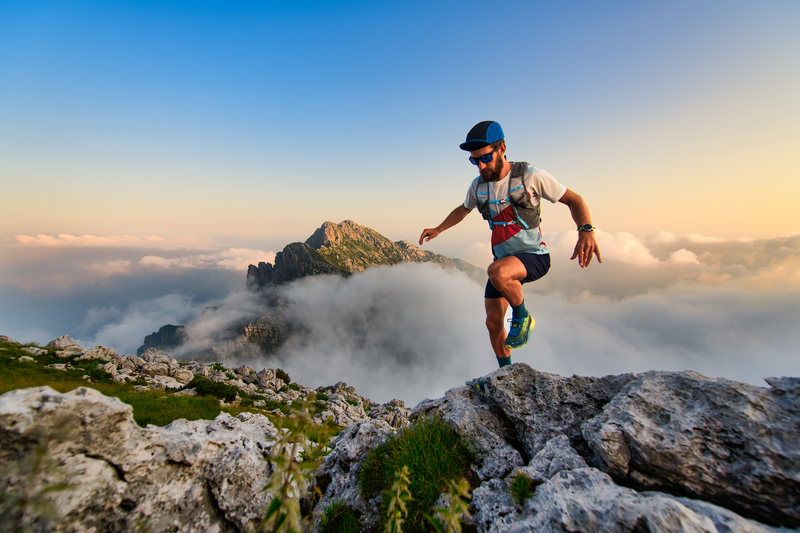
94% of researchers rate our articles as excellent or good
Learn more about the work of our research integrity team to safeguard the quality of each article we publish.
Find out more
SYSTEMATIC REVIEW article
Front. Psychol. , 21 February 2025
Sec. Educational Psychology
Volume 16 - 2025 | https://doi.org/10.3389/fpsyg.2025.1524645
Introduction: The relationship between students’ smartphone addiction, social media use, video games play, and their academic performance has been widely studied, yet the existing literature presents inconsistent findings. This meta-analysis synthesizes current research to provide a comprehensive examination of the impact of these technologies on academic achievement.
Methods: A total of 63 studies (yielding 64 effect sizes) were included, encompassing a sample of 124,166 students from 28 countries. The meta-analysis utilized correlation coefficients and sample sizes, reporting results based on the random effects model. Key statistics such as the Fisher’s Z value, confidence intervals, and heterogeneity (Q) test results were considered, and publication bias was assessed using Begg and Mazumdar’s rank correlation test, with the Kendall Tau coefficient determining bias significance.
Results and discussion: The meta-analysis revealed a small but statistically significant negative association between smartphone use, social media use, video game playing, and students’ academic performance [Q(64) = 2501.93, p < 0.001, d = −0.085]. It is concluded that increased use of these technologies was associated with poorer academic outcomes, potentially impacting key cognitive skills essential for academic success. The implications for educational psychology research and future research directions are discussed.
The rapid proliferation of digital technologies has significantly altered the ways students engage with academic work. While smartphones, social media, and video games offer potential educational benefits, concerns have emerged about their possible detrimental effects on students’ academic performance. Research on this topic has yielded mixed findings, with some studies indicating negative effects on learning outcomes, while others suggest potential advantages under certain conditions. Despite extensive research investigating the relationships between smartphone addiction (SA), social media use (SMU), video games (VGs), and academic performance (AP), findings remain inconsistent and, at times, controversial. Most studies suggest a negative relationship between these digital behaviors and academic outcomes (Kim et al., 2019; Yang et al., 2022). However, a smaller body of research has reported either no significant impact or a positive association between SA, SMU, VGs, and AP (Sert et al., 2019; Van Den Eijnden et al., 2018). These conflicting findings may stem from variations in the purposes for which smartphones, social media, and video games are used. Smartphones have become indispensable due to their multifunctionality and portability, contributing to increased dependency. Among younger generations, smartphones are commonly used for watching sports, gaming, online banking, communication, social media engagement, and even completing homework (Nayak, 2018).
Despite the volume of research, there is no clear consensus on the direction and magnitude of the effects of SA, SMU, and VGs on AP. While most studies point to a negative association, the effect sizes reported vary widely. This inconsistency underscores the need for a meta-analytic approach to estimate the true overall impact of SA, SMU, and VGs on academic outcomes. Given the widespread prevalence of these behaviors among students, it is critical to systematically examine their relationships with academic achievement. To date, no published meta-analysis has comprehensively addressed the link between SA, SMU, VGs, and student academic performance. The present study seeks to fill this gap in the literature, providing valuable insights for scholars, educators, and policymakers. By exploring these relationships, this research aims to inform strategies that can maximize the benefits of SA, SMU, and VGs while minimizing their potential adverse effects on academic achievement.
Previous studies on smartphone addiction (SA), social media use (SMU), and video game use (VGs) are informed by several theoretical frameworks. For example, Cognitive Load Theory (Sweller, 1994), posits that excessive engagement with these technologies can hinder learning by overloading working memory. Frequent notifications, multitasking, and prolonged screen time can deplete cognitive resources, leading to decreased attention and comprehension, ultimately impacting academic performance. Similarly, Self-Regulation Theory (Zimmerman, 2000), emphasizes the potential for digital distractions to impair students’ ability to manage their time and academic tasks effectively. Students with weak self-regulatory skills may be more susceptible to distractions from smartphones, social media, and video games, resulting in procrastination and reduced study time. Conversely, students who effectively regulate their digital habits may use these tools for productive academic engagement. In this regard, Social Learning Theory (Bandura, 1986), offers a contrasting perspective, suggesting that social media can facilitate knowledge sharing, and video games can enhance cognitive skills such as problem-solving and strategic thinking, potentially benefiting academic outcomes. This theory posits that digital platforms can be valuable learning and collaboration tools. Social media can foster peer learning, discussions, and access to diverse educational resources, while video games may improve cognitive functions like problem-solving, strategic thinking, and hand-eye coordination.
This study integrates these theoretical perspectives to provide a more nuanced understanding of the complex relationship between SA, SMU, VGU, and AP. Cognitive Load Theory explains the potential negative impact of excessive technology use on learning efficiency, while Self-Regulation Theory highlights the importance of students’ ability to manage their digital behavior. Social Learning Theory offers a counterpoint, suggesting that, under certain conditions, social media and video games can positively contribute to academic engagement. By synthesizing findings from previous research, this meta-analysis aims to clarify these complex interactions and provide insights into optimizing technology use for academic success. Yet, in the first place, this requires a clear conceptualization of SA, SMU, VGU, and AP.
The widespread availability of smartphones has facilitated instant access to information, entertainment, and remote communication, profoundly shaping modern social interactions and media consumption patterns (O’Dea, 2023; Scott et al., 2016). However, this ubiquitous access has also raised concerns about smartphone addiction (SA). SA is generally defined as the compulsive and uncontrollable overuse of smartphones, which can result in various negative outcomes, including withdrawal symptoms, diminished academic performance, strained social relationships, and physical health issues, all of which can impair an individual’s daily functioning (Kuss and Griffiths, 2017). In the literature, several terms - such as “problematic mobile or smartphone use” (Kim et al., 2015), “smartphone addiction” (Jeong, 2016; Kim et al., 2017), “smartphone dependence” (Lee et al., 2016; Yoo et al., 2020), and “smartphone overuse” (Kim et al., 2019; Hwang et al., 2012), are used interchangeably to describe this phenomenon, which is characterized by an individual’s inability to regulate their smartphone use, leading to adverse consequences. Among these consequences, impairments in attention and learning have been identified as significant contributors to decreased academic performance, particularly within educational settings (Dontre, 2021; Yang et al., 2018).
The emergence of social media has significantly reshaped how individuals communicate and share information, exerting a profound impact on modern society and education. The younger generation has played a central role in this digital transformation, seamlessly integrating various social media platforms into their daily routines (Lim et al., 2021; Mansour et al., 2020). As students engage in academic activities, they encounter the pervasive influence of social media, which presents both advantages and disadvantages (Alenezi and Brinthaupt, 2022; Lim et al., 2021; Shen, 2019). Social media use (SMU), also referred to as problematic social media use, social media disorder, or social media addiction (Sun and Zhang, 2020), has not yet been officially recognized as a behavioral addiction. However, it is generally understood as the inability to control one’s social media usage, leading to impairments in various aspects of daily functioning, particularly academic performance (Andreassen and Pallesen, 2014; Boer et al., 2021). SMU has become a widespread concern among adolescents and young adults (Swain and Pati, 2021; Tezci and İçen, 2017), prompting extensive research efforts aimed at understanding the scope of the problem (Cheng et al., 2021). The concept of SMU has been defined and assessed in various ways across the literature (Sun and Zhang, 2020).
In line with the rise of social media, advancements in digital technology have also transformed leisure activities, with video games (VGs) emerging as a prominent form of global entertainment. VGs are now played by a vast and diverse audience, including a majority of young people and adolescents (Gilbert, 2023). Recent statistics indicate that the global gaming population has reached approximately 2.7 billion active participants (Statista, 2024). This widespread engagement raises important questions about the potential effects of video game play on individuals’ lives and future prospects. Although some researchers use the terms “excessive gaming” and “problematic gaming” interchangeably, these terms refer to distinct phenomena with differing outcomes (Griffiths, 2010). Excessive gaming refers to spending extensive time playing VGs (Borgonovi, 2016; Osuagwu, 2015), which may not necessarily result in negative consequences (Griffiths, 2010). Conversely, problematic gaming, often referred to as gaming addiction or gaming disorder, is characterized by compulsive and persistent gaming behavior that leads to significant disruptions in personal, social, and academic functioning (World Health Organisation, 2020). Problematic gaming has been increasingly conceptualized within the framework of behavioral addiction (Dowling, 2014; Pontes et al., 2014).
While concerns about the potential harms of gaming persist, research has also highlighted numerous positive outcomes associated with VGs (Bavelier and Green, 2019; Cai et al., 2022). VGs have been effectively integrated into educational settings as tools to support learning, helping students reinforce academic content in subjects such as mathematics and science (Hussein et al., 2022). Additionally, gaming has been found to enhance various cognitive skills, including attention, critical thinking, problem-solving, and decision-making (Nuyens et al., 2019; Reynaldo et al., 2021). However, despite these potential benefits, excessive gaming remains a cause for concern, as it has been linked to harmful effects in certain contexts (Borgonovi, 2016).
Smartphone addiction (SA), characterized by excessive and compulsive smartphone use, has been associated with a range of negative outcomes, including disruptions to daily activities and adverse impacts on academic performance (Park and Lee, 2012). A substantial body of research has examined the relationship between problematic smartphone use and academic achievement, consistently reporting a negative association, albeit with varying degrees of severity (Alosaimi et al., 2016; Alotaibi et al., 2022; Arora et al., 2018; Asante and Hiadzi, 2018; Chen and Ji, 2015; Durak, 2018; Felisoni and Godoi, 2018; Han, 2022; Kim et al., 2019; Li et al., 2015; Longnecker, 2017; Mendoza et al., 2018; Olufadi, 2015; Rozgonjuk et al., 2018; Samaha and Hawi, 2016; Spiratos, 2021; Uzun and Kilis, 2019; Zhou et al., 2022). It is evident that excessive smartphone use in educational settings can impair students’ academic performance, resulting in lower grade point averages (GPA) (Lepp et al., 2014).
On the other hand, in the literature several studies purported either positive or no significant relationship between SA and AP (Bai et al., 2020; Bergdahl et al., 2020; Bun Lee, 2015; Domoff et al., 2020; Dos, 2014; Eoh et al., 2022; Fernández-Andújar et al., 2022; Lau, 2017; Lepp et al., 2015; Lin and Chiang, 2017; Przepiorka et al., 2021; Rashid and Asghar, 2016; Rosen et al., 2018; Sert et al., 2019; Winskel et al., 2019). The potential positive impact of SA on academic performance may be explained by shifting social norms, where high levels of smartphone use have become more accepted. Furthermore, smartphones, when used as educational tools, can enhance the learning process. For example, when students are permitted to use smartphones for academic purposes in class, such usage may facilitate improved learning outcomes and contribute positively to academic performance (Tessier, 2013). These divergent findings highlight the complex and multifaceted nature of SA and its impact on academic achievement, making it a subject of ongoing debate in the scholarly community.
Hypothesis 1: There is an association between smartphone addiction (SA) and academic performance (AP).
SMU presents both advantages and disadvantages for students’ academic pursuits (Alenezi and Brinthaupt, 2022; Lim et al., 2021; Shen, 2019). Social media platforms enable users to share personal experiences, connect with peers, and access information in ways that were once unimaginable. While these platforms offer valuable tools for enhancing learning, they can also serve as significant distractions, potentially undermining academic performance (Alenezi and Brinthaupt, 2022). The educational benefits of social media engagement are evident, but concerns have been raised regarding its potential detrimental effects on academic performance (Lim et al., 2021; Shen, 2019). Social media’s ability to rapidly disseminate information grants students access to a wealth of resources, thereby enriching their educational experiences (Lim et al., 2021; Mansour et al., 2020). However, the multitasking nature typical of SMU often leads to cognitive overload and fragmented attention (Karpinski et al., 2013), which may, in turn, hinder academic achievement. The constant influx of notifications, updates, and content poses a challenge for students striving to maintain focus on their studies (Karpinski et al., 2013). Given these dynamics, the relationship between SMU and academic performance has become a critical area of academic inquiry. Numerous studies have explored this connection, frequently identifying a negative association between SMU and academic outcomes (Alotaibi et al., 2022; Bhandarkar et al., 2021; Gazibara et al., 2020; Homaid, 2022; Molla-Esparza et al., 2020; Pedersen et al., 2018; Qahri-Saremi and Turel, 2016; Sampasa-Kanyinga et al., 2019; Shafiq and Parveen, 2023; Shen, 2019; Tsai and Liu, 2015; Tsitsika et al., 2014; Wakefield and Frawley, 2020; Van Den Eijnden et al., 2018). Nevertheless, some research has reported either a positive or no significant relationship between SMU and academic performance (Bardakcı, 2019; Çimen and Yılmaz, 2017; Dubuc et al., 2020; Lim et al., 2021; Kalam et al., 2023; Mansour et al., 2020; Masalimova et al., 2023; Tang and Patrick, 2018; Tomé-Fernández et al., 2020). These inconsistencies in the literature suggest a complex and multifaceted relationship between SMU and AP.
Hypothesis 2: There is an association between social media use (SMU) and academic performance (AP).
A growing body of research has highlighted the diverse benefits of video games (VGs) in various contexts, including education (Bavelier and Green, 2019; Cai et al., 2022). VGs have been effectively integrated into educational settings to provide supplemental learning experiences that support and enhance traditional educational methods (Hussein et al., 2019; Hussein et al., 2022). Furthermore, video games have been shown to improve cognitive abilities such as attention, critical thinking, problem-solving, and decision-making (Nuyens et al., 2019; Reynaldo et al., 2021). Gameplay often requires players to maintain focus, assess situations, and develop strategies to overcome challenges and progress through levels (Borgonovi, 2016).
While these benefits are evident, excessive video game use can have detrimental effects. Excessive gaming may hinder academic success, whereas moderate gaming has been linked to potential improvements in students’ academic performance (Bavelier and Green, 2019). On the contrary, excessive gaming is associated with lower academic grades (Ferguson, 2015), and a range of other negative outcomes, including reduced cognitive abilities (Nuyens et al., 2017), vision problems (Mylona et al., 2020), musculoskeletal pain (Kumar et al., 2023), sleep disturbances (Alimoradi et al., 2019; Lam, 2014), and poor nutrition (Ayenigbara, 2018). One of the primary concerns related to problematic gaming is its negative effect on students’ academic performance (Kumar et al., 2021). Numerous studies have investigated the relationship between problematic gaming and academic performance, consistently finding a negative correlation between the two (Brunborg et al., 2014; Ekşi et al., 2020; Ferguson, 2015; Hawi et al., 2018; Jaafar et al., 2021; Karnadi and Pangestu, 2021; Khan et al., 2014; Polat and Topal, 2022; Rehbein et al., 2015; Sahin et al., 2016; Samaha and Hawi, 2020; Schmitt and Livingston, 2015; Suryawanshi et al., 2021; Toker and Baturay, 2016; Yan and Yang, 2017; Zahra et al., 2020; Zhang et al., 2019; Zorbaz et al., 2015).However, some research has reported either a positive or no significant relationship between problematic gaming and academic outcomes (Al Asqah et al., 2020; Ciris et al., 2022; ELNahas et al., 2018; Van Den Eijnden et al., 2018; Nuyens et al., 2019; Reynaldo et al., 2021).These mixed findings underscore the complex nature of video gaming and its varied impacts on academic achievement.
Hypothesis 3: There is an association between video game use (VG) and academic performance (AP).
The dependent variable in this study is academic performance (AP), which is defined as the overall achievement demonstrated by students throughout their educational endeavors, spanning from primary to tertiary levels (Kumar et al., 2021). AP is a key indicator of students’ knowledge acquisition, comprehension, and the ability to apply learned material (Kumar et al., 2021). Previous research has employed a variety of methods to assess AP, including standardized tests, teacher evaluations, and classroom observations (Borgonovi, 2016; Kuh et al., 2014). Additionally, some studies have utilized self-evaluation rating scales, where students assess their own academic performance (Benjet et al., 2023), while others have relied on more objective measures such as academic grades or grade point averages (GPAs) as indicators of cumulative achievement (York et al., 2015). Generally, higher academic grades or GPAs reflect stronger academic performance.
Several studies have examined the combined effects of SA, SMU, and VGs on AP, frequently identifying a negative correlation (Alotaibi et al., 2022; Bhandarkar et al., 2021; Gazibara et al., 2020; Kim et al., 2019; Samaha and Hawi, 2020; Suryawanshi et al., 2021). However, other research has reported either positive or non-significant correlations between these factors and AP (Al Asqah et al., 2020; Bai et al., 2020; Fernández-Andújar et al., 2022; Kalam et al., 2023; Masalimova et al., 2023; Reynaldo et al., 2021). These contracting findings underscore the need for further investigation into the combined influence of SA, SMU, and VGs use on academic outcomes.
Hypothesis 4: There is a combined association among smartphone addiction (SA), social media use (SMU), video game (VGs) use, and academic performance (AP).
In light of the aforementioned considerations, and the profound influence of the digital age, it is essential to carefully examine the relationships between SA, SMU, VGs, and AP. Despite the growing body of literature, no consensus exists on the extent to which SA, SMU, and VGs impact academic performance. The present study seeks to address this gap through a systematic review and meta-analysis, synthesizing findings from 63 studies across multiple educational contexts. Specifically, this study aims to investigate potential relationships between these variables. Thus, the following research questions were formulated:
RQ1: How do past research demonstrate the relationships between SA, SMU, VGs, and AP?
RQ2: What factors moderate the relationship between SA, SMU, VGs, and AP as demonstrated by past research?
By addressing these research questions, this study contributes to a more nuanced understanding of how digital behaviors shape academic outcomes, offering practical insights for optimizing technology use in educational settings.
The present study employed a meta-analytical approach to analyze the overall impact of technology related factors including smartphone addiction, social media use, and video games play on students’ academic performance. This meta-analysis utilized a robust review protocol which is consistent with the PRISMA protocol (Kitchenham and Charters, 2007; Moher et al., 2015; Page et al., 2021).
Informed by a thorough review of the relevant literature, specific eligibility criteria were established to examine the association between SA, SMU, VGs, and AP. For the purpose of minimizing publication bias, the aim was to retrieve data from both published and unpublished studies.
Inclusion Criteria: Studies were considered eligible if they met the following conditions:
(i) The research focused on the impact of SA, SMU, and VGs on AP,
(ii) Academic performance was reported in terms of GPA, standardized test scores, or self-reported measures,
(iii) Sufficient and appropriate data were provided to facilitate the calculation of effect sizes,
(iv) The studies were publicly accessible either online or through library archives,
(v) The studies were published in English.
Exclusion criteria. To enhance the replicability of this meta-analysis, studies were excluded if they met any of the following conditions:
(i) The study was outside the scope of the research focus or assessed a multidimensional or complex phenomenon,
(ii) The results pertained to affective variables such as attitude or motivation,
(iii) The study did not report sufficient data to calculate effect sizes,
(iv) The sample size was fewer than 30 participants (Lin, 2018).
The following two databases were searched for potentially eligible studies: Web of Science, and Google Scholar. To conduct a comprehensive and systematic search, the following keywords were used: “smartphone addiction” OR “problematic smartphone use” OR “excessive smartphone use,” “social media addiction” OR “problematic social media use” OR “excessive social media use,” “videogame addiction” OR “problematic videogame use” OR “excessive videogame use,” and “academic performance” OR “academic achievement” OR “GPA.” A thorough literature search was carried out by two independent researchers in September 2023, across these major databases. Given the fast-evolving nature of social media research, only literature from the past decade (2014–2023) was included. The researcher independently selected studies through a sequential review of (a) their titles/abstracts and (b) their full texts (Freelon, 2013). In cases where duplicate data were identified, only data from peer-reviewed publications were used.
A total of 1,481 articles were initially gathered through the search process and entered into a comprehensive coding form. This meta-analysis employed a two-phase screening approach to apply the inclusion and exclusion criteria. In the first phase, both the first and second authors independently reviewed the titles and keywords of the articles to assess their relevance and ensure the inclusion criteria were strictly followed. During this phase, 840 duplicate articles were removed. Additionally, studies that did not meet the selection criteria were excluded, (n:534) leaving 107 articles after the first phase. In the second phase, the full texts of the remaining articles were obtained and carefully reviewed by two researchers. A coding sheet was developed for this stage, and both researchers independently evaluated the full texts to determine their suitability based on the inclusion criteria. As a result, only 63 articles met the criteria and were included in the final analysis (Figure 1). The coding variables included 13 categorical moderators: (a) authors’ names and publication year, (b) study title, (c) country, (d) sampling group, (e) gender, (f) age group, (g) educational level, (h) empirical approach, (i) research design, (j) academic performance indicators, (k) smartphone addiction constructs, (l) social media use constructs, and (m) video game constructs. These moderator variables were determined a priori and incorporated study characteristics into the coding forms and this process was guided by hypothesis and research questions of the study.
Figure 1. PRISMA 2020 flow diagram for meta-analysis (Page et al., 2021).
After coding all the data and ensuring they met the inclusion criteria for analysis, the prepared dataset was transferred to the JAMOVI 2.5 software package for final verification. Appropriate analysis methods were then selected. Using the MAJOR add-on within JAMOVI, the correlation coefficient was calculated based on the author names, sample sizes (n values), and r values from the studies. A random-effects model was applied to interpret the findings (Jeong, 2016; Kim et al., 2017). This model assumes that errors arise not only from sampling procedures but also from additional between-study variance (Jeong, 2016; Yoo et al., 2020). In analyses using this method, effect sizes are adjusted by the inverse of the variance’s weight, accounting for both sampling error and between-study error (Yoo et al., 2020). Effect sizes in the study were calculated following Cohen’s (1992), guidelines (Lee et al., 2016). The I-square (I2) statistic was used to estimate the degree of confidence interval overlap and is interpreted as indicating low (25%), moderate (50%), or high (75%) levels of total variance attributable to covariates (Kim et al., 2019). A high I2 value suggests significant heterogeneity, supporting the use of a random-effects model for the meta-analysis (Field and Gillett, 2010; Hwang et al., 2012).
Meta-analyses are often susceptible to publication bias, where studies with significant results are more likely to be published, potentially skewing the overall effect size when synthesizing results from multiple studies (Dontre, 2021; Kim et al., 2017; Yang et al., 2018). To address this issue, we assessed the symmetry of the effect distribution by visually inspecting funnel plots and performing Begg and Mazumdar's (1994) regression tests (Alenezi and Brinthaupt, 2022; Lim et al., 2021; Mansour et al., 2020). Additionally, we used trim-and-fill analyses (Shen, 2019), to estimate the number of potentially missing studies and evaluate their impact on the overall meta-analytic effect. Each study provided details on the number of participants, effect size (Pearson’s r), confidence intervals (lower and upper bounds), relative weight, residual values, and the summary effect size if excluded from the analysis. Descriptive statistics were visualized using Microsoft Excel. For moderator analyses, appropriate criteria were applied, and the relevant analyses were incorporated accordingly.
To rigorously assess the methodological quality of the studies included in this meta-analysis, Guidelines of Kitchenham and Charters (2007) were used. While most quality checklists in existing academic literature adhere to a combination of established guidelines, this study proposed a set of questions derived from widely used checklists and guidelines. These questions were designed to evaluate the design, conduct, analysis, and conclusions of each study included in the meta-analysis. The Evaluation Criteria (EC) presented below:
EC1: The aim of the study was clearly defined.
EC2: The context in which the study was conducted adequately described.
EC3: The research design was appropriate for addressing the study aims.
EC4: The characteristics of participants were clearly defined.
EC5: The data collection methods of the study were thoroughly described.
EC6: The study has received at least 10 citations.
EC7: Study provided a detailed description and justification of the data analysis procedures.
EC8: Results of the study were clearly presented.
EC9: Discussion and conclusion clearly compare the findings of the study with existing literature.
EC10: The study contributes to existing literature.
The scoring procedure assigned a value of 1 for “Yes” and 0 for “No,” allowing studies to score between 0 and 10 points. Papers with a score greater than 8 (>8) were selected for inclusion in this meta-analysis. The results of the quality assessment are presented in Table 1.
A total of 1,481 initial outputs were identified, and after the study selection process, 63 studies with 64 effect sizes (N = 124,166) published between 2014 and 2023 were included in the meta-analysis. The main characteristics of these studies are summarized in Table 1. All 63 studies were published in peer-reviewed journals. Academic achievement was measured using grade point average (GPA), self-reported grades, or standardized test scores. Among the retrieved studies, Turkey and the USA emerged as the leading contributors, with 10 studies conducted in Turkey and 9 in the USA. The meta-analysis included samples of students across primary, secondary, and tertiary education, with participants’ ages ranging from 7 to 27 years.
The characteristics and results of the studies are presented in Table 2. According to the analysis results, the highest positive correlation in “Smartphone Use and Academic Performance” (r = 0.17) was reported by Domoff et al. (2020), while the highest negative correlation (r = −0.38) was reported by Kim et al. (2019). The median of the observed correlations was (r = −0.143). Regarding the variable “Social Media Use and Academic Performance,” the lowest negative relationship (r = −0.407) was reported by Tsai and Liu (2015), whereas the highest positive relationship (r = 0.8) was reported by Bardakcı (2019). The median of the studies was (r = −0.0485). For the variable “Videogame Use and Academic Performance,” the lowest negative relationship (r = −0.271) was reported by Zorbaz et al. (2015), while the only positive relationship (r = 0.108) was reported by Ciris et al. (2022). The median of the observed studies was (r = −0.13).
The meta-analysis results purported that SA and VGs have a small negative impact on AP, whereas SMU’s impact is ambivalent. Table 3 illustrates the result of the meta-analysis.
According to the results of the meta-analysis, the effect sizes on academic performance are as follows: for SA, d = −0.129, 95% CI [−0.183, −0.075], indicating a small negative effect; for SMU, d = 0.025, 95% CI [−0.051, 0.102], showing a small positive effect, although this effect is not statistically significant. For VGs, d = −0.134, 95% CI [−0.187, −0.082], there is a small negative effect. The overall effect of all factors on AP is d = −0.085, 95% CI [−0.143, −0.028], indicating a small negative effect (Figures 2, 3).
According to the Begg and Mazumdar test, p > 0.394, suggesting no evidence of publication bias. The forest plot and funnel plot for the results are presented in the following figures, respectively.
Figure 4 presents a forest plot showing the combined effect sizes obtained from the smartphone variable.
Figure 5 presents a funnel plot assessing the distribution of data obtained from the smartphone.
Figure 6 presents a forest plot showing the combined effect sizes obtained from the social media variable.
Figure 7 presents a funnel plot assessing the distribution of data obtained from the social media.
Figure 8 presents a forest plot showing the combined effect sizes obtained from the video games variable.
Figure 9 presents a funnel plot assessing the distribution of data obtained from the video games.
Figure 10 presents a forest plot displaying the combined effect sizes obtained from the results of all factors.
Figure 11 presents a funnel plot assessing the distribution of data obtained from all factors.
Additionally, the countries where the studies were conducted are presented as Supplementary material, and a heat map has been created on a world map to highlight the countries with the highest study count. The heat map is shown in Figure 12.
According to the meta-analysis results, Turkey has the highest number of publications (10), followed by the following countries: United States (9), Lebanon (4), South Korea (4), China (4), Saudi Arabia (4), Poland (3), Canada (3), Egypt (1), United Arab Emirates (2), Taiwan (2), Spain (2), Pakistan (2), India (2), Australia (2), Netherlands (2), Romania (1), Indonesia (1), Malaysia (1), Brazil (1), Norway (1), Iceland (1), Serbia (1), Greece (1), Singapore (1), Sweden (1), Nigeria (1), Hong Kong (1).
As a secondary analysis, as subgroup analysis was conducted in order to investigate that age and educational level variables significantly moderate the relationship between SA, SMU, VGs, and AP. The results were presented in Table 4.
Table 4. Meta-analysis results on the impact of moderator variables in the relationship between factors and academic performance.
In Table 4, for values exhibiting high heterogeneity (I2 > 25%), the moderating effects of factors considered to influence AP were examined, with particular attention to age and educational level. The analyses indicated that the assessed moderator variables did not have a statistically significant effect on AP.
This meta-analysis provides an in-depth investigation into the relationships among smartphone addiction (SA), social media use (SMU), video games (VGs), and academic performance (AP) across primary, secondary, and tertiary education levels. While smartphones and digital technologies are increasingly integrated into students’ lives, there remains a significant gap in the literature concerning their potential as learning tools and their impact on AP. By synthesizing data from 63 studies encompassing 124,166 participants, this analysis offers valuable insights for education policymakers and practitioners regarding the implications of SA, SMU, and VGs on students’ academic outcomes. The findings of this study shed light on the degree to which SA, SMU, and VGs influence academic achievement, and whether these effects are moderated by variables such as age, and educational level.
First, the results demonstrate a weak but statistically significant negative association between SA and AP (d = −0.129, 95% CI [−0.183, −0.075]). Hypothesis 1 (SA → AP): Accepted (statistically significant negative effect). supporting prior research indicating that excessive smartphone use can detract from students’ academic success. Several studies have similarly reported negative correlations between SA and AP, suggesting that smartphone overuse may divert attention away from academic responsibilities, leading to poorer academic outcomes (Asante and Hiadzi, 2018; Durak, 2018; Felisoni and Godoi, 2018; Li et al., 2015; Longnecker, 2017; Mendoza et al., 2018; Olufadi, 2015; Rozgonjuk et al., 2018; Spiratos, 2021; Zhou et al., 2022).
Second, the effect of SMU on AP was found to be weakly positive, though not statistically significant (d = 0.025, 95% CI [−0.051, 0.102]). Hypothesis 2 (SMU → AP): Rejected (not statistically significant). This suggests that while social media use may not substantially enhance academic performance, it does not appear to exert a strong detrimental effect either. This aligns with other studies that highlight the potential of social media as a resource for academic collaboration, information sharing, and peer learning (Dubuc et al., 2020; Kalam et al., 2023; Lim et al., 2021; Tomé-Fernández et al., 2020).
Third, the analysis revealed a weak negative association between VGs and AP (d = −0.134, 95% CI [−0.187, −0.082]). Hypothesis 3 (VG → AP): Accepted (statistically significant negative effect). This finding is consistent with the literature, which indicates that excessive gaming may hinder academic performance due to its time-consuming nature and its potential to displace more productive academic activities (Brunborg et al., 2014; Ekşi et al., 2020; Ferguson, 2015; Samaha and Hawi, 2020; Schmitt and Livingston, 2015; Shi et al., 2019; Suryawanshi et al., 2021; Toker and Baturay, 2016; Zhang et al., 2019; Zorbaz et al., 2015). However, the overall effect of SA, SMU, and VGs on AP (d = −0.085, 95% CI [−0.143, −0.028]). Hypothesis 4 (SA, SMU, VG → AP): Accepted (statistically significant combined negative effect). This finding suggests that, when considered together, these digital behaviors exert only a weak negative influence on academic outcomes. Contrary to widespread concerns about the harmful effects of digital technology, these findings imply that, under certain conditions, SA, SMU, and VGs may not be as damaging to academic success as is commonly believed. Moreover, the nuanced results highlight the complex interplay between these factors and AP, suggesting that their impact may vary based on individual usage patterns and contexts.
When examining potential moderators of the relationship between SA, SMU, VGs, and AP, variables such as gender, age, and educational level were analyzed. The findings revealed no significant moderating effect of these variables on academic performance, suggesting that the influence of SA, SMU, and VGs on AP is relatively consistent across different demographic groups. This result underscores the importance of further exploring other potential moderating factors, such as cultural context, socioeconomic status, or psychological traits, which may help explain variations in how these digital behaviors impact AP.
The findings of this meta-analysis contribute to a nuanced understanding of the relationships among smartphone addiction (SA), social media use (SMU), video games use (VGs), and academic performance (AP). The results indicate that both SA and VGs usage exert a weak negative impact on students’ academic performance, while SMU demonstrates a weak, albeit statistically insignificant, positive effect. Excessive engagement with smartphones, social media, and video games—particularly when it interferes with daily responsibilities—can lead to behavioral addiction, which adversely affects academic outcomes.
Consequently, fostering self-control and encouraging students to moderate their smartphone use may yield beneficial effects on their academic performance. Based on the findings of this study, we advocate for the implementation of educational policies, targeted teacher training, cognitive-behavioral interventions, and the development of specific teaching and learning strategies that prioritize harm reduction. These measures should address the potential for addiction associated with smartphone use, social media, and video games. Such initiatives could facilitate optimal study habits and enhance students’ academic performance.
This meta-analysis highlights several avenues for future research while acknowledging inherent limitations. Longitudinal studies are essential to explore the long-term impacts of smartphone addiction, social media use, and video game engagement on academic performance, thereby facilitating a clearer understanding of causal relationships over time. Additionally, research should extend to diverse populations, encompassing various age groups, cultural backgrounds, and educational systems to enhance the generalizability of findings. Employing qualitative methodologies could further enrich insights into students’ experiences and perceptions regarding digital behaviors and their academic consequences. Moreover, intervention studies aimed at reducing problematic usage patterns while promoting beneficial aspects of social media for academic enhancement are warranted. Future investigations should also consider additional moderating factors, such as socioeconomic status, personality traits, and contextual influences, that may impact the relationships between smartphone addiction, social media use, video game engagement, and academic performance.
Despite these potential directions, this meta-analysis is not without its limitations. The heterogeneity of the included studies, which varied in methodologies, measures of academic performance, and definitions of the key constructs, may affect the consistency and comparability of results. This meta-analysis is not without its limitations. One of the primary challenges lies in the conceptualization and measurement of SA, SMU, and VGs. Given the pervasive and multifaceted nature of smartphone use, it is difficult to distinguish between casual, problematic, and addictive use, which may lead to inconsistent findings across studies. There is a pressing need for the development of more robust and standardized tools to accurately assess these behaviors. Furthermore, the emerging nature of SA, SMU, and VGs, coupled with their rapid socio-cultural integration, has limited the availability of high-quality research on their academic effects. This limitation may have constrained the scope of this meta-analysis. Additionally, the possibility of publication bias remains, despite efforts to incorporate both published and unpublished studies, potentially influencing the findings. Moreover, while the overall sample size is substantial, many individual studies had small sample sizes or narrow demographic ranges, which limits the ability to draw broad conclusions. Furthermore, reliance on self-reported data for measuring academic performance and usage behaviors can introduce biases that may affect the accuracy of the findings. Lastly, the predominance of cross-sectional designs in the included studies restricts the capacity to establish definitive causal relationships among the variables examined.
The original contributions presented in the study are included in the article/Supplementary material, further inquiries can be directed to the corresponding author/s.
MK: Conceptualization, Data curation, Formal analysis, Funding acquisition, Investigation, Methodology, Project administration, Resources, Software, Supervision, Validation, Visualization, Writing – original draft, Writing – review & editing.
The author(s) declare that no financial support was received for the research, authorship, and/or publication of this article.
We are very grateful to the editors and reviewers for their valuable time.
The author declares that the research was conducted in the absence of any commercial or financial relationships that could be construed as a potential conflict of interest.
The authors declare that no Generative AI was used in the creation of this manuscript.
All claims expressed in this article are solely those of the authors and do not necessarily represent those of their affiliated organizations, or those of the publisher, the editors and the reviewers. Any product that may be evaluated in this article, or claim that may be made by its manufacturer, is not guaranteed or endorsed by the publisher.
The Supplementary material for this article can be found online at: https://www.frontiersin.org/articles/10.3389/fpsyg.2025.1524645/full#supplementary-material
Al Asqah, M. I., Al Orainey, A. I., Shukr, M. A., Al Oraini, H. M., and Al Turki, Y. A. (2020). The prevalence of internet gaming disorder among medical students at King Saud University, Riyadh, Saudi Arabia. Saudi Med. J. 41, 1359–1363. doi: 10.15537/smj.2020.12.05584
Alenezi, W., and Brinthaupt, T. M. (2022). The use of social media as a tool for learning: perspectives of students in the faculty of education at Kuwait University. Contemp. Educ. Technol. 14:ep340. doi: 10.30935/cedtech/11476
Alimoradi, Z., Lin, C. Y., Broström, A., Bülow, P. H., Bajalan, Z., Griffiths, M. D., et al. (2019). Internet addiction and sleep problems: a systematic review and meta-analysis. Sleep Med. Rev. 47, 51–61. doi: 10.1016/j.smrv.2019.06.004
Alosaimi, F. D., Alyahya, H., Alshahwan, H., Mahyijari, N. A., and Shaik, S. A. (2016). Smartphone addiction among university students in Riyadh, Saudi Arabia. Saudi Med. J. 37, 675–683. doi: 10.15537/smj.2016.6.14430
Alotaibi, N. B. (2019). Cyber Bullying and the Expected Consequences on the Students’ Academic Achievement. IEEE Access. 7, 153417–153431. doi: 10.1109/ACCESS.2019.2947163
Alotaibi, M. S., Fox, M., Coman, R., Ratan, Z. A., and Hosseinzadeh, H. (2022). Smartphone addiction prevalence and its association on academic performance, physical health, and mental well-being among university students in umm Al-Qura University (UQU), Saudi Arabia. Int. J. Environ. Res. Public Health 19:3710. doi: 10.3390/ijerph19063710
Andreassen, C. S., and Pallesen, S. (2014). Social network site addiction: an overview. Curr. Pharm. Des. 2014;20:4053–4061. doi: 10.2174/13816128113199990616
Arora, T., Albahri, A., Omar, O. M., Sharara, A., and Taheri, S. (2018). The prospective association between electronic device use before bedtime and academic attainment in adolescents. J. Adolesc. Health 63:451458, 451–458. doi: 10.1016/j.jadohealth.2018.04.007
Asante, R. K. B., and Hiadzi, R. A. (2018). In-lecture smartphone use and academic performance: a reflection on the sustainable development goal number four. Ghana Soc. Sci. J. 15, 161–178.
Ayenigbara, I. O. (2018). Gaming disorder and effects of gaming on health: An overview. J. Addict. Med. Therap. Sci. 4, 001–003. doi: 10.17352/2455-3484.000025
Bai, C., Chen, X., and Han, K. (2020). Mobile phone addiction and school performance among Chinese adolescents from low-income families: a moderated mediation model. Child Youth Serv. Rev. 118:105406. doi: 10.1016/j.childyouth.2020.105406
Bardakcı, S. (2019). Exploring high school students’ educational use of YouTube. Int. Rev. Res. Open Distributed Learn. 20, 260–278. doi: 10.19173/irrodl.v20i2.4074
Bavelier, D., and Green, C. S. (2019). Enhancing attentional control: lessons from action video games. Neuron 104, 147–163. doi: 10.1016/j.neuron.2019.09.031
Begg, C. B., and Mazumdar, M. (1994). Operating characteristics of a rank correlation test for publication bias. Biometrics 50, 1088–1101. doi: 10.2307/2533446
Benjet, C., Orozco, R., Albor, Y. C., Contreras, E. V., Monroy-Velasco, I. R., Hernández Uribe, P. C., et al. (2023). A longitudinal study on the impact of internet gaming disorder on self-perceived health, academic performance, and social life of first-year college students. Am. J. Addict. 32, 343–351. doi: 10.1111/ajad.13386
Bergdahl, N., Nouri, J., Fors, U., and Knutsson, O. (2020). Engagement, disengagement, and performance when learning with technologies in upper secondary school. Comput. Educ. 149:103783. doi: 10.1016/j.compedu.2019.103783
Bhandarkar, A. M., Pandey, A. K., Nayak, R., Pujary, K., and Kumar, A. (2021). Impact of social media on the academic performance of undergraduate medical students. Med. J. Armed Forces India 77, S37–S41. doi: 10.1016/j.mjafi.2020.10.021
Boer, M., Stevens, G. W., Finkenauer, C., de Looze, M. E., and van den Eijnden, R. J. (2021). Social media use intensity, social media use problems, and mental health among adolescents: investigating directionality and mediating processes. Comput. Hum. Behav. 116:106645. doi: 10.1016/j.chb.2020.106645
Borgonovi, F. (2016). Video gaming and gender differences in digital and printed reading performance among 15-year-olds students in 26 countries. J. Adolesc. 48, 45–61. doi: 10.1016/j.adolescence.2016.01.004
Brunborg, G. S., Mentzoni, R. A., and Frøyland, L. R. (2014). Is video gaming, or video game addiction, associated with depression, academic achievement, heavy episodic drinking, or conduct problems? J. Behav. Addict. 3, 27–32. doi: 10.1556/JBA.3.2014.002
Bun Lee, E. (2015). Too much information: heavy smartphone and Facebook utilization by African American young adults. J. Black Stud. 46, 44–61. doi: 10.1177/0021934714557034
Cai, Z., Mao, P., Wang, D., He, J., Chen, X., and Fan, X. (2022). Effects of scaffolding in digital game-based learning on student’s achievement: a three-level meta-analysis. Educ. Psychol. Rev. 34, 537–574. doi: 10.1007/s10648-021-09655-0
Chen, R. S., and Ji, C. H. (2015). Investigating the relationship between thinking style and personal electronic device use and its implications for academic performance. Comput. Hum. Behav. 52, 177–183. doi: 10.1016/j.chb.2015.05.042
Cheng, C., Lau, Y. C., Chan, L., and Luk, J. W. (2021). Prevalence of social media addiction across 32 nations: meta-analysis with subgroup analysis of classification schemes and cultural values. Addict. Behav. 117:106845. doi: 10.1016/j.addbeh.2021.106845
Çimen, A., and Yılmaz, M. B. (2017). How should we blend? The impact of blending social networks on high school students’ achievement and social networking behaviors. Educ. Sci. 42, 91–107. doi: 10.15390/EB.2017.7190
Ciris, V., Baskonus, T., Kartal, T., and Tasdemir, A. (2022). A study on digital game addictions of adolescents in the COVID-19 pandemic. J. Educ. Sci. Environ. Health 8, 168–186. doi: 10.55549/jeseh.1113707
Coskun, S., and Karayagız Muslu, G. (2019). Investigation of problematic Mobile phones use and fear of missing out (FoMO) level in adolescents. Community Ment. Health J. 55, 1004–1014. doi: 10.1007/s10597-019-00422-8
Domoff, S. E., Foley, R. P., and Ferkel, R. (2020). Addictive phone use and academic performance in adolescents. Human Behav. Emerg. Technol. 2, 33–38. doi: 10.1002/hbe2.171
Dontre, A. J. (2021). The influence of technology on academic distraction: a review. Hum. Behav. Emerg. Technol. 3, 379–390. doi: 10.1002/hbe2.229
Dos, B. (2014). The relationship between mobile phone use, metacognitive awareness and academic achievement. Eur. J. Educ. Res. 5, 192–200. doi: 10.12973/eu-jer.3.4.192
Dowling, N. A. (2014). Issues raised by the DSM-5 internet gaming disorder classification and proposed diagnostic criteria. Addiction 109, 1408–1409. doi: 10.1111/add.12554
Dubuc, M., Aubertin-Leheudre, M., and Karelis, A. D. (2020). Lifestyle habits predict academic performance in high school students: the adolescent student academic performance longitudinal study (ASAP). Int. J. Environ. Res. Public Health 17:243. doi: 10.3390/ijerph17010243
Durak, H. Y. (2018). Investigation of nomophobia and smartphone addiction predictors among adolescents in Turkey: demographic variables and academic performance. Soc. Sci. J. 56, 492–517. doi: 10.1016/j.soscij.2018.09.003
Ekşi, F., Demirci, İ., and Tanyeri, H. (2020). Problematic technology use and well-being in adolescence: the personal and relational effects of technology. Addicta 7, 107–121. doi: 10.5152/ADDICTA.2020.19077
ELNahas, G., Elella, E. A., Hewedi, D., Elhabiby, M., Elkholy, H., Mansour, O., et al. (2018). Problematic online gaming among a sample of university students in Egypt. Addict. Disord. Treat. 17, 161–167. doi: 10.1097/ADT.0000000000000141
Eoh, Y., Lee, E., and Park, S. H. (2022). The relationship between children’s school adaptation, academic achievement, happiness, and problematic smartphone usage: a multiple informant moderated mediating model. Appl. Res. Qual. Life 17, 3579–3593. doi: 10.1007/s11482-022-10080-w
Felisoni, D. D., and Godoi, A. S. (2018). Cell phone usage and academic performance: An experiment. Comput. Educ. 117, 175–187. doi: 10.1016/j.compedu.2017.10.006
Ferguson, C. J. (2015). Do angry birds make for angry children? A meta-analysis of video game influences on children’s and adolescents’ aggression, mental health, prosocial behavior, and academic performance. Perspect. Psychol. Sci. 10, 646–666. doi: 10.1177/1745691615592234
Fernández-Andújar, M., Alonso, M. O., Sorribes, E., Villalba, V., and Calderon, C. (2022). Internet addiction, phubbing, psychological variables and self-perception of mathematical competence in college students. Mathematics 10:2631. doi: 10.3390/math10152631
Field, A. P., and Gillett, R. (2010). How to do a meta- analysis. Br. J. Math. Stat. Psychol. 63, 665–694. doi: 10.1348/000711010X502733
Freelon, D. (2013). ReCal OIR: ordinal, interval, and ratio intercoder reliability as a web service. Int. J. Internet Sci. 8, 10–16.
Gazibara, T., Cakic, J., Cakic, M., Grgurevic, A., and Pekmezovic, T. (2020). Searching for online health information instead of seeing a physician: a cross-sectional study among high school students in Belgrade, Serbia. Int. J. Public Health 65:1269
Gilbert, N. (2023). Number of gamers worldwide 2024: demographics, statistics, and predictions. Financesonline.Com Available at: https://financesonline.com/numberofgamersworldwide/ (Accessed September 16, 2024).
Griffiths, M. D. (2010). The role of context in online gaming excess and addiction: some case study evidence. Int. J. Ment. Heal. Addict. 8, 119–125. doi: 10.1007/s11469-009-9229-x
Han, S. (2022). Impact of smartphones on students: how age at first use and duration of usage affect learning and academic progress. Technol. Soc. 70:102002. doi: 10.1016/j.techsoc.2022.102002
Han, S., and Yi, Y. J. (2019). How Does the Smartphone Usage of College Students Affect Academic Performance?. J. Comput. Assist. Learn, 35, 13–22. doi: 10.1111/jcal.12306
Hawi, N. S., and Samaha, M. (2016). To excel or not to excel: strong evidence on the adverse effect of smartphone addiction on academic performance. Comput. Educ. 98, 81–89. doi: 10.1016/j.compedu.2016.03.007
Hawi, N. S., Samaha, M., and Griffiths, M. D. (2018). Internet gaming disorder in Lebanon: relationships with age, sleep habits, and academic achievement. J. Behav. Addict. 7, 70–78. doi: 10.1556/2006.7.2018.16
Homaid, A. A. (2022). Problematic social media use and associated consequences on academic performance decrement during Covid-19. Addict. Behav. 132:107370. doi: 10.1016/j.addbeh.2022.107370
Hussein, M. H., Ow, S. H., Cheong, L. S., Thong, M. K., and Ebrahim, N. A. (2019). Effects of digital gamebased learning on elementary science learning: a systematic review. IEEE Access 7, 62465–62478. doi: 10.1109/ACCESS.2019.2916324
Hussein, M. H., Ow, S. H., Elaish, M. M., and Jensen, E. O. (2022). Digital game-based learning in K-12 mathematics education: a systematic literature review. Educ. Inf. Technol. 27, 2859–2891. doi: 10.1007/s10639-021-10721-x
Hwang, K.-H., Yoo, Y. S., and Cho, O.-H. (2012). Smartphone overuse and upper extremity pain, anxiety, depression, and interpersonal relationships among college students. J. Korea Contents Assoc.n 12, 365–375. doi: 10.5392/JKCA.2012.12.10.365
Jaafar, N. R. N., Baharudin, A., Tajjudin, I., Ling, L. S., Amirul, M., Safarudin, D. S. S., et al. (2021). Factors correlated with internet gaming disorder among Malaysian university students. Malaysian J. Med. Health Sci. 17, 54–62.
Jankovic, B., Nikolic, M., Vukonjanski, J., and Terek, E. (2016). The impact of Facebook and smartphone usage on the leisure activities and college adjustment of students in Serbia. Comput. Hum. Behav. 55, 354–363. doi: 10.1016/j.chb.2015.09.022
Jeong, G.-C. (2016). Relationships among mental health, internet addiction, and smartphone addiction in university students. J. Korea Contents Assoc. 16, 655–665. doi: 10.5392/JKCA.2016.16.04.655
Kalam, A., Goi, C. L., and Tiong, Y. Y. (2023). Student motivations for social media use and their effects on academic performance- a meditational approach in emerging market. Interact. Technol. Smart Educ. 20, 313–334. doi: 10.1108/ITSE-09-2022-0115
Karnadi, E. B., and Pangestu, S. (2021). Does internet gaming disorder hinder academic performance? Jurnal Kajian Bimbingan Konseling 6, 1–14. doi: 10.17977/um001v6i12021p001
Karpinski, A. C., Kirschner, P. A., Ozer, I., Mellott, J. A., and Ochwo, P. (2013). An exploration of social networking site use, multitasking, and academic performance among United States and European university students. Comput. Hum. Behav. 29, 1182–1192. doi: 10.1016/j.chb.2012.10.011
Khan, M. L., Wohn, D. Y., and Ellison, N. B. (2014). Actual friends matter: An internet skills perspective on teens’ informal academic collaboration on Facebook. Comput. Educ. 79, 138–147. doi: 10.1016/j.compedu.2014.08.001
Kim, M. H., Min, S., Ahn, J. S., An, C., and Lee, J. (2019). Association between high adolescent smartphone use and academic impairment, conflicts with family members or friends, and suicide attempts. PLoS One 14:e0219831. doi: 10.1371/journal.pone.0219831
Kim, H.-J., Min, J.-Y., Kim, H.-J., and Min, K.-B. (2017). Accident risk associated with smartphone addiction: a study on university students in Korea. J. Behav. Addict. 6, 699–707. doi: 10.1556/2006.6.2017.070
Kim, H.-J., Min, J.-Y., Kim, H.-J., and Min, K.-B. (2019). Association between psychological and self-assessed health status and smartphone overuse among Korean college students. J. Ment. Health 28, 11–16. doi: 10.1080/09638237.2017.1370641
Kim, J.-H., Seo, M., and David, P. (2015). Alleviating depression only to become problematic mobile phone users: can face-to-face communication be the antidote? Comput. Hum. Behav. 51, 440–447. doi: 10.1016/j.chb.2015.05.030
Kitchenham, B., and Charters, S. (2007). Guidelines for performing systematic literature reviews in software engineering. 2. Technical Report EBSE 2007-001, Keele University and Durham University Joint Report.
Kuh, G. D., Jankowski, N., Ikenberry, S. O., and Kinzie, J. L. (2014). Knowing what students know and can do: The current state of student learning outcomes assessment in US colleges and universities. Urbana, IL: National Institute for Learning Outcomes Assessment.
Kumar, S., Agarwal, M., and Agarwal, N. (2021). Defining and measuring academic performance of HEI students- a critical review. Turkish J. Comp. Math. Educ. 12, 3091–3105.
Kumar, C. S., Sharma, M. K., Amudhan, S., Arya, S., Mahapatra, S., Anand, N., et al. (2023). Digital gaming, musculoskeletal, and related health hazards among adolescents and young adults. Indian J. Psychiatry 65, 698–700. doi: 10.4103/indianjpsychiatry.indianjpsychiatry_818_22
Kuss, D. J., and Griffiths, M. D. (2017). Social networking sites and addiction: ten lessons learned. Int. J. Environ. Res. Public Health 14:311. doi: 10.3390/ijerph14030311
Lam, L. T. (2014). Internet gaming addiction, problematic use of the internet, and sleep problems: a systematic review. Curr. Psychiatry Rep. 16, 444–449. doi: 10.1007/s11920-014-0444-1
Lau, W. F. (2017). Effects of social media usage and social media multitasking on the academic performance of university students. Comput. Hum. Behav. 68, 286–291. doi: 10.1016/j.chb.2016.11.043
Lee, K. E., Kim, S.-H., Ha, T.-Y., Yoo, Y.-M., Han, J.-J., Jung, J.-H., et al. (2016). Dependency on smartphone use and its association with anxiety in Korea. Public Health Rep. 131, 411–419. doi: 10.1177/003335491613100307
Lepp, A., Barkley, J. E., and Karpinski, A. C. (2014). The relationship between cell phone use, academic performance, anxiety, and satisfaction with life in college students. Comput. Hum. Behav. 31, 343–350. doi: 10.1016/j.chb.2013.10.049
Lepp, A., Barkley, J. E., and Karpinski, A. C. (2015). The relationship between cell phone use and academic performance in a sample of U.S. college students. SAGE Open 5:2158244015573169. doi: 10.1177/2158244015573169
Li, J., Lepp, A., and Barkley, J. E. (2015). Locus of control and cell phone use: implication for sleep quality, academic performance, and subjective well-being. Comput. Hum. Behav. 52, 450–457. doi: 10.1016/j.chb.2015.06.021
Lim, W. P., Loo, J. Y., Lee, K., Pui, H. M., and Ting, T. T. (2021). The impact of social media on student’s academic performance: a survey on TAR UC computing students in Malaysia during COVID-19 pandemic. Proceed. Int. Conf. Digital Transf. Applic.
Lin, L. (2018). Bias caused by sampling error in meta-analysis with small sample sizes. PLoS One 13:e0204056. doi: 10.1371/journal.pone.0204056
Lin, T. T. C., and Chiang, Y. H. (2017). Investigating predictors of smartphone dependency symptoms and effects on academic performance, improper phone use and perceived sociability. Int. J. Mob. Commun. 15:655. doi: 10.1504/IJMC.2017.086881
Longnecker, E. M. (2017). The relationship between smartphone use, symptoms of depression, symptoms of anxiety, and academic performance in college students (s. 11051303) [master of science, Iowa State University, digital repository]
Mansour, A., Almutairi, H., Alwehaibi, I., Alshowaiman, A., Alsaif, M., and Almutairi, E. (2020). The impact of using social media on the academic performance of Qassim university students, Unaizah, Saudi Arabia. Int. J. Med. Develop. Countries 4, 2085–2093. doi: 10.24911/ijmdc.51-1600133225
Masalimova, A. R., Kosheleva, Y. P., Kosarenko, N. N., Kashina, S. G., Sokolova, E. G., and Iakovleva, E. V. (2023). Effects of social networking sites on university students’ academic performance: a systematic review. J. Commun. Media Technol. 13:e202339. doi: 10.30935/ojcmt/13365
Mendoza, J. S., Pody, B. C., Lee, S., Kim, M., and McDonough, I. M. (2018). The effects of cell phones on attention and learning: the influence of time, distraction, and nomophobia. Comput. Hum. Behav. 86, 52–60. doi: 10.1016/j.chb.2018.04.027
Moher, D., Shamseer, L., Clarke, M., Ghersi, D., Liberati, A., Petticrew, M., et al. (2015). Preferred reporting items for systematic review and meta-analysis protocols (PRISMA-P) 2015 statement. Syst. Rev. 4:1. doi: 10.1186/2046-4053-4-1
Molla-Esparza, C., López-González, E., and Losilla, J. (2020). Sexting prevalence and socio-demographic correlates in Spanish secondary school students. Sex. Res. Soc. Policy 18, 97–111. doi: 10.1007/s13178-020-00434-0
Mylona, I., Deres, E. S., Dere, G. D. S., Tsinopoulos, I., and Glynatsis, M. (2020). The impact of internet and videogaming addiction on adolescent vision: a review of the literature. Front. Public Health 63:63. doi: 10.3389/fpubh.2020.00063
Nayak, J. K. (2018). Relationship among smartphone usage, addiction, academic performance and the moderating role of gender: a study of higher education students in India. Comput. Educ. 123, 164–173. doi: 10.1016/j.compedu.2018.05.007
Nuyens, F., Kuss, D. J., Lopez-Fernandez, O., and Griffiths, M. D. (2017). The experimental analysis of problematic video gaming and cognitive skills: a systematic review. Journal Thérapie Comportementale Cognitive 27, 110–117. doi: 10.1016/j.jtcc.2017.05.001
Nuyens, F. M., Kuss, D. J., Lopez-Fernandez, O., and Griffiths, M. D. (2019). The empirical analysis of non-problematic video gaming and cognitive skills: a systematic review. Int. J. Ment. Heal. Addict. 17, 389–414. doi: 10.1007/s11469-018-9946-0
O’Dea, S. (2023). Number of smartphone subscriptions worldwide from 2016 to 2027. Statista. Available at: https://www.statista.com/statistics/330695/number-of-smartphone-users-worldwide/ (Accessed June 30, 2024).
Olufadi, Y. (2015). A configurational approach to the investigation of the multiple paths to success of students through mobile phone use behaviors. Comput. Educ. 86, 84–104. doi: 10.1016/j.compedu.2015.03.005
Osuagwu, T. (2015). Excessive game-playing and children’s academic performance in Port Harcourt, Nigeria. GSTF J. Media Commun. 2:18. doi: 10.7603/s40874-014-0018-1
Page, M. J., McKenzie, J. E., Bossuyt, P. M., Boutron, I., Hoffmann, T. C., Mulrow, C. D., et al. (2021). The PRISMA 2020 statement: An updated guideline for reporting systematic reviews. Int. J. Surg. 88:105906. doi: 10.1016/j.ijsu.2021.105906
Park, N., and Lee, H. (2012). Social implications of smartphone use: Korean college students' smartphone use and psychological well-being. Cyberpsychol. Behav. Soc. Netw. 15, 491–497. doi: 10.1089/cyber.2011.0580
Pedersen, W., Vestel, V., and Bakken, A. (2018). At risk for radicalization and jihadism? A population-based study of Norwegian adolescents. Coop. Confl. 53, 61–83. doi: 10.1177/0010836717716721
Polat, A., and Topal, M. (2022). Relationship between digital game addiction with body mass index, academic achievement, player types, gaming time: a cross-sectional study. J. Educ. Technol. Online Learn. 5, 901–915. doi: 10.31681/jetol.1156594
Pontes, H. M., Kiraly, O., Demetrovics, Z., and Griffiths, M. D. (2014). The conceptualization and measurement of DSM-5 internet gaming disorder: the development of the IGD-20 test. PLoS One 9:e110137. doi: 10.1371/journal.pone.0110137
Przepiorka, A., Błachnio, A., Cudo, A., and Kot, P. (2021). Social anxiety and social skills via problematic smartphone use for predicting somatic symptoms and academic performance at primary school. Comput. Educ. 173, 104286–104213. doi: 10.1016/j.compedu.2021.104286
Qahri-Saremi, H., and Turel, O. (2016). School engagement, information technology use, and educational development: An empirical investigation of adolescents. Comput. Educ. 102, 65–78. doi: 10.1016/j.compedu.2016.07.004
Rashid, T., and Asghar, H. M. (2016). Technology use, self-directed learning, student engagement and academic performance: examining the relations. Comput. Hum. Behav. 63, 604–612. doi: 10.1016/j.chb.2016.05.084
Rehbein, F., Kliem, S., Baier, D., Mößle, T., and Petry, N. M. (2015). Prevalence of internet gaming disorder in German adolescents: diagnostic contribution of the nine DSM-5 criteria in a state-wide representative sample. Addiction 110, 842–851. doi: 10.1111/add.12849
Reynaldo, C., Christian, R., Hosea, H., and Gunawan, A. A. (2021). Using video games to improve capabilities in decision making and cognitive skill: a literature review. Procedia Comput. Sci. 179, 211–221. doi: 10.1016/j.procs.2020.12.027
Rosen, L. D., Carrier, L. M., Pedroza, J. A., Elias, S., O’Brien, K. M., Karina Kim, J. L., et al. (2018). The role of executive functioning and technological anxiety (FOMO) in college course performance as mediated by technology usage and multitasking habits. Psicología Educativa 24, 14–25. doi: 10.5093/psed2018a3
Rozgonjuk, D., Saal, K., and That, K. (2018). Problematic smartphone use, deep and surface approaches to learning, and social media use in lectures. Int. J. Environ. Res. Public Health 15:92. doi: 10.3390/ijerph15010092
Sahin, M., Gumus, Y. Y., and Dincel, S. (2016). Game addiction and academic achievement. Educ. Psychol. 36, 1533–1543. doi: 10.1080/01443410.2014.972342
Samaha, M., and Hawi, N. S. (2020). Internet gaming disorder and its relationships with student engagement and academic performance. Int. J. Cyber Behav. Psychol. Learn. 10, 14–33. doi: 10.4018/IJCBPL.2020100102
Sampasa-Kanyinga, H., Chaput, J.-P., and Hamilton, H. A. (2019). Social media use, school connectedness, and academic performance among adolescents. J. Prim. Prev. 40, 189–211. doi: 10.1007/s10935-019-00543-6
Schmitt, Z. L., and Livingston, M. G. (2015). Video game addiction and college performance among males: results from a 1 year longitudinal study. Cyberpsychol. Behav. Soc. Network. 18, 25–29. doi: 10.1089/cyber.2014.0403
Scott, D. A., Valley, B., and Simecka, B. A. (2016). Mental health concerns in the digital age. Int. J. Ment. Heal. Addict. 15, 604–613. doi: 10.1007/s11469-016-9684-0
Sert, H., Taskin Yilmaz, F., Karakoc Kumsar, A., and Aygin, D. (2019). Effect of technology addiction on academic success and fatigue among Turkish university students. Fatigue 7, 41–51. doi: 10.1080/21641846.2019.1585598
Shafiq, M., and Parveen, K. (2023). Social media usage: Analyzing its effect on academic performance and engagement of higher education students. Int. J. Educ. Dev. 98:102738. doi: 10.1016/j.ijedudev.2023.102738
Shen, J. (2019). Social-media use and academic performance among undergraduates in biology. Biochem. Mol. Biol. Educ. 47, 615–619. doi: 10.1002/bmb.21293
Shi, J., Boak, A., Mann, R., and Turner, N. E. (2019). Adolescent problem video gaming in urban and non-urban regions. Int. J. Ment. Heal. Addict. 17, 817–827. doi: 10.1007/s11469-018-9872-1
Spiratos, K. N. (2021). Problematic smartphone use among high school students and its relationships with depression, stress, selfesteem, grit and academic performance, doctoral dissertation. California State University.
Statista. (2024),. Number of video gamers worldwide 2019-2029. Available at: https://www.statista.com/statistics/748044/number-video-gamers-world/ (Accessed June 30, 2024).
Sun, Y., and Zhang, Y. (2020). A review of theories and models applied in studies of social media addiction and implications for future research. Addict. Behav. 114:106699. doi: 10.1016/j.addbeh.2020.106699
Suryawanshi, D. M., Rajaseharan, D., Venugopal, R., Mathew, M., Joy, A., and Goyal, R. (2021). The association between gaming practices and scholastic performance among medical students in India: Casecontrol study. JMIR Med. Educ. 7:e22235. doi: 10.2196/22235
Swain, R. K., and Pati, A. K. (2021). Use of social networking sites (SNSs) and its repercussions on sleep quality, psychosocial behavior, academic performance and circadian rhythm of humans-a brief review. Biol. Rhythm. Res. 52, 1139–1178. doi: 10.1080/09291016.2019.1620487
Sweller, J. (1994). Cognitive load theory, learning difficulty, and instructional design. Learn. Instr. 4, 295–312. doi: 10.1016/0959-4752(94)90003-5
Tang, S., and Patrick, M. E. (2018). Technology and interactive social media use among 8th and 10th graders in the US and associations with homework and school grades. Comput. Hum. Behav. 86, 34–44. doi: 10.1016/j.chb.2018.04.025
Tessier, J. (2013). Student impressions of academic cellphone use in the classroom. J. Coll. Sci. Teach. 43, 25–29. doi: 10.2505/4/jcst13_043_01_25
Toker, S., and Baturay, M. H. (2016). Antecedents and consequences of game addiction. Comput. Hum. Behav. 55, 668–679. doi: 10.1016/j.chb.2015.10.002
Tomé-Fernández, M., García-Garnica, M., Martínez-Martínez, A., and Olmedo-Moreno, E. M. (2020). An analysis of personal learning environments and age-related psychosocial factors of unaccompanied foreign minors. Int. J. Environ. Res. Public Health 17:3700. doi: 10.3390/ijerph17103700
Tsai, H., and Liu, S. (2015). Relationships between time-management skills, Facebook interpersonal skills, and academic achievement among junior high school students. Soc. Psychol. Educ. 18, 503–516. doi: 10.1007/s11218-015-9297-7
Tsitsika, A. K., Tzavela, E. C., Janikian, M., Ólafsson, K., Iordache, A., Schoenmakers, T. M., et al. (2014). Online social networking in adolescence: patterns of use in six European countries and links with psychosocial functioning. J. Adolesc. Health 55, 141–147. doi: 10.1016/j.jadohealth.2013.11.010
Uzun, A. M., and Kilis, S. (2019). Does persistent involvement in media and technology lead to lower academic performance? Evaluating media and technology use in relation to multitasking, self-regulation and academic performance. Comput. Hum. Behav. 90, 196–203. doi: 10.1016/j.chb.2018.08.045
Van Den Eijnden, R., Koning, I., Doornwaard, S., Van Gurp, F., and Ter Bogt, T. (2018). The impact of heavy and disordered use of games and social media on adolescents’ psychological, social, and school functioning. J. Behav. Addict. 7, 697–706. doi: 10.1556/2006.7.2018.65
Wakefield, J., and Frawley, J. K. (2020). How does students’ general academic achievement moderate the implications of social networking on specific levels of learning performance? Comput. Educ. 144:103694. doi: 10.1016/j.compedu.2019.103694
Wentworth, D. K., and Middleton, J. H. (2014). Technology use and academic performance. Comput. Educ. 78, 306–311. doi: 10.1016/j.compedu.2014.06.012
World Health Organisation. (2020). Addictive disorders: gaming disorder. Available at: https://www.who.int/news-room/questionsandanswers/item/addictivebehaviours%20gamingdisorder
Winskel, H., Kim, T. H., Kardash, L., and Belic, I. (2019). Smartphone use and study behavior: a Korean and Australian comparison. Heliyon 5:e02158. doi: 10.1016/j.heliyon.2019.e02158
Wu, J., and Siu, A. C. K. (2020). Problematic mobile phone use by Hong Kong adolescents. Front. Psychol. 11:551804. doi: 10.3389/fpsyg.2020.551804
Yan, Z., and Yang, Z. (2017). Generalized internet addiction among Chinese university students: Associations with internet attitudes, objectively measured smartphone usage, and personality post-secondary education student mental health: A global perspective (pp. 445–461). CDSPress. Available at: https://cdspress.ca/?page_id=6135.
Yang, Z., Asbury, K., and Griffiths, M. D. (2018). An exploration of problematic smartphone use among Chinese university students: associations with academic anxiety, academic procrastination, self-regulation and subjective well-being. Int. J. Ment. Heal. Addict. 17, 596–614. doi: 10.1007/s11469-018-9961-1
Yang, H. M., Tong, K. K., Li, Y., Tao, V. Y., Zhang, M. X., and Wu, A. M. (2022). Testing the influence of social axioms on internet gaming disorder tendency with a cross-lagged panel model: a one-year longitudinal study. Int. J. Ment. Heal. Addict. 20, 2587–2598. doi: 10.1007/s11469-021-00532-z
Yoo, J. H., Cheon, E. Y., and Kim, H. (2020). Effects of empathy, self-control, and smartphone dependency on interpersonal relationship in nursing students. J. Korea Academia Industrial Cooperation Society 21, 82–90. doi: 10.5762/KAIS.2020.21.4.82
York, T. T., Gibson, C., and Rankin, S. (2015). Defining and measuring academic success. Pract. Assessment Res. Eval. 20:5. doi: 10.7275/hz5x-tx03
Zahra, S., Ahsan, S., Kiani, S., Shahbaz, K., and Andleeb, S. N. (2020). Internet gaming, emotional intelligence, psychological distress, and academic performance among university students. Pak. J. Psychol. Res. 35, 253–270. doi: 10.33824/PJPR.2020.35.2.14
Zhang, M. X., Wang, X., Shu, M. Y., and Wu, A. M. (2019). Purpose in life, social support, and internet gaming disorder among Chinese university students: a 1-year follow-up study. Addict. Behav. 99:106070:106070. doi: 10.1016/j.addbeh.2019.106070
Zhou, D., Liu, J., Wang, T., Liu, J., and Li, G. (2022). Relationships among problematic smartphone use, mathematics anxiety, learning interest, and achievement: a multiple mediation model. Comput. Hum. Behav. 129:107171. doi: 10.1016/j.chb.2021.107171
Zhou, D., Liu, J., Ye, G., Wang, T., Xia, X., and Liu, J. (2022). Relationships among problematic smartphone use, mathematics achievement, teacher–student relationships, and subjective well-being: results from a large-scale survey in China. Behav. Sci. 12:454. doi: 10.3390/bs12110454
Zimmerman, B. J. (2000). “Attaining self-regulation: A social cognitive perspective” in Handbook of self-regulation. eds. M. Boekaerts, P. R. Pintrich, and M. Zeidner (Academic Press), 13–39.
Keywords: academic performance, meta-analysis, smartphone addiction, social media use, videogames
Citation: Kuş M (2025) A meta-analysis of the impact of technology related factors on students’ academic performance. Front. Psychol. 16:1524645. doi: 10.3389/fpsyg.2025.1524645
Received: 08 November 2024; Accepted: 04 February 2025;
Published: 21 February 2025.
Edited by:
Laisa Liane Paineiras-Domingos, Federal University of Bahia (UFBA), BrazilReviewed by:
María Inés Martín-García, University of Castilla-La Mancha, SpainCopyright © 2025 Kuş. This is an open-access article distributed under the terms of the Creative Commons Attribution License (CC BY). The use, distribution or reproduction in other forums is permitted, provided the original author(s) and the copyright owner(s) are credited and that the original publication in this journal is cited, in accordance with accepted academic practice. No use, distribution or reproduction is permitted which does not comply with these terms.
*Correspondence: Metin Kuş, bWV0aW5rdXNAaGl0aXQuZWR1LnRy
Disclaimer: All claims expressed in this article are solely those of the authors and do not necessarily represent those of their affiliated organizations, or those of the publisher, the editors and the reviewers. Any product that may be evaluated in this article or claim that may be made by its manufacturer is not guaranteed or endorsed by the publisher.
Research integrity at Frontiers
Learn more about the work of our research integrity team to safeguard the quality of each article we publish.