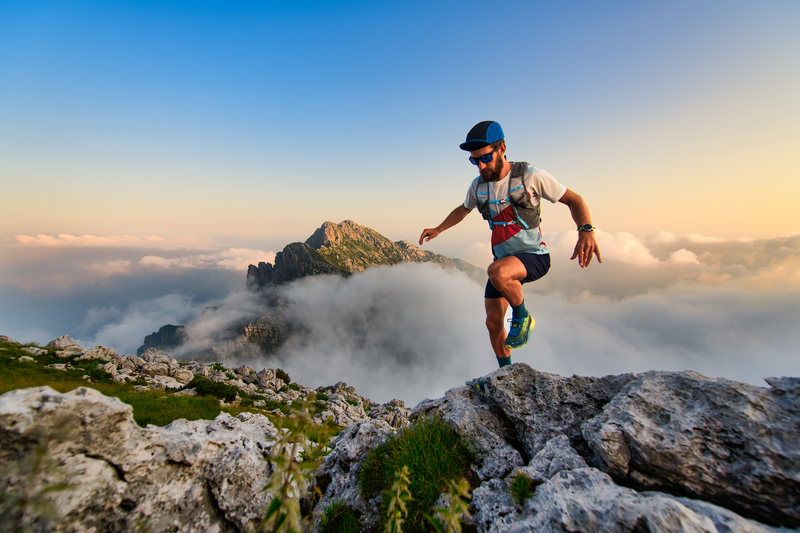
95% of researchers rate our articles as excellent or good
Learn more about the work of our research integrity team to safeguard the quality of each article we publish.
Find out more
ORIGINAL RESEARCH article
Front. Psychol. , 07 March 2024
Sec. Psychology of Aging
Volume 15 - 2024 | https://doi.org/10.3389/fpsyg.2024.1376207
This article is part of the Research Topic Gerontechnologies for Home Support View all 10 articles
Introduction: Voice assistants (VAs) have the potential to uphold and enhance the quality of life for older adults. However, the extent to which older adults accept and benefit from VAs may be relatively modest.
Methods: This study developed a comprehensive model combined with product and personal characteristics to explain the acceptance of VAs among older adults, using semi-structured interviews (Study 1) and questionnaires (Study 2).
Results: Results revealed that in terms of product characteristics, perceived usefulness and perceived enjoyment significantly affect behavior intention. Regarding personal characteristics of older adults, technological self-efficacy and dispositional resistance to change significantly affect behavior intention. However, no direct impact of perceived ease of use and perceived trust on behavior intention. Additionally, perceived enjoyment influenced both perceived ease of use and perceived usefulness.
Discussion: Results suggested the significant role of technology self-efficacy and dispositional resistance to change in predicting the acceptance of VAs among older adults. Our newly developed model offers valuable insights for tailoring VAs to this demographic during design and implementation.
Voice interaction is highly effective and humanized in Human-Computer Interaction (HCI) (Turow, 2021; Calahorra-Candao and Hoyos, 2024). The rapid development of intelligent voice technology, facilitated by advancements in deep learning, Internet of Things (IoT) technology, and enhanced computing power, is revolutionizing societal norms (Pham Thi and Duong, 2022; Ossadnik et al., 2023). A prominent manifestation of this technology is found in voice assistants (VAs). According to Juniper Research (2021), the number of VAs is projected to exceed the global population, reaching 8.4 billion by 2024.
According to the 2022 Revision of World Population Prospects (Department of Economic and Social Affairs of United Nations, 2022), the proportion of the global population aged 65 and above is projected to increase significantly, exceeding 1.5 billion by 2050. As of the end of 2022, the elderly population aged 60 and above in China reached 280.04 million (National Bureau of Statistics of China, 2023). The current study defined elderly as the people aged 60 and above. As VAs become increasingly popular and permeate into our daily lives, it is foreseeable that there will be an increasing number of elderly users using VAs in the future.
Previous research has indicated that VAs can support older people in various aspects. Firstly, due to the significant decline in cognitive and physical functions caused by aging, the ability of older adults to perform instrumental activities of daily living (IADLs), such as using smartphones and computers and engaging in shopping, is weakened (Lawton and Brody, 1969; Liu et al., 2023; Werner et al., 2023). Voice interaction offers a more natural and straightforward interaction, making it easier for older people to learn and operate (Kebede et al., 2022; Kuoppamäki et al., 2023). Additionally, VAs can facilitate communication between older adults and others, especially those with visual or hand function impairments (Kowalski et al., 2019). It also found that VAs can serve as social companions to some extent (Corbett et al., 2021; Yan et al., 2024). Lastly, VAs can also assist in daily health activities such as health tracking, medication management, and meal planning, which could support the elderly’s daily lives (Nallam et al., 2020; Schlomann et al., 2021; Yan et al., 2024).
Despite the potential of VAs to support the lives of the elderly and their overall good usability, previous research has indicated that the willingness of older adults to adopt VAs is relatively low (Song et al., 2022). Additionally, VAs were initially designed for younger people, and the designers are usually younger, having grown up in a more technologically advanced environment (Kim and Choudhury, 2021). As a result, the product design often overlooks older adults’ physiological and psychological characteristics. Therefore, it is crucial to identify the factors that promote or hinder the use of VAs among older adults.
To investigate the factors influencing the acceptance of VAs among older adults, we will review three aspects: the theoretical models related to technology acceptance, factors influencing technology acceptance among older adults, and research on the acceptance of VAs among older adults.
Several theories have been proposed to understand the factors driving user technology acceptance, including the Theory of Reasoned Action (TRA, Fishbein and Ajzen, 1975), the Technology Acceptance Model (TAM, Davis, 1989), the Technology Acceptance Model 2 (TAM2, Venkatesh and Davis, 2000), and the Unified Theory of Acceptance and Use of Technology (UTAUT, Venkatesh et al., 2003). Among these proposed technology acceptance models, TAM is currently the most widely used model for predicting technology acceptance (Ma et al., 2021; Yu et al., 2022). TAM was initially proposed to provide an explanatory framework for the factors influencing computer systems within organizational settings. As TAM theory evolved, it has been widely applied to investigate user acceptance of new technologies (Nagle and Schmidt, 2012; Zhao et al., 2018; Zhu and Cheng, 2022). Perceived usefulness and perceived ease of use, as proposed by TAM, are the main determinants influencing users’ intention of technology acceptance behavior. Perceived ease of use refers to the user’s subjective perception of the ease or difficulty of utilizing a particular technology. Perceived usefulness refers to the user’s subjective perception of the degree to which a technology is beneficial during use. Moreover, perceived ease of use positively influences perceived usefulness.
Furthermore, Ram and Sheth (1989) proposed the innovation resistance theory (IRT) based on consumers’ resistance to innovation, which categorized innovation resistance into functional barriers and psychological barriers. Functional barriers encompass risks, value, and usage barriers, while psychological barriers include traditional and image barriers. Several studies employ the IRT theory to analyze consumer innovation adoption behavior, especially in the initial adoption stage (Borraz-Mora et al., 2017; Dotzauer and Haiss, 2017; Leong et al., 2021). IRT has emerged as the favored framework among scholars specializing in the study of innovation resistance, either as a standalone model (Borraz-Mora et al., 2017; Hew et al., 2019) or when integrated with other established frameworks such as UTAUT (Lian and Yen, 2014) and TAM (Agag and El-Masry, 2016).
Given older adults’ physiological and psychological characteristics, many studies incorporate personal and perceptual characteristics variables when exploring technology acceptance among older adults using relevant theoretical frameworks. For instance, some studies have indicated that technological self-efficacy and technology anxiety play essential roles in the technology acceptance of older adults (Peral-Peral et al., 2020; Jeng et al., 2022). Chen and Chan (2014) found that these constructs may better predict technology acceptance behavior of older adults than the conventionally attitudinal factors (usefulness and ease of use). Furthermore, older adults tend to have a slower processing speed and a more challenging learning process when accepting and learning new technologies (Kebede et al., 2022). If using technology is enjoyable, older adults are more likely to adopt it (Talukder et al., 2020). Studies have found that incorporating perceived enjoyment can improve the predictive power of technology acceptance-related models (Phibbs, 2021; Zeng and Chen, 2022). Additionally, the fear of technology intrusiveness among older adults has been repeatedly identified as a significant barrier to adopting technologies (Fischl et al., 2017; Kong and Woods, 2018; Zambianchi et al., 2019). Song et al. (2022) found that perceived trust directly impacts the acceptance of VAs.
On the other hand, dispositional resistance to change (DRTC) within personality traits is also considered a valid antecedent for the technological acceptance of older adults. Due to the pro-innovation bias (Talke and Heidenreich, 2014), most researchers believe that consumers are willing to change and are thus tempted to try innovative products, as long as the benefits of the technology, such as perceived usefulness and perceived ease of use, are emphasized. However, the reality often is that consumers tend to reject innovation without considering the potential of the product, leading to the adoption process ending before it even begins. This is particularly evident among older adults (Ma et al., 2021). Despite successful implementation of innovation, there may still be resistance (Laukkanen et al., 2008). Consumers often resist innovation due to changes in their current habits or norms required for accepting new ideas (Snyder, 1961). Previous research on VAs has found that older adults accustomed to traditional interaction methods involving input and output devices tend to exhibit resistance when adopting VAs (Trajkova and Martin-Hammond, 2020). However, no study has delved into the influence of DRTC on behavior intention of VAs among older adults. Furthermore, despite the inherent simplicity of VA interactions, older adults still encounter misunderstandings and challenges when using VAs during the initial stages (Kim, 2021), which may lead older adults with higher levels of DRTC to discontinue usage altogether.
In recent years, the prospects for applying for VAs have expanded extensively. Numerous studies have initiated exploring the adoption and utilization of VAs among older adults.
Currently, research primarily focused on the advantages and disadvantages of VAs. VAs provided simplicity, convenience, and easy accessibility. It could support and enhance the social engagement, autonomy, and leisurely activities of older adults (Kowalski et al., 2019; Pradhan et al., 2020; Kim, 2021; Song et al., 2022; Werner et al., 2023). However, within VAs utilization, certain predicaments came to the forefront. Concerns about the privacy and security of financial information and recorded dialogues emerged (Bonilla and Martin-Hammond, 2020; Pradhan et al., 2020; Kim, 2021; Song et al., 2022; Werner et al., 2023). Additionally, challenges related to voice recognition were documented, including instances of speech misrecognition, difficulties in recalling specific commands, and issues with device response timing (Kowalski et al., 2019; Pradhan et al., 2020; Kim, 2021; Werner et al., 2023). Some older adults also believed VAs had not exhibited significant advantages and failed to demonstrate any discernible utility (Trajkova and Martin-Hammond, 2020; Kim, 2021).
Further research has delved into the impact of personal characteristics of older adults on the acceptance of VAs. Trajkova and Martin-Hammond (2020) found that many older adults refrained from using Echo. This avoidance was rooted in their belief in their ability to complete tasks independently. They hold a deep appreciation for this autonomy, potentially earmarking the adoption of such technology for a period when their physical state experiences a decline. Moreover, a lack of pertinent knowledge regarding VAs among the elderly may also engender difficulties in their utilization (Pradhan et al., 2020; Kim, 2021; Werner et al., 2023). Lastly, Song et al. (2022) have posited that self-efficacy might indirectly influence the acceptance of VAs among the elderly.
Previous studies have demonstrated the factors that promote or hinder older adults’ acceptance of VAs, primarily focusing on the characteristics of VA products, as well as older adults’ knowledge, experience, and physical condition as personal characteristics. DRTC and technological self-efficacy may have a stronger impact on older adults’ acceptance of technology (Chen and Chan, 2014; Touchaei and Hashim, 2023; Werner et al., 2023), but have been overlooked in the investigation of VAs’ acceptance. Therefore, it is necessary to further explore whether there are additional potential factors influencing the acceptance of VAs among older adults.
Moreover, to our understanding, most studies on the acceptance of VAs by older adults have relied on a single method. Except for Song et al. (2022), who used a questionnaire, current research has predominantly used qualitative methods to understand the viewpoints of the elderly towards VAs (Kowalski et al., 2019; Pradhan et al., 2020; Kim, 2021; Werner et al., 2023). However, the qualitative method has limitations, such as subjectivity, small sample sizes, and the inability to explore relationships between variables thoroughly. Further quantitative methods based on qualitative methods are an effective approach to remedy this limitation. Moreover, cross-validation between these two methods can enhance the credibility and accuracy of the research results, thereby bolstering the study’s robustness and comprehensiveness.
Besides, COVID-19 may have also altered the attitudes of older adults towards digital technology. While previous research has indicated that privacy concerns can hinder the adoption of VAs among older adults (Bonilla and Martin-Hammond, 2020; Pradhan et al., 2020; Kim, 2021; Song et al., 2022; Werner et al., 2023), the ongoing stress caused by the pandemic has highlighted the potential of VAs to support independent living and help older adults cope with the pressures brought by COVID-19 (Carstensen et al., 2020; Werner et al., 2023). This may lead older adults to prioritize the benefits of adopting VAs over privacy concerns, a trade-off that could extend into the post-COVID-19 era. Furthermore, factors such as perceived ease of use and self-efficacy in using VAs have been found to play a crucial role in the acceptance of VAs by older adults (Song et al., 2022). However, the COVID-19 pandemic has accelerated the adoption of digital technology among older adults (Diehl et al., 2022), potentially impacting the influence of perceived ease of use and self-efficacy on behavioral intentions regarding VAs adoption (Kim and Choudhury, 2021). Therefore, it is necessary to re-examine the impact of these factors on the acceptance of VAs.
To address these research gaps, we propose the following approach. First, we will extract factors influencing the acceptance of VAs among older adults from existing literature and theories and develop a preliminary model. Second, we will conduct semi-structured interviews to explore further the factors influencing the acceptance of VAs among older adults. Lastly, we will incorporate the newly emerged factors from the interviews into the preliminary model and employ partial least squares structural equation modeling (PLS-SEM) to determine the mechanisms influencing the acceptance of VAs among older adults. The research process is illustrated in Figure 1.
Based on existing literature, we propose a preliminary model that extends TAM with the addition of relevant constructs specific to VAs (i.e., perceived enjoyment, value barrier, and perceived trust) (Nguyen et al., 2018; Trajkova and Martin-Hammond, 2020) and older adults (i.e., technological self-efficacy) (Chen and Chan, 2014).
Perceived trust and self-efficacy are unique characteristics of VAs and have been widely used as standard metrics for measuring experience in VAs research (Kim and Choudhury, 2021; Song et al., 2022). Value barrier also has been repeatedly mentioned in interview-based studies on older adults’ perspectives on VAs (Trajkova and Martin-Hammond, 2020; Kim and Choudhury, 2021). Perceived enjoyment is also a relevant and unique factor for VAs, and has been explored among younger age groups (Nguyen et al., 2018).
Perceived usefulness and perceived ease of use are determinants in TAM, widely used in exploring technology acceptance among older adults. Meta-analyses have shown positive effects of perceived usefulness and perceived ease of use on behavioral intention (Zhou et al., 2020; Ma et al., 2021). Previous studies have extensively considered the influence of perceived usefulness and perceived ease of use on VAs acceptance (Nguyen et al., 2018; Song et al., 2022; Zhong et al., 2022). Given the significant advantages of VAs compared to other technologies, such as natural and simple interactions that can support independent living among older adults (Song et al., 2022), we expect that the practical benefits and convenience will positively influence older adults’ behavioral intention to use VAs. Furthermore, the similarity between interactions with VAs and interpersonal dialogues in daily life enables older adults to learn intuitively, and effortless learning can further enhance users’ perceived usefulness. Previous research has already demonstrated the positive relationship between perceived ease of use and perceived usefulness in the context of VAs (Nguyen et al., 2018; Song et al., 2022). Therefore, the following hypotheses are proposed:
H1: Perceived usefulness positively affects behavioral intention.
H2: Perceived ease of use positively affects behavioral intention.
H3: Perceived ease of use positively affects perceived usefulness.
Perceived enjoyment refers to the extent to which older adults perceive using VAs as entertaining and delightful, besides any expected performance outcomes (Davis et al., 1992; Nguyen et al., 2018). Empirical evidence from the research indicates that PE is one of the main reasons why mobile users access VAs (Nguyen et al., 2018). Hassenzahl (2018) also suggests that products should consider both utilitarian value and hedonic value, as the combination of these values better reflects the beneficial characteristics of the product. Therefore, in addition to perceived usefulness and perceived ease of use, Perceived enjoyment becomes another significant driving factor explaining behavioral intention in our comprehensive model. We propose the following hypothesis:
H4: Perceived enjoyment positively influences behavioral intention.
According to Ram and Sheth (1989), in this study, value barrier refers to the evaluation of value that older adults assign to VAs and their alternatives. Consumers typically use their current products as reference points. Suppose a new product does not offer more excellent value than the reference point, in that case, they are less likely to consider switching to an alternative because the perceived drawbacks of changing from the existing norm seem to outweigh the benefits (Leong et al., 2021). Trajkova and Martin-Hammond (2020) found that the main reason older adults discontinued using VAs was their difficulty finding valuable uses. Several studies have indicated that value barrier had a negative impact on behavioral intention in various contexts, including online shopping (Lian and Yen, 2014), mobile commerce (Moorthy et al., 2017), mobile banking (Laukkanen, 2016), and mobile payment systems (Kaur et al., 2020). Furthermore, when consumers attempt to assess the value difference between innovative and existing products, they consider various alternatives to accomplish their tasks, and the functions of VAs can also be replaced by other mediums (Trajkova and Martin-Hammond, 2020). This may also affect the perceived usefulness of VAs. Therefore, we propose the following hypotheses:
H5: Value barrier negatively influences behavioral intention.
H6: Value barrier negatively influences perceived usefulness.
Trust is a significant factor influencing users’ adoption of technology. Security concerns, privacy risks, and distrust are common reasons for digitally disengaging, mainly in web-based digital technologies (Kebede et al., 2022). VAs must record users’ voice commands and daily speech to respond effectively, which may lead users to perceive more risks than other technologies (Song et al., 2022). In this case, trust helps alleviate users’ concerns about sharing and potentially misusing their personal information (Nguyen et al., 2018). Therefore, we propose the following hypothesis:
H7: Perceived trust positively influences behavioral intention.
According to Chen and Chan (2014), in this study, technological self-efficacy refers to the belief of older adults in their ability to use VAs successfully. Contrary to a widespread belief or marketing claim that interacting with VAs is effortless due to their conversational capabilities, most participants found it challenging to engage in a smooth conversation with the technology (Kim, 2021). Furthermore, older adults face additional challenges in using VAs due to their lack of similar technological upbringing as younger generations (Charness and Boot, 2022) and age-related declines in physiological and cognitive functions (Kim, 2021).
The social cognitive theory posits that a heightened level of self-efficacy can enhance cognitive processes (Bandura, 1995). For older adults, a strong sense of self-efficacy can help them maintain a positive and proactive outlook. When faced with challenges in using VAs, old adults with high self-efficacy are more likely to approach the situation optimistically and proactively rather than dwelling on the difficulties. Werner et al. (2023) found that older adults who proactively initiate the use of VAs may believe in their ability to interact successfully with VAs, while those who do not initiate such usage may lack the same level of confidence. Numerous studies have also revealed a direct positive correlation between self-efficacy, perceived ease of use, and behavioral intention (Song et al., 2022; Zhu and Cheng, 2022). Therefore, we propose the following hypotheses:
H8: Technological self-efficacy positively influences perceived ease of use.
H9: Technological self-efficacy positively influences behavioral intention.
Based on the above, the preliminary research model is illustrated in Figure 2.
The aim of the semi-structured interviews was to explore further the factors influencing the acceptance of VAs among older adults and validate the preliminary model.
For practicality and accessibility, we collaborated with Tianjin communities using convenient sampling to recruit participants. A total of 19 participants (10 females), with a mean age of 71.68 and an age range of 61–80, were recruited to participate in the semi-structured interviews. All older adults signed an informed consent form and completed the Mini-Mental State Examination (MMSE) cognitive test (Folstein et al., 1975). The total score range of the MMSE scale is 0 to 30 points. With test scores influenced by cultural background, normal cutoff values are as follows: for illiterate individuals, the cutoff is >17 points; for those with primary education, the cutoff is >20 points; and for those with junior high school education or higher, the cutoff is >24 points. The participants were selected based on three criteria: normal cognition, age over 60 years, and experience using smart devices such as smartphones, tablets, smartwatches, and smart speakers. We aimed to include both current and potential users of VAs, and prior experience with smart devices was a prerequisite for using VAs (Song et al., 2022).
Considering that older adults may be unfamiliar with VAs and have difficulties understanding the technology, the interviewers played a series of videos demonstrating the functions of VAs (such as setting reminders, searching for information, controlling home appliances, etc.). Additionally, the interviewers used Tmall Genie Cube Sugar (a widely used smart speaker in China with no secondary modality) for the demonstration. Each elderly participant engaged in a conversation with the smart speaker to gain an understanding of VAs. The interviewer provided explanations to participants if they had any areas of unfamiliarity. Once all participants clearly understood the functionalities of VAs, the interviews began. The interviews were concluded when the information obtained from the participants reached saturation (Braun and Clarke, 2006).
The interview outline consisted of three aspects:
1. What do you think of VAs?
2. Will you use VAs in the future?
3. What factors influence older adults’ intentions to use VAs (product characteristics and personal characteristics)?
All interviews were audio-recorded and transcribed. To ensure the accuracy and consistency of the transcribed text with the interview results, researchers used the recordings to verify the transcribed results.
The interview data were analyzed using thematic analysis, following open, axial, and selective coding (Braun and Clarke, 2006). The first author continuously discussed the emerging themes with another author until the data reached saturation with repeated themes, and no new information emerged.
1. Open coding: The textual data was broken down into meaningful units (words, phrases, or sentences), and these dissected units were categorized and classified to form initial codes.
2. Axial coding: The codes obtained from open coding were further refined, adjusted, and grouped based on their similarities or connections. Similar or related codes were merged, and the underlying relationships between codes were clarified and organized through constant comparison, resulting in the development of categories.
3. Selective coding: At a more abstract level, the data from axial coding were further processed. The developed categories were consolidated and interconnected to explain the overarching themes and provide a comprehensive understanding of the data.
Through this rigorous analysis, the thematic analysis allowed for a systematic exploration and interpretation of the interview data.
This research encompasses 121 reference points (i.e., meaningful units) extracted to obtain 14 relatively independent initial concepts. Subsequently, centered around the core concept of factors influencing older adults’ acceptance of VAs, the 14 initial concepts were compared and categorized into seven distinct categories. Table 1 displays the initial concepts obtained through open coding and the categories formed by axial coding. Due to space constraints, only one reference point for each initial concept was shown here.
Finally, this study consolidates the developed categories and constructs that influence older adults’ acceptance of VAs into two themes: personal characteristics and VA product characteristics. These themes and connected categories were illustrated in Figure 3.
The results of the semi-structured interviews revealed factors influencing the usage of VAs among older adults can be categorized into two aspects: VA product characteristics and personal characteristics of older adults. We aligned the themes identified through thematic analysis with existing variables, ultimately determined that VA product characteristics including perceived usefulness, perceived ease of use, perceived enjoyment, value barrier, and perceived trust, while the personal characteristics including technological self-efficacy and dispositional resistance to change.
Regarding VA product characteristics, the interviews revealed that most older adults exhibited a positive attitude towards VAs, considering them as natural and straightforward interactions that support daily living and enable older adults to live more independently. The ease of activation through wake-up words or physical buttons on the devices makes VAs accessible to older adults, lowering the barriers to their usage. These results indicated perceived usefulness and ease of use were common factors influencing older adults’ acceptance of VAs, which aligns with previous research findings (Song et al., 2022; Werner et al., 2023). However, some barriers affected the perceived usefulness and ease of use for older adults. For instance, short listening times of VAs, structured question-and-answer approaches, and limitations in speech recognition quality for users with accents could hinder the perceived usability. Additionally, many participants tended to compare VAs with other means of assistance, sometimes perceiving their value as optional. This is consistent with findings from Trajkova and Martin-Hammond (2020), who observed a high churn rate of VAs among older adults due to the lack of perceived utility. These results support hypotheses H1, H2, H5, and H6.
Similarly, consistent with the findings of Kim (2021), the interviews also demonstrated that older adults had concerns about privacy and security risks related to VAs. These concerns were associated with the continuous listening capability of VAs in private spaces like homes. Furthermore, participants worried that others could easily access their personal information by conversing with VAs. Among these risks, financial security was perceived as a more profound concern for some old adults when using VAs. However, some users believed that the security risks posed by VAs could be disregarded. One reason for this belief was cognitive bias, where they were unaware of the risks of using VAs. Additionally, some users were not concerned about the risks due to their usage patterns, as they believed they would not engage in conversations that involved sensitive information. This finding partially supports hypothesis H7.
On the other hand, the interviews revealed that some older adults expressed pleasure and delight in using VAs due to their simplicity, which aligns with previous research findings. Akdim et al. (2022) found that when users perceive virtual communities have humanized interfaces, the perceived enjoyment is enhanced, which could encourage continued engagement. Emotional needs continue to grow with aging. The interaction of VAs, which are naturally simple and create a relaxed atmosphere, meet the emotional needs of older adults and may influence perceived usefulness. Therefore, we add the following hypotheses:
H10: Perceived ease of use positively influences perceived enjoyment.
H11: Perceived enjoyment positively influences perceived usefulness.
Regarding the personal characteristics of older adults, the interviews revealed that the belief in their ability to use VAs successfully may directly influence their usage behavior. If older adults perceive themselves as lacking the capability to use VAs, they might be more inclined to give up on using them. Werner et al. (2023) also found that older adults who willingly use VAs likely have a higher level of confidence in their ability to interact successfully with VAs, while those who do not use VAs may lack the same level of confidence. This finding supports hypothesis H9.
Furthermore, the interviews revealed that older adults tend to find contentment in their existing comfort or stability and are often hesitant to explore unfamiliar environments or adopt new technologies. Many older adults cited this as a reason for their refusal to use VAs. It is inherent in human nature to cling to established habits rather than embracing change and venturing into unknown territories (Mazar and Wood, 2022). In extensive research on resistance to change and individual differences, Oreg (2003) proposed that this pursuit of comfort, stability, and resistance to change is not merely a situational behavior but a fundamental personality trait. Some scholars have emphasized the significance of the DRTC in technology adoption, but its impact has not been thoroughly addressed in IT adoption literature, necessitating further investigation (Mzoughi and M’Sallem, 2013; Touchaei and Hashim, 2023). Currently, DRTC has not received attention in the context of VAs. This study considers DRTC a potential barrier for older adults in adopting VAs, as it can influence their value assessment and usage intention towards VAs. Therefore, we add the following hypotheses:
H12: DRTC positively influences the value barrier.
H13: DRTC negatively influences behavioral intention.
Our research has yielded valuable insights that were previously overlooked by using semi-structured interviews. One such example is the ease of use that typifies VAs. The interviews revealed that ease of use can be a source of pleasure for older individuals and also enhances their perception of the usefulness of VAs. Furthermore, our findings indicated that a predisposition to resist innovation can impact the acceptance of VAs among older adults. Consequently, we have incorporated hypotheses H10 to H13 into the original model, resulting in the final hypothesis model depicted in Figure 4.
To test the proposed model for older adults’ acceptance of VAs, a survey was designed and conducted. Data analyses were conducted using partial least squares structural equation modeling (PLS-SEM).
The selection criteria and participant recruitment process were consistent with Study 1. A total of 154 individuals aged 60 and above were recruited. Among them, 153 participants (Mean age = 70.08, SD = 5.19, 60.1% female) were included in the study. The characteristics of the participants are shown in Table 2. Participants’ experience with VAs can be found in Table 3.
We employed a quantitative approach using structured questionnaires to investigate the impact factors of older adults’ intentions to use VAs. The questionnaire completion process involved face-to-face interviews to facilitate synchronous communication between the interviewer and the older adults while completing the questionnaire. The accuracy of responses was verified by repeating the participants’ answers, which took approximately 30 min each participant. To ensure consistency in the survey, the interviews were conducted by the same experienced researcher. The questionnaire data was entered by two well-trained researchers and cross-checked upon completion.
The survey questionnaire for this study included the variables related to acceptance of VAs and demographic information. Table 4 presents the items related to the acceptance of VAs and its’ impact factors used in the current study. All the variables and indicators in the questionnaire were measured by a seven-point Likert scale ranging from 1 (strongly disagree) to 7 (strongly agree).
Given the potential unfamiliarity of the elderly with VAs, an introductory session was conducted before participants completed the questionnaire, following a similar approach as in Study 1.Older adults who completed the experiment were rewarded with a 30 yuan monetary incentive.
This study used the PLS-SEM approach for data analysis. The measurement model and structural model were analyzed using Smart PLS 3.3.9. PLS-SEM is selected due to its minimal sample size requirements, which are determined by the greater of the following two criteria: (1) ten times the maximum number of formative indicators employed to measure a single construct, or (2) ten times the maximum number of structural paths directed towards a specific latent construct within the structural model. It is suitable for analyzing complex structural equations with multiple latent variables and related items and is applicable for exploratory and predictive models in research and data analysis (Hair et al., 2017). PLS-SEM is widely used in various social sciences and related disciplines (Hair et al., 2019) and has been employed in TAM studies (Sagnier et al., 2020; Xu et al., 2023). As recommended, the data analysis was conducted in two stages. Firstly, we assessed the internal consistency, the convergent validity, and the discriminant validity of the measurement model (Hair et al., 2021). While it is unnecessary to report fit metrics, we reported the Standardized Root Mean Square Residual (SRMR), which is considered useful in detecting model misspecification (Xu et al., 2023). Secondly, following satisfactory results in the first stage, we proceeded with the structural model to test our hypotheses.
Composite Reliability (CR) and Cronbach’s alpha assessed the internal consistency reliability. The combined performance of these two indicators objectively determines the internal quality of the constructed measurement model. Following the standards proposed by Hair et al. (2011), the minimum threshold for both indicators is set at 0.7. As shown in Table 5, all CR values are above 0.8, and all Cronbach’s alpha values are above 0.7.
Convergent validity was evaluated by average variance extracted (AVE) and items outer loading. All latent variables, each consisting of three or more items, have standardized factor loadings greater than 0.7, and the AVE values for all latent variables are above 0.5, indicating high convergent validity of the measurement items in this study (Hair et al., 2021).
To assess discriminant validity, we used the Fornell-Larcker criterion and the HTMT values. Based on the Fornell-Larcker criterion, the bold values on the diagonal represent the square root of the AVE for each latent variable, and the values in the lower half of the diagonal represent the correlations between each latent variable and other latent variables. In this case, all AVE values were more significant than correlations with other constructs, and the HTMT values were less than 0.85 for all the constructs, confirming that the model has satisfactory discriminant validity (Hair et al., 2021). Table 5 presents the factor loadings and AVE values for all the constructs. Tables 6, 7 provide the AVE square root on the diagonal and the correlations among constructs and the HTMT results, respectively.
Moreover, the estimated SRMR value for the model in this study is 0.06, which is below the threshold of 0.08 (Hu and Bentler, 1999; Kline, 2023). This indicates that the model is acceptable in terms of model fit.
Before assessing the inner structural model, common method bias, each construct’s multicollinearity test, model fit evaluation, and descriptive statistics were calculated. To examine whether common method bias was present in our data, we employed Harman’s single-factor test. The highest eigenvalue corresponded to the first component, which accounted for 0.396 of the variances. This falls below the established threshold of 0.4 (Podsakoff et al., 2003), indicating that common method bias was not a significant concern in our study. Multicollinearity was assessed using variance inflation factors (VIF). The results showed that VIF values of all constructs ranged from 1.000 to 1.944, well below the threshold of 5.0 (Hair et al., 2016), indicating the absence of multicollinearity.
We used bootstrapping to test the relationships hypothesized in our model. Path significance was tested using a bootstrapping technique for the 153 cases with 5,000 samples (Hair et al., 2011). Table 8 lists all path coefficients and their significance. To assess the predictive strength of the model, we reported R2 values for each endogenous variable. As a rule of thumb, we followed (Hair et al., 2011) to report the R2 values where R2 of 0.25, 0.50, and 0.75 are considered weak, moderate, and substantial, respectively. Results suggest that our model can explain 67.4% of the variance in behavioral intention (moderate), 27.3% of the variance in perceived usefulness (moderate), 23.8% of the variance in perceived ease of use (weak), 20.7% of the variance in perceived enjoyment (weak), and 12.0% of the variance in value barrier (weak). These R2 values are comparable to those reported in the literature (Xu et al., 2023).
According to the path analysis, perceived usefulness, perceived enjoyment, and technological self-efficacy positively influenced behavioral intention. DRTC had a negative impact on behavioral intention. However, the effects of perceived ease of use, value barrier, and perceived trust on behavioral intention were not significant. This means that H1, H4, H9, and H13 are supported, while H2, H6, and H7 are not supported. Furthermore, perceived ease of use had a positive influence on perceived usefulness and perceived enjoyment, while perceived enjoyment had a positive influence on perceived usefulness. This means that H3, H10, and H11 are supported. Lastly, technological self-efficacy had a positive influence on perceived ease of use, DRTC had a positive influence on value barrier, while value barrier had a negative impact on perceived usefulness. This means that H5, H8, and H12 are supported. Table 8 and Figure 5 present the results of the hypothesis testing and the structural model, respectively.
Figure 5. Results of the structural model of current study Note: *p < 0.05, **p < 0.05, ***p < 0.001; Three dotted lines indicating non-significant paths were added in making all proposed factors shown in an integral model.
This study categorized the factors influencing the acceptance of VAs among older adults into VAs product characteristics and personal characteristics of older adults. Consistent with previous research, it was observed that perceived usefulness, perceived enjoyment, and technological self-efficacy significantly affected behavioral intention (Nguyen et al., 2018; Song et al., 2022; Zhong et al., 2022). However, there were several differences between the model created and the results produced by existing research. Primarily, inconsistencies existed in the research findings. Perceived trust and perceived ease of use were previously identified as significant factors influencing older adults’ acceptance of VAs (Kong and Woods, 2018; Kebede et al., 2022; Song et al., 2022). However, our study did not find evidence supporting this. Nonetheless, our model indicated how ease of use influenced the mechanism of older adults’ acceptance of VAs. Secondly, our research identified other important factors that influenced older adults’ acceptance of voice assistants, such as DRTC, which was not previously highlighted as a significant factor affecting older adults’ acceptance of VAs. The following sections explain the results in detail.
The result showed that perceived usefulness positively influenced behavioral intention, consistent with previous studies on the acceptance of VAs (Song et al., 2022; Zhong et al., 2022), indicating that older adults who perceived the benefits of VAs are more likely to have a higher intention to adopt them. Consequently, older adults may be inclined to embrace VAs due to their perception of VAs as valuable and convenient technologies. Furthermore, results also showed a positive impact of perceived enjoyment on behavioral intention, which was consistent with previous research on VAs (Nguyen et al., 2018; Zhong et al., 2022). As older adults perceive time as limited, they prioritize emotional satisfaction over other goals (Carstensen, 1995), and positive user emotions and experiences contribute to their acceptance of the product. If users experience perceived enjoyment through their interactions, it can lead to an expectation of internal psychological rewards, encouraging them to continue using the technology (Suki and Suki, 2011; Akdim et al., 2022). Moreover, perceived enjoyment positively influenced perceived usefulness, which may be related to fulfilling their emotional needs through positive user experiences. Schlomann et al. (2021) also found that some older adults view smart speakers as social companions, meeting their emotional needs and enhancing their perceived usefulness.
The impact of perceived ease of use on behavioral intention wasn’t significant, which contradicts previous studies (Song et al., 2022). However, perceived ease of use had a direct influence on perceived usefulness and perceived enjoyment. This may be because the effect of perceived ease of use on behavioral intention was indirect. In other words, if older adults perceive the natural and simplistic nature of voice interaction, they would consider VAs useful and enjoyable, leading to the intention to use them. VAs possess the distinctive advantage of being easy to use, allowing older adults to use them effectively without any major operational difficulties. While it is not considered a direct factor that influences the intention to use, it does play a significant role in enhancing the user experience including the perceived usefulness and enjoyment. This indicated the crucial role of perceived usefulness and perceived enjoyment in the process of older adults accepting VAs.
Results indicated that value barrier did not directly influence behavioral intention, suggesting that VAs were not perceived as hindrances. This is not considered a barrier because VAs are not complicated and have a humanized interface. However, value barrier negatively impacted perceived usefulness, indicating that if older adults perceived VAs as less valuable compared to existing methods, they might have had doubts about their practicality. The role of value in motivating older adults to adopt new technologies was crucial (Melenhorst et al., 2006). Older adults need to have gained more benefits than the effort required to understand and use VAs to perceive no value barriers.
The research indicated that perceived trust did not directly influence behavioral intention, but numerous studies found that fear of safety and invasion of privacy were barriers to digital engagement (Fischl et al., 2017; Kong and Woods, 2018; Zambianchi et al., 2019; Kebede et al., 2022). However, privacy calculus theory posits that disclosing personal information is based on a trade-off between perceived privacy risks and perceived benefits of information disclosure (Dinev and Hart, 2006). If the perceived benefits of information disclosure outweigh the perceived risks, users may be willing to disclose personal information despite their privacy concerns. Schomakers and Ziefle (2022) suggested that as long as certain boundaries are not crossed, security-related benefits may outweigh privacy concerns. For older adults, although they are aware that using VAs may lead to a partial loss of privacy due to their real-time listening capabilities, they may still choose to use them due to other features, such as simple and enjoyable interactions, after weighing the trade-offs. Additionally, the COVID-19 pandemic has increased social isolation among older adults, impacting their sense of security at home and their need for support, particularly for those living alone, which may lead them to be more willing to disclose some information to enhance their sense of security (Conroy et al., 2020; Schomakers and Ziefle, 2022).
The research findings indicated that technological self-efficacy had a significantly positive impact on perceived ease of use, consistent with previous studies on VAs (Song et al., 2022). Moreover, technological self-efficacy also significantly positively influenced behavioral intention, and it was the strongest predictor of older adults’ intention to use VAs, suggesting older adults’ perception of their capabilities plays a substantial role in the acceptance of VAs. Although this contradicted the conclusions of TAM, which indicated that self-efficacy was not considered as a predictor of behavioral intention (Venkatesh and Bala, 2008), TAM’s samples mainly consisted of young individuals, who generally possessed higher levels of self-efficacy in using technology (Czaja et al., 2006). Recent research suggested that older adults’ technology usage was more driven by their perception of their abilities than the technological features represented in TAM (Jokisch et al., 2021). This could be attributed to cohort effects, as the current generation of adults has already acquired basic information and communication technology skills daily, leading to a generally higher rate of technology adoption (Charness and Boot, 2022). They have more role models in their social environment who demonstrate beneficial integration of the internet into daily life or provide support in case of technological issues. However, these advantages may not be as prevalent among older adults. As a result, technological self-efficacy emerged as the most influential factor in predicting the acceptance of VAs among older adults, surpassing the significance of perceived usefulness.
The result revealed the negative impact of DRTC on behavioral intention, indicating the inhibiting effect of DRTC on seniors’ intention to adopt VAs. Devaraj et al. (2008) also suggested that incorporating personality traits into theoretical models can enhance their predictive ability in explaining technology adoption by users. The result of this study could partly explain why some seniors show reluctance in VAs adoption. Since VAs are regarded as an innovation, they may be incompatible with their habits and of little practical use. Furthermore, older adults exhibit a high level of resistance to change and technological innovation (Hoque and Sorwar, 2017), and the tendency to embrace familiarity impedes their experience of VAs.
In addition, DRTC positively influenced value barrier, indicating that older adults with a high inclination towards resisting innovation tend to perceive VAs as less valuable than existing products. This may be because VAs disrupt their traditional way of life. Individuals accustomed to and satisfied with their conventional lifestyle may alter their value assessment of VAs to maintain the status quo and persuade themselves (Talukder et al., 2020).
Over the past decade, VAs have become widespread and integrated into various devices used in various scenarios. The benefits of VAs should be accessible to everyone, especially vulnerable groups like older adults (Song et al., 2022). Initially designed for younger users, research on VAs has paid less attention to the personal characteristics of older adults. However, due to the heterogeneity of their physiological and psychological functions, older adults differ significantly from younger individuals regarding technology acceptance (Kim and Choudhury, 2021). This study identified factors influencing the acceptance of VAs among older adults, contributing to the development of enhanced voice assistants to facilitate the adoption of smart technology among seniors. Moreover, previous research has indicated that VAs support older adults in various domains. They assist in daily health activities, such as health monitoring, medication management, and meal planning, thereby enhancing overall well-being. VAs also serve as social companions to some extent, addressing social isolation and loneliness. By identifying factors influencing older adults’ acceptance of VAs, this study sheds light on the social issues faced by this demographic, including social isolation and loneliness, health and well-being concerns, ageism and stereotypes, as well as technology accessibility.
Regarding VA product features, the critical focus of improvement and optimization should be enhancing speech recognition and natural language processing quality. The perceived practicality, ease of use, and enjoyment of VAs depend on speech recognition accuracy and natural language processing capabilities. Specifically, efforts can be made to reduce the impact of environmental noise and user accents on speech recognition. Providing appropriate feedback, such as offering reasons for query failures or suggestions to improve query results, can guide users correctly and enhance the responsiveness of VAs.
Concerning the negative impact of value barrier on perceived usefulness, it is worth noting that smart voice products, such as smart speakers, are not specifically designed for older users, and many of the functions they need may not be available. Therefore, adding more features suitable for older adults, such as fall detection alerts, medication purchases, and hospital appointment arrangements, can enhance older adults’ perception of usefulness.
Furthermore, concerning the positive impact of perceived enjoyment on the use of VAs among older adults, it is essential to consider the prevalent risk of social isolation and susceptibility to feelings of loneliness and depression faced by this vulnerable demographic. Designers should contemplate crafting a unique persona for VAs, which can significantly reduce the psychological distance perceived by older users. Meticulous consideration should be given to creating an ideal personality, encompassing gender, tone, and speaking style. Given that older adults bear a lower cognitive load, employing easily memorable and concise vocabulary, such as “OK” and “got it,” can be beneficial (Song et al., 2022). Designers can also endeavor to break free from passive reliance on users’ established patterns and actively engage with them. Initiating greetings and informal conversations or increasing interaction during tasks can make the user experience more enjoyable and engaging.
Although trust does not directly influence the acceptance of VAs among older adults, it does not diminish the importance of trust-related concerns to them. Trust considerations may very well be a part of the deliberation process for older individuals. Given that voice data constitutes a common and significant category of information in people’s daily lives, designers should prioritize enhancing user data storage and utilization transparency. Providing clear information on how users can access and delete their voice data and offering privacy protection clauses can foster a sense of trust and reassurance among older users.
Regarding the personal characteristics of older adults, this study further reveals that individuals with higher levels of technological self-efficacy demonstrate greater ease of use and willingness to use VAs. When promoting VAs, marketers should adopt different communication strategies for older adults with varying levels of self-efficacy. For those with lower self-efficacy, it is essential to emphasize the ease of use of VAs, particularly highlighting the advantages over traditional interaction methods.
Considering the negative impact of DRTC on behavioral intention, personalized services tailored to their preferences could be implemented. VAs could be designed to learn and adapt to users’ interactions in real-time, identifying recurring usage patterns and adapting to individual habits. Allowing users to customize voice output settings, such as speech speed, tone, and intensity, can accommodate the lifestyle preferences of older adults and reduce the influence of DRTC. Furthermore, VAs should possess the capability to operate a wide array of devices, including smartphones, televisions, computers, and such. This expansion of usage scenarios for various applications facilitates the seamless integration of intelligent services into users’ lives (Abdolrahmani et al., 2018; Esau et al., 2022), thereby attenuating the impact of DRTC.
The raw data supporting the conclusions of this article will be made available by the authors, without undue reservation.
Ethical review and approval was not required for the study on human participants in accordance with the local legislation and institutional requirements. All participants signed an informed consent form.
XC: Conceptualization, Data curation, Formal analysis, Investigation, Methodology, Project administration, Resources, Software, Supervision, Validation, Writing – original draft, Writing – review & editing. HZ: Conceptualization, Data curation, Formal analysis, Investigation, Methodology, Validation, Writing – original draft, Writing – review & editing. BZ: Conceptualization, Data curation, Formal analysis, Methodology, Validation, Writing – review & editing, Investigation. DW: Conceptualization, Project administration, Resources, Supervision, Writing – review & editing, Methodology. CC: Conceptualization, Data curation, Investigation, Writing – review & editing. XB: Conceptualization, Project administration, Resources, Supervision, Writing – original draft, Writing – review & editing, Methodology.
This research was supported by the National Social Science Fund of China for the Major Program 2020 (20ZDA079).
The authors declare that the research was conducted in the absence of any commercial or financial relationships that could be construed as a potential conflict of interest.
All claims expressed in this article are solely those of the authors and do not necessarily represent those of their affiliated organizations, or those of the publisher, the editors and the reviewers. Any product that may be evaluated in this article, or claim that may be made by its manufacturer, is not guaranteed or endorsed by the publisher.
Abdolrahmani, A., Kuber, R., and Branham, S. M. (2018). “‘Siri talks at you’ an empirical investigation of voice-activated personal assistant (VAPA) usage by individuals who are blind”. In Proceedings of the 20th international ACM SIGACCESS conference on computers and accessibility, pp. 249–258.
Agag, G., and El-Masry, A. A. (2016). Understanding consumer intention to participate in online travel community and effects on consumer intention to purchase travel online and WOM: an integration of innovation diffusion theory and TAM with trust. Comput. Hum. Behav. 60, 97–111. doi: 10.1016/j.chb.2016.02.038
Akdim, K., Casaló, L. V., and Flavián, C. (2022). The role of utilitarian and hedonic aspects in the continuance intention to use social mobile apps. J. Retail. Consum. Serv. 66:102888. doi: 10.1016/j.jretconser.2021.10288
Bonilla, K., and Martin-Hammond, A. (2020). Older adults’ perceptions of intelligent voice assistant privacy, transparency, and online privacy guidelines. In Sixteenth symposium on usable privacy and security (SOUPS 2020).
Borraz-Mora, J., Bordonaba-Juste, V., and Polo-Redondo, Y. (2017). Functional barriers to the adoption of electronic banking: the moderating effect of gender. Rev. Econ. Apl. 25, 87–107.
Braun, V., and Clarke, V. (2006). Using thematic analysis in psychology. Qual. Res. Psychol. 3, 77–101. doi: 10.1191/1478088706qp063oa
Calahorra-Candao, G., and Hoyos, M. J. M.-d. (2024). The effect of anthropomorphism of virtual voice assistants on perceived safety as an antecedent to voice shopping. Comput. Hum. Behav. 153:108124. doi: 10.1016/j.chb.2023.108124
Carstensen, L. L. (1995). Evidence for a life-span theory of socioemotional selectivity. Curr. Dir. Psychol. Sci. 4, 151–156. doi: 10.1111/1467-8721.ep11512261
Carstensen, L. L., Shavit, Y. Z., and Barnes, J. T. (2020). Age advantages in emotional experience persist even under threat from the COVID-19 pandemic. Psychol. Sci. 31, 1374–1385. doi: 10.1177/0956797620967261
Charness, N., and Boot, W. R. (2022). A grand challenge for psychology: reducing the age-related digital divide. Curr. Dir. Psychol. Sci. 31, 187–193. doi: 10.1177/09637214211068144
Chen, K., and Chan, A. H. S. (2014). Gerontechnology acceptance by elderly Hong Kong Chinese: a senior technology acceptance model (STAM). Ergonomics 57, 635–652. doi: 10.1080/00140139.2014.895855
Conroy, K., Krishnan, S., Mittelstaedt, S., and Patel, S. S. (2020). Technological advancements to address elderly loneliness: practical considerations and community resilience implications for COVID-19 pandemic. Work. Older People 24, 257–264. doi: 10.1108/wwop-07-2020-0036
Corbett, C. F., Combs, E. M., Wright, P. J., Owens, O. L., Stringfellow, I., Nguyen, T., et al. (2021). Virtual home assistant use and perceptions of usefulness by older adults and support person dyads. Int. J. Environ. Res. Public Health 18:1113. doi: 10.3390/ijerph18031113
Czaja, S. J., Charness, N., Fisk, A. D., Hertzog, C., Nair, S. N., Rogers, W. A., et al. (2006). Factors predicting the use of technology: findings from the center for research and education on aging and technology enhancement (create). Psychol. Aging 21, 333–352. doi: 10.1037/0882-7974.21.2.333
Davis, F. D. (1989). Perceived usefulness, perceived ease of use, and user acceptance of information technology. Manag. Inf. Syst. Q. 13:319. doi: 10.2307/249008
Davis, F. D., Bagozzi, R. P., and Warshaw, P. R. (1992). Extrinsic and intrinsic motivation to use computers in the workplace 1. J. Appl. Soc. Psychol. 22, 1111–1132. doi: 10.1111/j.1559-1816.1992.tb00945.x
Department of Economic and Social Affairs of United Nations . (2022). World Population Prospects 2022. Available at: https://www.un.org/development/desa/pd/sites/www.un.org.development.desa.pd/files/wpp2022_summary_of_results.pdf
Devaraj, S., Easley, R. F., and Crant, J. M. (2008). Research note—how does personality matter? Relating the five-factor model to technology acceptance and use. Inf. Syst. Res. 19, 93–105. doi: 10.1287/isre.1070.0153
Diehl, C., Tavares, R., Abreu, T., Almeida, A. M. P., Silva, T. E., Santinha, G., et al. (2022). Perceptions on extending the use of technology after the COVID-19 pandemic resolves: a qualitative study with older adults. Int. J. Environ. Res. Public Health 19:14152. doi: 10.3390/ijerph192114152
Dinev, T., and Hart, P. (2006). An extended privacy calculus model for E-commerce transactions. Inf. Syst. Res. 17, 61–80. doi: 10.1287/isre.1060.0080
Dotzauer, K., and Haiss, F. (2017). Barriers towards the adoption of mobile payment services: An empirical investigation of consumer resistance in the context of Germany. Master’s Thesis. Karlstad University.
Esau, M., Krauß, V., Lawo, D., and Stevens, G. (2022). Losing its touch: understanding user perception of multimodal interaction and smart assistance. Designing Interactive Systems Conference.
Fischl, C., Asaba, E., and Nilsson, I. (2017). Exploring potential in participation mediated by digital technology among older adults. J. Occup. Sci. 24, 314–326. doi: 10.1080/14427591.2017.1340905
Fishbein, M., and Ajzen, I. (1975). Belief, attitude, intention and behaviour: An introduction to theory and research 27. Boston, MA: Addison-Wesley.
Folstein, M. F., Folstein, S. E., and McHugh, P. R. (1975). Mini-mental state. J. Psychiatr. Res. 12, 189–198. doi: 10.1016/0022-3956(75)90026-6
Hair, J. F., Hollingsworth, C. L., Randolph, A. B., and Chong, A. Y. (2017). An updated and expanded assessment of PLS-SEM in information systems research. Ind. Manag. Data Syst. 117, 442–458. doi: 10.1108/imds-04-2016-0130
Hair, J. F. Jr, Hult, G. T. M., Ringle, C., and Sarstedt, M. (2016). A primer on partial least squares structural equation modeling (PLS-SEM). Thousand Oaks, CA: SAGE Publications.
Hair, J. F. Jr, Hult, G. T. M., Ringle, C. M., Sarstedt, M., Danks, N. P., and Ray, S. (2021). Partial least squares structural equation modeling (PLS-SEM) using R: A workbook. London: Springer Nature
Hair, J. F., Ringle, C. M., and Sarstedt, M. (2011). PLS-SEM: indeed a silver bullet. J. Mark. Theory Pract. 19, 139–152. doi: 10.2753/mtp1069-6679190202
Hair, J. F., Risher, J. J., Sarstedt, M., and Ringle, C. M. (2019). When to use and how to report the results of PLS-SEM. Eur. Bus. Rev. 31, 2–24. doi: 10.1108/ebr-11-2018-0203
Hassenzahl, M. (2018). “The thing and I: understanding the relationship between user and product” in Funology 2. Human-computer interaction series. eds. M. Blythe and A. Monk (Cham: Springer), 301–313.
Hew, J. J., Leong, L. Y., Tan, G. W. H., Ooi, K. B., and Lee, V. H. (2019). The age of mobile social commerce: an artificial neural network analysis on its resistances. Technol. Forecast. Soc. Chang. 144, 311–324. doi: 10.1016/j.techfore.2017.10.007
Hoque, M. R., and Sorwar, G. (2017). Understanding factors influencing the adoption of mHealth by the elderly: an extension of the UTAUT model. Int. J. Med. Inform. 101, 75–84. doi: 10.1016/j.ijmedinf.2017.02.002
Hu, L., and Bentler, P. M. (1999). Cutoff criteria for fit indexes in covariance structure analysis: conventional criteria versus new alternatives. Struct. Equ. Model. 6, 1–55. doi: 10.1080/10705519909540118
Jeng, M., Pai, F., and Yeh, T. (2022). Antecedents for older adults’ intention to use smart health wearable devices-technology anxiety as a moderator. Behav. Sci. 12:114. doi: 10.3390/bs12040114
Jokisch, S., Scheling, L., Doh, M., and Wahl, H. W. (2021). Contrasting internet adoption in early and advanced old age: does internet self-efficacy matter? J. Gerontol. B 77, 312–320. doi: 10.1093/geronb/gbab096
Juniper Research (2021) Voice assistants: market forecasts, monetization strategies, competitive landscape 2021-2026. Juniper Research. Available at: https://www.juniperresearch.com/researchstore/innovation-disruption/voice-assistants-market-research-report?ch=smart%20speaker
Kaur, P., Dhir, A., Singh, N., Sahu, G. P., and Almotairi, M. (2020). An innovation resistance theory perspective on mobile payment solutions. J. Retail. Consum. Serv. 55:102059. doi: 10.1016/j.jretconser.2020.102059
Kebede, A. S., Ozolins, L., Holst, H., and Galvin, K. (2022). Digital engagement of older adults: scoping review. J. Med. Internet Res. 24:e40192. doi: 10.2196/40192
Kim, S. (2021). Exploring how older adults use a smart speaker-based voice assistant in their first interactions: qualitative study. JMIR Mhealth Uhealth 9:e20427. doi: 10.2196/20427
Kim, S., and Choudhury, A. (2021). Exploring older adults’ perception and use of smart speaker-based voice assistants: a longitudinal study. Comput. Hum. Behav. 124:106914. doi: 10.1016/j.chb.2021.106914
Kline, R. B. (2023). Principles and practice of structural equation modeling. New York City: Guilford Publications.
Kong, L., and Woods, O. (2018). Smart eldercare in Singapore: negotiating agency and apathy at the margins. J. Aging Stud. 47, 1–9. doi: 10.1016/j.jaging.2018.08.001
Kowalski, J., Jaskulska, A., Skorupska, K., Abramczuk, K., Biele, C., Kopeć, W., et al. (2019). Older adults and voice interaction: a pilot study with Google home. Ar xiv (Cornell University). Available at: http://arxiv.org/abs/1903.07195
Kuoppamäki, S., Jaberibraheem, R., Hellstrand, M., and McMillan, D. (2023). Designing multi-modal conversational agents for the kitchen with older adults: a participatory design study. Int. J. Soc. Robot. 15, 1507–1523. doi: 10.1007/s12369-023-01055-4
Laukkanen, T. (2016). Consumer adoption versus rejection decisions in seemingly similar service innovations: the case of the internet and mobile banking. J. Bus. Res. 69, 2432–2439. doi: 10.1016/j.jbusres.2016.01.013
Laukkanen, P., Sinkkonen, S., and Laukkanen, T. (2008). Consumer resistance to internet banking: postponers, opponents and rejectors. Int. J. Bank Mark. 26, 440–455. doi: 10.1108/02652320810902451
Lawton, M. P., and Brody, E. M. (1969). Assessment of older people: self-maintaining and instrumental activities of daily living. Gerontologist 9, 179–186. doi: 10.1093/geront/9.3_part_1.179
Lee, G., and Kim, Y. (2022). Effects of resistance barriers to service robots on alternative attractiveness and intention to use. SAGE Open 12:215824402210992. doi: 10.1177/21582440221099293
Leong, L.-Y., Hew, T.-S., Ooi, K.-B., and Lin, B. (2021). A meta-analysis of consumer innovation resistance: is there a cultural invariance? Ind. Manag. Data Syst. 121, 1784–1823. doi: 10.1108/imds-12-2020-0741
Li, J., Ma, Q., Chan, A. H., and Man, S. S. (2019). Health monitoring through wearable technologies for older adults: Smart wearables acceptance model. Applied ergonomics, 75, 162–169. doi: 10.1016/j.apergo.2018.10.006
Lian, J. W., and Yen, D. C. (2014). Online shopping drivers and barriers for older adults: age and gender differences. Comput. Hum. Behav. 37, 133–143. doi: 10.1016/j.chb.2014.04.028
Liu, M., Wang, C., and Hu, J. (2023). Older adults’ intention to use voice assistants: usability and emotional needs. Heliyon 9:e21932. doi: 10.1016/j.heliyon.2023.e21932
Ma, Q., Chan, A. H. S., and Teh, P.-L. (2021). Insights into older adults’ technology acceptance through meta-analysis. Int. J. Hum.-Comput. Interact. 37, 1049–1062. doi: 10.1080/10447318.2020.1865005
Mazar, A., and Wood, W. (2022). Illusory feelings, elusive habits: people overlook habits in explanations of behavior. Psychol. Sci. 33, 563–578. doi: 10.1177/09567976211045345
Melenhorst, A. S., Rogers, W. A., and Bouwhuis, D. (2006). Older adults’ motivated choice for technological innovation: evidence for benefit-driven selectivity. Psychol. Aging 21, 190–195. doi: 10.1037/0882-7974.21.1.190
Moorthy, K., Ching, S., Fatt, Y. W., Yee, C. M., Yin, E. C. K., Yee, K. S. P., et al. (2017). Barriers of mobile commerce adoption intention: perceptions of generation X in Malaysia. J. Theor. Appl. Electron. Commer. Res. 12, 37–53. doi: 10.4067/s0718-18762017000200004
Mzoughi, M. N., and M’Sallem, W. (2013). Predictors of internet banking adoption. Int. J. Bank Mark. 31, 388–408. doi: 10.1108/ijbm-10-2012-0105
Nagle, S., and Schmidt, L. (2012). Computer acceptance of older adults. Work (Reading, Mass.), 41, 3541–3548. doi: 10.3233/WOR-2012-0633-3541
Nallam, P., Bhandari, S., Sanders, J., and Martin-Hammond, A. (2020). A question of access: exploring the perceived benefits and barriers of intelligent voice assistants for improving access to consumer health resources among low-income older adults. Gerontol. Geriatr. Med. 6:233372142098597. doi: 10.1177/2333721420985975
National Bureau of Statistics of China . (2023) Wang Pingping: The total population decreased slightly and the level of urbanization continued to improve. Available at: https://www.stats.gov.cn/xxgk/jd/sjjd2020/202301/t20230118_1892285.html
Nguyen, Q. N., Ta, A., and Prybutok, V. R. (2018). An integrated model of voice-user interface continuance intention: the gender effect. Int. J. Hum.-Comput. Interact. 35, 1362–1377. doi: 10.1080/10447318.2018.1525023
Oreg, S. (2003). Resistance to change: developing an individual differences measure. J. Appl. Psychol. 88, 680–693. doi: 10.1037/0021-9010.88.4.680
Ossadnik, J., Muehlfeld, K., and Goerke, L. (2023). Man or machine–or something in between? Social responses to voice assistants at work and their effects on job satisfaction. Comput. Hum. Behav. 149:107919. doi: 10.1016/j.chb.2023.107919
Peral-Peral, B., Villarejo-Ramos, A. F., and Arenas-Gaitan, J. (2020). Self-efficacy and anxiety as determinants of older adults' use of internet banking services. Univ. Access Inf. Soc. 19, 825–840. doi: 10.1007/s10209-019-00691-w
Pham Thi, T. D., and Duong, N. T. (2022). Do people intend to use AI voice assistants? An empirical study in Vietnam. J. Hum. Behav. Soc. Environ. 33, 859–878. doi: 10.1080/10911359.2022.2106003
Phibbs, C. L. (2021). The impact of motivation, price, and habit on intention to use IoT-enabled technology: a correlational study [doctoral dissertation, Capella University]. Pro Quest Dissertations & Theses Global, Ann Arbor, MI.
Podsakoff, P. M., Mac Kenzie, S. B., Lee, J. Y., and Podsakoff, N. P. (2003). Common method biases in behavioral research: a critical review of the literature and recommended remedies. J. Appl. Psychol. 88, 879–903. doi: 10.1037/0021-9010.88.5.879
Pradhan, A., Lazar, A., and Findlater, L. (2020). Use of intelligent voice assistants by older adults with low technology use. ACM Trans. Comput.-Hum. Interact. 27, 1–27. doi: 10.1145/3373759
Ram, S., and Sheth, J. N. (1989). Consumer resistance to innovations: the marketing problem and its solutions. J. Consum. Mark. 6, 5–14. doi: 10.1108/eum0000000002542
Sagnier, C., Loup-Escande, E., Lourdeaux, D., Thouvenin, I., and Valléry, G. (2020). User acceptance of virtual reality: an extended technology acceptance model. Int. J. Hum.-Comput. Interact. 36, 993–1007. doi: 10.1080/10447318.2019.1708612
Schlomann, A., Wahl, H., Zentel, P., Heyl, V., Knapp, L., Opfermann, C., et al. (2021). Potential and pitfalls of digital voice assistants in older adults with and without intellectual disabilities: relevance of participatory design elements and ecologically valid field studies. Front. Psychol. 12:684012. doi: 10.3389/fpsyg.2021.684012
Schomakers, E., and Ziefle, M. (2022). Privacy vs. security: trade-offs in the acceptance of smart technologies for aging-in-place. Int. J. Hum.-Comput. Interact. 39, 1043–1058. doi: 10.1080/10447318.2022.2078463
Snyder, R. G. (1961). Vibrational spectra of crystalline n-paraffins: II. Intermolecular effects. J. Mol. Spectrosc. 7, 116–144. doi: 10.1016/0022-2852(61)90347-2
Song, Y., Yanpu, Y., and Cheng, P. (2022). The investigation of adoption of voice-user interface (VUI) in smart home systems among Chinese older adults. Sensors 22:1614. doi: 10.3390/s22041614
Suki, N. M., and Suki, N. M. (2011). Exploring the relationship between perceived usefulness, perceived ease of use, perceived enjoyment, attitude and subscribers’ intention towards using 3G mobile services. J. Inf. Technol. Manag. 22, 1–7.
Talke, K., and Heidenreich, S. (2014). How to overcome pro-change bias: incorporating passive and active innovation resistance in innovation decision models. J. Prod. Innov. Manag. 31, 894–907. doi: 10.1111/jpim.12130
Talukder, M. S., Sorwar, G., Bao, Y. K., Ahmed, J. U., and Palash, M. A. S. (2020). Predicting antecedents of wearable healthcare technology acceptance by elderly: a combined SEM-neural network approach. Technol. Forecast. Soc. Chang. 150:119793. doi: 10.1016/j.techfore.2019.119793
Touchaei, S. A., and Hashim, N. H. (2023). The antecedents of mobile banking adoption among senior citizens in Malaysia. Int. J. Hum.-Comput. Interact. 1–18. doi: 10.1080/10447318.2022.2161236
Trajkova, M., and Martin-Hammond, A. (2020). "Alexa is a toy": exploring older adults' reasons for using, limiting, and abandoning Echo. In Proceedings of the 2020 CHI conference on human factors in computing systems, pp. 1–13.
Turow, J. (2021). Journalism and the voice intelligence industry. Digit. J. 9, 1000–1006. doi: 10.1080/21670811.2020.1829979
Venkatesh, V., and Bala, H. (2008). Technology acceptance model 3 and a research agenda on interventions. Decis. Sci. 39, 273–315. doi: 10.1111/j.1540-5915.2008.00192.x
Venkatesh, V., and Davis, F. (2000). A theoretical extension of the technology acceptance model: four longitudinal field studies. Manag. Sci. 46, 186–204. doi: 10.1287/mnsc.46.2.186.11926
Venkatesh, V., Morris, M., Davis, G., and Davis, F. (2003). User acceptance of information technology: toward a unified view. MIS Q. 27, 425–478. doi: 10.2307/30036540
Werner, L., Huang, G., and Pitts, B. J. (2023). Smart speech systems: a focus group study on older adult user and non-user perceptions of speech interfaces. Int. J. Hum.-Comput. Interact. 39, 1149–1161. doi: 10.1080/10447318.2022.2050541
Xu, W., Liang, H. N., Yu, K., Wen, S., Baghaei, N., and Tu, H. (2023). Acceptance of virtual reality exergames among Chinese older adults. Int. J. Hum.-Comput. Interact. 39, 1134–1148. doi: 10.1080/10447318.2022.2098559
Yan, C., Johnson, K., and Jones, V. K. (2024). The impact of interaction time and verbal engagement with personal voice assistants on alleviating loneliness among older adults: an exploratory study. Int. J. Environ. Res. Public Health 21:100. doi: 10.3390/ijerph21010100
Yu, J., de Antonio, A., and Villalba-Mora, E. (2022). Design of an Integrated Acceptance Framework for Older Users and eHealth: Influential Factor Analysis. J Med Internet Res. 24, 31920. doi: 10.2196/31920
Zambianchi, M., Rönnlund, M., and Carelli, M. G. (2019). Attitudes towards and use of information and communication technologies (ICTs) among older adults in Italy and Sweden: the influence of cultural context, socio-demographic factors, and time perspective. J. Cross Cult. Gerontol. 34, 291–306. doi: 10.1007/s10823-019-09370-y
Zeng, F., and Chen, T. (2022). A study of the acceptability of smart homes to the future elderly in China. Univ. Access Inf. Soc. 22, 1007–1025. doi: 10.1007/s10209-022-00871-1
Zhao, Y., Ni, Q., and Zhou, R. (2018). What factors influence the mobile health service adoption? A meta-analysis and the moderating role of age. Int. J. Inf. Manag., 43, 342–350. doi: 10.1016/j.ijinfomgt.2017.08.006
Zhong, R., Ma, M., Zhou, Y., Lin, Q., Li, L., and Zhang, N. (2022). User acceptance of smart home voice assistant: a comparison among younger, middle-aged, and older adults. Univ. Access Inf. Soc. 1-18. doi: 10.1007/s10209-022-00936-1
Zhou, J., Zhang, B., Tan, R., Tseng, M.-L., and Zhang, Y. (2020). Exploring the systematic attributes influencing gerontechnology adoption for elderly users using a meta-analysis. Sustain. For. 12:2864. doi: 10.3390/su12072864
Keywords: older adults, voice assistants, technology acceptance model, behavior intention, dispositional resistance to change
Citation: Cao X, Zhang H, Zhou B, Wang D, Cui C and Bai X (2024) Factors influencing older adults’ acceptance of voice assistants. Front. Psychol. 15:1376207. doi: 10.3389/fpsyg.2024.1376207
Received: 25 January 2024; Accepted: 26 February 2024;
Published: 07 March 2024.
Edited by:
Zhiwei Zheng, Chinese Academy of Sciences (CAS), ChinaCopyright © 2024 Cao, Zhang, Zhou, Wang, Cui and Bai. This is an open-access article distributed under the terms of the Creative Commons Attribution License (CC BY). The use, distribution or reproduction in other forums is permitted, provided the original author(s) and the copyright owner(s) are credited and that the original publication in this journal is cited, in accordance with accepted academic practice. No use, distribution or reproduction is permitted which does not comply with these terms.
*Correspondence: Xuejun Bai, Ynh1ZWp1bkAxMjYuY29t
Disclaimer: All claims expressed in this article are solely those of the authors and do not necessarily represent those of their affiliated organizations, or those of the publisher, the editors and the reviewers. Any product that may be evaluated in this article or claim that may be made by its manufacturer is not guaranteed or endorsed by the publisher.
Research integrity at Frontiers
Learn more about the work of our research integrity team to safeguard the quality of each article we publish.