- 1U.S. Army DEVCOM Soldier Center, Natick, MA, United States
- 2Center for Applied Brain and Cognitive Sciences, Tufts University, Medford, MA, United States
- 3Department of Electrical and Computer Engineering, Tufts University, Medford, MA, United States
Introduction: Personnel performance under stress hinges on various factors, including individual traits, training, context, mental and physiological states, and task demands. This study explored the link between the traits of military personnel and their performance outcomes in five domains: move, shoot, communicate, navigate, and sustain.
Methods: A total of 387 U.S. Army soldiers participated in this study, undergoing trait assessments covering physical, cognitive, social–emotional, demographic/lifestyle, and health domains. Performance was measured through lab and field events assessing a broad range of individual and team-level skills under conditions demanding resilience to acute cognitive and physical stress exposure. Analysis used feature selection and elastic net regression.
Results: Analyses revealed complex associations between traits and performance, with physical, cognitive, health-related, social–emotional, and lifestyle traits playing roles in guiding and constraining performance. Measures of resilience, emotion regulation, grit, and mindfulness were identified as relevant predictors of several performance-related outcomes.
Discussion: Results carry implications for the selection, training, and operational effectiveness of personnel in high-stakes occupations including military and first response. Further research is necessary to explore the mechanisms underlying these associations and inform targeted interventions to boost personnel effectiveness.
1 Introduction
Human performance is inherently variable within and between individuals and across contexts, making it very challenging to predict (Newell, 1993; Smith et al., 2014). Relatively invariant individual traits across the physical, social–emotional, cognitive, demographic/lifestyle, and health domains may help account for inter-individual performance variability (Motowidlo et al., 1997; Muhs et al., 2018). However, while one or more trait-level variables might predict performance on a specific task executed in a specific context, those results may not generalize to performance in a novel context or with a modified task; indeed, the heterogeneity of performance contexts and tasks can make it difficult to generalize results between studies (Araújo et al., 2007; Salmon, 2020). Herein, our intent was to identify a reduced set of trait-level assessments (i.e., tasks, questionnaires) and measures (i.e., quantitative measures) that together can predict coarse performance constructs broadly applicable to Soldier performance across settings that demand resilience to acute stress.
1.1 Trait-level predictors of human performance
Human performance is a challenging and nebulous concept, with many different definitions proposed over the past several decades (Table 1). Definitions of human performance vary dramatically in level of analysis (e.g., individual versus group) and contextual specificity versus generality. They also vary regarding whether the processes underlying behavior (e.g., perception, emotion), behavior itself (i.e., observable outputs), and/or the results of behavior (e.g., winning a competition) are considered. In military training and operations, performance tends to be very outcome oriented, including assessments of task completion, efficiency and accuracy, and meeting or exceeding a superior’s intent. Performing tasks at an adequate level is continuously guided by mental and physical processes including attention, perception, memory, decision making, emotion, reasoning, agility, strength, endurance, flexibility, and balance.
The ability to predict human performance represents a formidable challenge for many occupational domains, with implications for personnel selection, training, and assessment. Performance prediction can be used to identify personnel who are likely to excel at particular occupational tasks, track the effectiveness of training trajectories, identify individualized performance optimization and enhancement techniques, optimize human-machine interaction, prevent injury and burnout, reduce the risk of stress disorders, and increase retention (Kaplan, 1965; Bartone et al., 2008; Campbell and Knapp, 2013; Farina et al., 2019; Brunyé et al., 2020; Samuelson et al., 2020). Herein, our analyses are restricted to the consideration of how traits predict performance. Traits are relatively invariant and enduring characteristics of individuals that differentiate them from others and have causal effects on performance (Steyer et al., 2015). While traits can indeed change over time due to experiences and training, we contrast traits with states, which are relatively transient and situationally dependent; states are not considered herein. In the present work, we conceptualize traits as falling into five general domains: physical, social–emotional, cognitive, demographic, and health-related.
1.1.1 Physical traits
Physical traits are both structural and functional, such as body dimensions and composition, and strength and endurance (Casadei and Kiel, 2023). Not all physical traits are constant; indeed, some physical traits are highly invariant (e.g., height) over time whereas others might change slowly over the course of months or years (e.g., body mass). Physical traits have been linked to a variety of performance measures in both the physical and cognitive domains. For example, cardiovascular fitness is associated with cognitive function, functional brain connectivity, lower rates of mental health disorders, and stress reactivity (Belsky et al., 2015; Kronman et al., 2020; Schilling et al., 2020; Neumann et al., 2022). The precise nature of these relationships, including their strength and directionality, remains to be determined. The present study assessed several physical traits in military personnel, including aerobic and anaerobic endurance, agility and flexibility, body dimensions, activity level, power, and movement quality. Measuring these traits involved administering questionnaires, body/anthropometric measurements, anaerobic fitness assessments, functional movement screening, and the Army Combat Fitness Test (ACFT).
1.1.2 Social–emotional traits
Social–emotional traits generally include those associated with relational and prosocial skills, and competence in recognizing and regulating emotions (Humphrey et al., 2011). While many social–emotional traits are likely relatively static (e.g., extraversion) over time, some might change through experiences and training (e.g., resilience). Social–emotional traits include relationship building and social problem-solving skills (including cooperation, turn-taking, and listening skills), self- and social-awareness, and the ability to regulate emotions and behavior and maintain resilience under conditions of stress and adversity. Resilience to stress exposure is likely a critical factor for sustaining performance, particularly in military contexts. During both training and operations, soldiers are frequently exposed to high-stress situations that can impact their cognitive and physical functioning. Psychological and physiological manifestations of resilience allow individuals to maintain optimal performance levels despite these challenges (Chappelle et al., 2018; Flood and Keegan, 2022; Biggs et al., 2023; McClung et al., 2023), providing a capacity to recover quickly from stress, adapt to changing environments, and remain focused under pressure (Algoe and Fredrickson, 2011; Franklin et al., 2012; Pfau and Russo, 2015; Crane et al., 2019; Kalisch et al., 2024). Resilience is not only vital for completing tasks efficiently but also for making accurate decisions, managing resources effectively, and maintaining overall mission readiness. In the present study, all performance tasks were performed under laboratory or field conditions that were inherently stressful (mentally and/or physically), as detailed in the Methods; those with higher trait levels of resilience are more likely to sustain performance under such conditions. The present study assessed several social–emotional traits in military personnel, including several self-reported measures of mindfulness, resilience, emotion regulation and stress responsiveness, impulsivity, personality, motivation, and trait affect.
1.1.3 Cognitive traits
Cognitive traits are defined as the structural and functional central and peripheral nervous system characteristics that allow individuals to acquire, retain, and flexibly use information (Shettleworth, 2009; Croston et al., 2015). While most cognitive traits are relatively static (e.g., risk taking propensity) over time, some may change over months or years due to experience and training (e.g., working memory capacity). People vary in their ability to process information, control attention and behavior, update memory, learn new materials, apply knowledge, reason and produce judgments, and make decisions (Lee and Webb, 2005; Der and Deary, 2006; Deary et al., 2010; Kanai and Rees, 2011; Boogert et al., 2018; Naug and Tait, 2021). Cognitive traits have been linked to various performance measures across civilian and military domains including air traffic control skills, academic success, second language learning, multitasking, motor sequence learning, and resistance to misinformation (Ackerman, 1992; Bo and Seidler, 2009; Linck et al., 2009; Poole and Kane, 2009; Rabin et al., 2011; McVay and Kane, 2012; Wingo et al., 2013; Kapa and Colombo, 2014; Pollard and Courage, 2017; Robison and Unsworth, 2017; Brydges et al., 2018; Hanson et al., 2021). The present study assessed several cognitive traits in military personnel, including attention, mental flexibility, response inhibition, risk taking, working memory, mental rotation, and spatial perspective switching.
1.1.4 Demographics and lifestyle
Demographics & Lifestyle related features are also highly heterogeneous across individuals; many are relatively static over time (e.g., handedness) whereas others change over the course of months or years (e.g., video game experience, exercise frequency). For example, military personnel vary in age, gender, handedness, race and ethnicity, education, and military experience and occupational specialization. They also vary in lifestyle choices such as whether they exercise, consume tobacco or alcohol, play video games, read, use social media, and engage in risky behavior. Both demographics and lifestyle choices have been previously linked to cognitive and physical performance. For example, information processing speed generally declines with age, inconsistent handedness predicts memory performance and cognitive flexibility, regular aerobic exercise increases executive function, chronic tobacco and alcohol use are associated with cognitive decrements, long-term dietary preferences and habits of military personnel are associated with physical performance during training, and video game experience predicts performance on military change detection, combat identification, and unmanned aerial vehicle control tasks (Hertzog, 1991; Ceballos, 2006; Durlach et al., 2009; Guiney and Machado, 2013; Prichard et al., 2013; Keebler et al., 2014; Lin et al., 2015). To capture some of these features, the present study administered questionnaires to assess a wide range of demographic and lifestyle variables.
1.1.5 Health-related traits
Health-related traits generally include health-related behaviors such as sleep patterns and nutrition and eating, and health-related physiological status such as gut microbial community, and blood-based nutrient levels. Health-related traits, while invariant relative to acute health states, are likely to change over time through behavior or exogenous influences; for example, sleep habits and patterns of injury tend to shift during military training. In general, health-related traits have been related to cognitive and physical performance, with links to cognitive and physical performance and academic success in both civilians and military personnel (Reilly and Edwards, 2007; Valladares et al., 2016; Sellaro and Colzato, 2017; Spencer et al., 2017; Grandou et al., 2019; Craven et al., 2022; Petrofsky et al., 2022). The present study included blood draws, vision and hearing tests, and several questionnaires to probe enduring patterns of injury, sleep, and nutrition.
1.2 Human performance
To quantify performance, the present research focuses on basic warrior skills outlined in the Soldier’s Manual of Common Tasks (Department of the Army, 2009). The manual emphasizes several core competencies related to moving, shooting, communicating, navigating, and sustaining performance. In the present analyses, these competency domains were leveraged as a priori constructs to categorize measures that were collected across several study-related events (to be detailed in the Method section).
In military contexts and other high-stakes domains, the ability to sustain performance under conditions of mental and physical stress is critical to success. In many cases, including Special Operations Aviation Unit (SOAR) training, Ranger School, and Survive, Evade, Resist, and Escape (SERE) School training, military personnel are exposed to extreme and enduring stressors that affect training and are intentionally designed to mimic the realities of military operations (Burke and Dyer, 1984; Lieberman et al., 2005, 2016; Nindl et al., 2018; Vartanian et al., 2018). Acute stress exposures trigger a cascade of neuroendocrine and immune responses that begin with sympathetic-adrenal-medullary (SAM) responses and the release of catecholamines, and continue with activation of the hypothalamic–pituitary–adrenal (HPA) axis and the release of glucocorticoids (Christensen, 1991; Sapolsky et al., 2000; Vedhara et al., 2000; Schommer et al., 2003; Smith and Vale, 2006; Lee et al., 2012; Gagnon and Wagner, 2016; Cain and Cidlowski, 2017). Together, these stress systems produce diverse central and peripheral nervous system effects and modulate the musculoskeletal, cardiovascular, gastrointestinal, and immune systems. While mild to moderate stressors are often considered adaptive over the short term, intense and prolonged stress exposures are generally detrimental to performance. For example, exposure to intense acute stressors is associated with diminished cognitive and physical functions including decision-making, executive function, working memory, and visuomotor control and coordination (Charmandari et al., 2005; Schwabe et al., 2012; Starcke and Brand, 2012; Morey et al., 2015; Gagnon and Wagner, 2016; Shields et al., 2016; Martin et al., 2019).
In the present study, Soldiers completed a comprehensive baselining activity to quantify their traits; they then participated in one or more study-related events that were designed to induce acute stress in laboratory and field environments. For example, the study-related events induced stress through threat of shock, live-fire (i.e., using live ammunition) exercises, time pressure, load carriage, and team-level fast-paced movements through complex terrain while assaulting enemy positions. Importantly, each study event also embedded performance measures to quantify both cognitive and physical performance; broadly, these measures can be categorized into the military-relevant domains of move, shoot, communicate, navigate, and sustain.
1.2.1 Move
A critical element of survival, Soldiers must be able to move effectively as a member of a team, under direct fire, and over, through, or around obstacles. Effective movement allows individuals and teams to avoid enemy targeting, leverage cover and concealment options, maintain visibility of enemy locations, and assume effective firing positions (Department of the Army, 2009). In the present study, we identified four measures of movement effectiveness related to performance on an anaerobic sprint test, aerobic capacity test, dynamic marksmanship test, and tactical movement in small unit formations.
1.2.2 Shoot
A critical element of infantry performance is the ability to effectively maintain and employ a weapon, most commonly the M16 rifle or M4 carbine. Effective shooting involves zeroing a weapon and engaging stationary or moving targets at varied ranges, and meeting or exceeding the standard of hitting 60% or more of targets within an assigned sector (Department of the Army, 2009). In the present study, we identified 12 measures of shooting performance, considering both fundamental (e.g., weapon stabilization, engagement close to center of mass) and relatively operational (e.g., effectively deciding to shoot or not shoot, prioritizing targets) measures from study events involving virtual reality, simulated marksmanship, and field live-fire shooting events.
1.2.3 Communicate
Performing voice communications with or without the assistance of a radio is an important element of team coordinated performance. Effective communication relies upon both language production and comprehension, and the ability to convey simple or complex concepts while following established standards (e.g., prowords, call signs, answering sequences) and using correct pronunciation and grammar (Department of the Army, 2009). In the present study, we identified three measures of communication performance related to speech accuracy and the exchange of information within small units (i.e., fire team and squad).
1.2.4 Navigate
While small-scale navigation is reliant upon individual and team mobility, for example between cover and concealment options, large-scale navigation is also reliant upon spatial awareness of location and orientation of the self, relative to proximal and distal objectives. Effective navigation allows individuals and teams to move with efficiency and effectiveness between proximal and distal objectives while relying on perception, memory, and supporting devices (e.g., compass, map) (Department of the Army, 2009). In the present study, we identified a navigation-related measure that assesses the ability to orient oneself toward distal (and imperceptible) waypoints in large-scale space using memory and a compass.
1.2.5 Sustain
A critical element of Soldier performance is the ability to effectively balance task-related demands and sustain physiological readiness throughout the duration of an exercise. Most related to the survive warrior skill (Department of the Army, 2009), effectively sustaining readiness over extended scenarios affords continuous movement, effective decision making and marksmanship, communication, and navigation through complex terrain. To quantify the ability to sustain physiological and neuromuscular readiness throughout the duration of an event, we identified eight performance measures examining the ability to maintain relatively low heart rates (relative to heart rate at VO2-max or age-predicted maximum heart rate) during challenging cognitive and physical work, maintain movement efficiency over the course of repeated bouts of physical exertion, and exert high levels of isometric torque on a dynamometer following strenuous physical work.
1.3 The present study
The present study examined whether traits of soldiers, including physical, social–emotional, cognitive, demographic/lifestyle, and health-related features, would predict performance measures across the domains of move, shoot, communicate, navigate, and sustain. A predictive model was built with 127 trait-level variables which were used as regressors to predict performance in military personnel. Note that we did not apply dimensionality reduction to these variables in order to retain traceability to the original assessment tools and their respective measures; indeed, one intent of this research was to precisely identify a reduced subset of assessments and measures that can be administered in the future to reliably predict performance. Dimensionality reduction of predictor variables, including principal components analysis, would not afford such traceability as predictors would be abstracted away from the original assessments.
To quantify performance, we used a breadth-first approach that aggregated data across a series of study events that took place in laboratory and field contexts, and with individuals and small teams. For each individual participant, we derived a total of five scores, one for each a priori performance domain: move, shoot, communicate, navigate, and sustain. These scores were used as outcomes in each of five models. This modeling approach allowed us to identify critical subsets of trait-level predictors (i.e., quantitative measures derived from trait assessments) that account for performance variation in each of the five domains while increasing probable generalizability of our findings across contexts and tasks.
2 Methods
2.1 Participants
A total of 387 enlisted U.S. Army soldiers (315 male, 4 female) participated voluntarily in a baselining event (when traits were measured) and then at least one of several study events. Military occupational specialties were predominantly infantry with the addition of some combat engineers and cavalry scouts. Demographic details of the sample are included in Table 2.
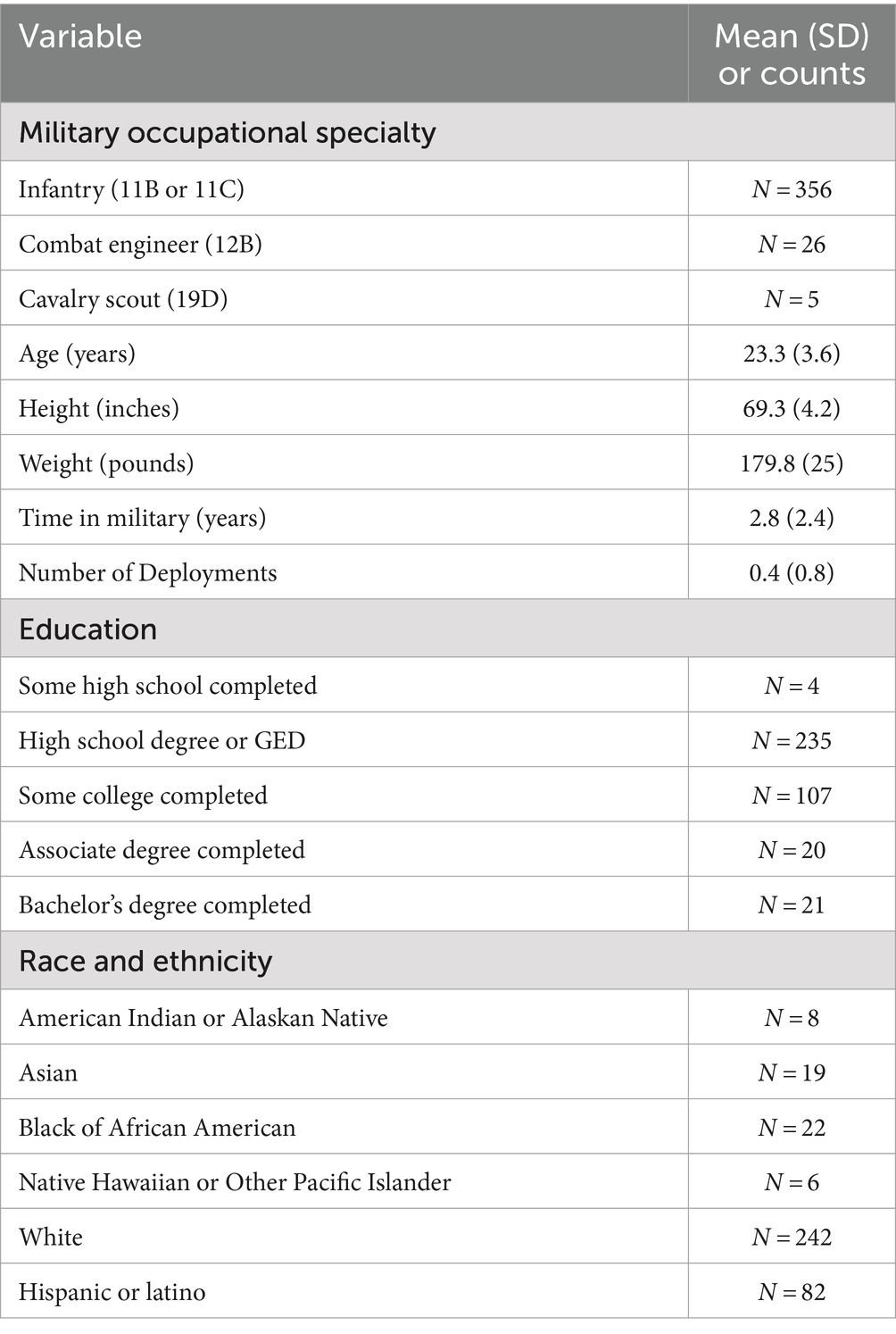
Table 2. Demographic details of the participant sample, including mean (and standard deviation) or participant counts.
2.2 Study events and performance measures
Data were collected across multiple study events: one involving the collection of 127 trait-related variables (hereafter referred to as features; detailed in Table 3), and six involving the collection of performance-related variables (hereafter referred to as outcomes).
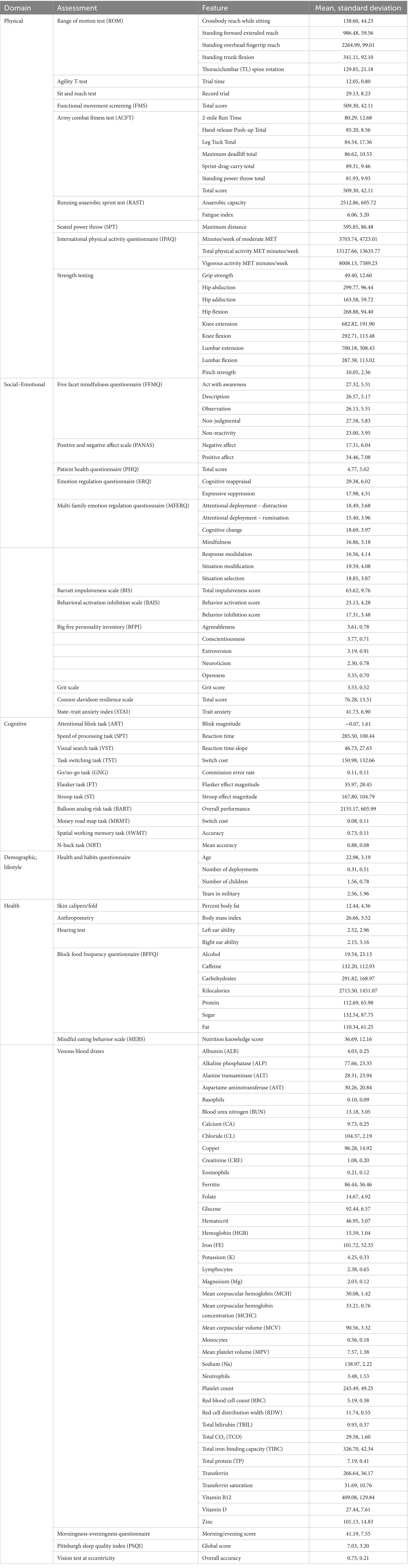
Table 3. The baseline assessments and features included in the analyses, including their domain, assessment used, features, and descriptive statistics.
For the collection of trait-related features, all participants completed a series of questionnaires, cognitive and physical tasks, and venous blood draws over the course of a three-to-five-day period (hereafter referred to as baselining). Note that baselining was not performed under conditions of stress, and took place on a rolling and as-needed basis (i.e., when needed for study events, between dates 2/21–8/23) and prior to participation in any other study events. The time between baselining and study event participation was within 4–6 weeks. Full methodological details of the baselining events can be found in (Giles et al., 2023); note that the present sample size exceeds that of this prior study, which was preliminary in nature.
Following baselining, participants completed at least one of the study events detailed in Table 4; there were a total of 50 performance outcomes derived from these events, distributed across the five a priori constructs (move, shoot, communicate, navigate, sustain).
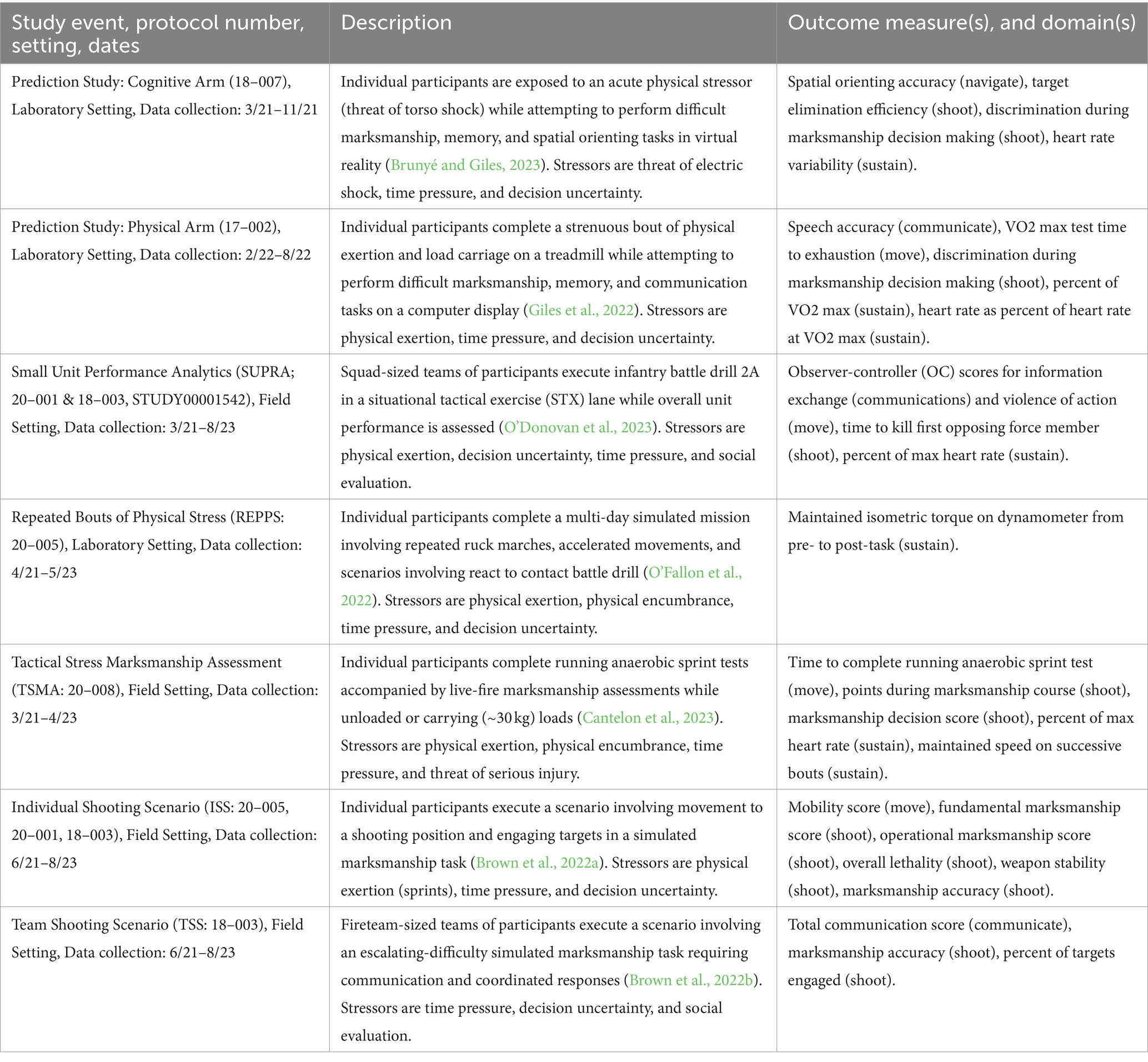
Table 4. The seven study events included in our analyses, along with their respective protocol numbers, data collection settings (laboratory versus field), task descriptions, and outcome measures and domain.
The baselining and study events received ethical approvals through the institutional review board(s) (IRB) at the United States Army DEVCOM Armaments Center, Walter Reed Army Institute of Research (WRAIR) IRB, and/or Tufts University (IRB protocol numbers included in Table 4).
2.3 Data processing
Baseline data were processed and summarized as previously outlined (Giles et al., 2023), resulting in a total of 127 trait-level features for use in our analyses. These features are listed in Table 3, and were standardized prior to inclusion in analyses. As detailed in Table 4, a total of 28 performance outcomes were available for analysis from the study events; each was categorized into a single performance outcome domain (move, shoot, communicate, navigate, sustain) based on the results of consensus meetings among authors.
Because all participants were involved in the baselining event but then variably involved in the subsequent study events, a method was needed to normalize and aggregate data across study events and their respective performance outcomes. To do so, each performance outcome was standardized to result in scaled data with a mean of 0 and standard deviation of 1. The additive inverse of the standardized score was used for any outcomes with higher values indicating poorer performance (for example, distance from center of mass or time to completion). In this manner, higher standardized scores within each measure consistently indicated higher performance. Then, within each a priori performance domain, we computed a mean standardized outcome score for each participant by averaging across each domain’s standardized outcome with each being equally weighted. The result of this process was a single outcome for each participant and performance domain, affording a regression-based analysis.
2.4 Data analysis
Data were analyzed in two phases: evaluation/elimination of features based on regression diagnostics and inter-rater-reliable priors, followed by feature-selection and model fitting using elastic net regularization techniques (Zou and Hastie, 2005). Note that because participants were variably involved in study events, each of the five composite performance outcomes had a different number of participants included in analysis (see Table 5).For regression diagnostics, the features were evaluated for collinearity in addition to assessing their individual associations with each of the five outcomes (Meuleman et al., 2014; Fox, 2019). Features that were correlated with other features above a threshold (≥ 0.80), that also produced variance inflation factors (VIFs) over 5 when included in the same model, were removed from the outcome subset (Belsley et al., 1980; Chatterjee and Simonoff, 2013; Dunn and Smyth, 2018). Additional features were also removed for lacking meaningful associations with the outcome if also deemed by priors as “unlikely” or “very unlikely” to be feature-selected for that specific outcome. Priors were based on ratings from a panel of five subject matter experts (cognitive, biomedical, and biomechanical science) who were asked to characterize the relationship between each feature and outcome. The subjective ratings were based on the relevance of each feature in predicting each of the five outcomes (move, shoot, communicate, navigate, sustain), and responses were provided using a five-point Likert scale ranging from Very Unlikely (1) to Very Likely (5). After elimination, the remaining features were distributed across the five outcomes as detailed in Table 5. Overall, the combination of regression diagnostics and input from specified priors allowed us to eliminate features from each model and facilitate a more parsimonious solution.
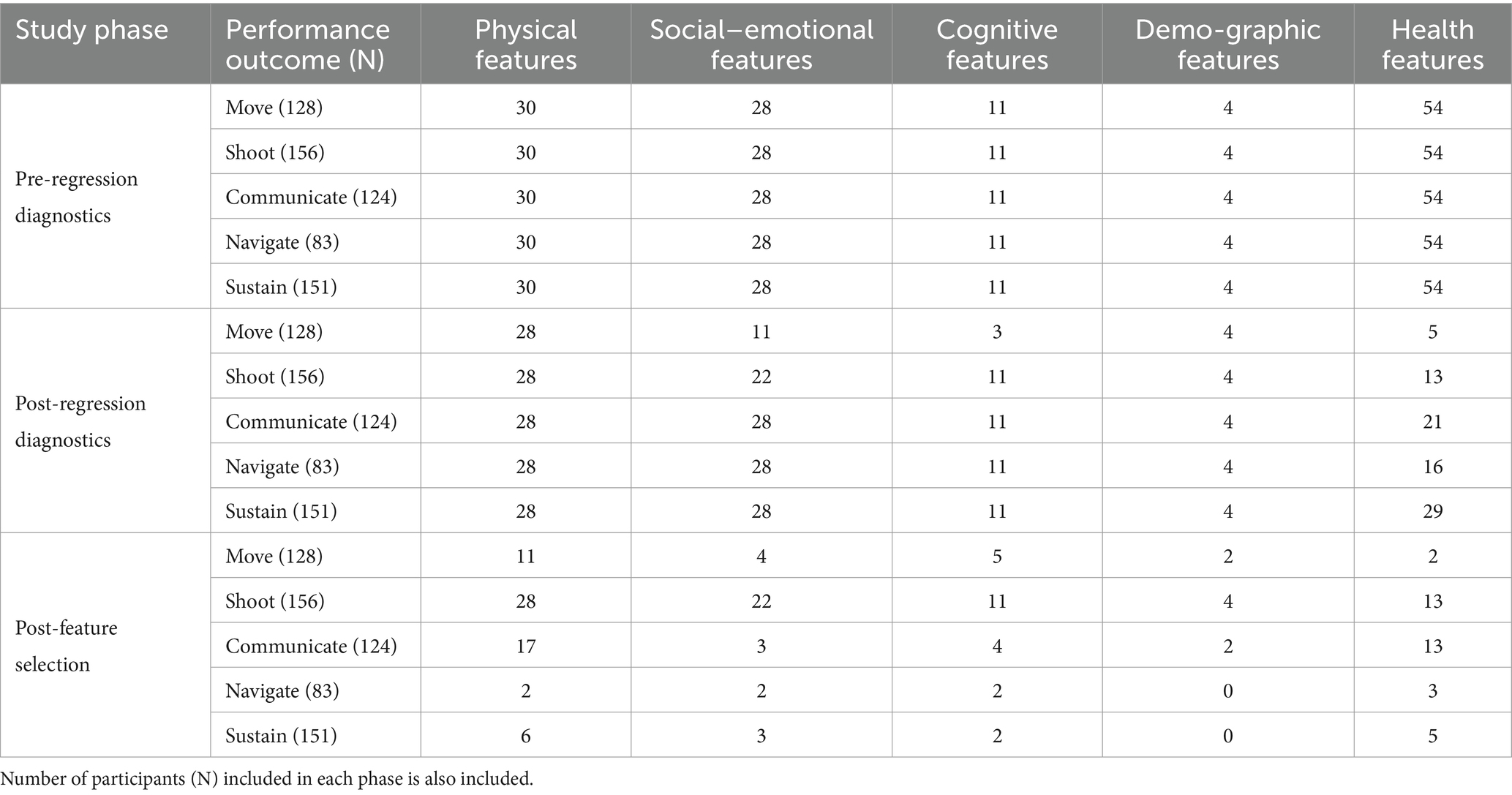
Table 5. The number of features (by feature category: physical, social–emotional, cognitive, demographic, health) included in each of the five models, at each of the phases (pre-regression diagnostics, post-regression diagnostics, post-feature selection).
Due to the large number of features remaining after the previous step and the associated risk of overfitting, elastic net regression was employed as a model regularization technique and feature selection method (Zou and Hastie, 2005). Elastic net is a machine-learning based algorithm that combines the L1 and L2 penalty features of lasso and ridge regression regularization methods to favor more parsimonious model solutions. The elastic net regression fitting method is particularly useful for instances in which there are many features relative to the number of participants (sample size), and for models containing groups of correlated variables (Friedman et al., 2010). These issues were relevant to the current data, despite removal of some highly correlated features in the preliminary diagnostics stage. Additionally, elastic net techniques offer a combination of feature selection and coefficient shrinkage to identify the most relevant features and reduce the other feature coefficients toward zero. Feature selection on its own would risk an imbalance of variance in the models, leading to an overly complex, sample-specific set of results. By also introducing some bias to the model via shrinkage, it is more consistent with the goal of establishing a subset of features that are generalizable to the testing of future samples.
Analyses were conducted using R Statistical Software (v2023.06.1 + 524; R Core Team) with the caret package (v6.0–94) (Kuhn, 2008). Five linear regression models were fitted, one for each outcome, using elastic net regularization methods optimized by 10-fold cross-validation. A 100-item parameter grid was used to find the ideal model combination for a range of mixing parameter values (α, 0–1) and tuning parameter values (λ, 0–1) to determine the best fit to each outcome subset. The final models were selected based on the parameter combination that minimized the root-mean-squared-error (RMSE), while maximizing the proportion of variance explained by the model (R2). The regression coefficients generated from the final models are reported in Table 6, and model parameters and fit indices are detailed in the respective results sections for each composite outcome.

Table 6. Results of the elastic net regression process including results for each composite outcome, the selected features, and the feature-specific regression coefficients.
3 Results
Table 5 details the number of features remaining in each model after the feature selection process.
3.1 Move outcome
The final model (α = 0.10, λ = 0.46; RMSE = 0.68, R2 = 0.16) selected 24 of the 54 features, accounting for 16% of the variance in the move outcome. The regression coefficients generated from the model are reported in Table 6; 11 of the features were related to physical traits (ACFT measures, agility, flexibility, strength, activity levels), 5 were related to cognitive traits (inhibitory control, useful field of view, visual search ability), 2 were related to health traits (alcohol consumption, blood magnesium), 4 were related to social/emotional traits (emotion regulation, resilience), and 2 were related to lifestyle traits (number of deployments, years in military).
3.2 Shoot outcome
The final model (α = 0.60, λ = 0.00; RMSE = 0.96, R2 = 0.15) selected all 78 features, accounting for 15% of the variance in the shoot outcome. The regression coefficients generated from the model are reported in Table 6; 28 of the features were related to physical traits (ACFT measures, agility, flexibility, strength, speed, endurance, activity levels), 11 were related to cognitive traits (attention, inhibitory control, working memory, risk taking, spatial perspective taking, speed of processing, useful field of view, visual search ability), 13 were related to health traits (blood analytes, consumption patterns, hearing ability), 22 were related to social/emotional traits (impulsiveness, behavioral activation and inhibition, emotion regulation, grit, mindfulness, resilience), and 4 were related to lifestyle traits (age, number of children, number of deployments, years in military).
3.3 Communicate outcome
The final model (α = 0.01, λ = 1.00; RMSE = 0.99, R2 = 0.25) selected 39 of the 92 features, accounting for 25% of the variance in the communicate outcome. The regression coefficients generated from the model are reported in Table 6; 17 of the features were related to physical traits (ACFT measures, agility, flexibility, endurance, activity levels), 4 were related to cognitive traits (attention, inhibitory control, working memory, spatial perspective taking), 13 were related to health traits (blood analytes, nutrition knowledge, percent body fat, hearing ability), 3 were related to social/emotional traits (conscientiousness, emotion regulation, expressive suppression), and 2 were related to lifestyle traits (number of deployments, years in military).
3.4 Navigate outcome
The final model (α = 0.90, λ = 0.18; RMSE = 0.85, R2 = 0.39) selected 9 of the 88 features, accounting for 39% of the variance in the navigation outcome. The regression coefficients generated from the model are reported in Table 6; 2 of the features were related to physical traits (flexibility), 2 were related to cognitive traits (spatial working memory, spatial perspective taking), 3 were related to health traits (blood zinc, nutrition knowledge, caffeine consumption), 2 were related to social/emotional traits (grit, mindfulness), and 0 were related to lifestyle traits.
3.5 Sustain outcome
The final model (α = 0.10, λ = 0.91; RMSE = 0.81, R2 = 0.12) selected 16 of the 101 features, accounting for 12% of the variance in the sustain outcome. The regression coefficients generated from the model are reported in Table 5; 6 of the features were related to physical traits (ACFT leg tuck, endurance, strength, flexibility), 2 were related to cognitive traits (attention, inhibitory control), 5 were related to health traits (blood analytes), 3 were related to social/emotional traits (emotion regulation, mindfulness, neuroticism), and 0 were related to lifestyle traits.
3.6 Outcome summary
Across the five outcomes, no single feature was selected in all five models. The most frequently selected features that were selected in at least 2 of the 5 models are included in Table 7. Overall, across models the most frequently selected features were physical (30 features), followed by Social/Emotional (29 features), Health (28 features), Cognitive (13 features), and Lifestyle (5 features). Interestingly, while none of the same features were selected across all five models, in general trait-level measures of grit, emotion regulation, resilience, and/or mindfulness held predictive value across many of the outcomes.
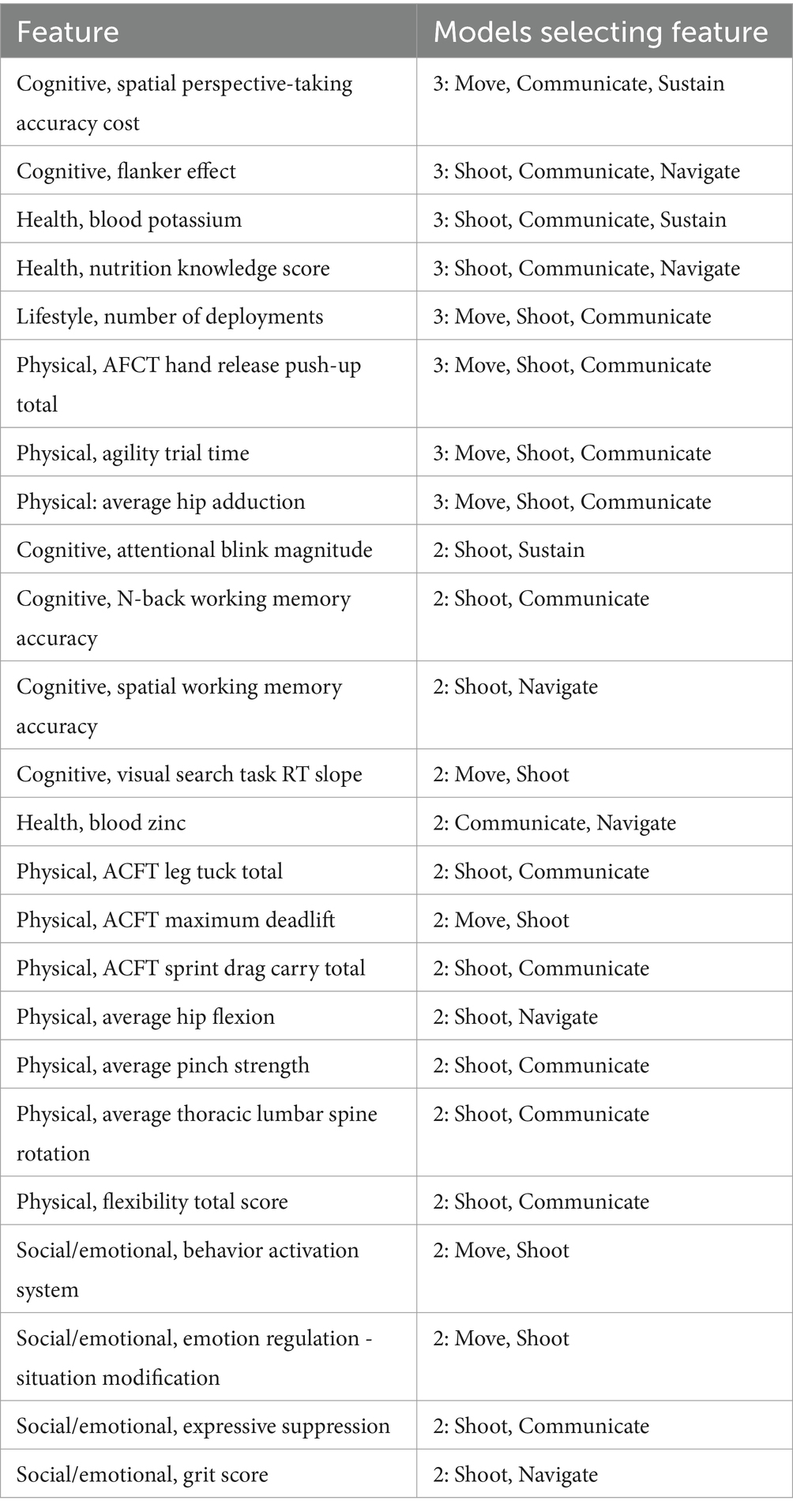
Table 7. The most frequently selected features across the five models, including the feature name and the models selecting the feature.
It is also important to point out that model error (as assessed via RMSE) was moderately high across all five models, suggesting that the model’s predictions are, on average, different from the actual observed values, indicating poor model accuracy. This often implies that the model is not capturing the underlying patterns in the data effectively, suggesting that different features (e.g., states) may prove valuable for complementing trait-based features.
4 Discussion
In this study, we investigated whether soldiers’ trait-level traits are associated with cognitive and physical performance outcomes across a range of laboratory and field tasks. Results indicated that traits measured across physical, social–emotional, cognitive, demographic/lifestyle, and health-related domains collectively contribute to explaining low-to-moderate variance in performance outcomes related to several domains critical for military occupational functioning: move, shoot, communicate, navigate, and sustain. The varied nature of features identified in each performance outcome underscores the multifaceted nature of human performance and highlights the importance of considering a broad array of individual traits when assessing, predicting, and optimizing performance.
When predicting the move domain, the model selected 24 diverse features but was able to only account for 16% of variance in the outcome, showing moderate model error (as indicated by RMSE values). Among the physical features, we found that several baseline measures of strength (e.g., maximum deadlift) and flexibility (e.g., maximum flexibility) were positively associated with performance outcomes; similarly, agility trial time (i.e., the time to complete the agility trial) was negatively associated with the move outcome. However, we also found a measure of power (i.e., ACFT hand release push-up total) was (unexpectedly) negatively associated with the move outcome. It is not entirely clear why this association arose; perhaps because move outcomes were derived primarily from tests of lower body movement (e.g., sprint tests, treadmill tests), upper body power is unrelated to, or even counterproductive to, their execution. That said, our findings generally align with previous research highlighting the importance of physical conditioning and readiness in military contexts, with an emphasis on strength, flexibility, and agility (Heinrich et al., 2012; Kraemer and Szivak, 2012; Lester et al., 2014; Smith et al., 2022).
When predicting the shoot domain, the model selected all available features but was only able to account for 15% of variance in the outcome, showing relatively high model error. Most prevalent among selected variables were physical traits, indicating the importance of power and endurance, speed, strength, agility, and flexibility. The strongest physical predictor was related to the amount of time it took to complete an agility trial, with those completing it faster also showing higher shoot outcomes; given that many of the shooting tasks examined herein involved acute bouts of physical exertion between shooting episodes (e.g., running between supported firing positions), this result is intuitive but also points to the importance of whole-body coordinated activity for attaining high marksmanship performance. Among the cognitive traits, the strongest features were related spatial cognitive processes, specifically the ability to temporarily maintain spatial information in working memory and transform spatial perspectives. The visuo-motor coordination involved in aiming a weapon and acquiring targets is inherently spatial, involving dynamic coordination of the body, head and eyes with the weapon’s intrinsic axes and variably sized and positioned external targets (Chung et al., 2004; Dudde et al., 2012; Palmer and Van Emmerik, 2020; Brown et al., 2022b). The ability to process, manipulate, and transform spatial information appears to critically underpin effective shooting performance, a possibility worthy of future investigation. This is particularly intriguing given the relative trainability of spatial skills and their apparently successful transfer to untrained tasks (Uttal et al., 2013). We also found that baseline performance on cognitive tasks examining the ability to effortfully control attention and switch between tasks was associated with shoot performance, supporting prior work demonstrating the role of executive functions in shoot/do not-shoot decisions (Biggs et al., 2015; Biggs, 2021; Biggs and Pettijohn, 2022). Among the health-related traits, blood levels of potassium were most strongly and negatively associated with shoot performance. High blood potassium levels (hyperkalemia) are associated with muscle weakness and fatigue (Makuch et al., 2019), which could play a role in the ability to effectively manage a weapon during strenuous physical activities. Finally, among lifestyle features, number of children emerged as strongly and positively related to shoot performance. While we are unaware of any previous research demonstrating this association, some studies suggest that parents evaluate themselves and their lives more positively than those without children, and they experience more positive emotions, gratification, and feelings of meaning and contribution (Umberson and Gove, 1989; White and Dolan, 2009; Nelson et al., 2013). Parents with more children might be especially adept at regulating their own emotions and those of others (Rutherford et al., 2015; Grolleman et al., 2023), and this may influence performance during stressful shooting events. Continuing research will explore these possible relationships and how they may moderate effects of parenthood on shooting performance.
When predicting the communicate domain, the model selected a high number of features (39) to account for a low-to-moderate amount of variance (25%) but with relatively high model error. The strongest features were related to blood analytes, working memory, conscientiousness, and number of deployments. For health-related blood analytes, we found that lower levels of basophils, potassium, hemoglobin, and sodium were related to higher communication performance. Basophils generally have an anti-inflammatory effect via histamine content, and blood potassium, sodium, and hemoglobin have been variably associated with cognitive function in clinical or aging populations (Jáuregui-Lobera, 2014; Cisternas et al., 2015; Kung et al., 2021; Gomes et al., 2023; Harner and Root, 2023; Suárez et al., 2024). Given the novelty of these health-related features for predicting communications outcomes, replication and extension will be important. In the cognitive domain, lower working memory accuracy on the n-back task was associated with higher communication outcomes. Some theories of working memory suggest that performance on working memory tasks is critically dependent upon executive function (Engle, 2002; Engle and Kane, 2003; McCabe et al., 2010); it could be the case that those with lower working memory capacity show lower inhibition levels during communication tasks, making them more likely to produce language that ultimately helps support team-oriented tasks.
When predicting the navigate domain, the model selected very few features but accounted for moderate amounts of variance (39%) with moderate model error. Selected features were predominantly cognitive in nature. The high variance accounted for in the model is likely because only a single performance measure was used to calculate the navigate outcome, derived from a single study; this may decrease the inter-measure variance associated with the composite performance outcome and facilitate prediction. However, it likely also reduces the generalizability of our results within this domain. Within the cognitive domain, working memory and perspective-taking were both positively associated with higher navigation performance. Specifically, higher spatial working memory scores and lower cost associated with switching perspectives on a spatial task, were both positively associated with this outcome. Visuo-spatial working memory is considered a fundamental process in successful performance on spatial cognition tasks including localization, orientation, and navigation (Garden et al., 2002; Baumann et al., 2011; Meneghetti et al., 2016, 2021), as are the attentional control and executive function skills required to transform spatial perspectives (Korthauer et al., 2017).
When predicting the sustain domain, the model selected only 16 features and only accounted for 12% of variance in the outcome, with moderate model error. The strongest association was a positive relationship between blood potassium and the ability to sustain speed or strength during acute bouts of physical exertion. Potassium plays a crucial role in various physiological processes, including muscle function, fluid balance, nerve transmission, and energy metabolism (Nielsen et al., 1986; Maughan et al., 1997; Sejersted and Sjøgaard, 2000; Demigné et al., 2004). Given the essential contribution of potassium to the proper functioning of muscle cells, low potassium levels, a condition known as hypokalemia, are associated with muscle weakness and fatigue. Alongside sodium, potassium also helps regulate fluid balance within cells and throughout the body, and maintaining adequate potassium levels can help prevent dehydration. Potassium is also involved in nerve transmission (e.g., between the brain and muscles) that supports coordinated movement and muscle contractions during exercise. Finally, potassium also plays a role in the conversion of carbohydrates into energy. It could be the case that those with higher basal levels of blood potassium may be better equipped to sustain adequate potassium levels to support exercise performance. Continuing research will be critical to assess the reliability and scope of such an effect, and identify its precise physiological mechanisms.
Interestingly, while no single feature was selected consistently across all five composite performance outcomes, there was some suggestion that features related to resilience were generally important for predicting several outcomes. For example, individual differences in emotion regulation were important for predicting move, shoot, communicate, and sustain. Individual differences in measures of resilience were important for predicting move and shoot outcomes, grit was important for predicting shoot and navigate outcomes, and mindfulness was important for predicting shoot, navigate, and sustain outcomes. Emotion regulation refers to the processes involved in managing and adaptively responding to emotionally salient experiences; when individuals with relatively high emotion regulation skills encounter adversity, they are better able to handle the adversity (maintain composure, reframe experiences, recover from setbacks) without becoming overwhelmed, a key protective factor in overall resilience (Troy and Mauss, 2011; Gross, 2013, 2015). Grit refers to a passion for and perseverance toward goals, which involves sustaining interest and effort in the face of challenges; those with higher grit are more likely to persist through challenges and continually striving toward goals, a core aspect of resilience (Duckworth et al., 2007; Caza et al., 2020; Datu, 2021). Mindfulness is also related to resilience because it allows individuals to maintain a balanced perspective in the face of stress, restrain from immediate reactions, and maintain a calm and composed mindset (Pidgeon and Keye, 2014). Indeed some research suggests that mindfulness-based stress reduction (MBSR) training improves resilience via increases in several aspects of mindfulness (e.g., decentering, acceptance) (Nila et al., 2016). The fact that numerous traits related to resilience were predictive of performance outcomes is compelling, suggesting that broad resilience-related traits are critical for sustaining real-world performance.
4.1 Implications for performance prediction, assessment, and training
Our findings have several implications for personnel selection, training, and operational practices. By identifying key trait-level predictors of performance outcomes, military organizations can tailor selection criteria, training programs, and support interventions to optimize individual and team performance. For instance, integrating targeted physical conditioning, cognitive training, and stress resilience programs into training curricula may enhance overall readiness and effectiveness in diverse operational contexts. Moreover, leveraging predictive models derived from measured traits can inform personnel assignment, task allocation, and performance optimization strategies, thereby enhancing mission success and personnel well-being.
A primary goal of this research was to identify a subset of traits that could be easily assessed in laboratory and field environments but prove helpful for predicting diverse performance outcomes relevant to military training and operations. We began with a set of 127 features that were measured by administering 40 diverse trait assessments (i.e., questionnaires and cognitive and physical tasks) and a venous blood draw to participants over the course of a multi-day baselining event. Through the modeling process, we were able to identify the most critical set of trait assessments to administer when attempting to predict each outcome. Specifically, to predict move there are only 16 assessments to administer, shoot has 34 assessments, communicate has 19 assessments, navigate has 9 assessments, and sustain has 10 assessments. In other words, we were able to reduce the number of assessments to be administered by 18–80% depending upon which outcome is to be predicted. For example, to predict the navigate outcome, scientists and practitioners can account for 39% of variance in this outcome by only administering the following 9 assessments: Block Food Frequency Questionnaire, Five Facet Mindfulness Questionnaire, Grit Scale, Hearing Tests, Military Eating Behavior Survey, Money Road Map Task, Spatial Working Memory Test, Strength Tests, and Venipuncture for Zinc. In this case, rather than requiring a multi-day baselining event to administer 41 assessments, this reduced baselining could likely take place over the course of 3–4 h. Other outcomes are more difficult to predict and require administering more assessments while accounting for relatively low outcome variance.
4.2 Limitations and future directions
Despite the valuable insights gained from this study, several limitations warrant consideration. First, the sample predominantly consisted of white male soldiers, limiting the generalizability of findings to relatively diverse military populations. Future research should aim to include more diverse samples to capture the full spectrum of trait-performance relationships across different demographic groups and military occupational specialties. Additionally, the study focused on trait-level variables and did not account for situational, contextual, or genetic factors that may influence performance outcomes. Future studies should explore the interactive effects of these variables on performance to provide a more comprehensive understanding of human performance dynamics in military settings; it is likely that the inclusion of more comprehensive characterizations of individuals will increase the variance accounted for in performance outcomes. It may also help reduce the relatively high error (measured as RMSE) in our models, which indicate that the models’ predictions are, on average, different from the actual observed values, suggesting relatively low model accuracy. Some of this is related to the inherent bias-variance trade-off, and the risk of producing an overly complex model due to the large number of features relative to observations. The regularization techniques used to avoid this outcome increase the bias of the model to produce a more generalizable result. However, this does decrease the sensitivity and predictive power to capture patterns in the current data. Some analytic approaches, including ensemble methods and decision trees, might assist in reducing model error and increase precision, but they may be less appropriate for high dimensional data due to the risk of overfitting.
Furthermore, some traits are more invariant than others; indeed, some traits will change through young adulthood (e.g., age, number of children) and some will remain relatively constant (e.g., personality, working memory capacity, range of motion). Moreover, some measured traits are modifiable by the self or through intervention. Thus, while we consider traits relatively invariant characteristics of individuals, they are not entirely static and can be expected to change over time necessitating recurring measurement (perhaps annually or biannually). While we made all attempts to execute study events within a few weeks of baselining to reduce variance in trait measures, this could indeed be a source of variance in our results. Indeed, more timely baselining (e.g., same day or week) and the measurement of ongoing states that influence performance [e.g., sleep, stress, hydration; (Brunyé et al., 2021)] will likely reduce model error and increase the proportion of outcome variance accounted for in our models.
It is worth noting that many feature selection processes are generally agnostic to the directionality of relationships between features and outcomes; indeed, most machine learning and artificial intelligence feature selection approaches (e.g., random forest, neural networks) provide metrics related to feature importance (which can be based on many different factors), but not the directionality of relationships between features and outcomes. At an extreme, the precise nature of these relationships is completely obscured by some feature selection and classification approaches (i.e., the black box phenomenon) (Oh et al., 2019; Liang et al., 2021). We explored the use the elastic net regression to increase transparency, quantify the variance accounted for by selected features, and reveal some insights into the strength and directionality of relationships between features and outcomes. However, apparent counterintuitive results may not hold practical value for understanding mechanistic relationships between features and outcomes; rather, they should motivate continuing research. Furthermore, while many of the identified features had small but non-zero coefficients characterizing their relationships to performance outcomes, a combination of many features with small effects can collectively have a substantial impact. It is also worth noting that due to the regularization techniques used to reduce model complexity, many feature coefficients have been shrunken toward zero. Although this does help to increase the generalizability of the model, it also means the true relationships between the feature and outcome may not be fully captured by the model. Overall, it is still meaningful when a feature is selected as a relevant predictor. The overall predictive power of the model might rely on the combined contributions of many such small-effect features; also, the regularization technique used herein ensured that these features contribute optimally without making the model overly complex or sensitive to noise.
5 Conclusion
In conclusion, predicting human performance outcomes is a challenging endeavor, and even highly comprehensive baseline assessment batteries only appear to account for low-to-moderate amounts of variance in applied performance outcomes. Complementing these relatively invariant predictors with an understanding of ongoing personnel states, such as through wearable biosensing, will likely prove valuable for performance prediction. Moving forward, interdisciplinary research efforts integrating psychological, physiological, and operational perspectives will be instrumental in advancing our understanding of human performance optimization in dynamic and challenging environments.
Data availability statement
The data analyzed in this study is subject to the following licenses/restrictions: Data were collected on a specialized and sensitive military population. Contact the corresponding author for data inquiries. Requests to access these datasets should be directed to Tad Brunye, dGhhZGRldXMudC5icnVueWUuY2l2QGFybXkubWls.
Ethics statement
The studies involving humans were approved by the US Army DEVCOM Armaments Center IRB, Walter Reed Army Institute of Research IRB, and/or Tufts University IRB. The studies were conducted in accordance with the local legislation and institutional requirements. The participants provided their written informed consent to participate in this study.
Author contributions
TB: Conceptualization, Data curation, Formal analysis, Funding acquisition, Methodology, Writing – original draft, Writing – review & editing. SG: Formal analysis, Writing – review & editing. JC: Conceptualization, Data curation, Methodology, Writing – review & editing. ME: Conceputalization, Data curation, Funding acquisition, Methodology, Writing – review & editing. SE-F: Conceptualization, Data curation, Methodology, Writing – review & editing. WE: Data curation, Writing – review & editing. GG: Conceptualization, Data curation, Methodology, Funding acquisition, Writing – review & editing. CH: Conceptualization, Data curation, Methodology, Writing – review & editing. SM: Formal analysis, Writing – review & editing. JM: Formal analysis, Writing – review & editing. KLM: Conceptualization, Data curation, Methodology, Funding acquisition, Writing – review & editing. KBM: Conceptualization, Data curation, Methodology, Writing – review & editing. MO’D: Conceptualization, Data curation, Methodology, Writing – review & editing. KR: Conceptualization, Data curation, Methodology, Writing – review & editing. JR: Conceptualization, Data curation, Methodology, Funding acquisition, Writing – review & editing.
Funding
The author(s) declare that financial support was received for the research, authorship, and/or publication of this article. Research reported herein was funded by the United States Army DEVCOM Soldier Center, Measuring and Advancing Soldier Tactical Readiness and Effectiveness (MASTR-E) program. The views expressed in this article are solely those of the authors and do not reflect the official policies or positions of the Department of Army, the Department of Defense, or any other department or agency of the United States government.
Acknowledgments
The authors would like to thank the United States Army Soldiers of the 2nd Brigade Combat Team, 101st Airborne Division (Air Assault); 2nd Battalion, 4th Infantry Regiment, 2nd Brigade Combat Team, 101st Airborne Division (Air Assault); 1st Battalion, 325th Airborne Infantry Regiment, 2nd Brigade Combat Team, 82nd Airborne Division; 2nd Brigade Combat Team, 101st Airborne Division (Air Assault); 8th Squadron, 1st Cavalry Regiment, 2nd Stryker Brigade Combat Team and 555th Engineer Brigade, 2nd Infantry Division; 91st Engineering Battalion, 1st Armored Brigade Combat Team, 1st Cavalry Division; 1st Battalion, 504th Airborne Infantry Regiment, 1st Brigade Combat Team, 82nd Airborne Division; 2nd Battalion, 27th Infantry Regiment, 3rd Brigade Combat Team, 25th Infantry Division; 2nd Battalion, 4th Infantry Regiment, 2nd & 3rd Brigade Combat Team, 10th Mountain Division.
Conflict of interest
The authors declare that the research was conducted in the absence of any commercial or financial relationships that could be construed as a potential conflict of interest.
The author(s) declared that they were an editorial board member of Frontiers, at the time of submission. This had no impact on the peer review process and the final decision.
Publisher’s note
All claims expressed in this article are solely those of the authors and do not necessarily represent those of their affiliated organizations, or those of the publisher, the editors and the reviewers. Any product that may be evaluated in this article, or claim that may be made by its manufacturer, is not guaranteed or endorsed by the publisher.
References
Ackerman, P. L. (1992). Predicting individual differences in complex skill acquisition: dynamics of ability determinants. J. Appl. Psychol. 77, 598–614. doi: 10.1037/0021-9010.77.5.598
Algoe, S. B., and Fredrickson, B. L. (2011). Emotional fitness and the movement of affective science from lab to field. Am. Psychol. 66, 35–42. doi: 10.1037/a0021720
Araújo, D., Davids, K., and Passos, P. (2007). Ecological validity, representative design, and correspondence between experimental task constraints and behavioral setting: comment on. Ecol. Psychol. 19, 69–78. doi: 10.1080/10407410709336951
Arra, K., Gunda, Y. R., and Gupta, S. (2023). Development of a predictive model for workers’ involvement in workplace accidents in an underground coal mine. Sādhanā 48:63. doi: 10.1007/s12046-023-02121-3
Bartone, P. T., Roland, R. R., Picano, J. J., and Williams, T. J. (2008). Psychological hardiness predicts success in US Army special forces candidates. Int. J. Sel. Assess. 16, 78–81. doi: 10.1111/j.1468-2389.2008.00412.x
Baumann, O., Skilleter, A. J., and Mattingley, J. B. (2011). Short-term memory maintenance of object locations during active navigation: which working memory subsystem is essential? PLoS One 6:e19707. doi: 10.1371/journal.pone.0019707
Belsky, D. W., Caspi, A., Israel, S., Blumenthal, J. A., Poulton, R., and Moffitt, T. E. (2015). Cardiorespiratory fitness and cognitive function in midlife: neuroprotection or neuroselection? Ann. Neurol. 77, 607–617. doi: 10.1002/ana.24356
Belsley, D. A., Kuh, E., and Welsch, R. E. (1980). Regression diagnostics: Identifying influential data and sources of collinearity. Hoboken, NJ: John Wiley and Sons.
Biggs, A. T. (2021). Developing scenarios that evoke shoot/don’t-shoot errors. Appl. Ergon. 94:103397. doi: 10.1016/j.apergo.2021.103397
Biggs, A. T., Cain, M. S., and Mitroff, S. R. (2015). Cognitive training can reduce civilian casualties in a simulated shooting environment. Psychol. Sci. 26, 1164–1176. doi: 10.1177/0956797615579274
Biggs, A. T., and Pettijohn, K. A. (2022). The role of inhibitory control in shoot/don’t-shoot decisions. Q. J. Exp. Psychol. 75, 536–549. doi: 10.1177/17470218211041923
Biggs, A. T., Seech, T. R., Johnston, S. L., and Russell, D. W. (2023). Psychological endurance: how grit, resilience, and related factors contribute to sustained effort despite adversity. J. Gen. Psychol. 151, 271–313. doi: 10.1080/00221309.2023.2253955
Bo, J., and Seidler, R. D. (2009). Visuospatial working memory capacity predicts the Organization of Acquired Explicit Motor Sequences. J. Neurophysiol. 101, 3116–3125. doi: 10.1152/jn.00006.2009
Boogert, N. J., Madden, J. R., Morand-Ferron, J., and Thornton, A. (2018). Measuring and understanding individual differences in cognition. Philos. Trans. R. Soc. B Biol. Sci. 373:20170280. doi: 10.1098/rstb.2017.0280
Brimley, S., Fitzgerald, B., Sayler, K., and Singer, P. W. (2013). Game changers: Disruptive technology and U.S. defense strategy. Washington, D.C.: Center for a New American Security.
Britt, T. W., Adler, A. B., and Castro, C. A. (2006). Military life: The psychology of serving in peace and combat. Westport, Connecticut: Greenwood Publishing Group.
Brown, S. A. T., Christopher, J. J., Villa, J. D., Goodenough, R. R., Hancock, C. L., O’Donovan, M., et al. (2022a). Methodology to assess individual shooting skills that is predictive of squad level performance in a close combat training engagement. In: Proceedings of the human factors and ergonomics society annual meeting, (Atlanta, GA), pp. 1487–1491.
Brown, S. A. T., Lam, P., Christopher, J. J., Goodenough, R. R., Villa, J. D., Bode, V. G., et al. (2022b). A novel methodology for evaluating military teamwork and team marksmanship performance. Digit. Hum. Model. Appl. Optim. 46, 53–62. doi: 10.54941/ahfe1001899
Brunyé, T. T., Brou, R., Doty, T. J., Gregory, F. D., Hussey, E. K., Lieberman, H. R., et al. (2020). A review of US Army research contributing to cognitive enhancement in military contexts. J. Cogn. Enhanc. 2020:167. doi: 10.1007/s41465-020-00167-3
Brunyé, T. T., and Giles, G. E. (2023). Methods for eliciting and measuring behavioral and physiological consequences of stress and uncertainty in virtual reality. Front. Virtual Real. 4:1435. doi: 10.3389/frvir.2023.951435
Brunyé, T. T., Yau, K., Okano, K., Elliott, G., Olenich, S., Giles, G. E., et al. (2021). Toward predicting human performance outcomes from wearable technologies: a computational modeling approach. Front. Physiol. 12:738973. doi: 10.3389/fphys.2021.738973
Brydges, C. R., Gignac, G. E., and Ecker, U. K. H. (2018). Working memory capacity, short-term memory capacity, and the continued influence effect: a latent-variable analysis. Intelligence 69, 117–122. doi: 10.1016/j.intell.2018.03.009
Burke, W. P., and Dyer, F. N. (1984). Physical fitness predictors of success and injury in ranger training. Alexandria, VA: Army Research Institute for the Behavioral and Social Sciences.
Cain, D. W., and Cidlowski, J. A. (2017). Immune regulation by glucocorticoids. Nat. Rev. Immunol. 17, 233–247. doi: 10.1038/nri.2017.1
Campbell, J. P., and Knapp, D. J. (2013). Exploring the limits in personnel selection and classification. London: Psychology Press.
Cantelon, J. A., Ramsay, J. W., Patterson, J., Eddy, M. D., and McKenzie, K. L. (2023). Combined effects of load carriage and tactical anaerobic sprints on executive function. Med. Sci. Sports Exerc. 55:610.
Casadei, K., and Kiel, J. (2023). Anthropometric Measurement. Treasure Island, FL: StatPearls Publishing.
Caza, A., Caza, B. B., and Baloochi, M. E. (2020). Resilient personality: is grit a source of resilience? Cheltenham, UK: Edward Elgar Publishing, 25–38.
Ceballos, N. A. (2006). Tobacco use, alcohol dependence, and cognitive performance. J. Gen. Psychol. 133, 375–388. doi: 10.3200/GENP.133.4.375-388
Chappelle, W., Thompson, W., Ouenpraseuth, S., Spencer, H., Goodman, T., Sanford, E., et al. (2018). “Pre-training cognitive and non-cognitive psychological predictors of U.S” in Air force Pararescue training outcomes. Wright-Patterson air force (Base, OH: Air Force Research Laboratory).
Charmandari, E., Tsigos, C., and Chrousos, G. (2005). Endocrinology of the stress response. Annu. Rev. Physiol. 67, 259–284. doi: 10.1146/annurev.physiol.67.040403.120816
Chatterjee, S., and Simonoff, J. S. (2013). Handbook of regression analysis. Hoboken, NJ: John Wiley and Sons.
Christensen, N. J. (1991). The biochemical assessment of sympathoadrenal activity in man. Clin. Auton. Res. 1, 167–172. doi: 10.1007/BF01826215
Chung, G. K. W., De La Cruz, G. C., de Vries, L. F., Kim, J. O., Bewley, W. L., de Souza e Silva, A., et al. (2004). Determinants of rifle marksmanship performance: Predicting shooting performance with advanced distributed learning assessments. Los Angeles, CA: University of California Los Angeles.
Cisternas, P., Lindsay, C. B., Salazar, P., Silva-Alvarez, C., Retamales, R. M., Serrano, F. G., et al. (2015). The increased potassium intake improves cognitive performance and attenuates histopathological markers in a model of Alzheimer’s disease. Biochim. Biophys. Acta (BBA) - Mol. Basis Dis. 1852, 2630–2644. doi: 10.1016/j.bbadis.2015.09.009
Crane, M. F., Boga, D., Karin, E., Gucciardi, D. F., Rapport, F., Callen, J., et al. (2019). Strengthening resilience in military officer cadets: a group-randomized controlled trial of coping and emotion regulatory self-reflection training. J. Consult. Clin. Psychol. 87, 125–140. doi: 10.1037/ccp0000356
Craven, J., McCartney, D., Desbrow, B., Sabapathy, S., Bellinger, P., Roberts, L., et al. (2022). Effects of acute sleep loss on physical performance: a systematic and Meta-analytical review. Sports Med. 52, 2669–2690. doi: 10.1007/s40279-022-01706-y
Croston, R., Branch, C. L., Kozlovsky, D. Y., Dukas, R., and Pravosudov, V. V. (2015). Heritability and the evolution of cognitive traits. Behav. Ecol. 26, 1447–1459. doi: 10.1093/beheco/arv088
Datu, J. A. D. (2021). Beyond passion and perseverance: review and future research initiatives on the science of grit. Front. Psychol. 11:526. doi: 10.3389/fpsyg.2020.545526
Deary, I. J., Penke, L., and Johnson, W. (2010). The neuroscience of human intelligence differences. Nat. Rev. Neurosci. 11, 201–211. doi: 10.1038/nrn2793
Demigné, C., Sabboh, H., Rémésy, C., and Meneton, P. (2004). Protective effects of high dietary potassium: nutritional and metabolic aspects. J. Nutr. 134, 2903–2906. doi: 10.1093/jn/134.11.2903
Department of the Army (2009). Soldier’s manual of common tasks. Washington, D.C: Department of the Army.
Der, G., and Deary, I. J. (2006). Age and sex differences in reaction time in adulthood: results from the United Kingdom health and lifestyle survey. Psychol. Aging 21, 62–73. doi: 10.1037/0882-7974.21.1.62
Duckworth, A. L., Peterson, C., Matthews, M. D., and Kelly, D. R. (2007). Grit: perseverance and passion for long-term goals. J. Pers. Soc. Psychol. 92, 1087–1101. doi: 10.1037/0022-3514.92.6.1087
Dudde, R., Muller, G., Bourdeaux, O., Weber, B., Gagey, B., and Gagey, P. (2012). The marksman’s motor control. 1 Stabilometric study of the changes during training. Paris, France: French Shooting Federation.
Dunn, P. K., and Smyth, G. K. (2018). “Chapter 3: linear regression models: diagnostics and model-building” in Generalized linear models with examples in R. eds. P. K. Dunn and G. K. Smyth (New York: Springer), 93–164.
Durlach, P. J., Kring, J. P., and Bowens, L. D. (2009). Effects of action video game experience on change detection. Mil. Psychol. 21, 24–39. doi: 10.1080/08995600802565694
Engle, R. W. (2002). Working memory capacity as executive attention. Curr. Dir. Psychol. Sci. 11, 19–23. doi: 10.1111/1467-8721.00160
Engle, R. W., and Kane, M. J. (2003). Executive attention, working memory capacity, and a two-factor theory of cognitive control. Psychol. Learn. Motiv. 2003:4005. doi: 10.1016/S0079-7421(03)44005-X
EUROCONTROL/FAA Action Plan 15 Safety (2010). Human performance in air traffic management safety: A white paper. Paris, France: EUROCONTROL.
Farina, E. K., Thompson, L. A., Knapik, J. J., Pasiakos, S. M., McClung, J. P., and Lieberman, H. R. (2019). Physical performance, demographic, psychological, and physiological predictors of success in the U.S. Army special forces assessment and selection course. Physiol. Behav. 210:112647. doi: 10.1016/j.physbeh.2019.112647
Flood, A., and Keegan, R. J. (2022). Cognitive resilience to psychological stress in military personnel. Front. Psychol. 13:9003. doi: 10.3389/fpsyg.2022.809003
Fowler, A., Golightly, D., Sharples, S., Harvey, C., Wilson, M. L., and Gibson, H. (2019). Human performance in rail: current assessment and future opportunities. In: Proceedings of the contemporary ergonomics and human factors conference, Chartered Institute of Ergonomics and Human Factors.
Fox, J. (2019). Regression diagnostics: An introduction. 2nd Edn. Thousand Oaks, CA: SAGE Publications.
Franklin, T. B., Saab, B. J., and Mansuy, I. M. (2012). Neural mechanisms of stress resilience and vulnerability. Neuron 75, 747–761. doi: 10.1016/j.neuron.2012.08.016
Friedman, J., Hastie, T., and Tibshirani, R. (2010). Regularization paths for generalized linear models via coordinate descent. J. Stat. Softw. 33, 1–22. doi: 10.18637/jss.v033.i01
Gagnon, S. A., and Wagner, A. D. (2016). Acute stress and episodic memory retrieval: neurobiological mechanisms and behavioral consequences. Ann. N. Y. Acad. Sci. 1369, 55–75. doi: 10.1111/nyas.12996
Garden, S., Cornoldi, C., and Logie, R. H. (2002). Visuo-spatial working memory in navigation. Appl. Cogn. Psychol. 16, 35–50. doi: 10.1002/acp.746
Gawron, V. J. (2000). Human performance measures handbook. Mahwah, NJ: Lawrence Erlbaum Associates Publishers.
Giles, G. E., Grandjean DA Costa, K., Olenich, S. A., Powell, K. J., Hart-Pomerantz, H., Adelman, M. J., et al. (2022). Load carriage and physical exertion influence soldier emotional responses. Med. Sci. Sports Exerc. 54, 2149–2157. doi: 10.1249/mss.0000000000002997
Giles, G. E., Navarro, E., Elkin-Frankston, S., Brunyé, T. T., Elmore, W. R., Seay, J. F., et al. (2023). Characterizing relationships among the cognitive, physical, social-emotional, and health-related traits of military personnel. Mil. Med. 188, e2275–e2283. doi: 10.1093/milmed/usad002
Gomes, K. B., Pereira, R. G., Braga, A. A., Guimarães, H. C., Resende, E. D. P. F., Teixeira, A. L., et al. (2023). Machine learning-based routine laboratory tests predict one-year cognitive and functional decline in a population aged 75+ years. Brain Sci. 13:690. doi: 10.3390/brainsci13040690
Grandou, C., Wallace, L., Fullagar, H. H. K., Duffield, R., and Burley, S. (2019). The effects of sleep loss on military physical performance. Sports Med. 49, 1159–1172. doi: 10.1007/s40279-019-01123-8
Grolleman, J. F., Gravesteijn, C., and Hoffenaar, P. J. (2023). Trajectories of change in parental self-esteem and emotion regulation from pregnancy until 4 years postpartum. J. Child Fam. Stud. 32, 1088–1101. doi: 10.1007/s10826-022-02306-0
Gross, J. J. (2015). Emotion regulation: current status and future prospects. Psychol. Inq. 26, 1–26. doi: 10.1080/1047840X.2014.940781
Guiney, H., and Machado, L. (2013). Benefits of regular aerobic exercise for executive functioning in healthy populations. Psychon. Bull. Rev. 20, 73–86. doi: 10.3758/s13423-012-0345-4
Hanson, J. A., Wagner, G., and Dretsch, M. (2021). Cognitive disinhibition and infrequent moderate-to-intense physical activity linked with obesity in U.S. soldiers. Eat. Weight Disord. 26, 973–981. doi: 10.1007/s40519-020-00932-5
Harner, A. E., and Root, M. M. (2023). Potassium and magnesium and cognitive decline in middle-aged adults. Nutr. Healthy Aging 8, 183–191. doi: 10.3233/NHA-220185
Heinrich, K. M., Spencer, V., Fehl, N., and Carlos Poston, W. S. (2012). Mission essential fitness: comparison of functional circuit training to traditional army physical training for active duty military. Mil. Med. 177, 1125–1130. doi: 10.7205/MILMED-D-12-00143
Hertzog, C. (1991). “Aging, information processing speed, and intelligence” in Annual review of gerontology and geriatrics (New York: Springer Publishing Company), 55–79.
Hockey, G. R. J. (1997). Compensatory control in the regulation of human performance under stress and high workload: a cognitive-energetical framework. Biol. Psychol. 45, 73–93. doi: 10.1016/S0301-0511(96)05223-4
Humphrey, N., Kalambouka, A., Wigelsworth, M., Lendrum, A., Deighton, J., and Wolpert, M. (2011). Measures of social and emotional skills for children and young people: a systematic review. Educ. Psychol. Meas. 71, 617–637. doi: 10.1177/0013164410382896
Jáuregui-Lobera, I. (2014). Iron deficiency and cognitive functions. Neuropsychiatr. Dis. Treat. 10, 2087–2095. doi: 10.2147/NDT.S72491
Kalisch, R., Russo, S. J., and Müller, M. B. (2024). Neurobiology and systems biology of stress resilience. Physiol. Rev. 104, 1205–1263. doi: 10.1152/physrev.00042.2023
Kanai, R., and Rees, G. (2011). The structural basis of inter-individual differences in human behaviour and cognition. Nat. Rev. Neurosci. 12, 231–242. doi: 10.1038/nrn3000
Kapa, L. L., and Colombo, J. (2014). Executive function predicts artificial language learning. J. Mem. Lang. 76, 237–252. doi: 10.1016/j.jml.2014.07.004
Kaplan, H. (1965). Prediction of success in Army aviation training. United States: Army Personnel Research Office.
Keebler, J. R., Jentsch, F., and Schuster, D. (2014). The effects of video game experience and active stereoscopy on performance in combat identification tasks. Hum. Factors 56, 1482–1496. doi: 10.1177/0018720814535248
Khripunov, I. (2023). “Application of culture methodology in non-nuclear domains” in Human factor in nuclear security: Establishing and optimizing security culture. ed. I. Khripunov (Cham: Springer International Publishing), 127–143.
Korthauer, L. E., Nowak, N. T., Frahmand, M., and Driscoll, I. (2017). Cognitive correlates of spatial navigation: associations between executive functioning and the virtual Morris water task. Behav. Brain Res. 317, 470–478. doi: 10.1016/j.bbr.2016.10.007
Kraemer, W. J., and Szivak, T. K. (2012). Strength training for the warfighter. J. Strength Cond. Res. 26:S107. doi: 10.1519/JSC.0b013e31825d8263
Kronman, C. A., Kern, K. L., Nauer, R. K., Dunne, M. F., Storer, T. W., and Schon, K. (2020). Cardiorespiratory fitness predicts effective connectivity between the hippocampus and default mode network nodes in young adults. Hippocampus 30, 526–541. doi: 10.1002/hipo.23169
Kuhn, M. (2008). Building predictive models in R using the caret package. J. Stat. Softw. 28, 1–26. doi: 10.18637/jss.v028.i05
Kung, W.-M., Yuan, S.-P., Lin, M.-S., Wu, C.-C., Islam, M. M., Atique, S., et al. (2021). Anemia and the risk of cognitive impairment: an updated systematic review and Meta-analysis. Brain Sci. 11:777. doi: 10.3390/brainsci11060777
Lee, S. R., Kim, H. K., Youm, J. B., Dizon, L. A., Song, I. S., Jeong, S. H., et al. (2012). Non-genomic effect of glucocorticoids on cardiovascular system. Pflugers Arch. - Eur. J. Physiol. 464, 549–559. doi: 10.1007/s00424-012-1155-2
Lee, M. D., and Webb, M. R. (2005). Modeling individual differences in cognition. Psychon. Bull. Rev. 12, 605–621. doi: 10.3758/BF03196751
Lester, M. E., Sharp, M. A., Werling, W. C., Walker, L. A., Cohen, B. S., and Ruediger, T. M. (2014). Effect of specific short-term physical training on fitness measures in conditioned men. J. Strength Cond. Res. 28:679. doi: 10.1519/JSC.0b013e318299912b
Liang, Y., Li, S., Yan, C., Li, M., and Jiang, C. (2021). Explaining the black-box model: a survey of local interpretation methods for deep neural networks. Neurocomputing 419, 168–182. doi: 10.1016/j.neucom.2020.08.011
Lieberman, H. R., Bathalon, G. P., Falco, C. M., Morgan, C. A., Niro, P. J., and Tharion, W. J. (2005). The fog of war: decrements in cognitive performance and mood associated with combat-like stress. Aviat. Space Environ. Med. 76, C7–C14
Lieberman, H. R., Farina, E. K., Caldwell, J., Williams, K. W., Thompson, L. A., Niro, P. J., et al. (2016). Cognitive function, stress hormones, heart rate and nutritional status during simulated captivity in military survival training. Physiol. Behav. 165, 86–97. doi: 10.1016/j.physbeh.2016.06.037
Lin, J., Wohleber, R., Matthews, G., Chiu, P., Calhoun, G., Ruff, H., et al. (2015). Video game experience and gender as predictors of performance and stress during supervisory control of multiple unmanned aerial vehicles. Proc. Hum. Fact. Ergon. Soc. Annu. Meet. 59, 746–750. doi: 10.1177/1541931215591175
Linck, J. A., Kroll, J. F., and Sunderman, G. (2009). Losing access to the native language while immersed in a second language evidence for the role of inhibition in second-language learning. Psychol. Sci. 20, 1507–1515. doi: 10.1111/j.1467-9280.2009.02480.x
Makuch, M., Makuch, M., and Tuzim, K. (2019). Hyperkalemia-a review article. J. Educ. Health Sport. 9, 167–177.
Martin, K., McLeod, E., Periard, J., Rattray, B., Keegan, R., and Pyne, D. B. (2019). The impact of environmental stress on cognitive performance: a systematic review. Hum Factors 61, 1205–1246. doi: 10.1177/0018720819839817
Maughan, R. J., Leiper, J. B., and Shirreffs, S. M. (1997). Factors influencing the restoration of fluid and electrolyte balance after exercise in the heat. Br. J. Sports Med. 31, 175–182. doi: 10.1136/bjsm.31.3.175
McCabe, D. P., Roediger, H. L., McDaniel, M. A., Balota, D. A., and Hambrick, D. Z. (2010). The relationship between working memory capacity and executive functioning: evidence for a common executive attention construct. Neuropsychology 24, 222–243. doi: 10.1037/a0017619
McClung, J. P., Beckner, M. E., and Farina, E. K. (2023). Assessing the physiological basis for resilience in military personnel. Stress. Health 39, 33–39. doi: 10.1002/smi.3271
McVay, J. C., and Kane, M. J. (2012). Why does working memory capacity predict variation in reading comprehension? On the influence of mind wandering and executive attention. J. Exp. Psychol. Gen. 141, 302–320. doi: 10.1037/a0025250
Meneghetti, C., Labate, E., Toffalini, E., and Pazzaglia, F. (2021). Successful navigation: the influence of task goals and working memory. Psychol. Res. 85, 634–648. doi: 10.1007/s00426-019-01270-7
Meneghetti, C., Zancada-Menéndez, C., Sampedro-Piquero, P., Lopez, L., Martinelli, M., Ronconi, L., et al. (2016). Mental representations derived from navigation: the role of visuo-spatial abilities and working memory. Learn. Individ. Differ. 49, 314–322. doi: 10.1016/j.lindif.2016.07.002
Meuleman, B., Loosveldt, G., and Emonds, V. (2014). “Regression analysis: assumptions and diagnostics” in The SAGE handbook of regression analysis and causal inference. ed. B. Meuleman (London: SAGE Publications), 83–110.
Morey, J. N., Boggero, I. A., Scott, A. B., and Segerstrom, S. C. (2015). Current directions in stress and human immune function. Curr. Opin. Psychol. 5, 13–17. doi: 10.1016/j.copsyc.2015.03.007
Motowidlo, S. J., Borman, W. C., and Schmit, M. J. (1997). A theory of individual differences in task and contextual performance. Hum. Perform. 10, 72–83. doi: 10.1207/s15327043hup1002_1
Muhs, K. S., Karwowski, W., and Kern, D. (2018). Temporal variability in human performance: a systematic literature review. Int. J. Ind. Ergon. 64, 31–50. doi: 10.1016/j.ergon.2017.10.002
Naug, D., and Tait, C. (2021). Slow-fast cognitive phenotypes and their significance for social behavior: what can we learn from honeybees? Front. Ecol. Evol. 9:414. doi: 10.3389/fevo.2021.766414
Naval Air Warfare Center . (2021). Research compendium: Training, human performance, modeling and simulation. Naval Air Warfare Center Training Systems Division. Available at: https://nps.edu/documents/115559645/121916825/2021+Dist+A+NAWCTSD+Research+Compendium+Feb+2021.pdf.
Nelson, S. K., Kushlev, K., English, T., Dunn, E. W., and Lyubomirsky, S. (2013). In defense of parenthood: children are associated with more joy than misery. Psychol. Sci. 24, 3–10. doi: 10.1177/0956797612447798
Neumann, R. J., Ahrens, K. F., Kollmann, B., Goldbach, N., Chmitorz, A., Weichert, D., et al. (2022). The impact of physical fitness on resilience to modern life stress and the mediating role of general self-efficacy. Eur. Arch. Psychiatry Clin. Neurosci. 272, 679–692. doi: 10.1007/s00406-021-01338-9
Newell, K. M. (1993). Variability and motor control. Champaign, Illinois: Human Kinetics Publishers.
Nielsen, B., SjØgaard, G., Ugelvig, J., Knudsen, B., and Dohlmann, B. (1986). Fluid balance in exercise dehydration and rehydration with different glucose-electrolyte drinks. Eur. J. Appl. Physiol. 55, 318–325. doi: 10.1007/BF02343806
Nila, K., Holt, D. V., Ditzen, B., and Aguilar-Raab, C. (2016). Mindfulness-based stress reduction (MBSR) enhances distress tolerance and resilience through changes in mindfulness. Ment. Health Prev. 4, 36–41. doi: 10.1016/j.mhp.2016.01.001
Nindl, B. C., Billing, D. C., Drain, J. R., Beckner, M. E., Greeves, J., Groeller, H., et al. (2018). Perspectives on resilience for military readiness and preparedness: report of an international military physiology roundtable. J. Sci. Med. Sport 21, 1116–1124. doi: 10.1016/j.jsams.2018.05.005
O’Donovan, M., Hancock, C. L., Coyne, M. E., Racicot, K., and Goodwin, G. A. (2023). Assessing the impact of dismounted infantry small unit proficiency on quantitative measures of collective military performance. Part 1: Recommended test methodologies. Natick, MA: U.S. Army DEVCOM Soldier Center.
O’Fallon, K. S., Mateer, E., Straight, C., Schindler, J. B., and McKenzie, K. L. (2022). Effects of a simulated multi-day Mission on muscle strength, soreness, and systemic inflammation: 536. Med. Sci. Sports Exerc. 54:135. doi: 10.1249/01.mss.0000876728.38116.f9
Oh, S. J., Schiele, B., and Fritz, M. (2019). “Towards reverse-engineering black-box neural networks” in Explainable AI: Interpreting, explaining and visualizing deep learning. eds. W. Samek, G. Montavon, A. Vedaldi, L. K. Hansen, and K.-R. Müller (Cham: Springer International Publishing), 121–144.
Palmer, C. J., and Van Emmerik, R. E. A. (2020). Constraints of load and posture on coordination variability and marksmanship performance. Mot. Control. 24, 435–456. doi: 10.1123/mc.2019-0082
Parush, A. (2015). Conceptual Design for Interactive Systems: Designing for performance and user experience. Burlington, MA: Morgan Kaufmann.
Petrofsky, L. A., Heffernan, C. M., Gregg, B. T., and Smith-Forbes, E. V. (2022). Effects of sleep deprivation in military service members on cognitive performance: a systematic review. Mil. Behav. Health 10, 202–220. doi: 10.1080/21635781.2021.1982088
Pfau, M. L., and Russo, S. J. (2015). Peripheral and central mechanisms of stress resilience. Neurobiol. Stress 1, 66–79. doi: 10.1016/j.ynstr.2014.09.004
Pidgeon, A. M., and Keye, M. (2014). Relationship between resilience, mindfulness, and pyschological well-being in university students. Int. J. Liber. Arts Soc. Sci. 2, 27–32.
Pollard, M. A., and Courage, M. L. (2017). Working memory capacity predicts effective multitasking. Comput. Hum. Behav. 76, 450–462. doi: 10.1016/j.chb.2017.08.008
Poole, B. J., and Kane, M. J. (2009). Working-memory capacity predicts the executive control of visual search among distractors: the influences of sustained and selective attention. Q. J. Exp. Psychol. 62, 1430–1454. doi: 10.1080/17470210802479329
Prichard, E., Propper, R., and Christman, S. (2013). Degree of handedness, but not direction, is a systematic predictor of cognitive performance. Front. Psychol. 4:9. doi: 10.3389/fpsyg.2013.00009
Quante, L., Zhang, M., Preuk, K., and Schießl, C. (2021). Human performance in critical scenarios as a benchmark for highly automated vehicles. Automot. Innov. 4, 274–283. doi: 10.1007/s42154-021-00152-2
Rabin, L. A., Fogel, J., and Nutter-Upham, K. E. (2011). Academic procrastination in college students: the role of self-reported executive function. J. Clin. Exp. Neuropsychol. 33, 344–357. doi: 10.1080/13803395.2010.518597
Reilly, T., and Edwards, B. (2007). Altered sleep–wake cycles and physical performance in athletes. Physiol. Behav. 90, 274–284. doi: 10.1016/j.physbeh.2006.09.017
Robison, M. K., and Unsworth, N. (2017). Individual differences in working memory capacity predict learned control over attentional capture. J. Exp. Psychol. Hum. Percept. Perform. 43, 1912–1924. doi: 10.1037/xhp0000419
Rothwell, W. J., Hohne, C. K., and King, S. B. (2012). Human performance improvement. Abingdon: Routledge.
Rutherford, H. J. V., Wallace, N. S., Laurent, H. K., and Mayes, L. C. (2015). Emotion regulation in parenthood. Dev. Rev. 36, 1–14. doi: 10.1016/j.dr.2014.12.008
Salmon, C. (2020). Multiple methodologies: addressing ecological validity and conceptual replication. Evol. Behav. Sci. 14, 373–378. doi: 10.1037/ebs0000213
Samuelson, K. W., Newman, J., Abu Amara, D., Qian, M., Li, M., Schultebraucks, K., et al. (2020). Predeployment neurocognitive functioning predicts postdeployment posttraumatic stress in Army personnel. Neuropsychology 34, 276–287. doi: 10.1037/neu0000603
Sapolsky, R. M., Romero, L. M., and Munck, A. U. (2000). How do glucocorticoids influence stress responses? Integrating permissive, suppressive, stimulatory, and preparative actions. Endocr. Rev. 21, 55–89. doi: 10.1210/edrv.21.1.0389
Schilling, R., Herrmann, C., Ludyga, S., Colledge, F., Brand, S., Pühse, U., et al. (2020). Does cardiorespiratory fitness buffer stress reactivity and stress recovery in police officers? A real-life study. Front. Psychiatry 11:594. doi: 10.3389/fpsyt.2020.00594
Schommer, N. C., Hellhammer, D. H., and Kirschbaum, C. (2003). Dissociation between reactivity of the hypothalamus-pituitary-adrenal axis and the sympathetic-adrenal-medullary system to repeated psychosocial stress. Psychosom. Med. 65, 450–460. doi: 10.1097/01.PSY.0000035721.12441.17
Schwabe, L., Joëls, M., Roozendaal, B., Wolf, O. T., and Oitzl, M. S. (2012). Stress effects on memory: an update and integration. Neurosci. Biobehav. Rev. 36, 1740–1749. doi: 10.1016/j.neubiorev.2011.07.002
Sejersted, O. M., and Sjøgaard, G. (2000). Dynamics and consequences of potassium shifts in skeletal muscle and heart during exercise. Physiol. Rev. 80, 1411–1481. doi: 10.1152/physrev.2000.80.4.1411
Sellaro, R., and Colzato, L. S. (2017). High body mass index is associated with impaired cognitive control. Appetite 113, 301–309. doi: 10.1016/j.appet.2017.03.008
Shettleworth, S. J. (2009). Cognition, evolution, and behavior. Oxford, UK: Oxford University Press.
Shields, G. S., Sazma, M. A., and Yonelinas, A. P. (2016). The effects of acute stress on core executive functions: a meta-analysis and comparison with cortisol. Neurosci. Biobehav. Rev. 68, 651–668. doi: 10.1016/j.neubiorev.2016.06.038
Smith, C., Doma, K., Heilbronn, B., and Leicht, A. (2022). Effect of exercise training programs on physical fitness domains in military personnel: a systematic review and meta-analysis. Mil. Med. 187, 1065–1073. doi: 10.1093/milmed/usac040
Smith, T. J., Henning, R. A., Wade, M. G., and Fisher, T. (2014). Variability in human performance. Boca Raton, FL: CRC Press.
Smith, S. M., and Vale, W. W. (2006). The role of the hypothalamic-pituitary-adrenal axis in neuroendocrine responses to stress. Dialogues Clin. Neurosci. 8, 383–395. doi: 10.31887/dcns.2006.8.4/ssmith
Spencer, S. J., Korosi, A., Layé, S., Shukitt-Hale, B., and Barrientos, R. M. (2017). Food for thought: how nutrition impacts cognition and emotion. NPJ Sci Food 1:7. doi: 10.1038/s41538-017-0008-y
Starcke, K., and Brand, M. (2012). Decision making under stress: a selective review. Neurosci. Biobehav. Rev. 36, 1228–1248. doi: 10.1016/j.neubiorev.2012.02.003
Steyer, R., Mayer, A., Geiser, C., and Cole, D. A. (2015). A theory of states and traits—revised. Annu. Rev. Clin. Psychol. 11, 71–98. doi: 10.1146/annurev-clinpsy-032813-153719
Suárez, V., Picotin, R., Fassbender, R., Gramespacher, H., Haneder, S., Persigehl, T., et al. (2024). Chronic hyponatremia and brain structure and function before and after treatment. Am. J. Kidney Dis. 2024:7. doi: 10.1053/j.ajkd.2023.11.007
Travis, T. W., and Brown, D. L. (2023). Human performance optimization: a framework for the military health system. Mil. Med. 188, 44–48. doi: 10.1093/milmed/usac411
Troy, A. S., and Mauss, I. B. (2011). “Resilience in the face of stress: emotion regulation as a protective factor” in Resilience and mental health: Challenges across the lifespan. ed. A. S. Troy (Cambridge, UK: Cambridge University Press), 30–44.
Umberson, D., and Gove, W. R. (1989). Parenthood and psychological well-being: theory, measurement, and stage in the family life course. J. Fam. Issues 10, 440–462. doi: 10.1177/019251389010004002
Uttal, D. H., Meadow, N. G., Tipton, E., Hand, L. L., Alden, A. R., Warren, C., et al. (2013). The malleability of spatial skills: a meta-analysis of training studies. Psychol Bull. 139, 352–402.
Valladares, M., Durán, E., Matheus, A., Durán-Agüero, S., Obregón, A. M., and Ramírez-Tagle, R. (2016). Association between eating behavior and academic performance in university students. J. Am. Coll. Nutr. 35, 699–703. doi: 10.1080/07315724.2016.1157526
Vartanian, O., Fraser, B., Saunders, D., Suurd Ralph, C., Lieberman, H. R., Morgan, C. A., et al. (2018). Changes in mood, fatigue, sleep, cognitive performance and stress hormones among instructors conducting stressful military captivity survival training. Physiol. Behav. 194, 137–143. doi: 10.1016/j.physbeh.2018.05.008
Vedhara, K., Hyde, J., Gilchrist, I. D., Tytherleigh, M., and Plummer, S. (2000). Acute stress, memory, attention and cortisol. Psychoneuroendocrinology 25, 535–549. doi: 10.1016/S0306-4530(00)00008-1
White, M. P., and Dolan, P. (2009). Accounting for the richness of daily activities. Psychol. Sci. 20, 1000–1008. doi: 10.1111/j.1467-9280.2009.02392.x
Wingo, J., Kalkut, E., Tuminello, E., Asconape, J., and Han, S. D. (2013). Executive functions, depressive symptoms, and college adjustment in women. Appl. Neuropsychol. Adult 20, 136–144. doi: 10.1080/09084282.2012.670154
Keywords: performance, prediction, traits, machine learning, military perception, memory, decision making, emotion
Citation: Brunyé TT, Goring SA, Cantelon JA, Eddy MD, Elkin-Frankston S, Elmore WR, Giles GE, Hancock CL, Masud SB, McIntyre J, McKenzie KL, Mitchell KB, O’Donovan MP, Racicot K and Ramsay JW (2024) Trait-level predictors of human performance outcomes in personnel engaged in stressful laboratory and field tasks. Front. Psychol. 15:1449200. doi: 10.3389/fpsyg.2024.1449200
Edited by:
Tommi Ojanen, Finnish Defence Resarch Agency, Finnish Defence Forces, FinlandReviewed by:
Nathalie Pattyn, Vrije University Brussels, BelgiumMatthew Bird, University of Pittsburgh, United States
Copyright © 2024 Brunyé, Goring, Cantelon, Eddy, Elkin-Frankston, Elmore, Giles, Hancock, Masud, McIntyre, McKenzie, Mitchell, O’Donovan, Racicot and Ramsay. This is an open-access article distributed under the terms of the Creative Commons Attribution License (CC BY). The use, distribution or reproduction in other forums is permitted, provided the original author(s) and the copyright owner(s) are credited and that the original publication in this journal is cited, in accordance with accepted academic practice. No use, distribution or reproduction is permitted which does not comply with these terms.
*Correspondence: Tad T. Brunyé, dGhhZGRldXMudC5icnVueWUuY2l2QGFybXkubWls