 2.038) and RT for incorrect responses (BF01 = 2.922) supported the H0, though only to a weak extent.
Results of the mixed-model ANOVA revealed a significant main effect of morphing level for both discriminability A′ (F(1.17, 73.65) = 460.9, p < 0.001, η2 = 0.829) and response bias B″D (F(2.27, 143.17) = 9.43, p < 0.001, η2 = 0.046). Pairwise comparisons showed significant differences between all morphing levels (see Figure 2) with decreased values for A′ for higher morphing levels (all p < 0.001). Pairwise comparisons for B″D showed differences for 0 compared to 2st morph (t(64) = 2.88, p = 0.033), 2st to 6st morph (t(64) = −5.03, p < 0.001) and 4 st to 6 st morph (t(64) = −5.35, p < 0.001). Results of the pairwise comparisons are listed in the Supplementary material.
Figure 2. Differences in discriminability A’ (left) and response bias B’’D (right) for different morphing levels. The asterisk on the upper line indicates significant differences between all morphing levels.
There were no significant main effects of cycle phase (FA’(1, 63) = 2.55, pA’ = 0.115, η2 = 0.013; FB″D (1, 63) = 0.24, pB″D = 0.625, η2 = 0.003) and no interaction effects of cycle phase and morphing level (FA’(1.17, 73.65) = 2.02, pA’ = 0.157, η2 = 0.021; FB″D (2.27, 143.17) = 1.04, pB″D = 0.364, η2 = 0.005).
In line with that finding, Bayesian ANOVA results were in favor of the H0 for both A′ (BF01 = 5.263) and B″D (BF01 = 3.124).
In line with SDT measures, for percent of correct answers we found a main effect for morphing level (F(1.87, 112.14) = 998.60, p < 0.001, η2 = 0.679), but no effect for voice-gender or cycle phase and no interaction effects (all p > 0.05). Bayesian ANOVAs also favored H0 regarding effects of menstrual cycle phase (BF01 = 7.136). Post-hoc tests showed significant differences in percent of correct answers for each morphing level compared to another (all p < 0.001).
Within the follicular phase group, we found negative correlations between A′ and mean RT for both feminine (rτ = −0.21, p = 0.003) and masculine (rτ = −0.35, p < 0.001) voices. In the luteal phase we also found negative correlations between A’ and mean RT for feminine (rτ = −0.26, p < 0.001) and masculine (rτ = −0.35, p < 0.001) voices. Additionally, we found a positive correlation between B″D and mean RT for masculine voices (rτ = 0.20, p = 0.003) that did not become apparent in the follicular phase (rτ = 0.06, p = 0.545).
For B″D model comparisons indicated the best model fit when only morphing level was included as a predictor (see Table 2). This model showed a significant influence only for 2 st Morphing (t(192) = −3.67, p < 0.001).
For RT, the model including the interaction of speaker and morphing level showed the best model fit according to AIC and BIC (see Table 3). All morphing levels influenced RT significantly as well as the interaction of speaker and morphing level for 4 st and 6 st morphing (see Table 4).
Table 4. Model estimates for the model with the best model fit (upper model) and the simplest model including hormones estrogen and progesterone (lower model).
As a comparison, we also took a closer look at the second-best model, which includes estrogen and progesterone. Again, the model showed a significant influence of voice-gender and morphing level, but no influence of either hormone level (see Table 4).
Model estimates for all models can be found in the Supplementary information.
Voice-gender categorization is an important part of everyday social interaction, further influencing mate choice and preferences (Weston et al., 2015). Despite the growing body of evidence, studies investigating the influencing factors on the ability to categorize a voice as masculine or feminine remain scarce. Important influences could be the menstrual cycle phase and female sex hormone profile. Hence, we investigated the associations of menstrual cycle phase with the performance in a voice-gender categorization task using masculine and feminine voices which were morphed toward the other sex, resulting in increasingly ambiguous stimuli.
In contrast to our hypotheses, we did not find any significant differences between cycle phases (follicular vs. luteal) for reaction times (RT), discriminability or response bias. These potential null effects were assessed using different statistical approaches, increasing the likelihood of the null hypothesis being true. In accordance with earlier studies, we observed an increase in RT as well as a decrease in discriminability and correct responses with increasing morphing levels (Smith et al., 2018; Smith et al., 2019). In other words, with increasing ambiguity of the voices’ sex, participants needed more time to decide and were less accurate in doing so. This effect most likely reflects a higher cognitive workload with increasing stimulus ambiguity. Thus, while accuracy was generally high, the paradigm also elicited consistent effects of increasing task difficulty on response times and choices. This may also increase behavioral differences between menstrual cycle phases if present.
Congruent with the findings for reaction times, we did not observe a significant difference between menstrual cycle phases for voice-gender categorization accuracy within our sample. In addition, E and P do not seem to show associations with voice-gender categorization, as investigated by regression models that treated these two variables as continuous predictors. In other studies, estradiol is associated with increased preferences for higher masculinity, both for voices (Feinberg et al., 2006) and faces (Hromatko et al., 2008). Our results did not show such straightforward connection between hormone levels and reaction to male voices. However, it is important to note that fluctuations in progesterone and estradiol are not the only possible explanation for behavioral changes across the cycle. Thus, potential effects could also be driven by an interplay of different sex hormones, which were not investigated in this study such as testosterone, which also influences changes in face preferences (Welling et al., 2007; Niu and Zheng, 2020). Another possible explanation is the influence of other variables affected by the menstrual cycle, such as mood (Cohen et al., 1987; Collins et al., 1985; Pierson et al., 2021) or attention (Pletzer et al., 2017; Thimm et al., 2014). These changes can in turn influence the response behavior.
In addition to the overall effect of morphing level, we found faster RTs for feminine voices compared to masculine voices with higher morphing levels in both cycle phases. This finding also replicates earlier findings on voice-gender categorization, which identified faster reaction times for feminine compared to masculine voices regardless of sex (Junger et al., 2014) or in female participants for morphed voices (Smith et al., 2018; Smith et al., 2019). A possible explanation for faster RTs for female voices could be a higher sensitivity for higher frequencies and specifically female voices in general (Lattner et al., 2005) resulting in a faster reaction to higher pitched voices. As the signal detection theory does not take reaction time into account, this slightly higher sensitivity could accelerate the responses to feminine voices while SDT measures can still be expected to show an other-sex effect for discriminability and response bias.
Regarding response bias, an overall tendency to categorize a voice as male – especially with higher uncertainty - became apparent, thus supporting the idea of an other-sex bias in heterosexual women. The resulting distribution resembles an U shape with smaller biases for original voices and the highest morphing level (6 st) and higher biases for ambiguous voices (2 st and 4 st) with the most pronounced effects for 2 st morphing. While this stronger tendency for 2 st morphing has been found before (Junger et al., 2013, 2014), the underlying mechanism is not yet clear. A possible explanation is the slight ambiguity of the stimuli which increases the effect of the reaction bias. While gender-categorization for unmorphed voices is in most cases an easy task, increased ambiguity heightens the cognitive processing load (Junger et al., 2013). The highest response bias for 2 st morphed voices could reflect the automatic use of heuristics to lighten the processing load (Gigerenzer and Gaissmaier, 2011). As the morphing level increases, overall performance decreases and categorization develops into a more conscious decision process, making the influence of reaction bias less pronounced. Since our sample showed a high variance across all morphing levels, our results must be interpreted with caution.
When looking at the menstrual cycle phases separately, we found a correlation between response bias and reaction time for masculine voices present in the luteal phase, even though there was no significant difference in response bias between phases. A possible explanation could be the influence of cycle phase on hearing sensitivity. Studies on hearing sensitivity could show that sensitivity is higher in the follicular phase than in the luteal phase (Emami et al., 2018; Williamson et al., 2020). A proposed explanation is an enhancing effect of estradiol on hearing which is influenced by the interaction of estradiol with progesterone levels (Williamson et al., 2020). Since there was no interaction of hormone levels in our data, this seems an unlikely explanation for our results. A more likely explanation could be a blunting effect of progesterone on the enhancing effect of estradiol (Al-Mana et al., 2010). Therefore, our results could hint toward a weaker influence of the response bias on reaction times in phases with higher acoustic sensitivity. As we did not assess general hearing sensitivity in this study, this explanation remains speculative and needs to be explored in future studies. Moreover, since interindividual differences in hormone levels are higher than intraindividual fluctuations (Gann et al., 2001), the finding above could be driven by individual hormone profiles that are not strictly linked to cycle phase.
In an exploratory analysis, we looked at regression models in which we examined the potential effect of female sex hormones (P and E) regardless of cycle phase to check for an overall effect of hormone levels. Model comparisons showed that there was no effect of hormones on RT or response bias. Significant predictors of those models were only morphing level and voice-gender. Thus, for the chosen sample, even dimensional models (which have more power compared to ANOVAs) suggest that female sex hormones do not influence sex-voice categorization as measured by RT, and response bias. In line with our previous results, we did not find a clear influence of either cycle phase or sex hormone levels on both accuracy and speed of voice-gender categorization.
Due to the manifold influences on hormonal effects, some limitations of the current study should be considered. For the current sample, we did not differentiate between early and late follicular phase. This may have impacted our results, as estradiol levels change from early to late follicular phase (Sacher et al., 2013). Similarly, we did not account for early, mid, or late luteal phase. However, previous studies show, that differences across cycle phases can be detected using this broader differentiation (Penton-Voak and Perrett, 2000; Johnston et al., 2001; Thimm et al., 2014; Nielsen et al., 2013; Senior et al., 2007). Furthermore, using hormone blood serum levels determined by ECLIAS entails additional uncertainties. Despite their high specificity and common use, a known limitation for all Immunoassays is the potential cross-reactivity with compounds similar to the target hormone, which could potentially influence the calculated blood serum level (Krasowski et al., 2014). Additionally, due to the wider reference range for cycle-phases, misclassification cannot be ruled out, especially in cases where blood serum levels are close to boundaries between cycle phases. Since we only included women in our study, who were rated as being unambiguously within one cycle phase by two independent raters and those ratings had to correspond with the women’s self-reports, we estimate the likelihood of misclassification within our sample as rather small though.
For feasibility reasons, our study used a between-subject design. Within-subject designs are more suitable at detecting changes over the menstrual cycle (Jones et al., 2019; Schmalenberger et al., 2021), so the current study design could have missed subtle changes over the cycle as well as the effect of intraindividual hormone fluctuations. The use of between-subject designs for investigating menstrual-cycle effects is suspected to have a substantial effect on the validity of cycle-phase determination and thus on the statistical power attainable. Referring to estimations by Gonzales and Ferrer (2016), the use of a between-subject design inevitably leads to a higher required sample size, independent of accuracy of cycle-phase determination accuracy. Future studies on voice-gender categorization are therefore advised to use a within-subject design to achieve more robust results, whenever possible. Further methodological precision could be achieved by directly measuring ovulation, using for instance LH tests in a standardized way as recommended by Blake et al. (2016).
Besides, additional hormones may also be considered in their interaction possibly influencing voice-gender categorization. For example, testosterone and thyroid hormones both influence cochlear development and hearing and may be investigated in future studies on voice-gender categorization tasks (Frisina et al., 2021). This is especially important considering that for feasibility reasons we could not measure all participants at the same time of day. Since all sex hormones present a specific circadian rhythm (Dabbs and de La Rue, 1991; Rahman et al., 2019), potential effects of time of day on the measured blood-serum level as well as potential interactions of progesterone and estradiol with the aforementioned additional hormones cannot be included in the analyses.
Looking at the manifold influences involved in analyses of hormonal effects, the sample size used in our study was likely not sufficient after all. Each factor brings a certain degree of variance into the analyses that cannot be accounted for in a regular power-analysis for between-subject comparisons. Therefore, sample size calculations should be based on methods considering the specifics of menstrual-cycle research (e.g., Gangestad et al., 2016; Gonzales and Ferrer, 2016; Schmalenberger et al., 2021). The shortcoming of not taking those specifics into account likely lead to a decrease in statistical power and therefore to a higher probability of accepting the null hypothesis while it was not true. Thus, we strongly recommend replicating the study design using a higher number of participants as well as a within-subjects design to increase statistical power.
As the originally spoken words were manipulated for the paradigm, especially the higher morphing levels sounded less natural, which could lead to diminished ecological validity and thus to a weakened influence of mechanisms important for mate choice. Additionally, stimuli were controlled for and changed in F0, but not in degree of breathiness, which could further influence voice-gender perception (Whitling et al., 2023). Nevertheless, considering that the exact same paradigm was used multiple times before and robustly showed effects of both gender and sexual orientation (Junger et al., 2013; Junger et al., 2014; Smith et al., 2018; Smith et al., 2019), we do assume this effect does not exert major influence on our results, especially in view of various studies successfully using mechanically morphed voices to examine even more complex social cues such as perceived dominance (e.g., Brown et al., 1973; Feinberg et al., 2005; Wang et al., 2018).
As mentioned earlier, there is a growing number of studies that do not find clear influences of menstrual cycle phase on female behavior such as mating behavior (Stern et al., 2021; Stern et al., 2022; Holzleitner et al., 2022). In two different studies, Harris (2011, 2013) tested different methods commonly used in studies on menstrual cycle effects on attractiveness ratings for male faces but did not find any influence of cycle phase on the participants’ ratings. Likewise, eye-tracking studies testing the influence of hormonal changes on attractiveness ratings for male faces and bodies did not find associations with cycle phase either (Garza and Byrd-Craven, 2019; Garza and Byrd-Craven, 2023). Furthermore, meta-analyses on preference shifts across sensory modalities (i.e., faces, voices and scent) showed only a few effects, which were likely due to an imprecise definition of the fertile phase (Wood, 2016; Wood et al., 2014). Nevertheless, there is still an ongoing debate about the presence or absence as well as the magnitude of the influence of menstrual cycle phase on behavior, as other studies using similar tasks still find large effects on similar questions (Jones et al., 2008). Additional disagreement arises through divergent interpretations of the increasing number of null effects (Wood, 2016; Gangestad, 2016).
Suspected reasons for an overestimation of a cycle dependent shift in attractiveness ratings are a high publication bias as well as a high degree of freedom when it comes to researchers’ decisions in sampling, study design and methods. Thus, confirmatory hypothesis testing can lead to arbitrary exclusion of participants, a broader definition of the fertile window and an inconsistent choice of moderators across analyses to achieve significant results (Wood et al., 2014; Harris, 2013). However, some of the studies presenting null effects show similar methodological shortcomings and are faced with criticism concerning sample sizes and statistical power (e.g., DeBruine et al., 2010) and thus regarding the interpretation of null effects (Gildersleeve et al., 2013). Moreover, studies seem to show only sparse evidence for the evolutionary perspective of female mate selection being driven by increasing chances for optimal offspring (Harris, 2013). One possible explanation is the negligence of socio-economic and sociosexual influences on mate choice which can be expected to play a stronger role than potential hormonal influences (Wood et al., 2014; Albert et al., 2018). Taken together, our results fall in line with the accumulating findings, that the associations of menstrual cycle phase with female (mating) behavior found in earlier studies might be a less robust effect than originally assumed, thus contributing to the ongoing debate about factors that might influence the complex interplay of sex hormones and behavior.
In conclusion, in this first study on associations of menstrual cycle phase with performance in voice-gender categorization, we did not find any significant differences between cycle phases for discriminability, response bias or reaction time in a between-subject design. Investigating effects regardless of cycle phase also did not show any significant associations of hormone levels and performance. Therefore, there might be no straightforward association between menstrual cycle phase or sex hormone level and voice-gender perception, supporting a growing body of literature reporting no or only subtle effects of menstrual cycle phase on female mating behavior. However, interpretation of results is impeded by multiple factors relevant not only in analyses, but also in study design for menstrual cycle research, such as sampling method and cycle phase confirmation. Keeping those drawbacks in mind, our study seems to support the idea, that earlier studies on the matter might have overestimated the influence of sex hormone fluctuations on women’s behavior. Further research in this line of research is needed to shed light on the interplay of hormones, socioeconomic factors, and behavior. The diverging results thus far highlight the importance of standardized best practices guidelines for sampling, sample size and interpretation of results for menstrual cycle research to minimize confounding factors and allow for a higher comparability of results across studies.
The original contributions presented in the study are included in the article/Supplementary material, further inquiries can be directed to the corresponding author.
The studies involving humans were approved by The Independent Ethics Committee of the RWTH Aachen Faculty of Medicine. The studies were conducted in accordance with the local legislation and institutional requirements. The participants provided their written informed consent to participate in this study.
SF: Data curation, Formal analysis, Investigation, Visualization, Writing – original draft, Writing – review & editing. EB: Writing – review & editing, Writing – original draft. BD: Conceptualization, Writing – review & editing, Writing – original draft. UH: Conceptualization, Funding acquisition, Supervision, Writing – review & editing, Writing – original draft. PH: Formal analysis, Project administration, Supervision, Writing – review & editing, Writing – original draft.
The author(s) declare that financial support was received for the research and/or publication of this article. This work has been funded by the DFG (HA 3202/7-3 and HA 3202/7-4) and supported by the International Research Training Group the Neuroscience of modulating aggression and impulsivity in psychopathology (IRTG-2150) of the German Research Foundation (DFG).
This work was supported by the Brain Imaging Facility of the Interdisciplinary Centre for Clinical Research (IZKF) Aachen within the Faculty of Medicine at the RWTH Aachen University. Furthermore, we want to thank Mirjam Leitner and Franziska Feldmeyer for their help with data collection.
The authors declare that the research was conducted in the absence of any commercial or financial relationships that could be construed as a potential conflict of interest.
The author(s) declared that they were an editorial board member of Frontiers, at the time of submission. This had no impact on the peer review process and the final decision.
The authors declare that no Gen AI was used in the creation of this manuscript.
All claims expressed in this article are solely those of the authors and do not necessarily represent those of their affiliated organizations, or those of the publisher, the editors and the reviewers. Any product that may be evaluated in this article, or claim that may be made by its manufacturer, is not guaranteed or endorsed by the publisher.
The Supplementary material for this article can be found online at: https://www.frontiersin.org/articles/10.3389/fpsyg.2025.1531021/full#supplementary-material
Albert, G., Pearson, M., Arnocky, S., Wachowiak, M., Nicol, J., and Murphy, D. R. (2018). Effects of masculinized and feminized male voices on men and Women’s distractibility and implicit memory. J. Individ. Differ. 39, 151–165. doi: 10.1027/1614-0001/a000259
Albert, K., Pruessner, J., and Newhouse, P. (2015). Estradiol levels modulate brain activity and negative responses to psychosocial stress across the menstrual cycle. Psychoneuroendocrinology 59, 14–24. doi: 10.1016/j.psyneuen.2015.04.022
Al-Mana, D., Ceranic, B., Djahanbakhch, O., and Luxon, L. M. (2010). Alteration in auditory function during the ovarian cycle. Hear. Res. 268, 114–122. doi: 10.1016/j.heares.2010.05.007
Anderl, C., Hahn, T., Notebaert, K., Klotz, C., Rutter, B., and Windmann, S. (2015). Cooperative preferences fluctuate across the menstrual cycle. Judgm. Decis. Mak. 10, 400–406. doi: 10.1017/S1930297500005544
Arnocky, S., Hodges-Simeon, C. R., Ouellette, D., and Albert, G. (2018). Do men with more masculine voices have better immunocompetence? Evol. Hum. Behav. 39, 602–610. doi: 10.1016/j.evolhumbehav.2018.06.003
Arruda, P., Da Diniz Rosa, M. R., Almeida, L. N. A., Pernambuco, A., De, L., and Almeida, A. A. (2019). Vocal acoustic and auditory-perceptual characteristics during fluctuations in estradiol levels during the menstrual cycle: a longitudinal study. J. Voice 33, 536–544. doi: 10.1016/j.jvoice.2018.01.024
Blake, K. R., Dixson, B. J. W., O'Dean, S. M., and Denson, T. F. (2016). Standardized protocols for characterizing women's fertility: a data-driven approach. Horm. Behav. 81, 74–83. doi: 10.1016/j.yhbeh.2016.03.004
Bobst, C., and Lobmaier, J. S. (2012). Men’s preference for the ovulating female is triggered by subtle face shape differences. Horm. Behav. 62, 413–417. doi: 10.1016/j.yhbeh.2012.07.008
Brown, B. L., Strong, W. J., and Rencher, A. C. (1973). Perceptions of personality from speech: effects of manipulations of acoustical parameters. J. Acoust. Soc. Am. 54, 29–35. doi: 10.1121/1.1913571
Bryant, G. A., and Haselton, M. G. (2009). Vocal cues of ovulation in human females. Biol. Lett. 5, 12–15. doi: 10.1098/rsbl.2008.0507
Cohen, I. T., Sherwin, B. B., and Fleming, A. S. (1987). Food cravings, mood, and the menstrual cycle. Horm. Behav. 21, 457–470. doi: 10.1016/0018-506X(87)90004-3
Collins, A., Eneroth, P., and Landgren, B. M. (1985). Psychoneuroendocrine stress responses and mood as related to the menstrual cycle. Psychosom. Med. 47, 512–527. doi: 10.1097/00006842-198511000-00002
Dabbs, J. J. M., and de La Rue, D. (1991). Salivary testosterone measurements among women: relative magnitude of circadian and menstrual cycles. Horm. Res. 35, 182–184. doi: 10.1159/000181899
DeBruine, L., Jones, B. C., Frederick, D. A., Haselton, M. G., Penton-Voak, I. S., and Perrett, D. I. (2010). Evidence for menstrual cycle shifts in women's preferences for masculinity: a response to Harris (in press) “menstrual cycle and facial preferences reconsidered”. Evol. Psychol. 8, 768–775. doi: 10.1177/147470491000800416
Derntl, B., Hack, R. L., Kryspin-Exner, I., and Habel, U. (2013). Association of menstrual cycle phase with the core components of empathy. Horm. Behav. 63, 97–104. doi: 10.1016/j.yhbeh.2012.10.009
Derntl, B., Kryspin-Exner, I., Fernbach, E., Moser, E., and Habel, U. (2008). Emotion recognition accuracy in healthy young females is associated with cycle phase. Horm. Behav. 53, 90–95. doi: 10.1016/j.yhbeh.2007.09.006
Emami, S. F., Gohari, N., Ramezani, H., and Borzouei, M. (2018). Hearing performance in the follicular-luteal phase of the menstrual cycle. Int. J. Otolaryngol. 2018, 7276359–7276356. doi: 10.1155/2018/7276359
Farage, M. A., Osborn, T. W., and MacLean, A. B. (2008). Cognitive, sensory, and emotional changes associated with the menstrual cycle: a review. Arch. Gynecol. Obstet. 278, 299–307. doi: 10.1007/s00404-008-0708-2
Farrar, D., Neill, J., Scally, A., Tuffnell, D., and Marshall, K. (2015). Is objective and accurate cognitive assessment across the menstrual cycle possible? A feasibility study. SAGE Open Med. 3:205031211456519. doi: 10.1177/2050312114565198
Faul, F., Erdfelder, E., Lang, A.-G., and Buchner, A. (2007). G*power 3: a flexible statistical power analysis program for the social, behavioral, and biomedical sciences. Behav. Res. Methods 39, 175–191. doi: 10.3758/bf03193146
Feinberg, D. R., Jones, B. C., Law Smith, M. L., Moore, F. R., DeBruine, L. M., Cornwell, R. E., et al. (2006). Menstrual cycle, trait estrogen level, and masculinity preferences in the human voice. Horm. Behav. 49, 215–222. doi: 10.1016/j.yhbeh.2005.07.004
Feinberg, D. R., Jones, B. C., Little, A. C., Burt, D. M., and Perrett, D. I. (2005). Manipulations of fundamental and formant frequencies influence the attractiveness of human male voices. Anim. Behav. 69, 561–568. doi: 10.1016/j.anbehav.2004.06.012
Fink, B., and Penton-Voak, I. S. (2002). Evolutionary psychology of facial attractiveness. Curr. Dir. Psychol. Sci. 11, 154–158. doi: 10.1111/1467-8721.00190
First, M. B., Williams, J. B., Karg, R. S., and Spitzer, R. L. (2016). Structured clinical interview for DSM-5 disorders, Clinician Version (SCID-5-CV). 0141–0768.
Fischer, J., Semple, S., Fickenscher, G., Jürgens, R., Kruse, E., Heistermann, M., et al. (2011). Do women’s voices provide cues of the likelihood of ovulation? The importance of sampling regime. PLoS One 6:e24490. doi: 10.1371/journal.pone.0024490
Foo, Y. Z., Simmons, L. W., and Rhodes, G. (2017). Predictors of facial attractiveness and health in humans. Sci. Rep. 7:39731. doi: 10.1038/srep39731
Frisina, R. D., Bazard, P., Bauer, M., Pineros, J., Zhu, X., and Ding, B. (2021). Translational implications of the interactions between hormones and age-related hearing loss. Hear. Res. 402:108093. doi: 10.1016/j.heares.2020.108093
Gallup, G. G., and Frederick, D. A. (2010). The science of sex appeal: an evolutionary perspective. Rev. Gen. Psychol. 14, 240–250. doi: 10.1037/a0020451
Gangestad, S. W. (2016). Comment: Wood et al.’s (2014) speculations of inappropriate research practices in ovulatory cycle studies. Emot. Rev. 8, 87–90. doi: 10.1177/1754073915580400
Gangestad, S. W., Haselton, M. G., Welling, L. L., Gildersleeve, K., Pillsworth, E. G., Burriss, R. P., et al. (2016). How valid are assessments of conception probability in ovulatory cycle research? Evaluations, recommendations, and theoretical implications. Evol. Hum. Behav. 37, 85–96. doi: 10.1016/j.evolhumbehav.2015.09.001
Gann, P. H., Giovanazzi, S., van Horn, L., Branning, A., and Chatterton, R. T. (2001). Saliva as a medium for investigating intra- and Interindividual differences in sex hormone levels in premenopausal women. Cancer Epidemiol. Biomarkers Prev. 10, 59–64
Garza, R., and Byrd-Craven, J. (2019). Fertility status in visual processing of men’s attractiveness. Evol. Psychol. Sci. 5, 328–342. doi: 10.1007/s40806-019-00190-4
Garza, R., and Byrd-Craven, J. (2023). The role of hormones in attraction and visual attention to facial masculinity. Front. Psychol. 14:1067487. doi: 10.3389/fpsyg.2023.1067487
Gigerenzer, G., and Gaissmaier, W. (2011). Heuristic decision making. Annu. Rev. Psychol. 62, 451–482. doi: 10.1146/annurev-psych-120709-145346
Gildersleeve, K., DeBruine, L., Haselton, M. G., Frederick, D. A., Penton-Voak, I. S., Jones, B. C., et al. (2013). Shifts in Women’s mate preferences across the ovulatory cycle: a critique of Harris (2011) and Harris (2012). Sex Roles 69, 516–524. doi: 10.1007/s11199-013-0273-4
Gildersleeve, K., Haselton, M. G., and Fales, M. R. (2014). Do women's mate preferences change across the ovulatory cycle? A meta-analytic review. Psychol. Bull. 140, 1205–1259. doi: 10.1037/a0035438
Goldstein, E. B. (2014). Sensation and perception. Internat. ed., 9th Edn. Belmont: Calif. Wadsworth Cengage Learning.
Gonzales, J. E., and Ferrer, E. (2016). Efficacy of methods for ovulation estimation and their effect on the statistical detection of ovulation-linked behavioral fluctuations. Behav. Res. Methods 48, 1125–1144. doi: 10.3758/s13428-015-0638-4
Green, D. M., and Swets, J. A. (1974). Signal detection theory and psychophysics. rev. Edn. Huntington, NY: RF Krieger.
Greenhouse, S. W., and Geisser, S. (1959). On methods in the analysis of profile data. Psychometrika 24, 95–112. doi: 10.1007/BF02289823
Guldner, S., Nees, F., and McGettigan, C. (2020). Vocomotor and social brain networks work together to express social traits in voices. Cereb. Cortex 30, 6004–6020. doi: 10.1093/cercor/bhaa175
Haraguchi, R., Hoshi, H., Ichikawa, S., Hanyu, M., Nakamura, K., Fukasawa, K., et al. (2021). The menstrual cycle alters resting-state cortical activity: a magnetoencephalography study. Front. Hum. Neurosci. 15:652789. doi: 10.3389/fnhum.2021.652789
Harris, C. R. (2011). Menstrual cycle and facial preferences reconsidered. Sex Roles 64, 669–681. doi: 10.1007/s11199-010-9772-8
Harris, C. R. (2013). Shifts in masculinity preferences across the menstrual cycle: still not there. Sex Roles 69, 507–515. doi: 10.1007/s11199-012-0229-0
Harris, C. R., Chabot, A., and Mickes, L. (2013). Shifts in methodology and theory in menstrual cycle research on attraction. Sex Roles 69, 525–535. doi: 10.1007/s11199-013-0302-3
Harwell, M. R., Rubinstein, E. N., Hayes, W. S., and Olds, C. C. (1992). Summarizing Monte Carlo results in methodological research: the one- and two-factor fixed effects ANOVA cases. J. Educ. Stat. 17, 315–339. doi: 10.3102/10769986017004315
Haselton, M. G., and Buss, D. M. (2000). Error management theory: a new perspective on biases in cross-sex mind reading. J. Pers. Soc. Psychol. 78, 81–91. doi: 10.1037/0022-3514.78.1.81
Hellbernd, N., and Sammler, D. (2016). Prosody conveys speaker’s intentions: acoustic cues for speech act perception. J. Mem. Lang. 88, 70–86. doi: 10.1016/j.jml.2016.01.001
Hidalgo-Lopez, E., Mueller, K., Harris, T., Aichhorn, M., Sacher, J., and Pletzer, B. (2020). Human menstrual cycle variation in subcortical functional brain connectivity: a multimodal analysis approach. Brain Struct. Funct. 225, 591–605. doi: 10.1007/s00429-019-02019-z
Hillairet de Boisferon, A., Dupierrix, E., Uttley, L., DeBruine, L. M., Jones, B. C., and Pascalis, O. (2019). Sex categorization of faces: the effects of age and experience. Iperception 10:2041669519830414. doi: 10.1177/2041669519830414
Hillenbrand, J. M., and Clark, M. J. (2009). The role of f 0 and formant frequencies in distinguishing the voices of men and women. Atten. Percept. Psychophys. 71, 1150–1166. doi: 10.3758/APP.71.5.1150
Holzleitner, I. J., Driebe, J. C., Arslan, R. C., Hahn, A. C., Lee, A. J., O’Shea, K. J., et al. (2022). No increased inbreeding avoidance during the ovulatory phase of the menstrual cycle. Evol. Hum. Sci. 4, 1–48. doi: 10.1017/ehs.2022.41
Hromatko, I., Tadinac, M., and Vranic, A. (2008). Femininity and masculinity across the menstrual cycle: a relation to mate value. Coll. Antropol. 32, 81–86
Hughes, S. M., and Puts, D. A. (2021). Vocal modulation in human mating and competition. Philos. Trans. R. Soc. Lond. Ser. B Biol. Sci. 376:20200388. doi: 10.1098/rstb.2020.0388
Jarosz, A. F., and Wiley, J. (2014). What are the odds? A practical guide to computing and reporting Bayes factors. J. Probl. Solving 7:2. doi: 10.7771/1932-6246.1167
Johnston, V. S., Hagel, R., Franklin, M., Fink, B., and Grammer, K. (2001). Male facial attractiveness: evidence for hormone-mediated adaptive design. Evol. Hum. Behav. 22, 251–267. doi: 10.1016/S1090-5138(01)00066-6
Johnston, L., Miles, L., and Macrae, C. N. (2008). Was that a man? Sex identification as a function of menstrual cycle and masculinity. Appl. Cognit. Psychol. 22, 1185–1194. doi: 10.1002/acp.1436
Jones, B. C., DeBruine, L. M., Perrett, D. I., Little, A. C., Feinberg, D. R., and Law Smith, M. J. (2008). Effects of menstrual cycle phase on face preferences. Arch. Sex. Behav. 37, 78–84. doi: 10.1007/s10508-007-9268-y
Jones, B. C., Hahn, A. C., and DeBruine, L. M. (2019). Ovulation, sex hormones, and Women’s mating psychology. Trends Cogn. Sci. 23, 51–62. doi: 10.1016/j.tics.2018.10.008
Jones, B. C., Hahn, A. C., Fisher, C. I., Wang, H., Kandrik, M., Han, C., et al. (2018). No compelling evidence that preferences for facial masculinity track changes in Women’s hormonal status. Psychol. Sci. 29, 996–1005. doi: 10.1177/0956797618760197
Jonge, X. J. D. E., Thompson, B., and Han, A. (2019). Methodological recommendations for menstrual cycle research in sports and exercise. Med. Sci. Sports Exerc. 51, 2610–2617. doi: 10.1249/MSS.0000000000002073
Junger, J., Habel, U., Brohr, S., Neulen, J., Neuschaefer-Rube, C., Birkholz, P., et al. (2014). More than just two sexes: the neural correlates of voice gender perception in gender dysphoria. PLoS One 9:e111672. doi: 10.1371/journal.pone.0111672
Jünger, J., Motta-Mena, N. V., Cardenas, R., Bailey, D., Rosenfield, K. A., Schild, C., et al. (2018). Do women’s preferences for masculine voices shift across the ovulatory cycle? Horm. Behav. 106, 122–134. doi: 10.1016/j.yhbeh.2018.10.008
Junger, J., Pauly, K., Brohr, S., Birkholz, P., Neuschaefer-Rube, C., Kohler, C., et al. (2013). Sex matters: neural correlates of voice gender perception. NeuroImage 79, 275–287. doi: 10.1016/j.neuroimage.2013.04.105
Karthikeyan, S., and Locke, J. L. (2015). Men’s evaluation of women’s speech in a simulated dating context: effects of female fertility on vocal pitch and attractiveness. Evol. Behav. Sci. 9, 55–67. doi: 10.1037/ebs0000014
Krasowski, M. D., Drees, D., Morris, C. S., Maakestad, J., Blau, J. L., and Ekins, S. (2014). Cross-reactivity of steroid hormone immunoassays: clinical significance and two-dimensional molecular similarity prediction. BMC Clin. Pathol. 14:33. doi: 10.1186/1472-6890-14-33
Lã, F. M. B., and Polo, N. (2020). Fundamental frequency variations across the menstrual cycle and the use of an Oral contraceptive pill. J. Speech Lang. Hear. Res. 63, 1033–1043. doi: 10.1044/2020_JSLHR-19-00277
Lattner, S., Meyer, M. E., and Friederici, A. D. (2005). Voice perception: sex, pitch, and the right hemisphere. Hum. Brain Mapp. 24, 11–20. doi: 10.1002/hbm.20065
Lewis, M. B. (2017). Fertility affects asymmetry detection not symmetry preference in assessments of 3D facial attractiveness. Cognition 166, 130–138. doi: 10.1016/j.cognition.2017.05.034
Lewis, M. B. (2020). Challenges to both reliability and validity of masculinity-preference measures in menstrual-cycle-effects research. Cognition 197:104201. doi: 10.1016/j.cognition.2020.104201
Little, A. C., and Jones, B. C. (2006). Attraction independent of detection suggests special mechanisms for symmetry preferences in human face perception. Proc. Biol. Sci. 273, 3093–3099. doi: 10.1098/rspb.2006.3679
Little, A. C., Jones, B. C., and DeBruine, L. M. (2008). Preferences for variation in masculinity in real male faces change across the menstrual cycle: women prefer more masculine faces when they are more fertile. Personal. Individ. Differ. 45, 478–482. doi: 10.1016/j.paid.2008.05.024
Little, A. C., Jones, B. C., Penton-Voak, I. S., Burt, D. M., and Perrett, D. I. (2002). Partnership status and the temporal context of relationships influence human female preferences for sexual dimorphism in male face shape. Proc. Biol. Sci. 269, 1095–1100. doi: 10.1098/rspb.2002.1984
Luther, T., Lewis, C. A., Grahlow, M., Hüpen, P., Habel, U., Foster, C., et al. (2021). Male or female? - influence of gender role and sexual attraction on sex categorization of faces. Front. Psychol. 12:718004. doi: 10.3389/fpsyg.2021.718004
Lynn, S. K., and Barrett, L. F. (2014). “Utilizing” signal detection theory. Psychol. Sci. 25, 1663–1673. doi: 10.1177/0956797614541991
Macrae, C. N., Hood, B. M., Milne, A. B., Rowe, A. C., and Mason, M. F. (2002). Are you looking at me? Eye gaze and person perception. Psychol. Sci. 13, 460–464. doi: 10.1111/1467-9280.00481
Maki, P. M., Rich, J. B., and Rosenbaum, R. S. (2002). Implicit memory varies across the menstrual cycle: estrogen effects in young women. Neuropsychologia 40, 518–529. doi: 10.1016/s0028-3932(01)00126-9
Maner, J. K., and Miller, S. L. (2014). Hormones and social monitoring: menstrual cycle shifts in progesterone underlie women’s sensitivity to social information. Evol. Hum. Behav. 35, 9–16. doi: 10.1016/j.evolhumbehav.2013.09.001
Marcinkowska, U. M., Ellison, P. T., Galbarczyk, A., Milkowska, K., Pawlowski, B., Thune, I., et al. (2016). Lack of support for relation between woman’s masculinity preference, estradiol level and mating context. Horm. Behav. 78, 1–7. doi: 10.1016/j.yhbeh.2015.10.012
McGettigan, C. (2015). The social life of voices: studying the neural bases for the expression and perception of the self and others during spoken communication. Front. Hum. Neurosci. 9:129. doi: 10.3389/fnhum.2015.00129
Nielsen, S. E., Ahmed, I., and Cahill, L. (2013). Sex and menstrual cycle phase at encoding influence emotional memory for gist and detail. Neurobiol. Learn. Mem. 106, 56–65. doi: 10.1016/j.nlm.2013.07.015
Niu, C., and Zheng, L. (2020). Androstadienone resulted in a rightward shift of women’s preference for sexually dimorphic male faces across the continuum of femininity-masculinity. Horm. Behav. 118:104635. doi: 10.1016/j.yhbeh.2019.104635
Oinonen, K. A., and Mazmanian, D. (2007). Facial symmetry detection ability changes across the menstrual cycle. Biol. Psychol. 75, 136–145. doi: 10.1016/j.biopsycho.2007.01.003
Pallier, C. (2002). Computing discriminability and bias with the R software. Available online at: https://www.pallier.org/pdfs/aprime.pdf, 1–6.
Parlee, M. B. (1983). Menstrual rhythm in sensory processes: a review of fluctuations in vision, olfaction, audition, taste, and touch. Psychol. Bull. 93, 539–548. doi: 10.1037/0033-2909.93.3.539
Penton-Voak, I. S., and Chen, J. Y. (2004). High salivary testosterone is linked to masculine male facial appearance in humans. Evol. Hum. Behav. 25, 229–241. doi: 10.1016/j.evolhumbehav.2004.04.003
Penton-Voak, I. S., and Perrett, D. I. (2000). Female preference for male faces changes cyclically: further evidence. Evol. Hum. Behav. 21, 39–48. doi: 10.1016/S1090-5138(99)00033-1
Penton-Voak, I. S., Perrett, D. I., Castles, D. L., Kobayashi, T., Burt, D. M., Murray, L. K., et al. (1999). Menstrual cycle alters face preference. Nature 399, 741–742. doi: 10.1038/21557
Peters, M., Simmons, L. W., and Rhodes, G. (2009). Preferences across the menstrual cycle for masculinity and symmetry in photographs of male faces and bodies. PLoS One 4:e4138. doi: 10.1371/journal.pone.0004138
Pierson, E., Althoff, T., Thomas, D., Hillard, P., and Leskovec, J. (2021). Daily, weekly, seasonal and menstrual cycles in women’s mood, behaviour and vital signs. Nat. Hum. Behav. 5, 716–725. doi: 10.1038/s41562-020-01046-9
Pisanski, K., Oleszkiewicz, A., Plachetka, J., Gmiterek, M., and Reby, D. (2018). Voice pitch modulation in human mate choice. Proc. R. Soc. B Biol. Sci. 285:20181634. doi: 10.1098/rspb.2018.1634
Pletzer, B., Harris, T.-A., and Ortner, T. (2017). Sex and menstrual cycle influences on three aspects of attention. Physiol. Behav. 179, 384–390. doi: 10.1016/j.physbeh.2017.07.012
Pletzer, B., Harris, T.-A., Scheuringer, A., and Hidalgo-Lopez, E. (2019). The cycling brain: menstrual cycle related fluctuations in hippocampal and fronto-striatal activation and connectivity during cognitive tasks. Neuropsychopharmacology 44, 1867–1875. doi: 10.1038/s41386-019-0435-3
Puts, D. A. (2005). Mating context and menstrual phase affect women’s preferences for male voice pitch. Evol. Hum. Behav. 26, 388–397. doi: 10.1016/j.evolhumbehav.2005.03.001
Puts, D. A., Bailey, D. H., Cárdenas, R. A., Burriss, R. P., Welling, L. L. M., Wheatley, J. R., et al. (2013). Women’s attractiveness changes with estradiol and progesterone across the ovulatory cycle. Horm. Behav. 63, 13–19. doi: 10.1016/j.yhbeh.2012.11.007
Puts, D. A., Jones, B. C., and DeBruine, L. M. (2012). Sexual selection on human faces and voices. J. Sex Res. 49, 227–243. doi: 10.1080/00224499.2012.658924
Raftery, A. E. (1995). Bayesian model selection in social research. Sociol. Methodol. 25:111. doi: 10.2307/271063
Rahman, S. A., Grant, L. K., Gooley, J. J., Rajaratnam, S. M. W., Czeisler, C. A., and Lockley, S. W. (2019). Endogenous circadian regulation of female reproductive hormones. J. Clin. Endocrinol. Metabol. 104, 6049–6059. doi: 10.1210/jc.2019-00803
Raj, A., Gupta, B., Chowdhury, A., and Chadha, S. (2010). A study of voice changes in various phases of menstrual cycle and in postmenopausal women. J. Voice 24, 363–368. doi: 10.1016/j.jvoice.2008.10.005
Rosenberg, L., and Park, S. (2002). Verbal and spatial functions across the menstrual cycle in healthy young women. Psychoneuroendocrinology 27, 835–841. doi: 10.1016/S0306-4530(01)00083-X
Sacher, J., Okon-Singer, H., and Villringer, A. (2013). Evidence from neuroimaging for the role of the menstrual cycle in the interplay of emotion and cognition. Front. Hum. Neurosci. 7, 1–7. doi: 10.3389/fnhum.2013.00374
Sanders, G., and Wenmoth, D. (1998). Verbal and music dichotic listening tasks reveal variations in functional cerebral asymmetry across the menstrual cycle that are phase and task dependent. Neuropsychologia 36, 869–874. doi: 10.1016/S0028-3932(98)00022-0
Schmalenberger, K. M., Tauseef, H. A., Barone, J. C., Owens, S. A., Lieberman, L., Jarczok, M. N., et al. (2021). How to study the menstrual cycle: practical tools and recommendations. Psychoneuroendocrinology 123:104895. doi: 10.1016/j.psyneuen.2020.104895
Senior, C., Lau, A., and Butler, M. J. (2007). The effects of the menstrual cycle on social decision making. Int. J. Psychophysiol. 63, 186–191. doi: 10.1016/j.ijpsycho.2006.03.009
Smith, E., Junger, J., Pauly, K., Kellermann, T., Dernt, B., and Habel, U. (2019). Cerebral and behavioural response to human voices is mediated by sex and sexual orientation. Behav. Brain Res. 356, 89–97. doi: 10.1016/j.bbr.2018.07.029
Smith, E., Junger, J., Pauly, K., Kellermann, T., Neulen, J., Neuschaefer-Rube, C., et al. (2018). Gender incongruence and the brain–behavioral and neural correlates of voice gender perception in transgender people. Horm. Behav. 105, 11–21. doi: 10.1016/j.yhbeh.2018.07.001
Stanislaw, H., and Todorov, N. (1999). Calculation of signal detection theory measures. Behav. Res. Methods Instrum. Comput. 31, 137–149. doi: 10.3758/bf03207704
Stern, J., Hildebrand, T., and Casto, K. (2022). Women’s Intrasexual competitiveness and jealousy across the ovulatory cycle: a hormone-based study. Soc. Psychol. Personal. Sci. 14, 647–661. doi: 10.1177/19485506221117712
Stern, J., Kordsmeyer, T. L., and Penke, L. (2021). A longitudinal evaluation of ovulatory cycle shifts in women’s mate attraction and preferences. Horm. Behav. 128:104916. doi: 10.1016/j.yhbeh.2020.104916
Thimm, M., Weis, S., Hausmann, M., and Sturm, W. (2014). Menstrual cycle effects on selective attention and its underlying cortical networks. Neuroscience 258, 307–317. doi: 10.1016/j.neuroscience.2013.11.010
Vrieze, S. I. (2012). Model selection and psychological theory: a discussion of the differences between the Akaike information criterion (AIC) and the Bayesian information criterion (BIC). Psychol. Methods 17, 228–243. doi: 10.1037/a0027127
Wagenmakers, E.-J. (2007). A practical solution to the pervasive problems of p values. Psychon. Bull. Rev. 14, 779–804. doi: 10.3758/bf03194105
Wang, T. Y., Kawaguchi, I., Kuzuoka, H., and Otsuki, M. (2018). Effect of manipulated amplitude and frequency of human voice on dominance and persuasiveness in audio conferences. Proceedings of the ACM on human-computer interaction, 2, 1–18.
Welling, L. L., Jones, B. C., DeBruine, L. M., Conway, C. A., Law Smith, M. J., Little, A. C., et al. (2007). Raised salivary testosterone in women is associated with increased attraction to masculine faces. Horm. Behav. 52, 156–161. doi: 10.1016/j.yhbeh.2007.01.010
Weston, P. S., Hunter, M. D., Sokhi, D. S., Wilkinson, I. D., and Woodruff, P. W. (2015). Discrimination of voice gender in the human auditory cortex. NeuroImage 105, 208–214. doi: 10.1016/j.neuroimage.2014.10.056
Whitling, S., Botzum, H. M., and van Mersbergen, M. R. (2023). Degree of breathiness in a synthesized voice signal as it differentiates masculine versus feminine voices. J. Voice. [in press]. doi: 10.1016/j.jvoice.2023.04.022
Williamson, T. T., Zhu, X., Pineros, J., Ding, B., and Frisina, R. D. (2020). Understanding hormone and hormone therapies’ impact on the auditory system. J. Neurosci. Res. 98, 1721–1730. doi: 10.1002/jnr.24588
Wood, W. (2016). Reply to Gangestad’s (2016) comment on Wood, Kressel, Joshi, and Louie (2014). Emot. Rev. 8, 90–94. doi: 10.1177/1754073915580401
Keywords: menstrual cycle, voice-gender categorization, estradiol, progesterone, signal detection theory, mating cues, follicular phase, luteal phase
Citation: Friedrich S, Brodkin ES, Derntl B, Habel U and Hüpen P (2025) Assessing the association between menstrual cycle phase and voice-gender categorization: no robust evidence for an association. Front. Psychol. 16:1531021. doi: 10.3389/fpsyg.2025.1531021
Received: 19 November 2024; Accepted: 19 March 2025;
Published: 02 April 2025.
Edited by:
Lisa L. M. Welling, Oakland University, United StatesReviewed by:
Ray Garza, Texas A&M International University, United StatesCopyright © 2025 Friedrich, Brodkin, Derntl, Habel and Hüpen. This is an open-access article distributed under the terms of the Creative Commons Attribution License (CC BY). The use, distribution or reproduction in other forums is permitted, provided the original author(s) and the copyright owner(s) are credited and that the original publication in this journal is cited, in accordance with accepted academic practice. No use, distribution or reproduction is permitted which does not comply with these terms.
*Correspondence: Philippa Hüpen, cmh1ZXBlbkB1a2FhY2hlbi5kZQ==
Disclaimer: All claims expressed in this article are solely those of the authors and do not necessarily represent those of their affiliated organizations, or those of the publisher, the editors and the reviewers. Any product that may be evaluated in this article or claim that may be made by its manufacturer is not guaranteed or endorsed by the publisher.
Research integrity at Frontiers
Learn more about the work of our research integrity team to safeguard the quality of each article we publish.