- 1Departamento Fundamentos de la Pedagogía, Facultad de Educación, Universidad Católica de la Santísima Concepción, Concepción, Chile
- 2Departamento de Ciencias, Universidad Técnica Federico Santa María, Concepción, Chile
- 3Departamento de Psicología, Facultad de Ciencias Sociales, Universidad de Concepción, Concepción, Chile
The importance of socioemotional teaching skills has been highlighted for its link with better academic, social, emotional, and behavioral results of students, as well as for its contribution to the work wellbeing, mental health, and prosperity of teachers. However, there are few instruments that measure these skills in teachers in the context of their professional practice. The purpose of this research was to analyze the psychometric properties of the socioemotional Skills Instrument for Teachers (SEMS-IT). An instrumental design and a sample of 853 Chilean secondary school teachers were used. To evaluate the dimensional structure of the instrument, a portion of the sample (n = 468) underwent a network estimation method with exploratory graph analysis (EGA) using a Gaussian GLASSO model. Then, in order to confirm the structural consistency and stability of the items, the analysis was replicated in a second sample (n = 385), where these results were additionally contrasted with those of the confirmatory factor analysis (CFA). The EGA findings confirmed a structure of four dimensions and 19 items in total: (a) cognitive management of teacher emotion (four items), (b) teacher empathic concern (four items), (c) teacher–student relationship (four items), and (d) adverse classroom climate (seven items), with a 7-point Likert scale response format. The CFA showed good and acceptable fit indicators, X2(171) = 354.546 (p < 0.001), Comparative Fit Index (CFI) = 0.971, Tucker–Lewis index (TLI) = 0.966, Root Mean Square Error of Approximation (RMSEA) = 0.061, and Standardized Root Mean Square Residual (SRMR) = 0.062. In conclusion, a tool for the assessment of teachers’ socioemotional skills, valid for school-based educational research, is provided. Implications of the findings at the theoretical and practical levels are discussed, as well as limitations and future projections for future research.
1 Introduction
1.1 Importance of socioemotional skills for teachers
Understanding and delimiting the concept of Social–Emotional Skills (SEMS) is not a simple task, as it is frequently used as a generic term that allows people to express, regulate and understand their thoughts, emotions, and behaviors in everyday situations and interactions with others, as well as to adapt to changing conditions (Schoon, 2021). In the present study, situated in the school context, SEMS are defined as the effective deployment of strategies that enable teachers to handle/manage complex situations, both interpersonally and intrapersonally, in a way that promotes their own well-being and that of their students, positively impacting socioemotional development—mutual/collective/group. Teachers’ SEMS have high associations with positive outcomes in social, behavioral, affective, academic, and student well-being outcomes (DeLay et al., 2016; Roorda et al., 2017). Recent studies have also shown that these skills function as a protective factor for teachers’ mental health, professional achievement, well-being, and thriving (Oliveira et al., 2021; Ornaghi et al., 2023; Sáez-Delgado et al., 2023; Sánchez-Pujalte et al., 2021; Serrano-Díaz et al., 2017; Zhang et al., 2023).
1.2 Models for the study of SEMS in teachers
There are several theoretical models in the socioemotional field (Fitzgerald et al., 2022; Muzzio and Strasser, 2022). Among the referent models are Mayer and Salovey’s emotional intelligence model 2000 (Mayer et al., 2000) and Bar-On (1997, 2006); both focused on the concept of emotional intelligence. Gross (1998) emphasizes the concept of emotional regulation. Bisquerra and Peréz (2007) is linked to the concept of emotional competencies. Collaborative for Academic, Social, and Emotional Learning (CASEL) (2008, 2013) includes the concept of social and emotional learning (SEL). Frameworks include that of Jones and Bouffard (2012); there is also Schonert-Reichl’s (2017) three-component framework on SEL that includes three distinct and interrelated dimensions (learning context, student SEL, and teacher SEL). Other models, such as the prosocial classroom model (Jennings and Greenberg, 2009), include five teaching consciences as a framework for understanding teachers’ SEC, where it is emphasized that with awareness comes competence (Rodriguez et al., 2020); the Social and Emotional Competence School Model of Collie (2020); and the DOMASEC-Domains and manifestations of socio-emotional competences of Schoon (2021), which focus specifically on social–emotional competences (SEC).
The delimitation and characterization of these models can be done by considering two central aspects. First, a key aspect of the models is the areas or contexts in which they have been formulated and/or mainly implemented, some of which have been applied in a variety of contexts, such as labor, clinical, educational, and organizational. Second, another key aspect of the models is the central construct that they define since the diversity and breadth of principles on which they are based are recognized, which vary between models of capacity, competence, trait and ability. However, the ability models stand out for having the potential to learn, that is, they allow socioemotional development, versus those models, such as trait models, that include factors that do not really change over time (they are stable), and, therefore, will not change with an intervention. The above background delimited the selection of specific theoretical models for the design of the instrument of this study. The models of Jennings and Greenberg (2009), Collaborative for Academic, Social, and Emotional Learning (CASEL) (2008, 2013), and Collie (2020) were mainly considered because, on the one hand, they describe integral socioemotional skills and, on the other hand, they have been focused on educational and school contexts, highlighting the importance of adapting and applying these theoretical models according to the needs and characteristics of the environment in which they are to be implemented, in this case specifically, focused on the socioemotional skills needed by teachers for the success of their professional practice.
1.3 Instruments used for the measurement of teachers’ SEMS
In empirical studies on teachers’ SEMS, it is possible to identify the use of different self-report instruments, among which the following stand out: Trait Meta-Mood Scale (TMMS), based on the model of Salovey and Mayer (1990), which assesses emotional intelligence in the dimensions of emotional attention, emotional clarity, and emotional repair; Petrides (2009) Trait Emotional Intelligence Questionnaire (TEIQue), an instrument that combines scales of emotion regulation and relationship management skills, which measures the degree to which subjects perceive themselves as controlling their own emotions, how they influence other people’s feelings, how they assert themselves, and how they establish positive relationships with others; Yoder’s (2014) Self-Assessing Social and Emotional Instruction and Competencies which measures teaching practices that affect students’ SEL and own SECs to implement those teaching practices with students; The Social and Emotional Competencies Questionnaire (SEC-Q) (Zych et al., 2018), which includes four scales (self-awareness, self-awareness and motivation, social awareness and prosocial behavior, and responsible decision making); and the EduSEL which is a multidimensional self-report instrument to assess educators’ SEL competencies (Hemi and Kasperski, 2023). A review of these instruments reveals significant limitations related to the age group and context for which they were originally designed. Although applied to teachers, many were originally designed for adolescents and adults in general e.g., (see Salovey et al., 1995; Bar-On, 1997; Mayer et al., 2002; Mayer et al., 2003; Zych et al., 2018), others were focused on clinical populations e.g., (see Bar-On, 1997; Gross and John, 2003; Pérez-Escoda et al., 2021). However, instruments specifically developed for teachers, addressing SEL and essential teaching skills, are limited/scarce e.g., (see Hemi and Kasperski, 2023; Aldrup et al., 2020; Yoder, 2014) and mostly focused on general SEL, but not on crucial, specific, delimited skills necessary for teaching success. Thus, it can be argued that there is a need to validate specific instruments for teachers that include variables relevant to the educational context and items that fit the classroom routine.
A relevant aspect to consider is that most psychometric studies in the area of educational psychology have used the traditional exploratory and confirmatory factor analyses, but recently, the so-called network analysis models, also known as network psychometrics, have caught the interest of researchers (Christensen et al., 2019). One of the potential differences between models that include latent variables is that they conceive of observable variables as the product of unobservable latent factors, whereas network analysis models implement graph theory to construct a network that can represent the different associations between observable variables (Cai et al., 2020). Therefore, the structural characteristics of network models exponentially enrich the possibility of revealing the multiple relationships between variables in a dynamic system, providing a new perspective for the visualization and study of various current phenomena in educational psychology. This type of analysis responds assertively to the complexity of the analyzed variables of human beings and its valuable contributions favor modelling to improve the proposal of psychometric instruments, in this case, to understand teacher SEMS (Borsboom, 2022).
1.4 Essential teacher’s SEMS
It is indisputable that teaching SEMS positively impacts the socioemotional development of their students within the school environment, acting as role models in social and emotional skills, norms, and behaviors. School, as the primary socialization environment after home, underscores the importance of educators promoting healthy interactions and effectively managing the learning environment (Schoon, 2021; Lechner et al., 2019; Lee et al., 2016). Although there is a wide variety of SEMS, among those that are critical for teachers, the following inter- and intrapersonal skills stand out: (a) cognitive management of teacher emotion (CMTE), (b) teacher empathic concern (TEC), (c) teacher–student relationship, and (d) Adverse Classroom Climate (ACC; Lechner et al., 2019; Martínez-Yarza et al., 2023; Scheirlinckx et al., 2023).
1.4.1 Cognitive management of teacher emotion
Gross’s (1998, 2002) model of emotion regulation illustrates how people influence their emotions, determining which emotions they experience and when and how they express them. The importance of cognitive management of emotions is highlighted here because it addresses one of the most reported aspects of emotion regulation, that is, emotional experience (Gross, 2001). This allows the adjustment of emotional responses and is considered a key aspect in understanding people’s everyday emotional regulation (Gross and John, 2003). In this study, it has been defined as the use of cognitive strategies (reappraisal) deployed by teachers to manage emotional responses in the context of their teaching. It is considered an intrapersonal skill.
Empirical evidence confirms the positive role of CMTE implemented in classrooms. A study involving 189 high school teachers in Germany showed that CMTE was related to teachers’ experience of positive emotions, such as enjoyment (Lee et al., 2016); another study on 205 high school teachers also in Germany confirmed the importance of teachers’ CMTE in predicting their behaviors for effective classroom instruction (Seiz et al., 2015). However, research on how teachers regulate their emotions is still scarce, and little empirical evidence is available. This is surprising, as how teachers regulate their emotions has been recognized as a fundamental aspect in improving their effectiveness (Gross, 2002), their personal and professional success, equipping them to show empathy toward their students, highlighting its indisputable importance in school contexts (Gross, 2001; Fan and Wang, 2022).
1.4.2 Teacher empathic concern
Empathy is understood as the ability to orient and respond to the thoughts, actions, feelings, and experiences of others (Coke et al., 1978; Decety and Cowell, 2014; Matravers, 2014); multidimensional in nature given that it integrates cognitive, socioemotional, and behavioral components; therefore, it can influence interpersonal and social relationships (Landler-Pardo et al., 2022; Shamay-Tsoory et al., 2009). Among the various types of empathy, empathic concern has been defined as an affective, sensitive, and compassionate response characterized by the fostering of altruistic motivation to support or help people (Batson et al., 2007; Fry and Runyan, 2018; Winczewski et al., 2016). In this study, TEC, has been defined as an emotion oriented toward students, activated by perceiving that they need something, triggering motivation, prosocial behavior, and a compassionate disposition to increase their well-being during class.
In educational settings, TEC plays an indispensable role (Landler-Pardo et al., 2022) since it is linked to the promotion of healthy intergroup relationships in the classroom (Fry and Runyan, 2018); it also allows for the deployment of empathic behaviors in complex interactions, being fundamental to consolidate strong and positive relationships with students (Landler-Pardo et al., 2022); this is why it is considered a significant component of teachers’ social–emotional learning, an important prerequisite for high-quality teacher–student interactions, development and positive outcomes of their students (Aldrup et al., 2022).
1.4.3 Teacher–student relationship
Teacher–Student Relationship (TSR) are dyadic social processes characterized by continuous and feedback two-way interactions between teachers and students in classrooms that provide insight into how teachers and students feel about each other and how teachers and students perceive their shared relationships (Brinkworth et al., 2018; Cooper and Miness, 2014; Wentzel, 2022). In this study, it has been defined as an interpersonal teacher’s skill. In educational contexts, TSR is recognized as a crucial aspect of making schools inclusive and committed to providing a learning environment for healthy development and optimal learning support for all students regardless of achievement, language, readiness for learning and behavior, or disability (Pastore and Luder, 2021; Wentzel, 2022).
Empirical evidence recognizes TSR as the core of the school experience (Gehlbach et al., 2012). A longitudinal study with 1,088 German high school students showed that positive teacher–student relationships improved satisfaction of competence, relatedness, and autonomy needs (Bakadorova and Raufelder, 2018). A systematic review examining 46 studies found that high-quality teacher–student relationships are linked to higher school engagement, reflected in better academic performance, higher attendance, and decreased disruptive behaviors and dropout rates (Quin, 2017). Therefore, a strong and positive TSR is critical to emotional well-being, academic success, and engagement in learning, acting as an essential pillar for an educational environment where mutual support and understanding foster a climate conducive to learning and personal development.
1.4.4 Adverse classroom climate
Classrooms are complex social systems, and the different situations generated within them are often multicomponent challenges for teachers (Pianta et al., 2012). ACC is defined in this study as the awareness of challenging situations with students in a given class according to the teacher’s self-report (behaviors, performances and/or attitudes) and corresponds to a teacher’s interpersonal skill.
Empirical evidence has shown that lower levels of conflict in the teacher–student relationship was related to higher levels of student enjoyment of learning processes (Clem et al., 2021). A study involving 267 students and 93 teachers conducted classroom climate observations revealing that emotional support favors the development of the student–teacher relationship, where students who received greater emotional support experienced closeness in the relationship and decreased conflicts with peers (Moen et al., 2019). Another study in a sample of 3,225 students from schools in Germany and Switzerland evidenced that classroom climate had a direct positive effect on counter-talk and social skills (Wachs et al., 2023). Therefore, the challenge of dealing with adversity in the classroom and the need to promote a positive climate highlight the crucial importance of teacher social–emotional development in the educational context.
1.5 The present study
The growing incorporation of social–emotional programs in schools underscores the need for precise methods to assess Social–Emotional Skills (SEMS) in both students and teachers. Although self-report instruments have been used for this purpose, there is a significant gap in tools specifically designed to capture the complexity of SEMS in the teaching environment, considering factors such as age, educational context, and the specific role of teachers in social–emotional development. This study set out to examine the psychometric properties of the SocioEmotional Skills Instrument for Teachers (SEMS-IT), aimed at assessing essential SEMS in teachers.
2 Method
An instrumental design was used to carry out the study, which, according to the classification of Ato et al. (2013), considers the analysis of the psychometric characteristics of measurement scales.
2.1 Sample
The total study sample consisted of 853 teachers from schools in southern Chile, with an age of M = 36.64 (SD = 10.20). Regarding sex, 610 (71.5%) were female, 229 (26.9%) were male, and 14 (1.6%) preferred not to state their sex. Two samples were used for the present study: sample 1 was composed of 468 teachers, age M = 35.79 (SD = 9.99), of whom 343 were women, 120 were men, and five indicated having another sex or preferred not to say. Regarding the declared contract hours, they had, on average, M = 35.51 (SD = 13.74) working hours per week and finally, regarding teaching experience, the participants indicated an M = 9.65 (SD = 8.74) years; and sample 2 was composed of 385 teachers of age M = 37.95 (SD = 9.79), of whom 267 indicated being female, 109 indicated being male and nine indicated having another sex or preferred not to say. Regarding reported contract hours, participants worked M = 39.89 (SD = 6.58) hours per week. Finally, participants had an average of 11.64 (SD = 8.91) years of experience.
2.2 Instrument
2.2.1 Preliminary construction of the SocioEmotional Skills Instrument for Teachers
For the design process of this instrument, the steps for instrument construction in research (Muñiz and Fonseca-Pedrero, 2019) and the guidelines of the International Test Commission (Hernández et al., 2020) were followed. Exhaustive reviews of the specialized literature were conducted to systematize the available evidence on the history, concept, instruments, and models available on Teachers’ Social–Emotional Competence (Lozano et al., 2021, 2022, 2023; Scheirlinckx et al., 2023). From these literature reviews, dimensions and items were proposed considering specific contributions of some related instruments (Davis, 1983; Gross and John, 2003; López-Angulo et al., 2020; Pagano and Vizioli, 2021; Péloquin and Lafontaine, 2010; Zhou and Ee, 2012). As a result of this process, the first version of the SocioEmotional Skills Instrument for Teachers (SEMS-IT) was constituted as a 7-point Likert-type scale (where 1 is never and 7 is always), with 29 items distributed in four dimensions: (1) CMTE (six items); (2) TEC (eight items); (3) TSR (eight items); (4) ACC (seven items).
2.2.2 Evidence of validity of the SEMS-IT
The validity of an instrument is obtained when evidence and theory allow the adequate interpretation of its scores for the purpose for which it was constructed (AERA, 2014; Taherdoost, 2016); for this reason, recommendations were followed to evidence four sources of instrument validity (see Figure 1).
2.2.3 Evidence of validity of consequence: ethics committee analysis
The instrument is part of a larger research project. It was submitted to the Ethics Committee of the University of affiliation of the first author of this article. The committee reviewed the instrument and the ethical protocols associated with its implementation in the target sample, to ensure the corresponding safeguards for the integrity of the participants. The respective approval was obtained.
2.2.4 Evidence of content validity: analysis by expert judges
This process considered the participation of seven doctors from the areas of Education and Psychology, four from Chile and three internationals (Mexico, Ecuador, and Spain). Each expert judge evaluated the instrument using a matrix with specific criteria (pertinence, relevance, clarity, and sufficiency of each item) to measure the teaching SEMS construct and its four proposed dimensions. The inter-rater consistency results were high (Cohen’s Kappa >0.7) in the four specified criteria and all its items (Pedrosa et al., 2013; Polit et al., 2007). In addition, the wording of the items was improved when this was suggested by more than one judge.
2.2.5 Evidence of response format validity: cognitive interviews
This process involved the development of cognitive interviews with nine secondary education teachers from different specialties and three schools selected by accessibility. The purpose of the cognitive interview was the identification of comprehension and/or writing difficulties of the different items (Castillo-Díaz and Padilla, 2013; Wolcott and Lobczowski, 2021). The interviews followed a protocol that considered the presentation of the study, the objective of the research project, confidentiality of personal information, instructions, clarification of doubts, and finally, the implementation of the interview. In the instruction stage, it was explained to the teachers that as they were answering the instrument, they should do so through the “thinking aloud” procedure, making observations, comments, suggestions, or consultations on the wording and/or content of the items, understanding of the instructions or the scale for answering. As a result of this process, minor changes were made to specific words in the items, such as some synonyms or adding examples in parentheses to make it easier to understand the instrument.
2.2.6 Evidence of validity of factorial structure
For the third phase of structural validity of the SocioEmotional Skills Instrument for Teachers (SEMS-IT), two samples of teachers were used. The first sample (n = 468) was used to carry out the exploratory graph analysis (EGA). The second sample (n = 385) was used to conduct confirmatory factor analysis (CFA). The results section of this study presents in detail the structural validity process of this instrument.
2.3 Data collection procedure
The participating schools were contacted, and the principals and their respective management teams were informed about the purposes of the research and their authorization was requested. Subsequently, the schools that agreed to participate provided a contact from the management team to coordinate the presentation of the study and extend the invitation to participate to the teachers. In face-to-face meetings and/or through the Zoom platform, as decided by each school, teachers were informed of the details of the research and a deadline was coordinated for sending and responding to the instrument. The instrument was applied in online format using the surveymonkey tool. The link was sent by e-mail. The average response time was 15 min. The data were collected during the second semester of the year 2023. The questionnaire sent to the teachers had three sections: the first one displayed the informed consent; then, those who agreed to participate displayed the second part on sociodemographic data of the teachers such as for example, sex, age, employment contract (hours per week), work experience (years); and finally, the third part was the SEMS-IT instrument. All the procedures were authorized by the scientific ethical committee of the sponsoring institution of the main author of this study, guaranteeing the conditions of confidentiality, voluntariness and protection of the data obtained.
2.4 Data analysis procedure
A descriptive statistical analysis of the total sample was performed. Subsequently, the sample was divided into two groups, the first to examine the structure of the SEMS-IT (n = 468) and the second for its confirmation (n = 385). Statistical-descriptive analyses were performed on both samples, and the lack of differences between them was verified. First, the Kolmogorov–Smirnov test with the Lilliefors modification (samples larger than 50 subjects) was used to verify the normality assumption. Then, the homoscedasticity assumption was verified using Levene’s test. Considering that the results of this process showed that the normality assumption was not met, and neither was the homoscedasticity assumption fully met, the comparison of the two samples was performed with the Yuen test (Wilcox, 2012), being the robust alternative to the Student’s T-test. To estimate the effect size, we used the one proposed by Algina et al. (2005), which is the robust option to Cohen’s d and is interpreted in the same way. To identify the factor structure of the instrument with sample 1 (n = 468), we first checked the local independence assumption, which states that the variables (items) are not related after conditioning on a latent variable (redundancy), in order to avoid, for example, model misspecification and biased parameters. For this, we used the UVA Function of the EGAnet package that uses an EBICglasso.qgraph network estimation method and the weighted topological overlap (wTO) (Christensen et al., 2023). EGA was then implemented with the EGAnet library (Golino and Christensen, 2021), verifying dimensionality using a Gaussian GLASSO model and Louvain’s algorithm that determined the number of communities through a visual representation of regularized partial correlations; this procedure has demonstrated more accuracy than other exploratory methods (Christensen et al., 2020).
In the framework of network analyses, reliability was examined using two estimates (Christensen et al., 2020): (a) structural consistency, which is the proportion of times the number of dimensions derived from the initial EGA was exactly recovered in the replicated bootstrap samples and (b) item stability, which is the number of times each item is replicated within the empirical dimension and in other dimensions identified in the replication networks. Both procedures were performed with the EGAnet library and the bootEGA function (Golino and Christensen, 2021) with GLASSO estimation considering 1,000 replicates and the LE eigenvalue algorithm. For the interpretation of structural consistency and item stability, a minimum value of 90% was used; that is, the dimension was expected to replicate exactly in 90% of the bootstrap samples, and the items were expected to replicate in at least 90% of the derived dimensions (Golino and Christensen, 2021). Next, the fit of the structure suggested by EGA was verified by CFA with the CFA function of the EGAnet package (Golino and Epskamp, 2017). WLSMV estimation was used, which is suitable for scales consisting of ordinal-level items. The model was evaluated using chi-square (χ2), CFI, TLI, RMSEA, and SRMR. The criteria used to adequately evaluate the model were as follows: (a) χ22 p < 0.05, (b) CFI and TLI greater than 0.9 correspond to an acceptable fit and above 0.95 to a good fit, and (c) RMSEA and SRMR with values less than 0.08 indicate an acceptable fit and less than 0.06 a good fit (Hu and Bentler, 1999). With the second sample (n = 385), EGA and bootEGA resampling were performed again to confirm the structural consistency and stability of the items. Finally, the results of these analyses were checked with the CFA analysis.
3 Results
3.1 Descriptive analysis of the samples
The possibility that the samples were disproportionate according to sex was analyzed with the chi-square test. The test result was X2 (3, N = 853) = 3.55, p = 0.31. Therefore, there was no evidence that the samples were unbalanced according to sex (see Table 1).
Also, to ensure equivalence between the data of the samples, first, a descriptive analysis was performed for each item of the instrument and the variables age, contract hours, and experience. Then, to evaluate significant differences, the assumption of normality was verified, identifying that all the variables in both samples did not comply with a normal distribution (p < 0.05). Subsequently, the homoscedasticity assumption was verified, in this case, the variables that did not meet this assumption were: (1) EC: employment contract (hours per week), (2) TSR-17, (3) CMTE-3, and (4) CMTE-5. With this background and considering that sample 1 was larger than sample 2, the Yuen test was performed, which is a robust option of the Student’s t-test. For the effect size this test uses the one proposed by Algina et al. (2005), an alternative to Cohen’s d and interpreted on the same scale. The results showed significant differences in the variables: (1) age T (487.64) = 2.69, p < 0.01, ES = 0.15; (2) employment contract (hours per week) T (478.95) = 2.96, p < 0.01, ES = 0.16, and (3) work experience (years) T (493.24) = 3.12, p < 0.01, ES = 0.16. In all these cases, differences of a small magnitude were identified. On the other hand, all the items of the instrument did not show significant differences between the samples (see Table 2).
3.2 Results of exploratory graph analysis on sample 1
Exploratory graph analysis with the GLASSO network estimation method and the Louvain community detection algorithm estimated four factors (Figure 2), representing the theoretical factors. Community 3 is consistent with the dimension “teacher empathic concern” (TEC1, TEC2, TEC3, TEC4, TEC5, TEC6, TEC7, TEC8). Community 4 is consistent with the dimension “cognitive management of teacher emotion” (CMTE1, CMTE3, CMTE5, CMTE7, CMTE8, CMTE10). Community 1 corresponds almost entirely to the dimension “teacher–student relationship” (TSR9, TSR11, TSR13, TSR14, TSR15, TSR17, TSR23), except for item TSR12, which was assigned to community 2, which corresponds theoretically to the dimension Adverse Classroom Climate (ACC10, ACC16, ACC18, ACC19, ACC20, ACC21, ACC22).
The item that presents this problem (TSR12) has the following wording “My students are uncomfortable when I give them physical affection (such as pats on the shoulder, a handshake)” one explanation may be the conflict and risks associated with the current context where physical contact could be interpreted as harassment, making it difficult for teachers to respond to this item.
The UVA analysis showed evidence of 2 pairs of items with large to very large redundancy (wTO > 0.30), four pairs of items with moderate to large redundancy (wTO > 0.25) and three pairs of items with small to moderate redundancy (wTO > 0.20). Considering this background, the strategy of eliminating redundant items was used.
With this background of theoretical correspondence and local redundancy in a multivariate dataset, it is decided to eliminate the items: TEC2, TEC3, CMTE7, TEC7, TEC8, CMTE8, TSR15, and TSR12 (see Figure 3).
To evaluate the stability of these dimensions and their reproduction in a resampling, the bootEGA function with 1,000 iterations was used. Due to the ordinal nature of the Likert scales, a nonparametric resampling was used, where the network structure obtained from the median of the iterations has four dimensions, like the empirical EGA (Figure 3).
The first review of stability was performed by reviewing the descriptive statistics (Table 1). It could be observed that the median was four dimensions, the same as that reflected by the empirical EGA, together with a narrow confidence interval (95% CI [3.76, 4.24]), as a complementary measure, the frequency of each dimensional solution can be observed (Table 3).
With the frequency analysis, four dimensions were identified 98.5% of the time, which corresponds to 985 times out of 1,000 bootstrap resamples; on the other hand, three dimensions were identified 1.5% of the time, or in 15 out of 1,000 bootstrap resamples. These results suggest that the four-dimensional solution has good stability (see Table 4).
To obtain a better understanding of the stability of each dimension, the structural consistency, or the frequency with which the empirical EGA dimension replicated exactly in the Bootstrap resampling was calculated. The structural stability result shows that dimension 1, which represents TSR, presents a stability (0.782), being the only one lower than 0.9 (Table 5).
Then, the stability values of the items in the empirical dimensions of the EGA were observed (zero values have been eliminated to facilitate interpretability); items TSR14 and TSR9 coincided 86.5 and 81.5%, respectively, with their theoretical dimension (dimension 1), which is considered unstable, on the other hand, the items of dimension 2 representing the ACC were the only ones that in their totality presented a stability of 100% with their theoretical dimension (see Table 6). These results suggest that, although, in general, the items are associated with their theoretical dimension, there is evidence of unstable items that cause problems with the consistency of the SEMS-IT closeness dimension. With this background, we proceeded to eliminate items TSR9 and TSR14.
Finally, in this last SEMS-IT solution of 19 items (see Figure 4), it could be observed that both the EGA analysis and the bootEGA resampling presented similar four-factor structures, where the dimension 1 corresponding to TSR was composed of the items TSR11, TSR13, TSR17 and TSR23; dimension 2 corresponding to ACC and was composed of items ACC10, ACC16, ACC18, ACC19, ACC20, ACC21, and ACC22; dimension 3 corresponding to TEC and was composed of items TEC1, TEC4, TEC5, and TEC6; finally dimension 4 corresponding to CMTE and was composed of items CMTE1, CMTE3, CMTE5, and CMTE10.
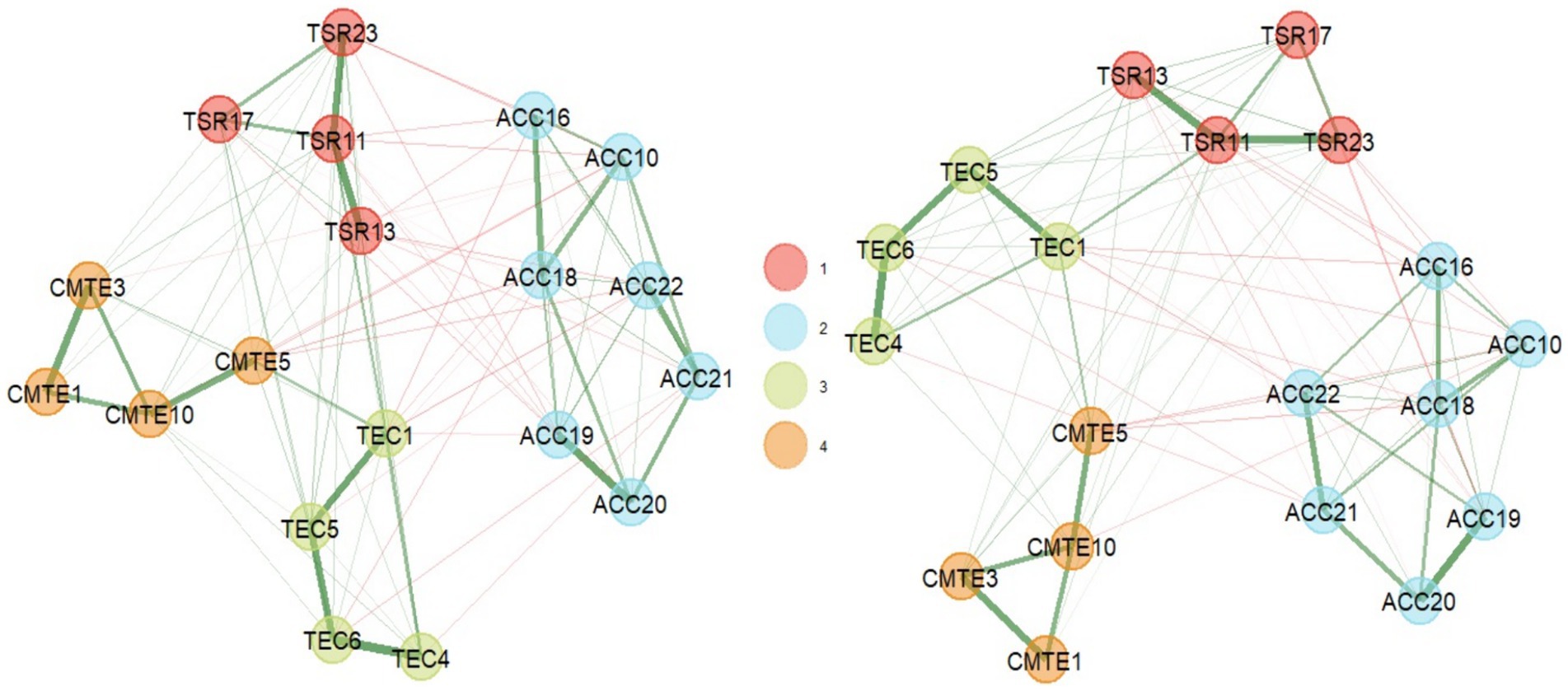
Figure 4. Dimensionality results of the SEMS-IT in its final solution (19 items), in EGA (left) and bootEGA (right).
Regarding the stability of the dimensions, the frequency analysis indicated that the four-dimensional solution replicated 99.3% of 1,000 resamples, and the structural consistency or the frequency with which the empirical EGA dimension replicated exactly on resampling was 0.999 for dimensions 1 and 2, respectively, dimension 3 replicated 0.994 and dimension 4 replicated 0.988.
Then, when observing the stability of the items, it could be seen that the items were replicated in their community at least 99% of the time in their dimension (Figure 5).
Finally, the fit of the structure suggested by EGA was estimated by CFA, using the CFA function of the EGAnet package, where the model presented good fit indicators, X2 (171) = 340.926***, CFI = 0.982, TLI = 0.979, RMSEA = 0.05, and SRMR = 0.055 (Figure 6).
3.3 Results of the exploratory graph analysis in sample 2
Finally, these findings were analyzed in a second sample with the items of the final solution that were selected. A new EGA was performed with the GLASSO network estimation method and the Louvain community detection algorithm, which confirmed the four-factor structure (Figure 1), consistent with the theoretical factor representation. The stability of these dimensions, as in the first part, was evaluated with the bootEGA function with 1,000 iterations, where the network structure obtained from the median of the iterations also had four dimensions, like the empirical EGA (Figure 7) has the new numbering of the items, and the adjustment of this numbering can also be seen in Appendix 1 the final scale.
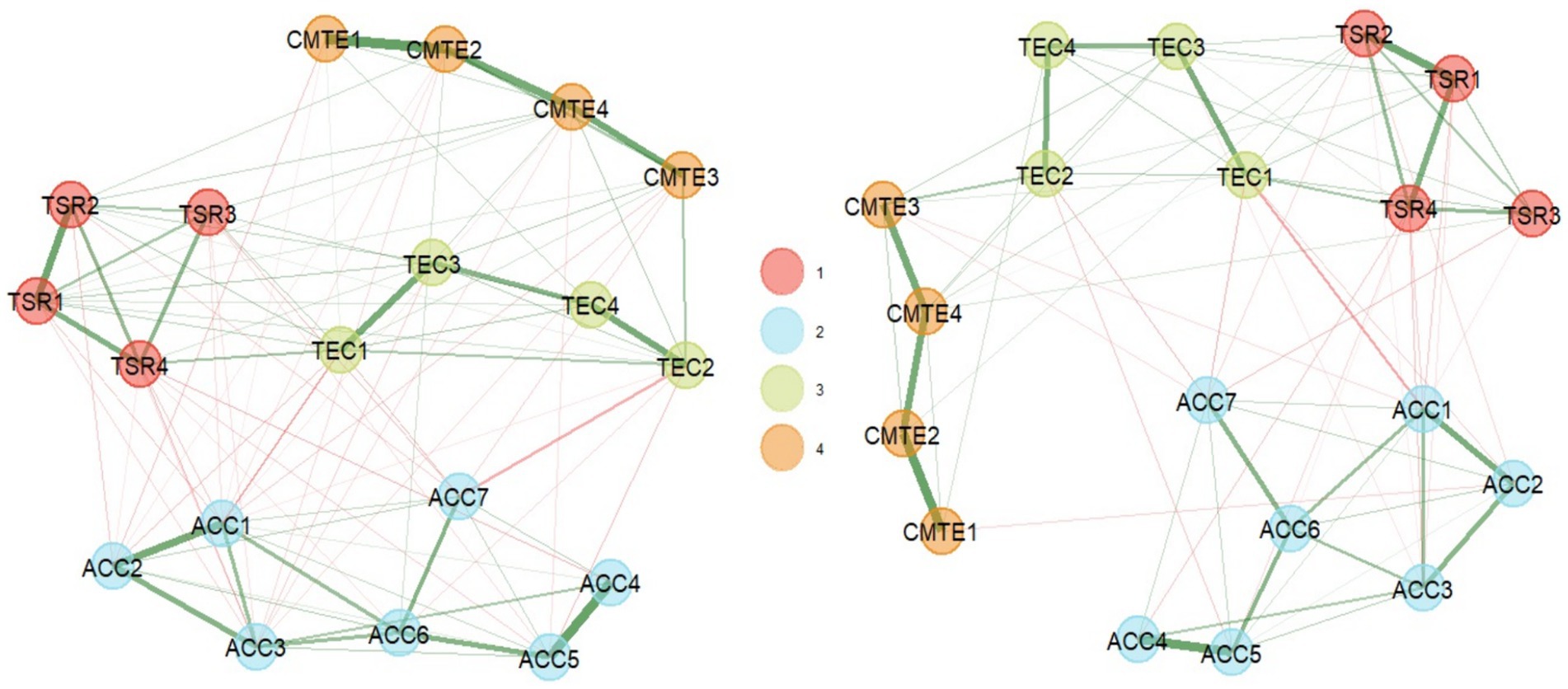
Figure 7. Dimensionality results in EGA (left) and bootEGA (right) of the SEMS-IT with 19 items (sample 2).
In the frequency analysis, four dimensions were identified 94.4% of the time, corresponding to 944 times out of 1,000 bootstrap resamples. Regarding structural consistency or the frequency with which the empirical dimension of EGA replicated exactly in the resampling was 0.997 for dimension 1 (TSR), dimension 2 (ACC) replicated 0.932, dimension 3 (TEC) replicated 0.924 and dimension 4 (CMTE) replicated 0.991. Then, when analyzing the stability of the items, it could be observed that they replicated in their community at least 93% of the time (Figure 8).
Finally, the fit of the structure suggested by EGA was checked by CFA, using the CFA function of the EGAnet package. The results evidenced good and acceptable fit indicators, X2 (171) = 354.546 (p < 0.001), CFI = 0.971, TLI = 0.966, RMSEA = 0.061, and SRMR = 0.062.
4 Discussion
This research focused on examining the psychometric properties of the SocioEmotional Skills Instrument for Teachers (SEMS-IT), an instrument that includes critical and essential teacher SEMS, using the network model. This study is relevant given that SEMS are fundamental for the successful development of a person and for the effective performance of teachers. Among the most important findings, it was evidenced that the SEMS-IT presents optimal metric properties and an adequate internal structure. These results inform that the SEMS-IT can be used as a type of brief measure of SEMS in teachers.
4.1 Strengths of this research
One of the strengths of this research is the use of network analysis with the EGAnet package. This type of analysis provides the area of social sciences with a deeper and more integrative understanding of the structure and dynamics of the constructs being studied toward a unifying theory (Borsboom and Cramer, 2013; Lange et al., 2020). It is constituted as a psychometric network model, in this case on an instrument to measure teaching SEMS, which offers significant advantages compared to other types of analysis given that it is characterized by: (1) flexibility in representation, given that it helps to model complex relationships between different variables, which is crucial to understand the interactions between SEMS (Golino and Epskamp, 2017; Handcock et al., 2008); (2) an approach focused on network topology, which allows identifying key nodes and their connections, which is valuable for understanding how SEMS relate to each other and how patterns of influence emerge (Epskamp et al., 2017; Leskovec and Sosič, 2016); (3) the detection of spillover effects through mediating pathways in the network, for example, if one SEMS affects another through a third, the EGAnet captures this (Christensen et al., 2020); (4) robustness to missing data, since it handles them more flexibly without the need for a complete correlation matrix, and can even estimate relationships when some data are absent without affecting parameter estimation (Christodoulou et al., 2023); and (5) intuitive visualization since it provides graphical representations of the network facilitating the interpretation and communication of results. All in all, the EGAnet is considered a powerful tool in psychometric analysis (Isvoranu and Epskamp, 2023; Marsman et al., 2018; Soares et al., 2021).
Another important aspect of constructing an instrument such as this is that in the field of psychometrics, it is possible to identify measurement scales based on traits and others based on skills (Bradberry and Su, 2006; Brannick et al., 2009). However, when dealing with psychological constructs but applied in an educational performance context, it is more beneficial to have scales that facilitate the identification of categories based on people’s responses, which are possible to improve and, therefore, guide the deployment of actions for socio-emotional development. This has an important value because, even if variables with low development are identified in teachers, they have the possibility of working on them until they reach a desirable performance for the effectiveness of their classroom practice (Lee et al., 2023). The instrument has also been limited to measure teachers’ own skills in their interaction with students, highlighting its value with respect to the specificity of the constructs it measures and in a defined context, which compared to instruments that have been designed without delimiting a context, do not contribute theoretically significant amounts of variance to the models, while instruments of specific skills and specific to a context, contribute substantial amounts to the variance of the modelled predictions (Spitzberg, 1991).
4.2 Implications of this study at the theoretical level
This study also has strong theoretical and practical implications. First, the findings obtained may be valuable for expanding the conceptual framework of the variable SEMS teachers (Dung and Zsolnai, 2021), especially considering that the possibilities of socioemotional development depend largely on the context in which a person develops (Jones et al., 2019). The structure of individual differences in many social and emotional attributes is required to be specified, especially in teachers and their performance in the educational area, resulting in the essential to identify the main domains of socioemotional content that are required to be assessed through instruments (Primi et al., 2019). This study does not intend to propose a definitive instrument, but rather to contribute with a measurement tool that contributes to the research of teaching SEMS and encourages other instruments that complement the included domains that have been rigorously selected within the wide number of possibilities.
Specifically, we integrated as dimensions of the instrument as follows: (a) the cognitive management of emotions, as it allows teachers a cognitive change where they can modify the emotional effects before various situations in the classroom, so when they want to feel more positive emotions or less negative emotions while teaching, they can change their way of thinking about the situation (Gross, 1998; Gross and John, 2003; Hagenauer et al., 2015); (b) empathic concern, which involves teachers’ deployment of a constellation of authentic emotions when they observe needs or difficulties (personal and/or social) in their students during their teaching, allowing them to understand their thoughts and feelings by responding compassionately with sensitivity, concern, attention, sympathy, without losing sight of their students’ learning (Fry and Runyan, 2018; Bialystok and Kukar, 2018; Meyers et al., 2019); (c) the TSR, which reflects the teacher’s influence and proximity to his or her students while teaching, which has been associated with significant changes in student performance and motivation (Simon et al., 2022; Wubbels and Brekelmans, 2005); (d) adverse situations in the classroom, which allows understanding the complex and problematic situations experienced by teachers during their teaching, which are directly related to teacher burnout, symptoms of depression, stress, anxiety, low professional optimism, in turn considered critical factors in the decision of teachers to move schools or leave their profession (Agyapong et al., 2022; Burić et al., 2019; Flores, 2020; McLean et al., 2020; Papastylianou et al., 2009; Sáez-Delgado et al., 2024).
Therefore, the theoretical contribution of this study is directed toward the inquiry of those theoretical–empirical models that integrate SEMS for effective performance and high quality of teacher education (LeTendre, 2017) that allow teachers to overcome the daily difficulties in their professional practice (Fitzgerald et al., 2022; Hen and Goroshit, 2016; Jones et al., 2013), and also includes those SEMS fundamental for themselves and their students to thrive in the 21st century (Collie and Perry, 2019; Scheirlinckx et al., 2023).
4.3 Implications of this study at the practical level
The implications of this study at a practical level consist in the proposal of a scale that contributes to the possibility of guaranteeing greater quality and validity of the research by providing more reliable empirical evidence in the collection of data on teachers’ SEMS, especially valuable when attempting to account for the effects of interventions on SEL in teachers. This is especially relevant, given that it has been noted in the literature that studies have assessed very heterogeneous and different variables through multiple instruments that may reflect inconsistency in assessment procedures (Oliveira et al., 2021). Therefore, adequate consistency is required between the instruments selected to measure teaching SEMS with the variables to be studied, the objectives and the contents addressed in a given intervention to achieve the sensitivity of actually measuring the construct intended to be improved in the program (Domitrovich et al., 2016). In this sense, this study makes available an instrument based on the approach that has been most frequently used for the measurement of SEMS, that of self-report, because it facilitates the operationalization of the skills that are intended to be measured (Schoon, 2021). It also stands out for being a tool that adequately captures SEMS that respond to a specific context, in this case, to school-level teachers considering their formative and modelling role of these skills in their students (Jones and Bouffard, 2012; Martínez-Yarza et al., 2023; Sáez-Delgado et al., 2022).
4.4 Limitations and future lines of research
The results obtained provide valuable information on the validity and reliability of the SEMS-IT. However, it is important to consider some limitations and areas for future research. One aspect to consider as a limitation is related to the type of instrument used (self-report), which, especially when applied to samples of teachers, could show possible social desirability biases in the responses obtained, that is, it could be questioned whether they reach a high average in the different SEMS. There is some background on the results of teachers’ self-reports regarding their SEMS, which do not necessarily correlate with the elicitation of other data, such as physiological measures (Ciuk et al., 2015). Therefore, as a future line of research one could consider exploring other alternative or complementary measurement strategies. Within the forms/methodologies to measure socioemotional aspects, it is recognized that these vary widely, not only due to the intrinsic content of the social and emotional that is sought to be evaluated but also because some authors make a distinction between skills and competencies. Regarding the instruments for the measurement of SEMS, there are several types that have been used in empirical research highlighting the self-report, situational (Aldrup et al., 2020; MacCann and Roberts, 2008; Mayer et al., 2002) observation (Achenbach, 2019; Goodman et al., 2000; Schoon, 2021) and physiological types. Given that each of these types of instruments has strengths and limitations, future studies could consider the application of more than one of them, which would provide a deeper insight into teaching SEMS during their teaching.
Another limitation is that the participants in this study are secondary school teachers; therefore, the conclusions of this study should be applied with care to this specific group. It would be useful to replicate this study with different samples of teachers to analyze the psychometric properties of the instrument and, consequently, the generalization of the results to teachers at other educational levels. A third limitation consists of the teaching SEMS integrated into this instrument. As mentioned above, it does not seek to be a unique instrument, but rather, a relevant measurement resource to measure some key and necessary SEMS to analyze in teachers; undoubtedly, future studies could explore other SEMS that could be included, in the extent that those relevant to teachers and their socioemotional challenges in the classroom are identified. A fourth limitation is important to mention, and this one refers to the lack of a specific analysis based on the network approach that allows analyzing the relationship between the different dimensions that make up the instrument with other related variables; however, this also allows guiding a path for future research, that is, exploring the relationships between these socioemotional skills with other constructs of teachers, and also of students (Palikara et al., 2021). This will strengthen the evidence and understanding of the role that these dimensions play in the outcomes of greatest interest regarding teacher effectiveness (Bardach et al., 2022).
Finally, researchers are encouraged to use this instrument not only for the diagnosis or description of teachers’ SEMS but also in intervention studies that seek to improve SEMS, considering that educators’ own social and emotional skills play an important role in the quality of the educational experiences they offer to their students. The issue of measuring SEMS has become an increasingly urgent concern among both researchers and school leaders, who are more frequently beginning to integrate social–emotional work into classrooms to experiment with and replicate the promising effects reported in research. This is where the present study becomes very valuable to be able to evaluate the effect of interventions or teacher socioemotional development, using a sensitive measurement instrument capable of accurate assessment, which will allow causal inferences to be made about change over time in teacher socioemotional indicators (LeTendre, 2017).
In conclusion, this study contributes to the existing literature on teacher SEMS and provides a useful tool for its evaluation. The findings may have significant implications for teacher training and professional development, as well as for promoting positive and effective learning environments.
Data availability statement
The raw data supporting the conclusions of this article will be made available by the authors, without undue reservation.
Ethics statement
The studies involving humans were approved by Comité Ético Científico, Universidad Católica de la Santísima Concepción. The studies were conducted in accordance with the local legislation and institutional requirements. The participants provided their written informed consent to participate in this study. Written informed consent was obtained from the individual(s) for the publication of any potentially identifiable images or data included in this article.
Author contributions
FS-D: Conceptualization, Funding acquisition, Investigation, Methodology, Project administration, Resources, Supervision, Validation, Writing – original draft, Writing – review & editing. JM-N: Data curation, Formal analysis, Methodology, Software, Validation, Visualization, Writing – original draft. YL-A: Conceptualization, Investigation, Validation, Visualization, Writing – review & editing.
Funding
The author(s) declare that financial support was received for the research, authorship, and/or publication of this article. This study was funded by FONDECYT Regular Project No. 1241902 entitled “Promotion of teacher prosperity through PROSEL-IT intervention based on virtual worlds with immersive experiences and its effect on socioemotional competencies, resilience and well-being” of the National Research and Development Agency of Chile (ANID) assigned to FS-D.
Acknowledgments
The authors are grateful to the teachers from schools in southern Chile who participated in this study.
Conflict of interest
The authors declare that the research was conducted in the absence of any commercial or financial relationships that could be construed as a potential conflict of interest.
Publisher’s note
All claims expressed in this article are solely those of the authors and do not necessarily represent those of their affiliated organizations, or those of the publisher, the editors and the reviewers. Any product that may be evaluated in this article, or claim that may be made by its manufacturer, is not guaranteed or endorsed by the publisher.
Supplementary material
The Supplementary material for this article can be found online at: https://www.frontiersin.org/articles/10.3389/fpsyg.2024.1421164/full#supplementary-material
References
Achenbach, T. (2019). International findings with the Achenbach system of empirically based assessment (ASEBA): applications to clinical services, research, and training. Child Adolesc. Psychiatry Ment. Health 13, 1–10. doi: 10.1186/s13034-019-0291-2
AERA (2014). “American Educational Research Association (2014)” in Standards for educational and psychological testing (Washington DC, United States of America: American Educational Research Association).
Agyapong, B., Obuobi-Donkor, G., Burback, L., and Wei, Y. (2022). Stress, burnout, anxiety and depression among teachers: a scoping review. Int. J. Environ. Res. Public Health 19:10706. doi: 10.3390/ijerph191710706
Aldrup, K., Carstensen, B., and Klusmann, U. (2022). Is empathy the key to effective teaching? A systematic review of its association with teacher-student interactions and student outcomes. Educ. Psychol. Rev. 34, 1177–1216. doi: 10.1007/s10648-021-09649-y
Aldrup, K., Carstensen, B., Köller, M., and Klusmann, U. (2020). Measuring teachers’ social-emotional competence: development and validation of a situational judgment test. Front. Psychol. 11, 1–20. doi: 10.3389/fpsyg.2020.0089
Algina, J., Keselman, H., and Penfield, R. (2005). An alternative to Cohen’s standardized mean difference effect size: a robust parameter and confidence interval in the two independent groups case. Psychol. Methods 10, 317–328. doi: 10.1037/1082-989X.10.3.317
Ato, M., López-García, J., and Benavente, A. (2013). A classification system for research designs in psychology. Ann. Psychol. 29, 1038–1059. doi: 10.6018/analesps.29.3.178511
Bakadorova, O., and Raufelder, D. (2018). The essential role of the teacher-student relationship in students’ need satisfaction during adolescence. J. Appl. Dev. Psychol. 58, 57–65. doi: 10.1016/j.appdev.2018.08.004
Bardach, L., Klassen, R., and Perry, N. (2022). Teachers’ psychological characteristics: do they matter for teacher effectiveness, teachers’ well-being, retention, and interpersonal relations? An integrative review. Educ Psych Rev 34, 259–300. doi: 10.1007/s10648-021-09614-9
Bar-On, R. (1997). The emotional quotient inventory (EQ-i): A test of emotional intelligence. Toronto, Canada: Multi-Health Syst.
Bar-On, R. (2006). The Bar-On model of emotional-social intelligence (ESI). Psicothema 18, 13–25. Available at: https://reunido.uniovi.es/index.php/PST/article/view/8415/8279
Batson, C., Eklund, J., Chermok, V., Hoyt, J., and Ortiz, B. (2007). An additional antecedent of empathic concern: valuing the welfare of the person in need. J. Pers. Soc. Psychol. 93, 65–74. doi: 10.1037/0022-3514.93.1.65
Bialystok, L., and Kukar, P. (2018). Authenticity and empathy in education. Theory Res. Educ. 16, 23–39. doi: 10.1177/1477878517746647
Bisquerra, R., and Peréz, N. (2007). Las competencias emocionales. Educ. XX1, 61–82. doi: 10.1109/PESGM.2012.6344804
Borsboom, D. (2022). Possible futures for network psychometrics. Psychometrika 87, 253–265. doi: 10.1007/s11336-022-09851-z
Borsboom, D., and Cramer, A. (2013). Network analysis: an integrative approach to the structure of psychopathology. Annu. Rev. Clin. Psychol. 9, 91–121. doi: 10.1146/annurev-clinpsy-050212-185608
Bradberry, T., and Su, L. (2006). Ability-versus skill-based assessment of emotional intelligence. Psicothema 18, 59–66
Brannick, M., Wahi, M., Arce, M., Johnson, H., Nazian, S., and Goldin, S. (2009). Comparison of trait and ability measures of emotional intelligence in medical students. Med. Educ. 43, 1062–1068. doi: 10.1111/j.1365-2923.2009.03430.x
Brinkworth, M., McIntyre, J., Juraschek, A., and Gehlbach, H. (2018). Teacher-student relationships: the positives and negatives of assessing both perspectives. J. Appl. Dev. Psychol. 55, 24–38. doi: 10.1016/j.appdev.2017.09.002
Burić, I., Slišković, A., and Penezić, Z. (2019). Understanding teacher well-being: a cross-lagged analysis of burnout, negative student-related emotions, psychopathological symptoms, and resilience. Educ. Psychol. 39, 1136–1155. doi: 10.1080/01443410.2019.1577952
Cai, Y., Dong, S., Yuan, S., and Hu, C. (2020). Network analysis and its applications in psychology. Adv. Psych. Sci. 28, 178–190. doi: 10.3724/SP.J.1042.2020.00178
Castillo-Díaz, M., and Padilla, J. (2013). How cognitive interviewing can provide validity evidence of the response processes to scale items. Soc. Indic. Res. 114, 963–975. doi: 10.1007/s11205-012-0184-8
Christensen, A., Garrido, L., and Golino, H. (2023). Unique variable analysis: a network psychometrics method to detect local dependence. Multivariate Behav. Res. 58, 1165–1182. doi: 10.1080/00273171.2023.2194606
Christensen, A., Golino, H., and Silvia, P. (2020). A psychometric network perspective on the validity and validation of personality trait questionnaires. Eur. J. Personal. 34, 1095–1108. doi: 10.1002/per.2265
Christensen, A., Gross, G., Golino, H., Silvia, P., and Kwapil, T. (2019). Exploratory graph analysis of the multidimensional schizotypy scale. Schizophr. Res. 206, 43–51. doi: 10.1016/j.schres.2018.12.018
Christodoulou, A., Karekla, M., Costantini, G., and Michaelides, M. (2023). A network analysis approach on the psychological flexibility/inflexibility model. Behav. Ther. 54, 719–733. doi: 10.1016/j.beth.2023.01.002
Ciuk, D., Troy, A., and Jones, M. (2015). Measuring emotion: self-reports vs. physiological indicators. Annual Meeting of the Midwest Political Science Association. 1–28.
Clem, A., Rudasill, K., Hirvonen, R., Aunola, K., and Kiuru, N. (2021). The roles of teacher–student relationship quality and self-concept of ability in adolescents’ achievement emotions: temperament as a moderator. Eur. J. Psychol. Educ. 36, 263–286. doi: 10.1007/s10212-020-00473-6
Coke, J., Batson, C., and McDavis, K. (1978). Empathic mediation of helping: a two-stage model. J. Pers. Soc. Psychol. 36, 752–766. doi: 10.1037/0022-3514.36.7.752
Collaborative for Academic, Social, and Emotional Learning (CASEL) (2008). SEL assessment, tools, needs and outcome assessments. Chicago, Illinois: Collaborative for Academic, Social, and Emotional Learning.
Collaborative for Academic, Social, and Emotional Learning (CASEL) (2013). Effective social and emotional learning programs. Chicago, Illinois, USA: Preschool and Elementary School Edition.
Collie, R. (2020). The development of social and emotional competence at school: an integrated model. Int. J. Behav. Dev. 44, 76–87. doi: 10.1177/0165025419851864
Collie, R., and Perry, N. (2019). Cultivating teacher thriving through social–emotional competence and its development. Aust. Educ. Res. 46, 699–714. doi: 10.1007/s13384-019-00342-2
Cooper, K., and Miness, A. (2014). The co-creation of caring student-teacher relationships: does teacher understanding matter? High School J. 97, 264–290. doi: 10.1353/hsj.2014.0005
Davis, M. (1983). Measuring individual differences in empathy: evidence for a multidimensional approach. J. Pers. Soc. Psychol. 44, 113–126. doi: 10.1037/0022-3514.44.1.113
Decety, J., and Cowell, J. (2014). Friends or foes: is empathy necessary for moral behavior? Psychol. Sci. 9, 525–537. doi: 10.1177/1745691614545130
DeLay, D., Zhang, L., Hanish, L., Miller, C., Fabes, R., Martin, C., et al. (2016). Peer influence on academic performance: a social network analysis of social-emotional intervention effects. Prev. Sci. 17, 903–913. doi: 10.1007/s11121-016-0678-8
Domitrovich, C., Bradshaw, C., Berg, J., Pas, E., Becker, K., Musci, R., et al. (2016). How do School-based prevention programs impact teachers? Findings from a randomized trial of an integrated classroom management and social-emotional program. Prev. Sci. 17, 325–337. doi: 10.1007/s11121-015-0618-z
Dung, D., and Zsolnai, A. (2021). Teachers’ social and emotional competence: a new approach of teacher education in Vietnam. Hung Educ. Res. J. 12, 131–144. doi: 10.1556/063.2021.00050
Epskamp, S., Rhemtulla, M., and Borsboom, D. (2017). Generalized network psychometrics: combining network and latent variable models. Psychometrika 82, 904–927. doi: 10.1007/s11336-017-9557-x
Fan, J., and Wang, Y. (2022). English as a foreign language teachers’ professional success in the Chinese context: the effects of well-being and emotion regulation. Front. Psychol. 13, 1–12. doi: 10.3389/fpsyg.2022.952503
Fitzgerald, M., Shipman, K., Pauletic, M., Ellesworth, K., and Dymnicki, A. (2022). Promoting educator social emotional competence, well-being, and student–educator relationships: a pilot study. Ment. Health Prev. 26, 200234–200210. doi: 10.1016/j.mhp.2022.200234
Flores, M. (2020). Surviving, being resilient and resisting: teachers’ experiences in adverse times. Cambridge J. Educ. 50, 219–240. doi: 10.1080/0305764X.2019.1664399
Fry, B., and Runyan, J. (2018). Teaching empathic concern and altruism in the smartphone age. J. Moral Educ. 47, 1–16. doi: 10.1080/03057240.2017.1374932
Gehlbach, H., Brinkworth, M., and Harris, A. (2012). Changes in teacher–student relationships. Br. J. Educ. Psychol. 82, 690–704. doi: 10.1111/j.2044-8279.2011.02058.x
Golino, H., and Christensen, A. (2021). EGAnet: Exploratory Graph Analysis—A framework for estimating the number of dimensions in multivariate data using network psychometrics.
Golino, H., and Epskamp, S. (2017). Exploratory graph analysis: a new approach for estimating the number of dimensions in psychological research. PLoS One 12, e0174035–e0174026. doi: 10.1371/journal.pone.0174035
Goodman, R., Ford, T., Simmons, H., Gatward, R., and Meltzer, H. (2000). Using the strengths and difficulties questionnaire (SDQ) to screen for child psychiatric disorders in a community sample. Br. J. Psychiatry 177, 534–539. doi: 10.1192/bjp.177.6.534
Gross, J. (1998). The emerging field of emotion regulation: an integrative review. Rev. Gen. Psychol. 2, 271–299. doi: 10.1037/1089-2680.2.3.271
Gross, J. (2001). Emotion regulation in adulthood: timing is everything. Curr. Dir. Psychol. Sci. 10, 214–219. doi: 10.1111/1467-8721.00152
Gross, M. (2002). Social and emotional issues for exceptionally intellectually gifted students. In The social and emotional development of gifted children: What do we know? eds. M. Neihart, S. M. Reis, N. M. Robinson, and S. M. Moon (UK: Prufrock Press Inc.), pp. 19–29.
Gross, J., and John, O. (2003). Individual differences in two emotion regulation processes: implications for affect, relationships, and well-being. J. Pers. Soc. Psychol. 85, 348–362. doi: 10.1037/0022-3514.85.2.348
Hagenauer, G., Hascher, T., and Volet, S. (2015). Teacher emotions in the classroom: associations with students’ engagement, classroom discipline and the interpersonal teacher-student relationship. Eur. J. Psychol. Educ. 30, 385–403. doi: 10.1007/s10212-015-0250-0
Handcock, M., Hunter, D., Butts, C., Goodreau, S., and Morris, M. (2008). Statnet: software tools for the representation, visualization, analysis and simulation of network data. J. Stat. Softw. 24, 1548–7660. doi: 10.18637/jss.v024.i01
Hemi, M., and Kasperski, R. (2023). Development and validation of ‘EduSEL’: Educators’ socio-emotional learning questionnaire. Pers. Individ. Differ. 201, 111926–111913. doi: 10.1016/j.paid.2022.111926
Hen, M., and Goroshit, M. (2016). Social-emotional competencies among teachers: an examination of interrelationships. Cogent Educ. 3, 1–9. doi: 10.1080/2331186X.2016.1151996
Hernández, A., Hidalgo, M., Hambleton, R., and Gómez, J. (2020). International test commission guidelines for test adaptation: a criterion checklist. Psicothema 32, 390–398. doi: 10.7334/psicothema2019.306
Hu, L., and Bentler, P. (1999). Cutoff criteria for fit indexes in covariance structure analysis: conventional criteria versus new alternatives. Struct. Equ. Modeling Mult. J. 6, 1–55. doi: 10.1080/10705519909540118
Isvoranu, A., and Epskamp, S. (2023). Which estimation method to choose in network psychometrics? Deriving guidelines for applied researchers. Psychol. Methods 28, 925–946. doi: 10.1037/met0000439
Jennings, P., and Greenberg, M. (2009). The prosocial classroom: teacher social and emotional competence in relation to student and classroom outcomes. Rev. Educ. Res. 79, 491–525. doi: 10.3102/0034654308325693
Jones, S., and Bouffard, S. (2012). Social and emotional learning in schools: from programs to strategies. Social policy report. Soc. Res. Child. Dev. 26, 1–33. Available at: https://files.eric.ed.gov/fulltext/ED540203.pdf
Jones, S., Bouffard, S., and Weissbourd, R. (2013). Educators´ social and emotional skills vital to learning. Phi Delta Kappan. 94, 62–65. doi: 10.1177/003172171309400815
Jones, S., McGarrah, M., and Kahn, J. (2019). Social and emotional learning: a principled science of human development in context. Educ. Psychol. 54, 129–143. doi: 10.1080/00461520.2019.1625776
Landler-Pardo, G., Arviv, R., Levi-Keren, M., and Weinberger, Y. (2022). Being empathic in complex situations in intercultural education: a practical tool. Intercult. Educ. 33, 391–405. doi: 10.1080/14675986.2022.2090688
Lange, J., Dalege, J., Borsboom, D., van Kleef, G., and Fischer, A. (2020). Toward an integrative psychometric model of emotions. Perspect. Psychol. Sci. 15, 444–468. doi: 10.1177/1745691619895057
Lechner, C. M., Anger, S., and Rammstedt, B. (2019). “Socio-emotional skills in education and beyond: recent evidence and future research avenues” in Research handbook on the sociology of education. ed. R. Becker (UK: Edward Elgar Publishing), 427–453.
Lee, E., Lacey, H., Van Valkenburg, S., McGinnis, E., Huber, B., Benner, G., et al. (2023). What about me? The importance of teacher social and emotional learning and well-being in the classroom. Bey Behav 32, 53–62. doi: 10.1177/10742956221145942
Lee, M., Pekrun, R., Taxer, J., Schutz, P., Vogl, E., and Xie, X. (2016). Teachers’ emotions and emotion management: integrating emotion regulation theory with emotional labor research. Soc. Psychol. Educ. 19, 843–863. doi: 10.1007/s11218-016-9359-5
Leskovec, J., and Sosič, R. (2016). Snap: a general-purpose network analysis and graph-mining library. ACM Trans. Intell. Syst. Technol. 8, 1–20. doi: 10.1145/2898361
LeTendre, G. (2017). “Socio-emotional learning and teacher quality” in International Handbook of Teacher Quality and Policy. eds. M. Akiba and G. LeTendre (UK: Routledge), 1–13.
López-Angulo, Y., Mella-Norambuena, J., Sáez-Delgado, F., and Álvarez-Jara, R. (2020). La Inteligencia emocional en apoderados chilenos confinados por la COVID-19. CienciAmérica 9, 285–298. doi: 10.33210/ca.v9i2.320
Lozano, G., Sáez-Delgado, F., and López-Angulo, Y. (2022). Competencias socioemocionales en docentes de primaria y secundaria: una revisión sistemática. Pag Educ. 15, 01–22. doi: 10.22235/pe.v15i1.2598
Lozano, G., Saez-Delgado, F., López-Angulo, Y., and Mella-Norambuena, J. (2021). Teachers’ social–emotional competence: history, concept, models, instruments, and recommendations for educational quality. Sustain. For. 13:12142. doi: 10.3390/su132112142
Lozano, G., Sáez-Delgado, F., López-Angulo, Y., Mella-Norambuena, J., Contreras-Saavedra, C., and Ramos-Huenteo, V. (2023). Programas de intervención docente en competencias socioemocionales: una revisión sistemática de la literatura. Aula Enc. 25, 218–245. doi: 10.17561/ae.v25n2.7391
MacCann, C., and Roberts, R. (2008). New paradigms for assessing emotional intelligence: theory and data. Emotion 8, 540–551. doi: 10.1037/a0012746
Marsman, M., Borsboom, D., Kruis, J., Epskamp, S., van Bork, R., Waldorp, L., et al. (2018). An introduction to network psychometrics: relating Ising network models to item response theory models. Multivariate Behav. Res. 53, 15–35. doi: 10.1080/00273171.2017.1379379
Martínez-Yarza, N., Santibáñez, R., and Solabarrieta, J. (2023). A systematic review of instruments measuring social and emotional skills in school-aged children and adolescents. Child Indic. Res. 16, 1475–1502. doi: 10.1007/s12187-023-10031-3
Matravers, D. (2014). “Empathy as a route to knowledge” in Empathy: Philosophical and psychological perspectives. eds. A. Coplan and P. Goldie (Oxford: Oxford University Press), 19–30.
Mayer, J., Salovey, P., and Caruso, D. (2000). Emotional intelligence: Key readings on the Mayer and Salovey model : Dude Publishing Available at: https://psycnet.apa.org/record/2004-16474-000.
Mayer, J., Salovey, P., and Caruso, D. (2002). Mayer–Salovey–Caruso emotional intelligence test (MSCEIT) user’s manual. Toronto, Canada: MHS Available at: https://scholars.unh.edu/personality_lab/27/.
Mayer, J., Salovey, P., Caruso, D., and Sitarenios, G. (2003). Measuring emotional intelligence with the MSCEIT V2.0. Emotion 3, 97–105. doi: 10.1037/1528-3542.3.1.97
McLean, L., Abry, T., Taylor, M., and Gaias, L. (2020). The influence of adverse classroom and school experiences on first year teachers’ mental health and career optimism. Teach. Teach. Educ. 87, 1–13. doi: 10.1016/j.tate.2019/102956
Meyers, S., Rowell, K., Wells, M., and Smith, B. (2019). Teacher empathy: a model of empathy for teaching for student success. Coll. Teach. 67, 160–168. doi: 10.1080/87567555.2019.1579699
Moen, A., Sheridan, S., Schumacher, R., and Cheng, K. (2019). Early childhood student–teacher relationships: what is the role of classroom climate for children who are disadvantaged? Early Child Educ J. 47, 331–341. doi: 10.1007/s10643-019-00931-x
Muñiz, J., and Fonseca-Pedrero, E. (2019). Diez pasos para la construcción de un test. Psicothema 31, 7–16. doi: 10.7334/psicothema2018.291
Muzzio, E., and Strasser, K. (2022). An integrative framework of early socio-emotional competencies: cognition, regulation and communication. Int. J. Psychol. 56, e1330–e1317. doi: 10.30849/ripijp.v56i1.1330
Oliveira, S., Roberto, M., Pereira, N., Marques-Pinto, A., and Veiga-Simão, A. (2021). Impacts of social and emotional learning interventions for teachers on teachers’ outcomes: a systematic review with meta-analysis. Front. Psychol. 12, 1–19. doi: 10.3389/fpsyg.2021.677217
Ornaghi, V., Conte, E., Cavioni, V., Farina, E., and Pepe, A. (2023). The role of teachers’ socio-emotional competence in reducing burnout through increased work engagement. Front. Psychol. 14, 1–11. doi: 10.3389/fpsyg.2023.1295365
Pagano, A., and Vizioli, N. (2021). Adaptación del Cuestionario de Regulación Emocional (ERQ) en población adulta de la Ciudad Autónoma de Buenos Aires y el Conurbano Bonaerense. Rev. Psic. 21, 18–32. doi: 10.18682/pd.v21i1.3881
Palikara, O., Castro-Kemp, S., Gaona, C., and Eirinaki, V. (2021). The mediating role of school belonging in the relationship between socioemotional well-being and loneliness in primary school age children. Austr. J. Psych. 73, 24–34. doi: 10.1080/00049530.2021.1882270
Papastylianou, A., Kaila, M., and Polychronopoulos, M. (2009). Teachers’ burnout, depression, role ambiguity and conflict. Soc. Psychol. Educ. 12, 295–314. doi: 10.1007/s11218-008-9086-7
Pastore, G., and Luder, R. (2021). Teacher-student-relationship quality in inclusive secondary schools: theory and measurement of emotional aspects of teaching. Front. Educ. 6, 1–7. doi: 10.3389/feduc.2021.643617
Pedrosa, I., Suárez-Álvarez, J., and García-Cueto, E. (2013). Content validity evidences: theoretical advances and estimation methods. Ac Psicol. 10, 3–20. doi: 10.5944/ap.10.2.11820
Péloquin, K., and Lafontaine, M. (2010). Measuring empathy in couples: validity and reliability of the interpersonal reactivity index for couples. J. Pers. Assess. 92, 146–157. doi: 10.1080/00223890903510399
Pérez-Escoda, N., Alegre Rosselló, A., and López-Cassà, E. (2021). Validación y fiabili-dad del cuestionario de desarrollo emocional en Adultos (CDE-A35). Educ S XXI. 39, 37–60. doi: 10.6018/educatio.422081
Petrides, K. (2009). “Psychometric properties of the trait emotional intelligence questionnaire (TEIQue)” in Assessing emotional intelligence: Theory, research, and applications. eds. J. Parker, D. Saklofske, and C. Stough (Boston, MA: Springer US), 85–101.
Pianta, R., Hamre, B., and Allen, J. (2012). “Teacher-student relationships and engagement: conceptualizing, measuring, and improving the capacity of classroom interactions” in Handbook of research on student engagement. (eds.) S. Christenson, A. Reschly, and C. Wylie (Boston, MA: Springer US), 365–386.
Polit, D., Beck, C., and Owen, S. (2007). Is the CVI an acceptable indicator of content validity? Appraisal and recommendations. Res Nur Health. 30, 459–467. doi: 10.1002/nur.20199
Primi, R., Santos, D., Hauck, N., Fruyt, F., and John, O. (2019). Mapping self-report questionnaires for socio-emotional characteristics: what do they measure? Estud. Psicol. 36, 1–15. doi: 10.1590/1982-0275201936e180138
Quin, D. (2017). Longitudinal and contextual associations between teacher-student relationships and student engagement: a systematic review. Rev. Educ. Res. 87, 345–387. doi: 10.3102/0034654316669434
Rodriguez, V., Solis, S., Mascio, B., Kiely, K., Jennings, P., and Brotman, L. (2020). With awareness comes competency: the five Awarenesses of teaching as a framework for understanding teacher social-emotional competency and well-being. Early Educ. Dev. 31, 940–972. doi: 10.1080/10409289.2020.1794496
Roorda, D., Jak, S., Zee, M., Oort, F., and Koomen, H. (2017). Affective teacher-student relationships and students’ engagement and achievement: a meta-analytic update and test of the mediating role of engagement. School Psych. Rev. 46, 239–261. doi: 10.17105/SPR-2017-0035.V46-3
Sáez-Delgado, F., López-Angulo, Y., Mella-Norambuena, J., Baeza-Sepúlveda, C., Contreras-Saavedra, C., and Lozano-Peña, G. (2022). Teacher self-regulation and its relationship with student self-regulation in secondary education. Sustain. For. 14:16863. doi: 10.3390/su142416863
Sáez-Delgado, F., López-Angulo, Y., Mella-Norambuena, J., Hartley, K., and Sepúlveda, F. (2023). Mental health in school teachers: an explanatory model with emotional intelligence and coping strategies. Elec. J. Res. Educ. Psychol. 21, 559–586. doi: 10.25115/ejrep.v21i61.8322
Sáez-Delgado, F., Manriquez, C., López-Angulo, Y., Mella-Norambuena, J., and Contreras-Saavedra, C. (2024). Self-regulatory processes in secondary school teachers during online classes by covid-19. Zona Próxima. 41, 89–109. doi: 10.14482/zp.41.456.654
Salovey, P., and Mayer, J. (1990). Emotional Intelligence. Imag. Cogn. Per. 9, 185–211. doi: 10.2190/DUGG-P24E-52WK-6CDG
Salovey, P., Mayer, J., Goldman, S., Turvey, C., and Palfai, T. (1995). “Emotional attention, clarity, and repair: exploring emotional intelligence using the trait Meta-mood scale” in Emotion, disclosure, & health. ed. J. W. Pennebaker (Washington DC, USA: Am Psychol As), 125–154.
Sánchez-Pujalte, L., Mateu, D., Etchezahar, E., and Gómez Yepes, T. (2021). Teachers’ burnout during COVID-19 pandemic in Spain: trait emotional intelligence and socioemotional competencies. Sustain. For. 13, 1–11. doi: 10.3390/su13137259
Scheirlinckx, J., Van Raemdonck, L., Abrahams, L., Teixeira, K., Alves, G., Primi, R., et al. (2023). Social–emotional skills of teachers: mapping the content space and defining taxonomy requirements. Front. Educ. 8, 1–15. doi: 10.3389/feduc.2023.1094888
Schonert-Reichl, K. (2017). Social and emotional learning and teachers. Fut. Chil. 27, 137–155. doi: 10.1353/foc.2017.0007
Schoon, I. (2021). Towards an integrative taxonomy of social-emotional competences. Front. Psychol. 12, 51–59. doi: 10.3389/fpsyg.2021.515313
Seiz, J., Voss, T., and Kunter, M. (2015). When knowing is not enough: the relevance of teachers’ cognitive and emotional resources for classroom management. Front. Learn. Res. 3, 55–77. doi: 10.14786/flr.v3i1.141
Serrano-Díaz, N., Pocinho, M., and Aragón-Mendizábal, E. (2017). Competencias emocionales y síndrome de burnout en el profesorado de Educación Infantil. J. Psychol. Educ. 13, 1–12. doi: 10.23923/rpye2018.01.153
Shamay-Tsoory, S., Aharon-Peretz, J., and Perry, D. (2009). Two systems for empathy: a double dissociation between emotional and cognitive empathy in inferior frontal gyrus versus ventromedial prefrontal lesions. Brain 132, 617–627. doi: 10.1093/brain/awn279
Simon, K., Petrovic, L., Baker, C., and Overstreet, S. (2022). An examination of the associations among teacher secondary traumatic stress, teacher–student relationship quality, and student socio-emotional functioning. School Ment. Health. 14, 213–224. doi: 10.1007/s12310-022-09507-4
Soares, G., Santiago, P., Werneck, R., Michel-Crosato, E., and Jamieson, L. (2021). A psychometric network analysis of OHIP-14 across Australian and Brazilian populations. JDR Clin. Trans. Res. 6, 333–342. doi: 10.1177/238008442093993
Spitzberg, B. (1991). An examination of trait measures of interpersonal competence. Com. Repo. 4, 22–29. doi: 10.1080/08934219109367517
Taherdoost, H. (2016). Validity and reliability of the research instrument; how to test the validation of a questionnaire/survey in a research. Intern. J. Ac. Res. Manag. 5, 28–36. doi: 10.2139/ssrn.3205040
Wachs, S., Valido, A., Espelage, D. L., Castellanos, M., Wettstein, A., and Bilz, L. (2023). The relation of classroom climate to adolescents’ countering hate speech via social skills: a positive youth development perspective. J. Adolesc. 95, 1127–1139. doi: 10.1002/jad.12180
Wentzel, K. (2022). Does anybody care? Conceptualization and measurement within the contexts of teacher-student and peer relationships. Educ. Psychol. Rev. 34, 1919–1954. doi: 10.1007/s10648-022-09702-4
Wilcox, R. (2012). Introduction to robust estimation and hypothesis testing. 3rd Edn. Amsterdam, Netherlands: Elsevier.
Winczewski, L., Bowen, J., and Collins, N. (2016). Is empathic accuracy enough to facilitate responsive behavior in dyadic interaction? Distinguishing Ability From Motivation. Psychol Sci. 27, 394–404. doi: 10.1177/0956797615624491
Wolcott, M., and Lobczowski, N. (2021). Using cognitive interviews and think-aloud protocols to understand thought processes. Curr. Pharm. Teach. Learn. 13, 181–188. doi: 10.1016/j.cptl.2020.09.005
Wubbels, T., and Brekelmans, M. (2005). Two decades of research on teacher–student relationships in class. Int. J. Educ. Res. 43, 6–24. doi: 10.1016/j.ijer.2006.03.003
Yoder, N. (2014). Self-assessing social and emotional instruction and competencies: a tool for teachers. Center on Great Teachers and Leaders. Available at: https://files.eric.ed.gov/fulltext/ED553369.pdf
Zhang, W., He, E., Mao, Y., Pang, S., and Tian, J. (2023). How teacher social-emotional competence affects job burnout: the chain mediation role of teacher-student relationship and well-being. Sustain. For. 15, 1–13. doi: 10.3390/su15032061
Zhou, M., and Ee, J. (2012). Development and validation of the social emotional competence questionnaire (SECQ). Int. J. Emot. Educ. 4, 27–42. Available at: https://www.um.edu.mt/library/oar/bitstream/123456789/6140/1/ENSECV4I2P2.pdf
Keywords: network approach, socioemotional skills, psychometric, teachers, validation
Citation: Sáez-Delgado F, Mella-Norambuena J and López-Angulo Y (2024) Psychometric properties of the SocioEmotional Skills Instrument for Teachers using network approach: English and Spanish version. Front. Psychol. 15:1421164. doi: 10.3389/fpsyg.2024.1421164
Edited by:
Silvia Cristina da Costa Dutra, University of Zaragoza, SpainReviewed by:
Gül Kadan, Cankiri Karatekin University, TürkiyeLuis Manuel Mota de Sousa, Universidade Atlântica, Portugal
Sara Kashefian-Naeeini, Shiraz University of Medical Sciences, Iran
Xavier Oriol, University of Girona, Spain
Copyright © 2024 Sáez-Delgado, Mella-Norambuena and López-Angulo. This is an open-access article distributed under the terms of the Creative Commons Attribution License (CC BY). The use, distribution or reproduction in other forums is permitted, provided the original author(s) and the copyright owner(s) are credited and that the original publication in this journal is cited, in accordance with accepted academic practice. No use, distribution or reproduction is permitted which does not comply with these terms.
*Correspondence: Fabiola Sáez-Delgado, ZnNhZXpAdWNzYy5jbA==