- 1The Hebrew University Business School, The Hebrew University, Jerusalem, Israel
- 2Musicology Department, The Hebrew University of Jerusalem, Jerusalem, Israel
Introduction: In stressful times, people often listen to “coping songs” that help them reach emotional well-being goals. This paper is a first attempt to map the connection between an individual’s well-being goals and their chosen coping song.
Methods: We assembled a large-scale dataset of 2,804 coping songs chosen by individuals from 11 countries during COVID-19 lockdown. Individuals reported their well-being goals and also named their coping song. We applied an unsupervised topic-modeling approach to identify 15 self-emerging topics from the song lyrics, and connected them to well-being goals.
Results: We found significant association between certain lyrics’ topics and specific well-being goals. This association weakened for participants for which music is highly important. No significant patterns were found for the songs’ acoustic features.
Discussion: This paper posits that song lyrics, despite their brevity and presumed simplicity, can be meaningful for self-regulation of emotional states, and should receive more attention by researchers and streaming services alike.
Introduction
Coping songs to achieve mood regulation goals
Listening to music is a simple yet powerful way of coping with stressful situations (e.g., Gabrielsson, 2001; Juslin et al., 2008; Vidas et al., 2021). Music can help regulate one’s stress levels (Baltazar et al., 2019; De Witte et al., 2020) and anxiety (Knight and Rickard, 2001), and help manage negative and painful feelings (Saarikallio and Erkkilä, 2007). In stressful times, people may relate to “coping songs,” or musical pieces that they listen to regularly and that help them through their hardship (Grocke and Wigram, 2006; Silverman, 2009; Hamilton et al., 2013; Vidas et al., 2021). Thus, adopting a coping song can be viewed as a self-regulation praxis that enhances well-being (McFerran and Saarikallio, 2014).
What characterizes the choice of coping songs during stressful times? Do their lyrics share common themes? Is there a distinct musical composition? Or perhaps a certain music-lyrics combination? Is there a link between one’s desire for mood regulation and the chosen song? This paper aims to shed light on these questions.
On the theoretical level, mood regulation involves consciously or unconsciously, setting goals aiming towards a desired emotional state (e.g., venting stress or anxiety, diversion from a current difficulty, enhancing enjoyment, reducing loneliness), and then selecting a musical piece that aids in achieving these goals (Baltazar and Saarikallio, 2019). This paper primarily examines the link between these goals and the chosen coping song.
Considerable research has examined various ways in which music supports emotional regulation and well-being goals (e.g., MacDonald et al., 2013; Baltazar and Saarikallio, 2016). However, the specific connection between self-selected coping songs–including both their music and lyrics- and emotional regulation goals remains largely unexplored. In addition, only a limited number of studies have examined mood regulation mechanisms associated with song lyrics (Hanser et al., 2016; ter Bogt et al., 2017; Baltazar and Saarikallio, 2019; Baltazar and Västfjäll, 2020). This gap is surprising, as songs are the most common form of music that individuals listen to.1
We study the link between emotional regulation goals and the chosen coping song in a real-life setting by using a large-scale unique dataset containing 2,804 songs chosen by 5,619 participants in 11 countries following the first COVID-19 pandemic lockdown. We analyze this large data set employing unsupervised topic-modeling methods that enable us to extract themes in the songs’ lyrics, that we then connect to the participants self-reported emotional goals.
Our analysis shows a significant association between certain lyrics topics and specific well-being goals. We found that this association weakens—but is still significant—for participants who indicated that music is highly important in their lives. We did not identify significant relationships between goals and the nominated songs’ acoustic features.
Our contribution is significant in substantive, methodological, and practical terms. Substantially, this is a novel attempt to link the choice of coping songs to mood-regulation goals in a large-scale, real-life setting. We suggest that song lyrics, despite their brevity and presumed simplicity, can be meaningful for listeners. Methodologically, this study is pioneering in linking such an extensive, self-selected collection of coping songs—diverse in language, culture, genre, and epoch—with well-being goals in an ecologically valid yet relatively controlled setting. We also demonstrate the application of unsupervised text analysis techniques to large-scale song data to uncover thematic elements in lyrics. Our findings underscore the importance of incorporating song lyrics in music and well-being research, and have practical implications for streaming services that seek to align songs with listeners’ needs. For streaming platforms, this could mean applying our results within recommendation algorithms to create personalized playlists. By using listening data—or even by directly engaging users—platforms can identify users’ emotional states and well-being goals, and integrate our procedure and results to analyze lyrical themes to recommend songs that more accurately support mood regulation goals.
Literature review
Predicting which music could assist a given individual to obtain a chosen goal is challenging due to the lack of a complete model of goals and music choice strategy. There are often a number of routes to obtaining the same goal, including blends of various strategies (Gross, 2015). Also, music choice depends on contextual and individual traits such as age (Groarke and Hogan, 2019), gender (Carlson et al., 2015; ter Bogt et al., 2017), personality traits (Garrido et al., 2015), and level of musical sophistication (Carvalho et al., 2022).
Although predicting which musical piece an individual will choose for a specific mood regulation goal is challenging, significant progress has been made in understanding how people use music to regulate their mood or emotions. Studies have shown that music is used for reducing stress and anxiety (De Witte et al., 2020), for diversion, pleasure, elevating and maintaining good mood (Randall et al., 2014; Cook et al., 2019), for solace, release from negative emotions, and repair through reappraisal (Hanser et al., 2016), and for reducing feelings of loneliness through reconnecting to positive nostalgic memories, or surrogating relationships (McFerran and Saarikallio, 2014; Schäfer et al., 2020).
The role of lyrics in mood regulation
The personal “meaning” of a song for an individual arises from a complex web of interactions between its text, musical composition and performance, the ideas it conveys, and the very personal interpretation and emotions the song evokes in the listener. Within this web, lyrics can potentially play an important role. Yet popular song lyrics have mainly been studied from a socio-cultural perspective as indicators of changes in public sentiment and the relative importance of various social themes such as inequality, hardships, sexuality, violence, or substance use (e.g., Zullow, 1991; Brand et al., 2019; Christenson et al., 2019; Qiu et al., 2019). Other studies have reported analysis of song lyrics (with or without acoustic features) for genre or emotion identification (Logan et al., 2004; Han et al., 2022). Many of these studies use variations of word counting procedures, although usage of more sophisticated topic modeling methods such as Latent Dirichlet Allocation (LDA) is becoming more prevalent (Kleedorfer et al., 2008; Sasaki et al., 2014; Fell et al., 2019, 2022; Nakai and Itoh, 2022).
Those studies that have explored lyrics as a possible aid for personal mood or affect regulation through songs, suggest that listeners can use the message in the lyrics to work through their emotions and possibly to see these emotions in a new light as reflected for example in empowering lyrics (Baltazar and Saarikallio, 2019; Baltazar and Västfjäll, 2020). Alternatively, those in a sad or distressing situation may find solace in sad songs with which they can identify. Here the lyrics can provide a sense of acceptance and belonging (“the artist that sings the songs feels what I am feeling” - Hanser et al., 2016; ter Bogt et al., 2017).
Other studies have sought to examine the relative contribution of the music versus the lyrics in the emotions perceived in the songs. While some found that lyrics influence mood more than music (Stratton and Zalanowski, 1994), others have shown the opposite (Sousou, 1997) with a moderating effect of the listener’s culture (Barradas and Sakka, 2022). The relative role of lyrics depends upon the song’s valence, i.e., lyrics were found to be important in enhancing emotions for songs with sad and angry music, but not in songs whose music was associated with happiness or calm (Ali and Peynircioğlu, 2006; Baltazar and Västfjäll, 2020).
In this paper, we inspect lyrics through the perspective of coping songs and their relationship to mood self-regulation. We focus on the individual level (rather than the socio-cultural), and quantify the connection between the lyrics of the selected songs and self-regulation goals. By analyzing a large number of songs, we can identify common themes in the lyrics and link them to well-being goals.
The COVID pandemic as a unique opportunity to study how songs help in mood regulation
The COVID-19 pandemic has provided a unique opportunity to explore, in a real setting, the link between coping songs and mood regulation across a large number of songs and individuals. The stress, anxiety, and loneliness experienced by millions, called clearly for implementation of emotion regulation strategies, wherein music listening served an important function (Carlson et al., 2015, 2021; Granot et al., 2021; Hennessy et al., 2021; Krause et al., 2021; Martín et al., 2021; Ziv and Hollander-Shabtai, 2021).
Research has shown that music did indeed help many people to cope with the negative emotions provoked by the COVID-19 crisis (Cabedo-Mas et al., 2021; Carlson et al., 2021; Krause et al., 2021; Martín et al., 2021; Ziv and Hollander-Shabtai, 2021), especially those experiencing high distress (Hennessy et al., 2021). This has been found to hold cross-culturally (Granot et al., 2021; Hennessy et al., 2021). Yet, the size of the positive effect varied as a function of individual differences including age (Ferreri et al., 2021; Granot et al., 2021; Henry et al., 2021; Martín et al., 2021), importance of music in one’s life (Granot et al., 2021; Henry et al., 2021), musical training (Martínez-Castilla et al., 2021) and personality traits like openness to experience (Granot et al., 2021), empathy (Hennessy et al., 2021), and sensitivity to musical reward (Mas-Herrero et al., 2021). The mood regulation strategies found useful are consistent with Baltazar and Saarikallio’s (2019) proposed model, and include reappraisal and positive reframing (Hennessy et al., 2021; Henry et al., 2021), discharge of negative emotions, especially in those higher in anxiety (Hennessy et al., 2021), and seeking out positive/happy music (Ferreri et al., 2021). Yet, none of these studies looked at specific real-time musical selections that supported these coping strategies. One of the few studies that did so examined acoustic features of self-selected songs that helped to “manage stress” among Australian students (Vidas et al., 2021). They found that the 271 songs in their data set had lower energy and valence, and tended to be more instrumental, compared to a benchmark dataset of Howlin and Rooney (2021), which contains 153 songs self-selected to reduce pain. They also found no significant correlations between acoustic/musical features such as energy, valence, tempo, danceability and acousticness, and well-being measures. However, the paper does not address songs’ lyrics.
Our study extends the literature by examining how individuals choose songs to cope with stressful situations. We specifically address both song lyrics and acoustic features, directly investigating the link between the selection of coping songs and mood-regulation goals—an area that has received limited attention. Utilizing a unique dataset of self-selected coping songs and individually rated emotional regulation goals, we explore, on a large scale and in a real-life context, whether the musical composition and lyrics of these songs can shed light on how people use them to cope during challenging times.
Methods
Data collection
Exploring the connection between coping songs and self-regulation goals requires assembling a large dataset of self-selected songs for achieving various well-being goals associated with mood regulation from a large number of individuals. This is challenging, as it requires obtaining information from many individuals on their coping songs, as well as information on their mood and on their self-regulation goals. Asking people to explicitly indicate what song would help them obtain a specific affective goal, could result in cognitive biases such as time-relatedness (e.g., recency, i.e., the song was heard recently), or social biases (responding according to what is socially appropriate or is expected of them). Overcoming these biases requires careful manipulations which are hardly feasible for a large-scale data collection.
Another option for obtaining such data could presumably be using the Experience Sampling Method in which participants are asked to fill-out questionnaires several times in response to experimenters’ scheduled cues. This enables to capture music listening in “natural settings” over very differing contexts and situations as indeed has been successfully implemented in a number of studies (e.g., Juslin et al., 2008; Randall et al., 2014). Yet, this method has its drawbacks, as respondents are very much aware of participating in a study and can probably easily discern its goals.
For the current paper, we used a large-scale unique dataset assembled by Granot et al. (2021), following the first COVID-19 pandemic lockdown, which monitored participants’ emotional states as well as their coping mechanisms. Granot et al. (2021) administered a 15-min online questionnaire during the first wave of the COVID-19 pandemic (between July and November 2020), to a convenience sample of 5,619 participants from 11 countries that were severely affected by the pandemic: Argentina, Brazil, China, Colombia, Italy, Mexico, the Netherlands, Norway, Spain, the UK, and the US. The questionnaire was available in six languages (Chinese, Dutch, English, Italian, Norwegian, and Spanish). As explained to respondents, the survey was intended to “gain a better understanding of how people cope with the COVID19 crisis by using daily activities, especially music, in order to reduce negative feelings and maintain wellbeing in these complex times.” The questionnaire contained seven sections that examined respondents’ feelings, emotions, and coping activities besides music, during COVID-19 lockdown.
Coping songs
Our focus is on the question that was not used in the Granot et al. (2021) paper, which asked participants to provide the title of/link to the song that helped them cope most during lockdown (see Supplementary Appendix A). If the name of the song was provided instead of a link, we searched for the song and used the most popular version. Of the entire sample, we removed 1,366 participants who did not provide a link to/title of a song. As our study analyzes lyrics, we retained only the 2,804 responses that included songs with lyrics. Note that the survey captured a snapshot of coping songs at a specific point in time. It did not inquire about the use of coping songs pre- pandemic, nor did it track changes in use throughout the entire lockdown.
Well-being goals
For each of the 2,804 participants we used the “Goals” section from Granot et al.’s (2021) questionnaire. This section, being central to the study, always appeared first, (with other five sections presented in a randomized order). Participants were asked to rank how important on a five-point Likert scale (from 0 “irrelevant” to 4 “to a very large degree”) five wellbeing goals were in their coping with the lockdown and the situation that was imposed on them. The goals were: release and venting of negative emotions (e.g., stress, anxiety, anger); Diversion from the crisis; Enjoyment and maintaining good mood; Reducing loneliness and creating a sense of togetherness; Connecting with myself and detachment from the surroundings. Participants could also add goals in a blank space but these did not reach 2% of the sample.
Participants’ sample statistics
The number of respondents per country who provided a song link/title ranges from 83 (China) to 498 (Colombia), with a median of 250. On average, two-thirds of the respondents were female (range between countries 50–76%); 30% were younger than 24 years old; 45% were 25–44; 20% were 45–64; and 4% were over 64. About 34% of respondents reported having a low level of spirituality, compared with 66% who reported a medium or high level. Most respondents assigned a high level of importance to music (75% between 4 and 5), while only 8% assigned it a low level (between 1 and 2). See Supplementary Appendix B for complete descriptive sample statistics.
Extracting songs’ lyrics and genre
Lyrics were extracted using a YouTube plugin we developed for the project. A designated script first automatically opened the song’s YouTube link, and then used the plugin to extract the lyrics (which in most cases are displayed in the information section underneath the video box), into a dedicated file. Lyrics in languages other than English (953 out of 2,804), were translated into English using Google Translate.
The genre of each song was retrieved using PyTube, a Python library which obtains the video title from the YouTube link in the dataset. We then created a Python script to run ChatGPT to extract the song name and artist from each video title. As a last step, we ran a Python script to obtain from ChatGPT the genre of the song using the song name and artist.
Lyrics analysis
Content analysis of songs’ lyrics is challenging due to their non-standard grammatical structure, the limited number of words in each song, and the repetition of words and phrases. Thus, common topic modeling approaches such as LDA (Kleedorfer et al., 2008; Sasaki et al., 2014; Fell et al., 2019, 2022; Nakai and Itoh, 2022) often fail to model the topic structure in corpuses of songs. We therefore implemented Non-Negative Matrix Factorization approach (NMF), an unsupervised topic modeling algorithm that simultaneously performs dimensionality reduction and clustering (George and Vasudevan, 2020). This model regards the song corpus as a collection of documents (M’sik and Casablanca, 2020), and classifies them into a predefined number of topics. Each topic contains all of the words in the corpus, with differing weights, arranged in descending order so that the most dominant words in each topic receive the highest weights.
As a preliminary stage, we performed a massive cleaning of the data to omit stop words (e.g., “is,” “the,” “chorus”), artists’ names, syllables that are not words (e.g., “haa,” “wawa”), punctuation marks, and numbers. We also performed a stemming procedure wherein inflected words are reduced to their roots (e.g., “likes” or “liking” became “like”). The cleaning process resulted in a lexicon of 6,000 words. For the lyric analysis, songs that were chosen by multiple respondents and repeated multiple times were removed so that each song occurs only once. This is important, so that the model is not biased toward songs that were chosen several times. Ultimately, the model was applied to 2,386 songs.
There is no established way of determining the number of topics. We tried various options between 1 and 30 topics, and calculated the coherence score for each such run. The coherence score is a measure of the probability of the topics be interpretable by humans. The higher the probability, the higher the likelihood that the topics can be interpreted (i.e., that people are able to comprehend the topic’s underlying theme) (gensim.models.CoherenceModel library). Based on the coherence graph, we set the number of topics at 15. See Supplementary Appendix C for the coherence score graph.
Interpretation of topics themes
While topic modeling algorithms do not suggest a procedure for interpreting the resulting topics, to facilitate interpretation and communication, we named the topics using a three-stage process. First, we presented the 10 words with the highest weights in each of the 15 topics to 40 MTurk respondents (paid $1 each), and asked them to suggest a title for the topic based on the commonality between the words. We then fed the words into ChatGPT and asked it to suggest titles using the prompt: “Find a common denominator between the following 10 words: …” We integrated the responses to obtain 15 topic titles. In the third stage, we conducted an MTurk experiment wherein we presented to 210 MTurk respondents the top 10 words of the topic, and asked them to choose between the suggested topic title and an alternative title, to be chosen randomly from the titles suggested for the other topics (this design makes sense, as in topic modeling, all of the words in the lexicon are part of all the topics, but in differing weights). The overwhelming majority of the respondents chose the suggested title over its alternative (see Supplementary Figure D1). Note that, despite this procedure, topic naming is not an inherent part of topic modeling, and alternative naming approaches could result in slightly different topic names.
Lyrics and well-being goals
We regressed the goals against the lyric topics. For each of the 15 topics i = {1, 2, … 15}, we ran a linear regression wherein the dependent variable had the frequency yij of the topic i in the coping song chosen by respondent j, and the independent variables were the respondents’ ratings of each of the five goals. The control variables include respondents’ demographics (age, gender, number of children, personal status), as well as their levels of religiosity and spirituality, “Religiosity” indicating prayer, reading religious texts, and attending worship “Spirituality level” meaning sense of meaning and purpose in life beyond material values as indicated on a 5-point scale: not at all…very strongly. We added the interaction terms between the goals and the degree of music’s importance to the respondent. The complete model for topic i is:
As the regression results created a very specific matching of topics to goals, and due to the large number of regressions, we ran a Placebo Permutation Test (Abadie and Gardeazabal, 2003) to verify that the results indeed indicated a specific matching and were not a random outcome. Therein, the independent variables in each observation—which were the respondent’s ratings of the five goals—were randomly permutated. Thus, we maintained the respondent’s average rating level while randomly permutating her responses across the five goals. These permutated results serve as a placebo test, i.e., running the regression on these permutated data should yield zero significant coefficients, which is indeed what we obtained.
Extracting song’s acoustic features
The literature suggests a wide range of high- and low-level acoustic features (Han et al., 2022). For better interpretability, we chose features that were found to influence the general emotional response to the song (Eerola, 2011, 2012). For example, a song with high volume, fast tempo, little roughness, simple harmony, and tendency toward the Major mode, would likely be perceived as “happy” and could be used to maintain one’s good mood. Loud, fast, heavy bass, and rough sounds in a song would likely be perceived as threatening or reflecting anger, and thus might help to vent negative emotions (Juslin et al., 2015; Karreman et al., 2017).
We used the Essentia (Bogdanov et al., 2013) audio analysis library to extract musical features, encompassing spectral, tonal, and rhythmic descriptors. Analysis was run on WAV files extracted from the YouTube links. Two types of acoustic features were extracted: Five features for a general characterization of the dataset, and another set of five features for testing the possible associations to well-being goals.
The features for the data set characterization included song-length, estimated key in which the song is written (e.g., E flat), mode (major versus minor), “danceability” (reflecting beat regularity and strength), and tempo. Danceability was measured on a scale of 0–3 with 3 being “more danceable.” We defined the tempo as “slow” for average of 80 BPM, “medium” as 80–120 BPM, and “fast” as over 120 BPM.
For the main analysis we extracted 161 low-level musical features that refer to spectral, tonal, and rhythm descriptors. As each feature has a differing range of values, we normalized the values to the range between 0 and 1. For our purposes, we chose to focus on 5 main feature groups: Loudness - the volume of the song; Mode - major/minor; Tempo - based on the beat duration (fast/slow); Harmony - the degree of harmony in the song; and Timbre—bright/dark sound. For each parameter, we calculated the average of their values. See Supplementary Appendix E for the exact features used for each parameter.
Acoustic features and well-being goals
For each of the 5 feature groups i = {1, …, 5}, we ran a linear regression wherein the dependent variable was the average value yij of the feature group i in the coping song chosen by respondent j; and the independent variables were the respondent’s rating of each of the five goals. The control variables included respondents’ demographics (age, gender, number of children, personal status), as well as their levels of religiosity and spirituality. The regression model for each feature group was:
Results
In the first part of the results, we provide descriptive information about the coping songs in terms of genre, artists, and acoustic features. We then present the 15 topics of the coping songs’ lyrics, emerging from the NMF topic modeling. Finally, we present results for the main question in which we were interested: the relationship between participants’ well-being goals and the lyrics and acoustic features of their nominated coping songs.
Coping songs’ characteristics
As shown in Figures 1 and 2, the distribution of coping songs spans a wide range of artists and musical genres. The popular genres were Latin, Pop, and Rock, where the strong presence of Latin might be attributed to the high representation of Spanish-speaking respondents.
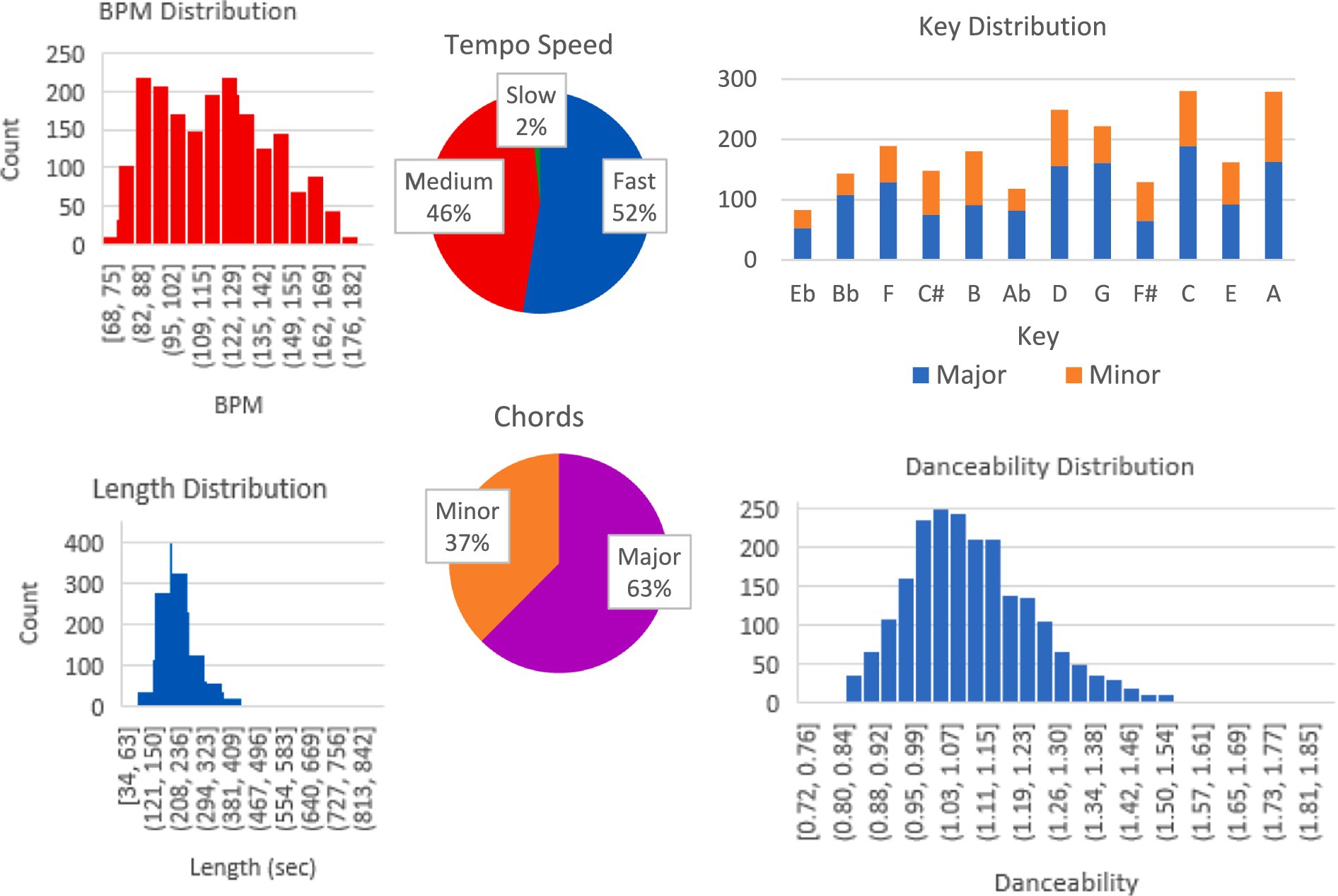
Figure 2. The distribution acoustic features of the nominated coping songs. The figure indicates that the coping songs varied greatly in their musical features, mostly dominated by a major key, medium-to-fast tempo, and low danceability.
Lyrics analysis: 15 emerging topics
We used the model on the extracted lyrics of the coping songs to identify 15 emerging topics that reflect the self-organization of the lyrics in the song corpus. Table 1 shows the 10 words with the highest weights in each topic. While extracted topics from topic modeling algorithms are not easily interpreted, and for many purposes do not require naming, herein, for ease of communication and better interpretability, we applied a 3-stage procedure using human encoders to identify the topic (see Methods for details on the identification procedure and validation experiments). The 15 topics named are: Soul, Time, Desire, Thought, Loneliness, Breakup, Love Story, Absolute, Ups and Downs, Optimism, Friendship, Life and Death, Belonging, Loss, and Night.
Topic distribution
Figure 3 presents the average distribution of topics across all songs (top). The most common topics are Soul and Time (songs such as “Natural” by Imagine Dragons; “No Rain” by Blind Melon; “Time After Time” by Cyndi Lauper). The bottom panel shows several examples of the distribution of the topics within a song (i.e., the normalized frequency of the topics in a song). The higher the value for a particular topic, the more dominant the topic is within that song. The song “Forever Young,” for example, is distributed 63% over the topic “Desire,” 15% over the topic Life and Death, and at far lower probabilities for the other topics (Figure 3B).
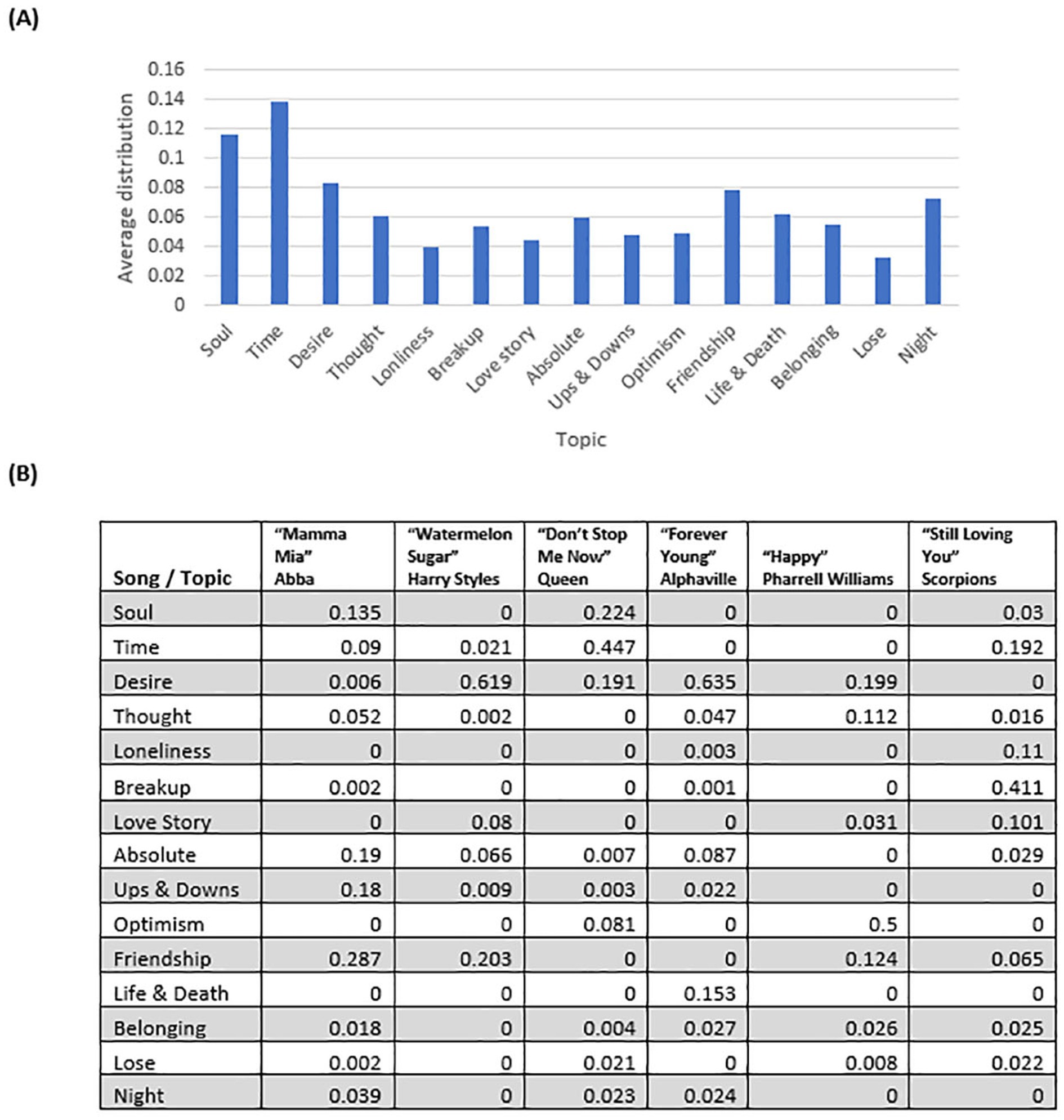
Figure 3. Topic distribution across all songs (A), and examples of the distribution of topics within a song (B).
The topic distribution for specific demographic segments and countries is presented in Supplementary Appendix D. Time and Soul are the two most dominant topics for all countries, together with Desire, Friendship, and Night. Countries differ in the ubiquity of the topic Life and Death. While it was relatively prevalent in Brazil, Colombia, and Spain, its occurrence was lower in other countries (Supplementary Figure D2). For age groups, while Time and Soul were common for most age groups, for the 64+ age group, other topics such as Friendship, Night, and Life and Death were more dominant than Soul (Supplementary Figure D3). Interestingly, the topic Belonging was dominant among participants with high level of religiosity (scoring 5 on the 1–5 scale) versus those who reported/described themselves as less religious (Supplementary Figure D4). No significant differences were found in the topic distribution across genders (Supplementary Figure D5).
Lyrics and well-being goals
The relationships between the well-being goals and the nominated coping songs were assessed using a set of 15 linear regressions over all respondents, where the dependent variable yij is the frequency of topic i in the song chosen by respondent j, and the independent variables are the ratings of the five goals reported by respondent j (see Methods for the formal model). Table 2 shows the significant coefficients ( in this 15 × 5 matrix, where the positive coefficients are highlighted in green and the negative in red, with the color intensity representing the intensity of the effect. The complete table (15 regressions with 5 goals and additional control variables) is presented in Supplementary Appendix D.
Table 2 reveals very specific matching between lyric topics and goals. For example, the goal Diversion (from the crisis) was found to be positively associated with the topic Loss, and negatively with Ups and Downs. Hence, the higher the respondent rated her goal as Diversion from the crisis, the higher the frequency of the topic Loss, and the lower the frequency of the topic Up and Down in the coping song s/he chose. The well-being goal of Enjoyment and maintaining good mood is negatively correlated with Life and Death, and Night. The goal of Reducing loneliness and creating a sense of togetherness is positively associated with Absolute (featuring words such as “always,” “never,” “everything,” and “nothing”), and negatively with Optimism. Finally, the goal of Connecting with myself and detachment from the surroundings is positively associated with the topic Belonging. While the interpretation of these relationships depends upon the interpretation of the topics (see Discussion), the core of this finding is this very specific match between song lyrics and specific well-being goals. To further validate that these relationships are not spurious, we conducted several tests, described in more detail in the Methods section.
Interaction with the respondent’s indicated importance of music
A possible moderator of influence that we tested was the perceived importance of music to the respondent. Granot et al. (2021) found that music’s importance in one’s life is strongly connected to the music < > well-being goals relationship, i.e., the variable of music’s importance was the strongest predictor of music’s rated effectiveness in reaching the respondent’s well-being goals, above and beyond musical education, the musical genre, and demographic variables.
Table 3 shows the significant coefficients obtained from the regressions for the interaction terms between the goals and the respondents’ response as to the music’s importance to them (“How important to you is music in general?”) (see Methods; the complete result table is in Supplementary Appendix F). Most of the significant coefficients are opposite in sign to the main effect in Table 2, indicating that music’s importance mostly moderates the goals < > topic relationships. A possible interpretation thereof is that music lovers (e.g., those who indicate that music is highly important to them), relate to the song as a whole, attributing higher relative importance to the acoustic features relative to the lyrics.
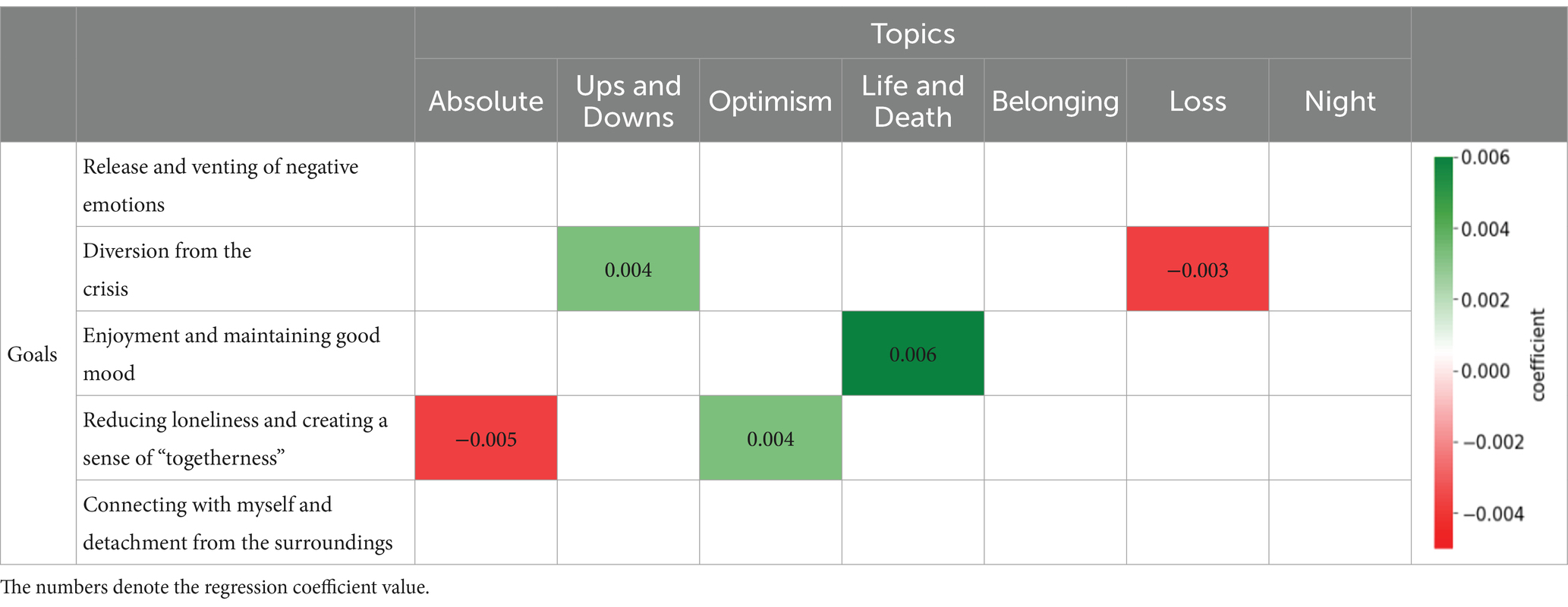
Table 3. The significant regression results of the interaction variables, where the number denotes the coefficient value.
Acoustic features and well-being goals
Similar to the lyrics, we ran a set of five linear regressions (for each musical features group), where the dependent variable yij is the frequency of group i in the song chosen by respondent j, and the independent variables are the ratings of the five goals reported by the respondent j, and additional control variables (see Methods for the formal model; see Supplementary Appendix E for the list of Essentia features used).
The acoustic features of music have been shown to have a strong effect on emotions (e.g., Schubert, 2004; Juslin and Lindström, 2010). Therefore, we could expect the coping song’s acoustic features to play a dominant role in achieving the well-being goals, in addition to, or even instead of, the lyrics. To test this, we assessed the connection between the respondents’ well-being goals and acoustic features of their nominated coping songs. Surprisingly, we did not find consistent relationships between well-being goals and coping songs’ acoustic features. The regression coefficients (described in Supplementary Appendix G), are mostly insignificant and do not exhibit any systematic pattern.
Note that this result does not imply that acoustic features are unimportant for well-being goals; it only means that on average, no clear pattern emerges across individuals. It could be that people differ greatly in their musical tastes and preferences, resulting in diverse acoustic features of the nominated coping songs, which blur the average choice. Moreover, the fact that the coping songs consist of many musical genres from various decades, decreases the probability of finding consistent acoustic patterns, especially for the dimension of valence (Eerola and Vuoskoski, 2012). Lyrics, on the other hand, are more explicit, i.e., the set of words that various people choose to describe loneliness is narrower than the spectrum of acoustic features that they might choose to resonate their lonely feelings. This narrower lyric choice led to the more consistent pattern in the lyrics vs. the acoustic features.
Discussion
In this paper, we explored the relationship between people’s choice of coping songs and their stated well-being goals. We used the unique circumstances of the first wave of the COVID-19 pandemic to assemble and analyze a large-scale, multinational dataset of 2,804 coping songs nominated by 5,619 individuals from 11 countries. We implemented a topic-modeling approach to identify 15 topics that span the songs’ lyrics space. These topics were not pre-defined, but rather self-emerged from the data. For interpretability, we termed them: Soul, Time, Desire, Thought, Loneliness, Breakup, Love Story, Absolute, Ups and Downs, Optimism, Friendship, Life and Death, Belonging, Loss, and Night. We found specific matching between respondents’ well-being goals and the incidence of the related topics in the lyrics of their nominated songs (Table 2).
Is there a unifying overarching pattern to the results in Table 2? While this paper does not aim to test specific hypotheses, two possible explanations come to mind. The first suggests that both the well-being goals and the coping songs were driven by a third factor related to the individual’s emotional state. This emotional state induces both the well-being goal, as well as the nominated coping song. For example, an individual who feels overwhelmed by the crisis might tend more to choose a song with the topic Loss, and at the same time might also feel the need to set a goal of Diversion from the crisis. When happy, one will set the goal of Enjoyment and maintaining good mood, and in turn, choose songs less related to Life and Death. Thus, the well-being goals and coping songs are connected via the listener’s underlying emotional state. This explanation is consistent with recent findings showing that lyrics of favorite songs are associated with personality traits and attachment style (thoughts, feelings, and behavior related to close relationships; Alaei et al., 2022), and hence may help to fill psychological needs (Qiu et al., 2019).
An alternative explanation suggests that well-being goals are regarded as a lever for moving from one emotional state to another. To help in this transition, and specifically, where the existing emotional state is already negative, people select a coping song that reflects their existing emotional state rather than their desired one. They can then engage in any of the emotion-focused strategies ranging from expression and ventilation, to reappraisal, acceptance, or even suppression (Gross, 2015). However, they do this within the safe environment of the songs rather than while confronting the real-life stressful situation. This is consistent with Scheff’s (1979) view of Catharsis that contains three stages: The first, evocation of the negative emotions through music (or drama, as in Aristotle); the second is the distancing of the listener as separate from the virtual protagonist, enabling cognitive 3rocessing that is at the heart of the therapeutic stage; and the third is the emotional release that may include crying, laughing, or other somatic reactions.
Our results support the latter explanation. For example, someone who seeks Diversion from the crisis likely feels immersed in/overwhelmed by the crisis. As per our findings, such an individual will tend to choose a negative valence topic such as Loss, which reflects the crisis, and is less likely to choose the topic of Ups and Downs, which may indicate an attempt to achieve a more optimistic outlook. Similarly, someone who seeks to Reduce Loneliness and Create a Sense of Togetherness, feels lonely, and thus will be less inclined to choose lyrics with Optimism as a dominant topic, and more inclined toward the topic Absolute, which includes existential words such as “never” or “nothing,” that actually reflect her existing emotional state. An individual whose goal is Connecting with myself and detachment from the surroundings might have chosen this goal due to feeling engulfed by those around her, while the topic Belonging, which conveys the theme of attachment to home or a community, reflects the situation of being surrounded by others.
For the goal Enjoyment and maintaining good mood, the existing and desired states are similar, and the song selection reflects both. Someone seeking to maintain her good mood will tend less to select lyrics with topics such as Life and Death and Night that might place her farther from a good mood.
These findings are consistent with Thoma et al. (2012) who found that given a choice, listeners prefer mood-congruent musical pieces, which can lead to positive results through providing a sense of meaning for the negative emotion, e.g., feeling less lonely (“I’m not the only one experiencing these feelings”), acceptance, and deeper processing of the emotion (Baltazar and Saarikallio, 2019; Garrido et al., 2022). More generally, according to the instrumental approach to emotion regulation, individuals may choose to experience negative emotions if they expect this to help them obtain a desired goal such as feeling anger in preparation for confrontation, or worry if they believe that avoidance is beneficial (e.g., Tamir and Ford, 2012).
Note that both these explanations depend upon the interpretation and naming of the topics. While such naming has been done in other contexts (Dzyabura and Peres, 2021), one should be cautious when relying on these interpretations so as to infer psychological mechanisms. The power of our findings is the specific matching between goals and lyrics topics. It is not necessary that all of the matchings be a result of the same coping strategy, and it could well be that individuals employ differing coping song strategies for differing well-being goals.
While the goal < > lyrics relationships show a clear, specific matching, we did not find clear matching between the well-being goals and the coping songs’ acoustic features. Accordingly, Table 3 indicates that the effect size is smaller for those who rated music as important in their lives. For those individuals, focusing on the musical elements distracts them from lyrics, thus weakening the lyrics < > goals relationship. It seems that while lyrics have a clearer/more consistent interpretation across listeners, music is by nature abstract, lending itself to a broad range of interpretations. Thus, while one individual might use an energetic tune to reach the goal of Diversion from the crisis, another might choose a song featuring a more serene musical ambience to achieve the same goal. This heterogeneity, however, does not converge into a unified average pattern of musical choice.
Limitations and future research
This study utilized a unique, large-scale ecological dataset of coping songs, employing a bottom-up thematic and acoustic analysis focused on emergent self-patterns. However, this approach has certain limitations. First, as an exploratory study, the scope of mood regulation goals was relatively limited. Future research could broaden these goals and incorporate questionnaires that assess individual differences in emotion regulation strategies and empathy. Additionally, our analysis was based on a single time point. Examining the use of the same coping song over time would provide insights into its dynamic role in mood regulation.
As noted in the Methods section, we limited our analysis to a select number of musical features. Including a more extensive set of features and employing more sophisticated analytical methods might reveal more stable patterns. The greatest challenge—and possibly the most ambitious—is finding a way to analyze music and lyrics as a unified expression, as it is likely the unique interaction between them that drives their impact.
Regarding lyrics analysis, we acknowledged the complexity in defining thematic topics meaningfully, a challenge further intensified by the need for translations in approximately one-third of the songs. Nonetheless, a separate analysis of non-translated lyrics showed consistent patterns. Future studies should continue investigating the role of lyrics in music listening and music therapy contexts.
Conclusion
In stressful times, people often find solace in coping songs, or songs that they listen to repeatedly to help them face hardship. Our data-driven lyrics analysis indicates that coping songs’ lyrics relate to specific topics, which we name as Soul, Time, Desire, Thought, Loneliness, Breakup, Love Story, Absolute, Ups and Downs, Optimism, Friendship, Life and Death, Belonging, Loss, and Night. These topics vary across cultures, ages, and religiosity. We found a significant association between certain topics and specific well-being goals. We demonstrate this for a large-scale dataset of 2,804 songs from various genres and eras, in multiples languages, collected from a large variety of individuals in 11 countries in the real-life circumstances of the first wave of the COVID-19 pandemic.
Our findings open a window onto a novel perspective on music classification, very different from the traditional acoustic genre-based categorization. We suggest that for emotional well-being purposes, classification based on lyrics topics might be valuable. Such classification could improve the matching between songs and affected individuals, and could enhance the effect of music through lyrics-driven pairing in music apps, playlist creation, and musical events.
Data availability statement
The data analyzed in this study is available at: https://osf.io/c6zge/?view_only=5ee154c047f743a8a779a601dc8c733e.
Ethics statement
The studies involving humans were approved by the Ethics Committee, Faculty of Humanities, The Hebrew University. The studies were conducted in accordance with the local legislation and institutional requirements. The participants provided their written informed consent to participate in this study.
Author contributions
AL: Investigation, Writing – original draft, Writing – review & editing, Formal analysis, Software, Visualization. RG: Investigation, Writing – original draft, Writing – review & editing, Conceptualization, Data curation, Funding acquisition, Methodology, Resources. RP: Conceptualization, Funding acquisition, Methodology, Project administration, Resources, Supervision, Writing – original draft, Writing – review & editing.
Funding
The author(s) declare that no financial support was received for the research, authorship, and/or publication of this article.
Conflict of interest
The authors declare that the research was conducted in the absence of any commercial or financial relationships that could be construed as a potential conflict of interest.
Publisher’s note
All claims expressed in this article are solely those of the authors and do not necessarily represent those of their affiliated organizations, or those of the publisher, the editors and the reviewers. Any product that may be evaluated in this article, or claim that may be made by its manufacturer, is not guaranteed or endorsed by the publisher.
Supplementary material
The Supplementary material for this article can be found online at: https://www.frontiersin.org/articles/10.3389/fpsyg.2024.1431741/full#supplementary-material
Footnotes
1. ^For example, an analysis of Spotify’s snapshot data of 32,833 popular tracks from 2020 indicates that the average instrumentalness (that is, the probability that a Spotify track does not contain vocals) is 0.084, i.e., the overwhelming majority of the popular Spotify tracks contain lyrics, see https://rstudio-pubs-static.s3.amazonaws.com/831415_8e26d20044e343efb68b42048a136bf6.html
References
Abadie, A., and Gardeazabal, J. (2003). The economic costs of conflict: a case study of the Basque Country. Am. Econ. Rev. 93, 113–132. doi: 10.1257/000282803321455188
Alaei, R., Rule, N. O., and MacDonald, G. (2022). Individuals’ favorite songs’ lyrics reflect their attachment style. Pers. Relat. 29, 778–794. doi: 10.1111/pere.12448
Ali, S. O., and Peynircioğlu, Z. F. (2006). Songs and emotions: are lyrics and melodies equal partners? Psychol. Music 34, 511–534. doi: 10.1177/0305735606067168
Baltazar, M., and Saarikallio, S. (2016). Toward a better understanding and conceptualization of affect self-regulation through music: a critical, integrative literature review. Psychol. Music 44, 1500–1521. doi: 10.1177/0305735616663313
Baltazar, M., and Saarikallio, S. (2019). Strategies and mechanisms in musical affect self-regulation: a new model. Music. Sci. 23, 177–195. doi: 10.1177/1029864917715061
Baltazar, M., and Västfjäll, D. (2020). Songs perceived as relaxing: Musical features, lyrics, and contributing mechanisms at international conference: Psychology and music: Interdisciplinary encounters. Belgrade: Faculty of Music, University of Arts, Belgrade.
Baltazar, M., Västfjäll, D., Asutay, E., Koppel, L., and Saarikallio, S. (2019). Is it me? Or the music? Stress reduction and the role of regulation strategies and music. Music Sci. 2:2059204319844161. doi: 10.1177/2059204319844161
Barradas, G. T., and Sakka, L. S. (2022). When words matter: a cross-cultural perspective on lyrics and their relationship to musical emotions. Psychol. Music 50, 650–669. doi: 10.1177/03057356211013390
Bogdanov, D., Wack, N., Gómez, E., Gulati, S., Herrera, P., and Mayor, O. (2013). “Essential: an open-source library for sound and music analysis,” in Proceedings of the 21st ACM International Conference on Multimedia, Barcelona, 855–858.
Brand, C. O., Acerbi, A., and Mesoudi, A. (2019). Cultural evolution of emotional expression in 50 years of song lyrics. Evol. Hum. Sci. 1:e11. doi: 10.1017/ehs.2019.11
Cabedo-Mas, A., Arriaga-Sanz, C., and Moliner-Miravet, L. (2021). Uses and perceptions of music in times of COVID-19: a Spanish population survey. Front. Psychol. 11:606180. doi: 10.3389/fpsyg.2020.606180
Carlson, E., Saarikallio, S., Toiviainen, P., Bogert, B., Kliuchko, M., and Brattico, E. (2015). Maladaptive and adaptive emotion regulation through music: a behavioral and neuroimaging study of males and females. Front. Hum. Neurosci. 9:466. doi: 10.3389/fnhum.2015.00466
Carlson, E., Wilson, J., Baltazar, M., Duman, D., Peltola, H. R., Toiviainen, P., et al. (2021). The role of music in everyday life during the first wave of the coronavirus pandemic: a mixed-methods exploratory study. Front. Psychol. 12:647756. doi: 10.3389/fpsyg.2021.647756
Carvalho, M., Cera, N., and Silva, S. (2022). The “ifs” and “hows” of the role of music on the implementation of emotional regulation strategies. Behav. Sci. 12:199. doi: 10.3390/bs12060199
Christenson, P. G., de Haan-Rietdijk, S., Roberts, D. F., and ter Bogt, T. F. (2019). What has America been singing about? Trends in themes in the US top-40 songs: 1960–2010. Psychol. Music 47, 194–212. doi: 10.1177/0305735617748205
Cook, T., Roy, A. R., and Welker, K. M. (2019). Music as an emotion regulation strategy: an examination of genres of music and their roles in emotion regulation. Psychol. Music 47, 144–154. doi: 10.1177/0305735617734627
De Witte, M., Spruit, A., van Hooren, S., Moonen, X., and Stams, G. J. (2020). Effects of music interventions on stress-related outcomes: a systematic review and two meta-analyses. Health Psychol. Rev. 14, 294–324. doi: 10.1080/17437199.2019.1627897
Dzyabura, D., and Peres, R. (2021). Visual elicitation of brand perception. J. Mark. 85, 44–66. doi: 10.1177/0022242921996661
Eerola, T. (2011). Are the emotions expressed in music genre specific? An audio-based evaluation of datasets spanning classical, film, population, and mixed genres. J. New Music Res. 40, 349–366. doi: 10.1080/09298215.2011.602195
Eerola, T. (2012). Modeling listeners’ emotional response to music. Top. Cogn. Sci. 4, 607–624. doi: 10.1111/j.1756-8765.2012.01188.x
Eerola, T., and Vuoskoski, J. K. (2012). A review of music and emotion studies: approaches, emotion models, and stimuli. Music Percep. Interdiscip. J. 30, 307–340. doi: 10.1525/mp.2012.30.3.307
Fell, M., Cabrio, E., Korfed, E., Buffa, M., and Gandon, F. (2019). Love me, love me, say (and write!) that you love me: enriching the WASABI song corpus with lyrics annotations. arXiv [Preprint] arXiv:1912.02477.
Fell, M., Nechaev, Y., Meseguer-Brocal, G., Cabrio, E., Gandon, F., and Peeters, G. (2022). Lyrics segmentation via bimodal text-audio representation. Nat. Lang. Eng. 28, 317–336. doi: 10.1017/S1351324921000024
Ferreri, L., Singer, N., McPhee, M., Ripollés, P., Zatorre, R. J., and Mas-Herrero, E. (2021). Engagement in music-related activities during the COVID-19 pandemic as a mirror of individual differences in musical reward and coping strategies. Front. Psychol. 12:673772. doi: 10.3389/fpsyg.2021.673772
Gabrielsson, A. (2001). “Emotions in strong experiences with music in P” in Music and emotion: Theory and research. eds. N. Juslin and J. A. Sloboda (Oxford: Oxford University Press), 431–449.
Garrido, S., Baker, F. A., Davidson, J. W., Moore, G., and Wasserman, S. (2015). Music and trauma: the relationship between music, personality, and coping style. Front. Psychol. 6:977. doi: 10.3389/fpsyg.2015.00977
Garrido, S., Du Toit, M., and Meade, T. (2022). Music listening and emotion regulation: Young people’s perspectives on strategies, outcomes, and intervening factors. Psychomusicol. Music Mind Brain 32, 7–14.
George, S., and Vasudevan, S. (2020). Comparison of LDA and NMF topic modeling techniques for restaurant reviews. Ind. J. Nat. Sci. 10, 28210–28216.
Granot, R., Spitz, D. H., Cherki, B. R., Loui, P., Timmers, R., Schaefer, R. S., et al. (2021). “Help! I need somebody”: music as a global resource for obtaining wellbeing goals in times of crisis. Front. Psychol. 12:648013. doi: 10.3389/fpsyg.2021.648013
Groarke, J. M., and Hogan, M. J. (2019). Listening to self-chosen music regulates induced negative affect for both younger and older adults. PLoS One 14:e0218017. doi: 10.1371/journal.pone.0218017
Grocke, D., and Wigram, T. (2006). Receptive methods in music therapy: Techniques and clinical applications for music therapy clinicians, educators, and students. London: Jessica Kingsley Publishers.
Gross, J. J. (2015). Emotion regulation: current status and future prospects. Psychol. Inq. 26, 1–26. doi: 10.1080/1047840X.2014.940781
Hamilton, J. B., Sandelowski, M., Moore, A. D., Agarwal, M., and Koenig, H. G. (2013). “You need a song to bring you through”: the use of religious songs to manage stressful life events. Gerontologist 53, 26–38. doi: 10.1093/geront/gns064
Han, D., Kong, Y., Han, J., and Wang, G. (2022). A survey of music emotion recognition. Front. Comp. Sci. 16:166335. doi: 10.1007/s11704-021-0569-4
Hanser, W. E., ter Bogt, T. F., Van den Tol, A. J., Mark, R. E., and Vingerhoets, A. J. (2016). Consolation through music: a survey study. Music. Sci. 20, 122–137. doi: 10.1177/1029864915620264
Hennessy, S., Sachs, M., Kaplan, J., and Habibi, A. (2021). Music and mood regulation during the early stages of the COVID-19 pandemic. PLoS One 16:e0258027. doi: 10.1371/journal.pone.0258027
Henry, N., Kayser, D., and Egermann, H. (2021). Music in mood regulation and coping orientations in response to COVID-19 lockdown measures within the United Kingdom. Front. Psychol. 12:647879. doi: 10.3389/fpsyg.2021.647879
Howlin, C., and Rooney, B. (2021). Patients choose music with high energy, danceability, and lyrics in analgesic music listening interventions. Psychol. Music 49, 931–944. doi: 10.1177/0305735620907155
Juslin, P. N., Barradas, G., and Eerola, T. (2015). From sound to significance: exploring the mechanisms underlying emotional reactions to music. Am. J. Psychol. 128, 281–304. doi: 10.5406/amerjpsyc.128.3.0281
Juslin, P. N., Liljeström, S., Västfjäll, D., Barradas, G., and Silva, A. (2008). An experience sampling study of emotional reactions to music: listener, music, and situation. Emotion 8, 668–683. doi: 10.1037/a0013505
Juslin, P. N., and Lindström, E. (2010). Musical expression of emotions: modelling listeners’ judgements of composed and performed features. Music. Anal. 29, 334–364. doi: 10.1111/j.1468-2249.2011.00323.x
Karreman, A., Laceulle, O. M., Hanser, W. E., and Vingerhoets, A. J. (2017). Effects of emotion regulation strategies on music-elicited emotions: an experimental study explaining individual differences. Personal. Individ. Differ. 114, 36–41. doi: 10.1016/j.paid.2017.03.059
Kleedorfer, F., Knees, P., and Pohle, T. (2008). Oh oh oh whoa! Toward automatic topic detection in song lyrics. ISMIR 14, 287–292.
Knight, W. E., and Rickard, N. S. (2001). Relaxing music prevents stress-induced increases in subjective anxiety, systolic blood pressure, and heart rate in healthy males and females. J. Music. Ther. 38, 254–272. doi: 10.1093/jmt/38.4.254
Krause, A. E., Dimmock, J., Rebar, A. L., and Jackson, B. (2021). Music listening predicted improved life satisfaction in university students during early stages of the COVID-19 pandemic. Front. Psychol. 11:631033. doi: 10.3389/fpsyg.2020.631033
Logan, B., Kositsky, A., and Moreno, P. (2004). Semantic analysis of song lyrics at. ICME 2, 827–830.
M’sik, B., and Casablanca, B. M. (2020). Topic modeling coherence: a comparative study between LDA and NMF models using COVID-19 corpus. Int. J. Adv. Trends Comput. Sci. Eng. 9, 5756–5761. doi: 10.30534/ijatcse/2020/231942020
MacDonald, R., Kreutz, G., and Mitchell, L. (2013). Music, health, and wellbeing. Oxford: Oxford University Press.
Martín, J. C., Ortega-Sánchez, D., Miguel, I. N., and Martín, G. M. G. (2021). Music as a factor associated with emotional self-regulation: a study on its relationship to age during COVID-19 lockdown in Spain. Heliyon 7:e06274. doi: 10.1016/j.heliyon.2021.e06274
Martínez-Castilla, P., Gutiérrez-Blasco, I. M., Spitz, D. H., and Granot, R. (2021). The efficacy of music for emotional wellbeing during the COVID-19 lockdown in Spain: an analysis of personal and context-related variables. Front. Psychol. 12:1193. doi: 10.3389/fpsyg.2021.647837
Mas-Herrero, E., Dagher, A., Farrés-Franch, M., and Zatorre, R. J. (2021). Unraveling the temporal dynamics of reward signals in music-induced pleasure with TMS. J. Neurosci. 41, 3889–3899. doi: 10.1523/JNEUROSCI.0727-20.2020
McFerran, K. S., and Saarikallio, S. (2014). Depending on music to feel better: being conscious of responsibility when appropriating the power of music. Arts Psychother. 41, 89–97. doi: 10.1016/j.aip.2013.11.007
Nakai, Y., and Itoh, T. (2022). “Classification and visualization of lyric collections using guided LDA at 2022,” in 26th International Conference Information Visualisation, 259–262. Vienna: IEEE.
Qiu, L., Chen, J., Ramsay, J., and Lu, J. (2019). Personality predicts words in favorite songs. J. Res. Pers. 78, 25–35. doi: 10.1016/j.jrp.2018.11.004
Randall, W. M., Rickard, N. S., and Vella-Brodrick, D. A. (2014). Emotional outcomes of regulation strategies used during personal music listening: a mobile experience sampling study. Music. Sci. 18, 275–291. doi: 10.1177/1029864914536430
Saarikallio, S., and Erkkilä, J. (2007). The role of music in adolescents’ mood regulation. Psychol. Music 35, 88–109. doi: 10.1177/0305735607068889
Sasaki, S., Yoshii, K., Nakano, T., Goto, M., and Morishima, S. (2014). LyricsRadar: A lyrics retrieval system based on latent topics of lyrics. Taipei: ISMIR, 585–590.
Schäfer, K., Saarikallio, S., and Eerola, T. (2020). Music may reduce loneliness and act as social surrogate for a friend: evidence from an experimental listening study. Music Sci. 3:2059204320935709. doi: 10.1177/2059204320935709
Scheff, T. J. (1979). Catharsis in healing, ritual, and Drama. Berkeley, CA: University of California Press.
Schubert, E. (2004). Modeling perceived emotion with continuous musical features. Music. Percept. 21, 561–585. doi: 10.1525/mp.2004.21.4.561
Silverman, M. J. (2009). The effect of single-session psychoeducational music therapy on verbalizations and perceptions in psychiatric patients. J. Music. Ther. 46, 105–131. doi: 10.1093/jmt/46.2.105
Sousou, S. D. (1997). Effects of melody and lyrics on mood and memory. Percept. Mot. Skills 85, 31–40. doi: 10.2466/pms.1997.85.1.31
Stratton, V. N., and Zalanowski, A. H. (1994). Affective impact of music vs. lyrics. Empir. Stud. Arts 12, 173–184. doi: 10.2190/35T0-U4DT-N09Q-LQHW
Tamir, M., and Ford, B. Q. (2012). When feeling bad is expected to be good: emotion regulation and outcome expectancies in social conflicts. Emotion 12, 807–816. doi: 10.1037/a0024443
ter Bogt, T. F., Vieno, A., Doornwaard, S. M., Pastore, M., and Van den Eijnden, R. J. (2017). “You’re not alone”: music as a source of consolation among adolescents and young adults. Psychol. Music 45, 155–171. doi: 10.1177/0305735616650029
Thoma, M. V., Ryf, S., Mohiyeddini, C., Ehlert, U., and Nater, U. M. (2012). Emotion regulation through listening to music in everyday situations. Cognit. Emot. 26, 550–560. doi: 10.1080/02699931.2011.595390
Vidas, D., Larwood, J. L., Nelson, N. L., and Dingle, G. A. (2021). Music listening as a strategy for managing COVID-19 stress in first-year university students. Front. Psychol. 12:647065. doi: 10.3389/fpsyg.2021.647065
Ziv, N., and Hollander-Shabtai, R. (2021). Music and COVID-19: changes in uses and emotional reaction to music under stay-at-home restrictions. Psychol. Music 50, 475–491. doi: 10.1177/03057356211003326
Keywords: songs, well-being, lyrics, emotion regulation, topic modeling, acoustic features analysis, COVID-19
Citation: Levy A, Granot R and Peres R (2024) Lyrics do matter: how “coping songs” relate to well-being goals. The COVID pandemic case. Front. Psychol. 15:1431741. doi: 10.3389/fpsyg.2024.1431741
Edited by:
Changiz Mohiyeddini, Oakland University William Beaumont School of Medicine, United StatesReviewed by:
Jonathan Berger, Stanford University, United StatesErikson Saragih, Universitas Sumatera Utara, Indonesia
Copyright © 2024 Levy, Granot and Peres. This is an open-access article distributed under the terms of the Creative Commons Attribution License (CC BY). The use, distribution or reproduction in other forums is permitted, provided the original author(s) and the copyright owner(s) are credited and that the original publication in this journal is cited, in accordance with accepted academic practice. No use, distribution or reproduction is permitted which does not comply with these terms.
*Correspondence: Roni Granot, cm9uaS5ncmFub3RAbWFpbC5odWppLmFjLmls