- 1Key Laboratory of Cognition and Personality, Ministry of Education, Southwest University, Chongqing, China
- 2Faculty of Psychology, Southwest University, Chongqing, China
- 3College of Teacher Education, Southwest University, Chongqing, China
Background: Rumination impedes problem solving and is one of the most important factors in the onset and maintenance of multiple psychiatric disorders. The current study aims to investigate the impact of social rejection on rumination and explore the underlying neural mechanisms involved in this process.
Methods: We utilized psychological questionnaire and resting-state brain imaging data from a sample of 560 individuals. The predictive model for rumination scores was constructed using resting-state functional connectivity data through connectome-based predictive modeling. Additionally, a mediation analysis was conducted to investigate the mediating role of the prediction network in the relationship between social rejection and rumination.
Results: A positive correlation between social rejection and rumination was found. We obtained the prediction model of rumination and found that the strongest contributions came from the intra- and internetwork connectivity within the default mode network (DMN), dorsal attention network (DAN), frontoparietal control network (FPCN), and sensorimotor networks (SMN). Analysis of node strength revealed the significance of the supramarginal gyrus (SMG) and angular gyrus (AG) as key nodes in the prediction model. In addition, mediation analysis showed that the strength of the prediction network mediated the relationship between social rejection and rumination.
Conclusion: The findings highlight the crucial role of functional connections among the DMN, DAN, FPCN, and SMN in linking social rejection and rumination, particular in brain regions implicated in social cognition and emotion, namely the SMG and AG regions. These results enhance our understanding of the consequences of social rejection and provide insights for novel intervention strategies targeting rumination.
1. Introduction
Rumination refers to an individual’s passive and repeated recollection, reflection, and analysis of problems in a negative emotional state and is a negative style of cognitive appraisal of painful events (Nolen-Hoeksema, 1991; Nolen-Hoeksema et al., 2008). In a recent review, Watkins and Roberts (2020) defined rumination as repetitive thoughts about the symptoms, causes, circumstances, meanings, and consequences of negative mood. Research suggests that rumination is not only associated with emotional and mental health problems such as anxiety (Olatunji et al., 2013), depression (Spasojević and Alloy, 2001), and sadness (Kirkegaard Thomsen, 2006) but may also lead to impaired memory and executive control (Davis and Nolen-Hoeksema, 2000; Watkins and Brown, 2002) as well as cognitive dissonance (through excessive attention to and memory of negative information)(Watkins and Teasdale, 2001; Whitmer and Gotlib, 2013) and impaired positive problem solving (Lyubomirsky and Nolen-Hoeksema, 1995; Watkins and Baracaia, 2002). Theories suggest that rumination is one of the key factors in the maintenance of depression and other disorders (Lyubomirsky and Nolen-Hoeksema, 1995). Rumination can exacerbate neurological disorders in several ways, including amplifying and prolonging existing negative emotions and thoughts, interfering with problem solving and positive instrumental behavior, and reducing sensitivity to changing circumstances (Nolen-Hoeksema et al., 2008; Offredi et al., 2016; Watkins and Roberts, 2020). In other words, such self-focused rumination often leads to maladaptive responses and chronic pain, resulting in impaired problem-solving skills and increased negative emotions, and may form a vicious cycle. It is crucial to have an in-depth comprehension of the neural mechanisms underlying rumination, which can contribute to enhancing our understanding of the etiology and mechanisms of these disorders, thereby increasing diagnostic accuracy and providing valuable insights for treatment and intervention.
An important feature of rumination is an intense self-focus (Watkins and Moulds, 2005), and this constant, self-referential cognitive activity is closely linked to the default mode network (DMN) (Berman et al., 2011; Hamilton et al., 2015; Rosenbaum et al., 2017; Zhou et al., 2020), which supports internal reflection and autobiographical memory (Buckner et al., 2008). The DMN can be further divided into three functionally distinct subsystems; researchers found that the subsystems of the DMN were not equally active during rumination and exhibited altered functional connectivity (Chen et al., 2020; Zhou et al., 2020). Another important feature of rumination is that it is associated with continuous negative emotions (Thomsen et al., 2003), which is related to brain regions involved in emotion regulation. For example, several studies have shown a significant correlation between rumination and activation of the amygdala (Ray et al., 2005; Mandell et al., 2014; Belzung et al., 2015). Furthermore, researchers found that depressed patients with high levels of rumination had reduced nodal centrality of the amygdala during task performance (Zhang et al., 2020). This suggests that the amygdala is unable to play a full role in emotion regulation in rumination, resulting in lack of regulation of emotional states. In addition, individuals tend to ignore changes in the external environment during rumination. As previous studies have found, the dorsal attentional network (DAN), which helps to focus and maintain attention to external stimuli, is negatively associated with rumination (Lois and Wessa, 2016; Rosenbaum et al., 2018a,b). Reduced functional connectivity has also been found between the frontoparietal network and DAN in depressed patients with high levels of rumination (Kaiser et al., 2015). To summarize, rumination is closely related to brain networks responsible for functions such as self-referential processing, attentional control, and emotion regulation.
While numerous studies have examined the effects of rumination, much less attention has been given to the factors that influence rumination. Rumination, as a complex cognitive process, can be influenced by a variety of factors, such as personality traits, genes, environmental conditions, events, and feedback modalities (Cox et al., 2010; Johnson et al., 2014; Watkins and Roberts, 2020). Although the social environment plays an important role in individual growth, few studies have investigated the effects of social relationships on rumination. Indeed, studies have shown that the negative effects of rejection are not limited to the emotional and psychological levels but may also extend to cognition and thought patterns (Voncken et al., 2008; Gunther Moor et al., 2010; Slavich et al., 2010; Rudert et al., 2021). For example, social rejection has been shown to lead to negative thought patterns (Monroe et al., 2007; Cacioppo and Hawkley, 2009). There is growing evidence that social rejection may influence individuals’ emotional and cognitive responses by eliciting ruminative thought processes, which may contribute to the development and maintenance of mood disorders (Goodman and Southam-Gerow, 2010; Wesselmann et al., 2013). In Williams (2009) temporal need-threat model, rumination is one of the events experienced after the occurrence of rejection. Additionally, researchers have proposed a psychobiological model of social rejection and depression, in which rejection-related events activate brain regions involved in processing negative emotions and pain, triggering negative self-referential thoughts and feelings of self-consciousness (Slavich et al., 2010), which are key features of rumination. Therefore, exploring the role of social rejection on rumination not only contributes to a deeper understanding of the effects of rejection on individuals but also provides new ideas for coping with its negative effects.
Recent advances in neuroimaging techniques have enabled researchers to investigate how brain functional connectivity is related to specific cognitive and affective processes. The connectome-based predictive model (CPM) is one such technique that has shown promise for predicting individual differences in cognitive and emotional traits (Shen et al., 2017). In this study, we used the CPM approach to identify a resting-state functional connectivity network that predicted rumination; we believed that this network would involve brain areas related to self-referential thoughts, emotions, and attention. Furthermore, we use mediation analyses to examine the role of this network in the relationship between social rejection and rumination. Our findings would provide novel insights into the neural mechanisms underlying the link between social rejection and rumination, and might have important implications for the development of interventions to mitigate the negative consequences of social rejection.
In summary, the present study aimed to demonstrate the followings: (1) social rejection is positively correlated with rumination, (2) brain functional connectivity features of rumination, and (3) social rejection-induced enhancement of ruminative traits (leading individuals to have a greater risk of anxiety and depression) through brain functional connectivity features.
2. Methods
2.1. Participants
The present study was conducted in a population of healthy adults, and none of them had a history of neurological or psychiatric illness (self-reported, with no history of brain damage, schizophrenia, major depression, anxiety disorder, and insomnia). All subjects in this study were from Southwest University, Chongqing, China. Psychological questionnaire and brain data were obtained from our ongoing project, Gene-Brain-Behavior (GBB). The GBB project aims to establish a multimodal database focusing on cognition and brain development to explore the relationships of structural and functional brain development with creativity, emotion, personality, etc.; these data have been used in several previous studies (Chen et al., 2019; Zhuang et al., 2021). The research protocol was approved by the Ethics Committee of the Brain Imaging Center of Southwest University. All participants signed an informed consent form prior to the data acquisition and were paid 50 RMB for their participation. Of the subjects recruited for this study, 593 had complete data available for psychological measures that were able to be matched with brain data. Thirteen subjects were excluded due to excessive head movement (mean head movements >0.3 mm), and 20 subjects were excluded due to substandard brain imaging data (artefacts, etc.). Thus, data from 560 subjects (mean age: 19.32 ± 1.27 years; range: 16–26 years; 401 females and 159 males) were included in the analyses. Basic details of the participants, along with additional information, are available in the Supplementary material.
2.2. Psychological questionnaire
Rumination was measured with the Ruminative Responses Scale (RRS) (Nolen-Hoeksema and Morrow, 1991). The RRS contains 22 items rated a scale from 1 (almost never) to 4 (almost always) regarding the frequency of ruminative thoughts (e.g., Think “Why do I have problems other people do not have?”). The RRS comprises three subscales: reflective pondering, brooding and depression-related. We removed the third subscale, depression-related responses, as in previous studies (Li et al., 2022), because we focused on the behavioral of ruminative thoughts rather than its emotional consequences and because of the spurious discriminatory validity given its overlap with symptoms of depression. Thus, the final rumination score encompassed two subscales (reflective pondering and brooding) and had a Cronbach’s alpha coefficient of 0.92 (Lackner and Fresco, 2016).
Social rejection was assessed using the Perceived Rejection survey from the NIH Toolbox on Emotion (Salsman et al., 2013). On this survey, participants rate the 8 items on a scale from 1 (never) to 5 (always) regarding their feelings of rejection (e.g., “Does not listen when I ask for help”). The Cronbach’s alpha coefficient for the Perceived Rejection survey was 0.93 (Salsman et al., 2013).
2.3. Image data acquisition and preprocessing
The resting-state scan lasted 8 min, during which participants were told to keep their bodies as still as possible, not to think about specific things, and to keep their eyes open as much as possible. During the scan, a lab assistant was present outside the scanning room to monitor the participant’s state and respond to his or her needs.
Functional and structural data were obtained using a Siemens 3 T Trio scanner (Siemens Medical System, Erlangen, Germany) at the Brain Imaging Center of Southwest University. Resting-state fMRI data were obtained using a gradient-echo echo-planar imaging (GRE-EPI) sequence with the following parameters: repetition time (TR) = 2,000 ms, echo time (TE) = 30 ms, flip angle (FA) = 90°, field of view (FOV) = 220 × 220 mm2, slices = 32, thickness = 3 mm, interslice gap = 1 mm, and voxel size = 3.4 × 3.4 × 4 mm3. A high-resolution, three-dimensional magnetization-prepared rapid acquisition gradient echo (MPRAGE) sequence with the following parameters was conducted: TR = 1,900 ms, TE = 2.52 ms, FA = 9°, slices = 176, FOV = 256 × 256 mm2, thickness = 1 mm, and voxel size = 1 × 1 × 1 mm3. Images were preprocessed using the CONN toolbox (Whitfield-Gabrieli and Nieto-Castanon, 2012). Slice timing correction was applied. During head motion estimation and correction, we also regressed out outlier scans due to head motion. Potential outlier scans were identified based on two criteria: the observed global BOLD signal and the amount of subject motion during scanning. Acquisitions with framewise displacement above 0.9 mm or global BOLD signal changes above 5 s.d. were flagged as potential outliers. The images were segmented into grey matter, white matter (WM), and cerebrospinal fluid (CSF) and normalized to standard MNI space (Ashburner and Friston, 2005). An 8-mm full-width at half-maximum Gaussian kernel was applied to smooth the images. The images were then denoised using the anatomical component-based correction (aCompCor) method, and we regressed out signals from WM, CSF, and 12 head movement parameters. After denoising, the images were linearly detrended and bandpass filtered (0.01–0.1 Hz). Global signal regression was not used in this study, as hemispheric segregation and hemispheric integration are based on the functional connectivity (FC) between these regions and corresponding homotopic regions.
2.4. Functional network construction
FC matrices were constructed using the GRETNA toolbox (Wang et al., 2015a). The atlas used to calculate FC in this study partitioned the cortex into 400 ROIs and grouped them into 7 major brain networks with specific functions: the visual network (VIS), somatomotor network (SMN), dorsal attention network (DAN), salience network (SAL), limbic network (LIM), frontoparietal control network (FPCN) and default mode network (DMN)(Schaefer et al., 2018). In constructing the functional connectivity matrix, the time series data of the node were extracted from each ROI, and the “edges” were represented by the Pearson correlation coefficients of the time series of the nodes, which underwent a Fisher z transformation (Zar, 1999). The significance of the negative values of the resting-state functional connectivity matrix is not clear and is susceptible to preprocessing methods and noise, which may be artificially created false correlations that do not necessarily indicate negative coupling between regions (Murphy et al., 2009; Chai et al., 2012).Therefore, negative values in the matrix were set to zero as previous studies (Chan et al., 2014, 2021; Wang et al., 2021a, 2022). Each subject’s data were constructed as a 400 × 400 matrix for further analysis.
2.5. Connectome-based predictive modeling
Here, we used a leave-one-out approach of connectome-based predictive modeling (CPM) (Shen et al., 2017) to predict rumination from rs-fMRI connectivity, which has been widely used in recent years to predict anxiety (Wang et al., 2021b), irritability (Scheinost et al., 2021), loneliness (Feng et al., 2019), etc. CPM consists of three steps: feature selection, model construction, and model validation (Ren et al., 2021). First, we calculated the correlation coefficients between resting-state functional connectivity and rumination scores and selected the significant edges (p < 0.01)(Kucyi et al., 2021; Scheinost et al., 2021), including both positive and negative edges. The retained edges were used to form the positive and negative prediction networks. For leave-one-out cross-validation, one participant was excluded from the training set in each iteration, and the model was trained with data from N-1 participants (i.e., 559 in this study). Then, data from the excluded participant were used as a validation set to obtain the predicted value, and this process was repeated until each participant had obtained one. Thus, a positive network and a negative network were obtained in each iteration, and the final prediction network consisted of edges that appeared in each iteration (Figure 1A). Next, the values of all these edges in each network were summed to obtain the strength of the prediction network. The general linear model (GLM) was trained with these data to reflect the relationship between prediction network strength and rumination. The predictive efficacy was reflected by the Pearson correlation between the predicted and observed scores. If a relatively strong correlation was observed, the prediction model was successful. We further controlled for covariates such as age, sex, and mean head movement to determine whether these factors had a confounding effect (see at Supplementary material). Finally, we performed an iterative test (1,000 iterations) to obtain the distribution of the observed correlation coefficients (Figure 1B). The number of times that the distribution was greater than the true value was divided by the number of permutations to calculate the p value.
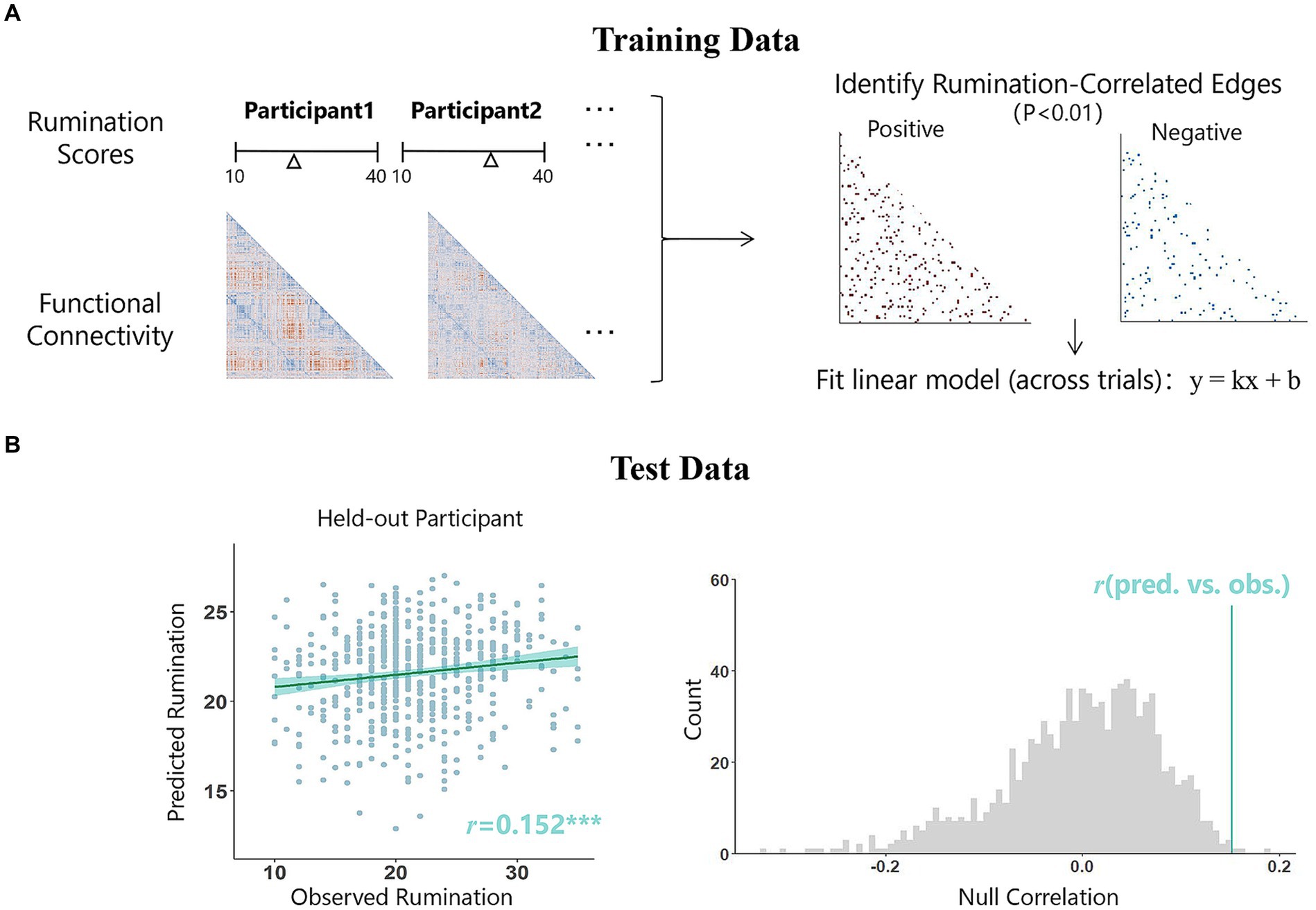
Figure 1. Connectivity-based predictive model of rumination. (A) For the leave-one-out method, in each iteration, data from N-1 participants are used as the training data, while the data from the remaining individual serve as the testing data. Within the training data, we calculated the correlation coefficients between resting-state functional connectivity and rumination scores and selected the significant edges (p < 0.01), the values of all these edges in each network were summed to obtain the strength of the prediction network. Next, the general linear model was trained to reflect the relationship between prediction network strength and rumination scores. This model will then be used in the test set to obtain a predicted score. This process will be repeated N times until each participant has received a prediction score. Edges retained each iteration form the final prediction network (red: positive correlation; blue, negative correlation). (B) The predictive efficacy of the model was judged by calculating the Pearson’s correlation coefficient between the predicted scores and the observed scores. This correlation value (indicated by the blue line) was then compared with the null distribution of r values from 1,000 random permutations. The scatterplot on the left side of the figure demonstrates the relationship between the predicted scores obtained using the negative prediction network and the actual scores in this study, while the right side presents the results of the permutation test, pperm = 0.004.
2.6. Model validation
To enhance the rigor of our study and attain more robust outcomes, we employed the ten-fold cross-validation method for model validation. In contrast to leave-one-out method, the adoption of ten-fold cross-validation involves a more stringent evaluation, mitigating the randomness introduced by specific data partitioning and better controlling the risk of overfitting. In this method, we randomly divided all 560 participants into 10 groups. The model was trained using data from 9 of these groups and tested with data from the remaining group; this process was repeated for all groups. Here, we used the same significance threshold as the leave-one-out approach above (p < 0.01). Since random grouping may introduce bias regarding model prediction efficacy, we repeated this process 100 times to reduce the effect, after which we obtained the averaged r and p values. Then, we performed 1,000 random permutations to generate the null distribution for significance testing.
2.7. Mediation analysis
We conducted a mediation analysis to explore whether rumination network strength mediated the association between social rejection and rumination scores. Analyses were conducted using Model 4 of the indirect macro PROCESS designed for SPSS (Preacher and Hayes, 2008). In the present study, the independent variable (X) was the social rejection score, the dependent variable (Y) was the rumination score, and the mediation variable (M) was the strength of the prediction network. Indirect effects were considered significant if zero was not included in the bootstrapped 95% confidence intervals (CIs) (5,000 samples).
3. Results
3.1. Psychological questionnaire results
We verified the completeness, logical consistency, and reasonable timing of the collected questionnaires, assessed the rationality of data distribution, and examined the presence of outliers and non-genuine responses. At this stage, no participants were excluded. We used Pearson’s correlation coefficients to explore the correlation between social rejection and rumination, and the results showed that there was a significant positive correlation (r = 0.283, p < 0.001).
3.2. CPM Results
3.2.1. Predictive efficacy of the leave-one-out approach
The network strength of a positive or negative prediction network is obtained by summing the values of all edges within the network. Predictive efficacy was represented by the correlation between the observed rumination scores and predicted rumination scores. After leave-one-out cross-validation, there were 182 edges in the positive network and 299 edges in the negative network (Figure 2A). The results showed that the negative network significantly predicted individual differences in rumination scores (r = 0.152, p < 0.001, pperm = 0.004), while the positive network did not significantly predict these differences (r = 0.045, p = 0.288). The pperm values was based on permutation testing (1,000 permutations). Controlling for head movement, sex, and age did not affect the validation of the results.
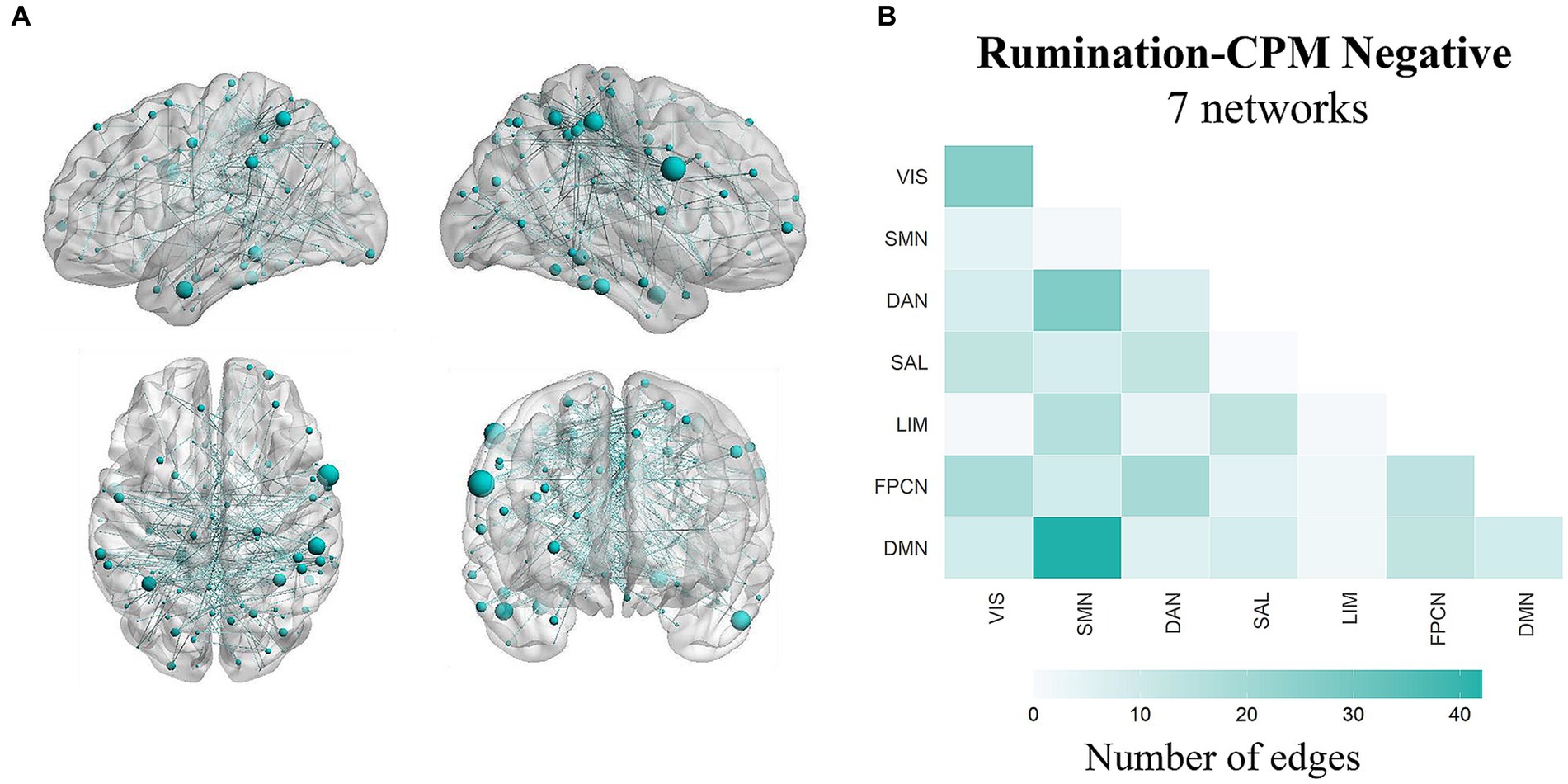
Figure 2. Functional neuroanatomical basis of the predictive network. (A) Edges from the negative prediction network. Only edges that appeared in each iteration were retained. (B) The number of edges in the negative prediction network assigned to each intra- or internetwork pair based on the Schaefer400 and Yeo-Krienen 7-network atlases. VIS, visual network; SMN, somatomotor network; DAN, dorsal attention network; SAL, salience network; LIM, limbic network; FPCN, frontoparietal control network; DMN, default mode network.
3.2.2. Functional neuroanatomical basis of the predictive network
To better understand the functional neuroanatomical implications of the prediction network, we assigned the 299-edge negative network into intranetwork and internetwork connections according to the Schaefer400 atlas (Schaefer et al., 2018). The Schaefer400 atlas can classify nodes into 7 networks or 17 networks; here, we used the 7-network classification, consistent with the Yeo-Krienen networks (Yeo et al., 2011). Similar to the findings of previous studies (Chen et al., 2020), the DMN was closely associated with rumination and contributed strongly to the prediction of rumination among the networks. The top five network pairs contributing to negative edges were as follows: DMN-SMN, DAN-SMN, VIS–VIS, FPCN-DAN, and FPCN-VIS (Figure 2B).
To further understand the importance of each node within the prediction network, we obtained the node strength by calculating the sum of the edge weights connected to the node (He et al., 2021). Specifically, weights were correlation coefficients between the functional connectivity and rumination scores. Then, we summed the absolute values of these correlation coefficients (since all correlation coefficients were negative) to obtain the strength of these nodes. The results showed that regions with higher node strength were mainly located in the supramarginal gyrus, angular gyrus, middle temporal gyrus, 3 inferior temporal gyrus, fusiform gyrus and parahippocampal gyrus (Figure 3).
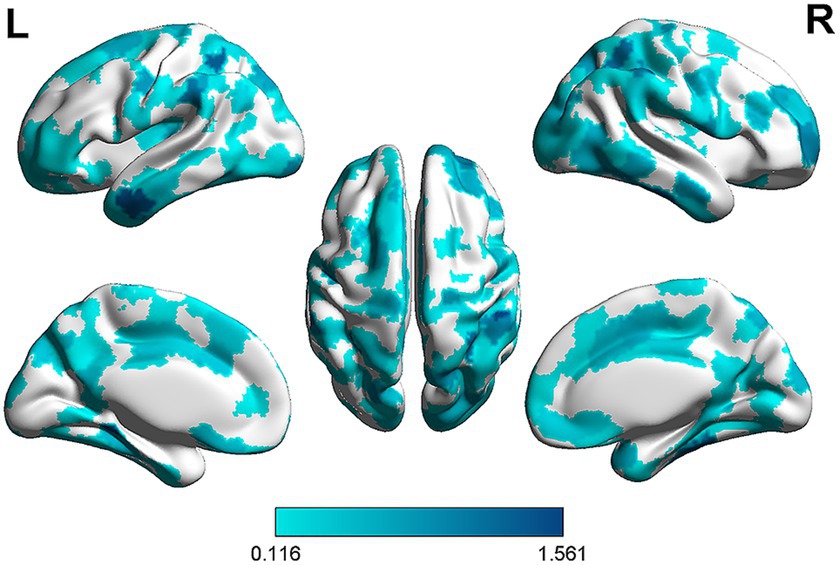
Figure 3. The strength of nodes in the prediction network. Edge weights were obtained by calculating the correlation coefficients between rumination scores and functional connectivity in the prediction network. Node strength was computed by summing the absolute value of the correlation coefficients. The darker the color, the greater the weight of the ROI in the predictive model, indicating a stronger association with rumination.
3.2.3. Model validation
Tenfold cross-validation yielded similar results as the leave-one-out method. The negative network significantly predicted rumination scores (r = 0.125, p = 0.005, pprem = 0.016), while the positive network did not.
3.3. Mediation analysis
We defined the sum of the FC values as the strength of the prediction network. In the mediation analysis, the independent, mediating, and dependent variables were social rejection, prediction network strength, and rumination. The relationship between social rejection and rumination (c’ = 0.206, p < 0.001) was mediated by prediction network strength (a = −0.520, p < 0.001; b = −0.508, p < 0.001). The 95% confidence interval of the mediation analysis did not include 0 (LLCI = 0.038, ULCI = 0.121), indicating that this indirect effect was significant (Figure 4).
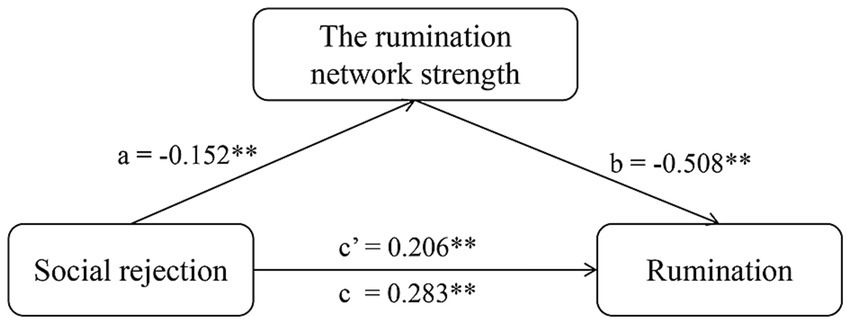
Figure 4. The mediation model of the relationships among social rejection, rumination network strength, and rumination. Mediation analysis is a method used to explain the mechanism through which an independent variable influences a dependent variable via an intermediate variable. In the present study, the independent variable (X) was the social rejection scores, the dependent variable (Y) was the rumination scores, and the mediation variable (M) was the strength of the prediction network. Path a represents the influence of X on M. Path b represents the influence of the M on the Y. Path c, the total effect, includes both the direct and indirect effects, representing the overall impact of X on Y. Path c’ represents the direct impact of X on Y when not considering the mediator variable M. *p < 0.05; **p < 0.01.
4. Discussion
Rumination has received widespread attention as an important factor in the maintenance of a variety of psychiatric disorders (Watkins and Roberts, 2020). However, to date, no studies have constructed predictive models of rumination from the functional connectivity characteristics of healthy individuals. In addition, research has consistently focused on the effects of rumination rather than the factors that influence rumination. In the present study, we established a resting-state functional connectivity network capable of predicting rumination scores using CPM and assessed the validity of the prediction model using two different cross-validation methods (leave-one-out and tenfold cross-validation). The model maintained predictive validity after controlling for sex, age and mean head movement. We then calculated the strength of the prediction network by summing the values of all FC edges and verified its mediating role in the relationship between social rejection and rumination. These results suggest that social rejection may lead to more rumination by affecting functional networks in the brain.
The present study assigned functional connectivity values to a functionally explicit large-scale network to further investigate the neural basis of the prediction network; the results indicated that the following networks strongly contributed to the predictive power of the network: the default mode network (DMN), dorsal attention network (DAN) and frontoparietal control network (FPCN). The DMN is one of the networks that has received the most attention regarding the cognitive mechanisms of rumination due to its high relevance to self-awareness (Davey et al., 2016). Studies have demonstrated that the DMN is involved in self-referential processes, theory of mind and autobiographical memory (Buckner and Carroll, 2007; Sheline et al., 2009; Qin and Northoff, 2011). A task-state fMRI study found that the overall FC within the entire DMN tended to decrease during rumination, including FC between the core and dorsal medial prefrontal cortex (dmPFC) subsystems (Chen et al., 2020). The dmPFC subsystem is widely believed to be associated with mentalizing and present-oriented thoughts (Van Overwalle, 2011; Wagner et al., 2012; Andrews-Hanna et al., 2014). Therefore, our results may indicate that during rumination, people tend to devote more attention to past events and thus less attention to their present state. Notably, rumination is a multifaceted process and its link to DMN cannot be simply defined. Since individuals may engage differently with their past experiences and present states during rumination, it is essential to recognize that changes in FC patterns can be context-dependent and influenced by various factors (Zhou et al., 2020; Tsuchiyagaito et al., 2021). Future research may be able to explore the relationship between the different cognitive processes involved in rumination state and DMN connectivity by adopting more fine-grained approaches.
Extensive experiments have shown that the DAN supports the maintenance of concentration, allowing people to focus on the same thing for longer periods of time and ignore external influences (Corbetta and Shulman, 2002; Corbetta et al., 2008), while the FPCN supports executive control of attention and emotions, playing an important role in inhibitory control and cognitive flexibility (Bressler and Menon, 2010; Zhang et al., 2017; Kupis et al., 2021). Dixon et al. (2018) found that one subsystem in the FPCN regulates mental activities such as introspection through connections with the DMN, while the other subsystem is connected to the DAN and regulates processes such as top-down attentional selection. Therefore, decreased FC within and between these networks predicts less attention to the external environment and stimuli, during which time individuals’ cognitive control may be compromised, leading to a greater likelihood of dwelling on recurring memories and suffering negative emotions.
In addition, the SMN also contributed strongly to the prediction model. A number of previous studies have identified that the SMN plays a role in the perception of external stimuli, body awareness and emotional and cognitive processing (Pineda, 2008; Banissy et al., 2010; Wood et al., 2016; Davis et al., 2017). In addition, decreased FC between the SMN and the DAN might underlie attention deficits (Wang et al., 2015b; Zhu et al., 2016). Studies on bipolar disorder have suggested that changes in connectivity between the SMN and DMN may be related to impaired emotion processing and executive function (Martino et al., 2016; Kazemi et al., 2018; Zhu et al., 2022). This may indicate that impaired cognitive control during rumination makes it difficult for individuals to shift their attention away from negative stimuli and that this continuous processing of self-relevant negative events leads to persistence of ruminative thoughts.
Analysis of node strengths revealed that key nodes predicting rumination scores were located in the supramarginal gyrus (SMG), angular gyrus (AG), middle temporal gyrus (MTG), inferior temporal gyrus (ITG), fusiform gyrus (FFG) and parahippocampal gyrus (PHG), areas which are closely related to memory, attention and emotion. The SMG supports attention orientation, emotion regulation, language and verbal working memory (Rushworth et al., 2001; Silk et al., 2010; Deschamps et al., 2014), and researchers have found that the SMG is also capable of integrating perceptual information from multiple modalities and is associated with self-related emotions (Silani et al., 2013). The AG supports memory retrieval, attention and spatial cognition, semantic memory, reasoning, and other higher-order cognitive functions (Seghier, 2013; Humphreys et al., 2021). The MTG is activated by processes regarding memory and emotion (Chan et al., 2009; Fan et al., 2017), and some studies have suggested that it may be related to poor emotion regulation and neuroticism (DeYoung et al., 2010; Zhang et al., 2022). In addition, the ITG and the FFG are brain regions closely associated with the processing of perceptual information (Rossion et al., 2003; Domínguez-Borràs et al., 2009), such as visual and auditory information, and FFG has also been found to play a crucial role in face processing (Rossion et al., 2000).While the PHG is related to emotion regulation and autobiographical memory processes (Almeida et al., 2009; Frank et al., 2014).
The results from the questionnaire analysis showed that social rejection and rumination were positively correlated. Furthermore, we found that the prediction network played a mediating role in the relationship between social rejection and rumination. Rejection damages interpersonal relationships and threatens basic needs such as sense of belonging and security (Kemeny, 2009). Once individuals experience rejection, they suffer pain, negative emotions, and lack of satisfaction of their basic needs, leading them to focus on the experience of rejection and continually recall, consider and assess the meaning and importance of the rejection event (Williams, 2009). After suffering social rejection, people were more inclined to respond by withdrawing from social contact, hoping to protect themselves from further social loss. This withdrawal was accompanied by rumination, which may represent heightened motivation to prevent similar circumstances (Molden et al., 2009). However, studies have shown that rumination may also occur after interpersonal difficulties lead to reduced satisfaction with our relationships, leading individuals to become less confident, rejection sensitive, exhibit maladaptive responses and reduced interpersonal problem solving, ultimately preventing them from recovering from rejection (Lam et al., 2003; Kuehner and Buerger, 2005; Pearson et al., 2010; Wesselmann et al., 2013). Numerous studies have shown that the DMN is broadly related to social cognition and is coupled with various other networks, such as the attention network, during different social cognition tasks (Schurz et al., 2014; Xie et al., 2016; Schurz et al., 2020). In the present study, the SMG and the AG contributed the most to the prediction network. A study showed that the SMG is associated with emotional egocentric bias (Silani et al., 2013), and we hypothesized that social rejection may trigger self-referential rumination by triggering emotional experiences strongly related to the self. The AG is a region in the core system in the default mode network associated with functions from mentalization to semantic processing and memory retrieval; this region is responsible for detecting behaviourally relevant stimuli and environmental changes (Seghier, 2013; Spreng and Andrews-Hanna, 2015). The mediation analysis results may explain the tendency of people to immerse themselves in introspection after rejection, decreasing their cognitive load during other cognitive tasks and overlooking changes in the environment. In conclusion, we hypothesize that social rejection may predispose people to rumination by inducing more assessment of self- and social-related events as an emotion-regulation strategy for coping with the negative experience of rejection.
There are still some limitations of our study. First, we explored the influence of social relationships on rumination; therefore, we chose social rejection, which is one of the most important characteristics in social relationships; however, such data are inevitably subjective in nature. Subsequent experiments could attempt to use other approaches, such as the construction of social networks, to utilize objective data. Second, this study examined the relationship between social rejection and rumination from a cross-sectional perspective, without further exploring the wider consequences of rumination on social cognition and relationships. Follow-up studies should further explore the possible long-term relationship between rumination and rejection with longitudinal designs as well as the impact of this vicious circle on depression and anxiety. Besides, at the stage of constructing the functional connectivity matrix, we excluded negative values as their meaning was unclear, which could have resulted in the loss of valuable information. Finally, the results of this study inspired us to consider new approaches to intervening rumination from a social perspective. Future research could explore the effectiveness and feasibility of interventions, such as providing mental health education and social support, offering training in emotion regulation skills and social skills, and implementing socio-emotional interventions.
5. Conclusion
This study was the first to develop a network capable of predicting rumination scores that was trained with resting-state functional connectivity data from a healthy population. We found that the functional connectivity among the DMN, DAN, FPCN and SMN contributed strongly to the predictive model. We explored the relationship between social rejection and rumination, demonstrating that the experience of rejection may lead to rumination. These findings enhance understanding of the impact of social rejection and can inform the development of interventions for rumination from a social cognitive perspective.
Data availability statement
The raw data supporting the conclusions of this article will be made available by the corresponding author. Requests to access the datasets should be directed to author JQ at cWl1ajMxOEBzd3UuZWR1LmNu.
Ethics statement
The studies involving humans were approved by the Brain Imaging Center Institutional Review Board at the Southwest University, China. The studies were conducted in accordance with the local legislation and institutional requirements. The participants provided their written informed consent to participate in this study.
Author contributions
LG: Conceptualization, Methodology, Validation, Visualization, Writing – original draft, Writing – review & editing. QF: Conceptualization, Methodology, Validation, Visualization, Writing – review & editing. XW: Methodology, Software, Validation, Visualization, Writing – review & editing. YG: Methodology, Software, Writing – review & editing. LH: Conceptualization, Software, Writing – review & editing. JQ: Conceptualization, Data curation, Funding acquisition, Supervision, Writing – review & editing.
Funding
The author(s) declare financial support was received for the research, authorship, and/or publication of this article. This research was supported by the Major Research Plan of National Social Science Foundation of China (21&ZD312).
Conflict of interest
The authors declare that the research was conducted in the absence of any commercial or financial relationships that could be construed as a potential conflict of interest.
Publisher’s note
All claims expressed in this article are solely those of the authors and do not necessarily represent those of their affiliated organizations, or those of the publisher, the editors and the reviewers. Any product that may be evaluated in this article, or claim that may be made by its manufacturer, is not guaranteed or endorsed by the publisher.
Supplementary material
The Supplementary material for this article can be found online at: https://www.frontiersin.org/articles/10.3389/fpsyg.2023.1264221/full#supplementary-material
References
Almeida, J. R., Mechelli, A., Hassel, S., Versace, A., Kupfer, D. J., and Phillips, M. L. (2009). Abnormally increased effective connectivity between parahippocampal gyrus and ventromedial prefrontal regions during emotion labeling in bipolar disorder. Psychiatry Res. Neuroimaging 174, 195–201. doi: 10.1016/j.pscychresns.2009.04.015
Andrews-Hanna, J. R., Smallwood, J., and Spreng, R. N. (2014). The default network and self-generated thought: component processes, dynamic control, and clinical relevance. Ann. N. Y. Acad. Sci. 1316, 29–52. doi: 10.1111/nyas.12360
Ashburner, J., and Friston, K. J. (2005). Unified segmentation. NeuroImage 26, 839–851. doi: 10.1016/j.neuroimage.2005.02.018
Banissy, M. J., Sauter, D. A., Ward, J., Warren, J. E., Walsh, V., and Scott, S. K. (2010). Suppressing sensorimotor activity modulates the discrimination of auditory emotions but not speaker identity. J. Neurosci. 30, 13552–13557. doi: 10.1523/JNEUROSCI.0786-10.2010
Belzung, C., Willner, P., and Philippot, P. (2015). Depression: from psychopathology to pathophysiology. Curr. Opin. Neurobiol. 30, 24–30. doi: 10.1016/j.conb.2014.08.013
Berman, M. G., Peltier, S., Nee, D. E., Kross, E., Deldin, P. J., and Jonides, J. (2011). Depression, rumination and the default network. Soc. Cogn. Affect. Neurosci. 6, 548–555. doi: 10.1093/scan/nsq080
Bressler, S. L., and Menon, V. (2010). Large-scale brain networks in cognition: emerging methods and principles. Trends Cogn. Sci. 14, 277–290. doi: 10.1016/j.tics.2010.04.004
Buckner, R. L., Andrews-Hanna, J. R., and Schacter, D. L. (2008). The brain's default network: anatomy, function, and relevance to disease. Ann. N. Y. Acad. Sci. 1124, 1–38. doi: 10.1196/annals.1440.011
Buckner, R. L., and Carroll, D. C. (2007). Self-projection and the brain. Trends Cogn. Sci. 11, 49–57. doi: 10.1016/j.tics.2006.11.004
Cacioppo, J. T., and Hawkley, L. C. (2009). Perceived social isolation and cognition. Trends Cogn. Sci. 13, 447–454. doi: 10.1016/j.tics.2009.06.005
Chai, X. J., Castañón, A. N., Öngür, D., and Whitfield-Gabrieli, S. (2012). Anticorrelations in resting state networks without global signal regression. NeuroImage 59, 1420–1428. doi: 10.1016/j.neuroimage.2011.08.048
Chan, M. Y., Han, L., Carreno, C. A., Zhang, Z., and Rodriguez, R. M. (2021). Long-term prognosis and educational determinants of brain network decline in older adult individuals. Nature Aging 1, 1053–1067. doi: 10.1038/s43587-021-00125-4
Chan, S. W., Norbury, R., Goodwin, G. M., and Harmer, C. J. (2009). Risk for depression and neural responses to fearful facial expressions of emotion. Br. J. Psychiatry 194, 139–145. doi: 10.1192/bjp.bp.107.047993
Chan, M. Y., Park, D. C., Savalia, N. K., Petersen, S. E., and Wig, G. S. (2014). Decreased segregation of brain systems across the healthy adult lifespan. Proc. Natl. Acad. Sci. 111, E4997–E5006. doi: 10.1073/pnas.1415122111
Chen, Q., Beaty, R. E., Cui, Z., Sun, J., and He, H. (2019). Brain hemispheric involvement in visuospatial and verbal divergent thinking. NeuroImage 202:116065. doi: 10.1016/j.neuroimage.2019.116065
Chen, X., Chen, N.-X., Shen, Y.-Q., Li, H.-X., and Li, L. (2020). The subsystem mechanism of default mode network underlying rumination: a reproducible neuroimaging study. NeuroImage 221:117185. doi: 10.1016/j.neuroimage.2020.117185
Corbetta, M., Patel, G., and Shulman, G. L. (2008). The reorienting system of the human brain: from environment to theory of mind. Neuron 58, 306–324. doi: 10.1016/j.neuron.2008.04.017
Corbetta, M., and Shulman, G. L. (2002). Control of goal-directed and stimulus-driven attention in the brain. Nat. Rev. Neurosci. 3, 201–215. doi: 10.1038/nrn755
Cox, S. J., Mezulis, A. H., and Hyde, J. S. (2010). The influence of child gender role and maternal feedback to child stress on the emergence of the gender difference in depressive rumination in adolescence. Dev. Psychol. 46, 842–852. doi: 10.1037/a0019813
Davey, C. G., Pujol, J., and Harrison, B. J. (2016). Mapping the self in the brain's default mode network. NeuroImage 132, 390–397. doi: 10.1016/j.neuroimage.2016.02.022
Davis, R. N., and Nolen-Hoeksema, S. (2000). Cognitive inflexibility among ruminators and nonruminators. Cogn. Ther. Res. 24, 699–711. doi: 10.1023/A:1005591412406
Davis, J. D., Winkielman, P., and Coulson, S. (2017). Sensorimotor simulation and emotion processing: impairing facial action increases semantic retrieval demands. Cog. Affect. Behav. Neurosci. 17, 652–664. doi: 10.3758/s13415-017-0503-2
Deschamps, I., Baum, S. R., and Gracco, V. L. (2014). On the role of the supramarginal gyrus in phonological processing and verbal working memory: evidence from rTMS studies. Neuropsychologia 53, 39–46. doi: 10.1016/j.neuropsychologia.2013.10.015
DeYoung, C. G., Hirsh, J. B., Shane, M. S., Papademetris, X., Rajeevan, N., and Gray, J. R. (2010). Testing predictions from personality neuroscience: brain structure and the big five. Psychol. Sci. 21, 820–828. doi: 10.1177/0956797610370159
Dixon, M. L., De La Vega, A., Mills, C., Andrews-Hanna, J., Spreng, R. N., Cole, M. W., et al. (2018). Heterogeneity within the frontoparietal control network and its relationship to the default and dorsal attention networks. Proc. Natl. Acad. Sci. 115, E1598–E1607. doi: 10.1073/pnas.1715766115
Domínguez-Borràs, J., Trautmann, S.-A., Erhard, P., Fehr, T., Herrmann, M., and Escera, C. (2009). Emotional context enhances auditory novelty processing in superior temporal gyrus. Cereb. Cortex 19, 1521–1529. doi: 10.1093/cercor/bhn188
Fan, J., Zhong, M., Gan, J., Liu, W., Niu, C., Liao, H., et al. (2017). Spontaneous neural activity in the right superior temporal gyrus and left middle temporal gyrus is associated with insight level in obsessive-compulsive disorder. J. Affect. Disord. 207, 203–211. doi: 10.1016/j.jad.2016.08.027
Feng, C., Wang, L., Li, T., and Xu, P. (2019). Connectome-based individualized prediction of loneliness. Soc. Cogn. Affect. Neurosci. 14, 353–365. doi: 10.1093/scan/nsz020
Frank, D., Dewitt, M., Hudgens-Haney, M., Schaeffer, D., Ball, B., Schwarz, N., et al. (2014). Emotion regulation: quantitative meta-analysis of functional activation and deactivation. Neurosci. Biobehav. Rev. 45, 202–211. doi: 10.1016/j.neubiorev.2014.06.010
Goodman, K. L., and Southam-Gerow, M. A. (2010). The regulating role of negative emotions in children’s coping with peer rejection. Child Psychiatry Hum. Dev. 41, 515–534. doi: 10.1007/s10578-010-0185-2
Gunther Moor, B., Crone, E. A., and van der Molen, M. W. (2010). The heartbrake of social rejection: heart rate deceleration in response to unexpected peer rejection. Psychol. Sci. 21, 1326–1333. doi: 10.1177/0956797610379236
Hamilton, J. P., Farmer, M., Fogelman, P., and Gotlib, I. H. (2015). Depressive rumination, the default-mode network, and the dark matter of clinical neuroscience. Biol. Psychiatry 78, 224–230. doi: 10.1016/j.biopsych.2015.02.020
He, L., Wei, D., Yang, F., Zhang, J., Cheng, W., Feng, J., et al. (2021). Functional connectome prediction of anxiety related to the COVID-19 pandemic. Am. J. Psychiatr. 178, 530–540. doi: 10.1176/appi.ajp.2020.20070979
Humphreys, G. F., Ralph, M. A. L., and Simons, J. S. (2021). A unifying account of angular gyrus contributions to episodic and semantic cognition. Trends Neurosci. 44, 452–463. doi: 10.1016/j.tins.2021.01.006
Johnson, D. P., Whisman, M. A., Corley, R. P., Hewitt, J. K., and Friedman, N. P. (2014). Genetic and environmental influences on rumination and its covariation with depression. Cognit. Emot. 28, 1270–1286. doi: 10.1080/02699931.2014.881325
Kaiser, R. H., Andrews-Hanna, J. R., Wager, T. D., and Pizzagalli, D. A. (2015). Large-scale network dysfunction in major depressive disorder: a meta-analysis of resting-state functional connectivity. JAMA Psychiatry 72, 603–611. doi: 10.1001/jamapsychiatry.2015.0071
Kazemi, R., Rostami, R., Khomami, S., Baghdadi, G., Rezaei, M., Hata, M., et al. (2018). Bilateral transcranial magnetic stimulation on DLPFC changes resting state networks and cognitive function in patients with bipolar depression. Front. Hum. Neurosci. 12:356. doi: 10.3389/fnhum.2018.00356
Kemeny, M. E. (2009). Psychobiological responses to social threat: evolution of a psychological model in psychoneuroimmunology. Brain Behav. Immun. 23, 1–9. doi: 10.1016/j.bbi.2008.08.008
Kirkegaard Thomsen, D. (2006). The association between rumination and negative affect: a review. Cognit. Emot. 20, 1216–1235. doi: 10.1080/02699930500473533
Kucyi, A., Esterman, M., Capella, J., Green, A., and Uchida, M. (2021). Prediction of stimulus-independent and task-unrelated thought from functional brain networks. Nat. Commun. 12:1793. doi: 10.1038/s41467-021-22027-0
Kuehner, C., and Buerger, C. (2005). Determinants of subjective quality of life in depressed patients: the role of self-esteem, response styles, and social support. J. Affect. Disord. 86, 205–213. doi: 10.1016/j.jad.2005.01.014
Kupis, L., Goodman, Z. T., Kornfeld, S., Hoang, S., and Romero, C. (2021). Brain dynamics underlying cognitive flexibility across the lifespan. Cereb. Cortex 31, 5263–5274. doi: 10.1093/cercor/bhab156
Lackner, R. J., and Fresco, D. M. (2016). Interaction effect of brooding rumination and interoceptive awareness on depression and anxiety symptoms. Behav. Res. Ther. 85, 43–52. doi: 10.1016/j.brat.2016.08.007
Lam, D., Schuck, N., Smith, N., Farmer, A., and Checkley, S. (2003). Response style, interpersonal difficulties and social functioning in major depressive disorder. J. Affect. Disord. 75, 279–283. doi: 10.1016/S0165-0327(02)00058-7
Li, X., Qin, F., Liu, J., Luo, Q., and Zhang, Y. (2022). An insula-based network mediates the relation between rumination and interoceptive sensibility in the healthy population. J. Affect. Disord. 299, 6–11. doi: 10.1016/j.jad.2021.11.047
Lois, G., and Wessa, M. (2016). Differential association of default mode network connectivity and rumination in healthy individuals and remitted MDD patients. Soc. Cogn. Affect. Neurosci. 11, 1792–1801. doi: 10.1093/scan/nsw085
Lyubomirsky, S., and Nolen-Hoeksema, S. (1995). Effects of self-focused rumination on negative thinking and interpersonal problem solving. J. Pers. Soc. Psychol. 69, 176–190. doi: 10.1037/0022-3514.69.1.176
Mandell, D., Siegle, G. J., Shutt, L., Feldmiller, J., and Thase, M. E. (2014). Neural substrates of trait ruminations in depression. J. Abnorm. Psychol. 123, 35–48. doi: 10.1037/a0035834
Martino, M., Magioncalda, P., Huang, Z., Conio, B., Piaggio, N., and Duncan, N. (2016). Contrasting variability patterns in the default mode and sensorimotor networks balance in bipolar depression and mania. Proc. Natl. Acad. Sci. 113, 4824–4829. doi: 10.1073/pnas.1517558113
Molden, D. C., Lucas, G. M., Gardner, W. L., Dean, K., and Knowles, M. L. (2009). Motivations for prevention or promotion following social exclusion: being rejected versus being ignored. J. Pers. Soc. Psychol. 96, 415–431. doi: 10.1037/a0012958
Monroe, S. M., Slavich, G. M., Torres, L. D., and Gotlib, I. H. (2007). Severe life events predict specific patterns of change in cognitive biases in major depression. Psychol. Med. 37, 863–871. doi: 10.1017/S0033291707000281
Murphy, K., Birn, R. M., Handwerker, D. A., Jones, T. B., and Bandettini, P. A. (2009). The impact of global signal regression on resting state correlations: are anti-correlated networks introduced? NeuroImage 44, 893–905. doi: 10.1016/j.neuroimage.2008.09.036
Nolen-Hoeksema, S. (1991). Responses to depression and their effects on the duration of depressive episodes. J. Abnorm. Psychol. 100, 569–582. doi: 10.1037/0021-843X.100.4.569
Nolen-Hoeksema, S., Blair, E., and Sonja, L. (2008). Rethinking rumination. Perspectives of. Psychol. Sci. 3, 400–424. Perspectives on psychological science. doi: 10.1111/j.1745-6924.2008.00088.x
Nolen-Hoeksema, S., and Morrow, J. (1991). A prospective study of depression and posttraumatic stress symptoms after a natural disaster: the 1989 Loma Prieta earthquake. J. Pers. Soc. Psychol. 61, 115–121. doi: 10.1037/0022-3514.61.1.115
Offredi, A., Caselli, G., Manfredi, C., Ruggiero, G. M., Sassaroli, S., Liuzzo, P., et al. (2016). Effects of anger rumination on different scenarios of anger: an experimental investigation. Am. J. Psychol. 129, 381–390. doi: 10.5406/amerjpsyc.129.4.0381
Olatunji, B. O., Naragon-Gainey, K., and Wolitzky-Taylor, K. B. (2013). Specificity of rumination in anxiety and depression: a multimodal meta-analysis. Clin. Psychol. Sci. Pract. 20, 225–257. doi: 10.1037/h0101719
Pearson, K. A., Watkins, E. R., Mullan, E. G., and Moberly, N. J. (2010). Psychosocial correlates of depressive rumination. Behav. Res. Ther. 48, 784–791. doi: 10.1016/j.brat.2010.05.007
Pineda, J. A. (2008). Sensorimotor cortex as a critical component of an'extended'mirror neuron system: does it solve the development, correspondence, and control problems in mirroring? Behav. Brain Funct. 4, 47–16. doi: 10.1186/1744-9081-4-47
Preacher, K. J., and Hayes, A. F. (2008). Asymptotic and resampling strategies for assessing and comparing indirect effects in multiple mediator models. Behav. Res. Methods 40, 879–891. doi: 10.3758/BRM.40.3.879
Qin, P., and Northoff, G. (2011). How is our self related to midline regions and the default-mode network? NeuroImage 57, 1221–1233. doi: 10.1016/j.neuroimage.2011.05.028
Ray, R. D., Ochsner, K. N., Cooper, J. C., Robertson, E. R., Gabrieli, J. D., and Gross, J. J. (2005). Individual differences in trait rumination and the neural systems supporting cognitive reappraisal. Cog. Affect. Behav. Neurosci. 5, 156–168. doi: 10.3758/CABN.5.2.156
Ren, Z., Daker, R. J., Shi, L., Sun, J., and Beaty, R. E. (2021). Connectome-based predictive modeling of creativity anxiety. NeuroImage 225:117469. doi: 10.1016/j.neuroimage.2020.117469
Rosenbaum, D., Haipt, A., Fuhr, K., Haeussinger, F. B., Metzger, F. G., Nuerk, H.-C., et al. (2017). Aberrant functional connectivity in depression as an index of state and trait rumination. Sci. Rep. 7:2174. doi: 10.1038/s41598-017-02277-z
Rosenbaum, D., Maier, M. J., Hudak, J., Metzger, F. G., Wells, A., Fallgatter, A. J., et al. (2018a). Neurophysiological correlates of the attention training technique: a component study. NeuroImage 19, 1018–1024. doi: 10.1016/j.nicl.2018.06.021
Rosenbaum, D., Thomas, M., Hilsendegen, P., Metzger, F. G., Haeussinger, F. B., Nuerk, H.-C., et al. (2018b). Stress-related dysfunction of the right inferior frontal cortex in high ruminators: an fNIRS study. NeuroImage 18, 510–517. doi: 10.1016/j.nicl.2018.02.022
Rossion, B., Caldara, R., Seghier, M., Schuller, A. M., Lazeyras, F., and Mayer, E. (2003). A network of occipito-temporal face-sensitive areas besides the right middle fusiform gyrus is necessary for normal face processing. Brain 126, 2381–2395. doi: 10.1093/brain/awg241
Rossion, B., Dricot, L., Devolder, A., Bodart, J.-M., Crommelinck, M., De Gelder, B., et al. (2000). Hemispheric asymmetries for whole-based and part-based face processing in the human fusiform gyrus. J. Cogn. Neurosci. 12, 793–802. doi: 10.1162/089892900562606
Rudert, S. C., Janke, S., and Greifeneder, R. (2021). Ostracism breeds depression: longitudinal associations between ostracism and depression over a three-year-period. J. Affect. Disord. Rep. 4:100118. doi: 10.1016/j.jadr.2021.100118
Rushworth, M. F., Krams, M., and Passingham, R. E. (2001). The attentional role of the left parietal cortex: the distinct lateralization and localization of motor attention in the human brain. J. Cogn. Neurosci. 13, 698–710. doi: 10.1162/089892901750363244
Salsman, J. M., Butt, Z., Pilkonis, P. A., Cyranowski, J. M., Zill, N., and Hendrie, H. (2013). Emotion assessment using the NIH toolbox. Neurology 80, S76–S86. doi: 10.1212/WNL.0b013e3182872e11
Schaefer, A., Kong, R., Gordon, E. M., Laumann, T. O., Zuo, X.-N., and Holmes, A. (2018). Local-global parcellation of the human cerebral cortex from intrinsic functional connectivity MRI. Cereb. Cortex 28, 3095–3114. doi: 10.1093/cercor/bhx179
Scheinost, D., Dadashkarimi, J., Finn, E. S., Wambach, C. G., MacGillivray, C., and Roule, A. (2021). Functional connectivity during frustration: a preliminary study of predictive modeling of irritability in youth. Neuropsychopharmacology 46, 1300–1306. doi: 10.1038/s41386-020-00954-8
Schurz, M., Maliske, L., and Kanske, P. (2020). Cross-network interactions in social cognition: a review of findings on task related brain activation and connectivity. Cortex 130, 142–157. doi: 10.1016/j.cortex.2020.05.006
Schurz, M., Radua, J., Aichhorn, M., Richlan, F., and Perner, J. (2014). Fractionating theory of mind: a meta-analysis of functional brain imaging studies. Neurosci. Biobehav. Rev. 42, 9–34. doi: 10.1016/j.neubiorev.2014.01.009
Seghier, M. L. (2013). The angular gyrus: multiple functions and multiple subdivisions. Neuroscientist 19, 43–61. doi: 10.1177/1073858412440596
Sheline, Y. I., Barch, D. M., Price, J. L., Rundle, M. M., Vaishnavi, S. N., and Snyder, A. (2009). The default mode network and self-referential processes in depression. Proc. Natl. Acad. Sci. 106, 1942–1947. doi: 10.1073/pnas.0812686106
Shen, X., Finn, E. S., Scheinost, D., Rosenberg, M. D., Chun, M. M., Papademetris, X., et al. (2017). Using connectome-based predictive modeling to predict individual behavior from brain connectivity. Nat. Protoc. 12, 506–518. doi: 10.1038/nprot.2016.178
Silani, G., Lamm, C., Ruff, C. C., and Singer, T. (2013). Right supramarginal gyrus is crucial to overcome emotional egocentricity bias in social judgments. J. Neurosci. 33, 15466–15476. doi: 10.1523/JNEUROSCI.1488-13.2013
Silk, T. J., Bellgrove, M. A., Wrafter, P., Mattingley, J. B., and Cunnington, R. (2010). Spatial working memory and spatial attention rely on common neural processes in the intraparietal sulcus. NeuroImage 53, 718–724. doi: 10.1016/j.neuroimage.2010.06.068
Slavich, G. M., O’Donovan, A., Epel, E. S., and Kemeny, M. E. (2010). Black sheep get the blues: a psychobiological model of social rejection and depression. Neurosci. Biobehav. Rev. 35, 39–45. doi: 10.1016/j.neubiorev.2010.01.003
Spasojević, J., and Alloy, L. B. (2001). Rumination as a common mechanism relating depressive risk factors to depression. Emotion 1, 25–37. doi: 10.1037/1528-3542.1.1.25
Spreng, R. N., and Andrews-Hanna, J. R. (2015). The default network and social cognition. Brain Map. 1316, 165–169. doi: 10.1016/B978-0-12-397025-1.00173-1
Thomsen, D. K., Mehlsen, M. Y., Christensen, S., and Zachariae, R. (2003). Rumination—relationship with negative mood and sleep quality. Personal. Individ. Differ. 34, 1293–1301. doi: 10.1016/S0191-8869(02)00120-4
Tsuchiyagaito, A., Misaki, M., Zoubi, O. A., Investigators, T., Paulus, M., and Bodurka, J. (2021). Prevent breaking bad: a proof of concept study of rebalancing the brain's rumination circuit with real-time fMRI functional connectivity neurofeedback. Hum. Brain Mapp. 42, 922–940. doi: 10.1002/hbm.25268
Van Overwalle, F. (2011). A dissociation between social mentalizing and general reasoning. NeuroImage 54, 1589–1599. doi: 10.1016/j.neuroimage.2010.09.043
Voncken, M. J., Alden, L. E., Bögels, S. M., and Roelofs, J. (2008). Social rejection in social anxiety disorder: the role of performance deficits, evoked negative emotions and dissimilarity. Br. J. Clin. Psychol. 47, 439–450. doi: 10.1348/014466508X334745
Wagner, D. D., Haxby, J. V., and Heatherton, T. F. (2012). The representation of self and person knowledge in the medial prefrontal cortex. Wiley Interdiscip. Rev. Cogn. Sci. 3, 451–470. doi: 10.1002/wcs.1183
Wang, Z., Goerlich, K. S., Ai, H., Aleman, A., Luo, Y.-J., and Xu, P. (2021b). Connectome-based predictive modeling of individual anxiety. Cereb. Cortex 31, 3006–3020. doi: 10.1093/cercor/bhaa407
Wang, J., Wang, X., Xia, M., Liao, X., Evans, A., and He, Y. (2015a). GRETNA: a graph theoretical network analysis toolbox for imaging connectomics. Front. Hum. Neurosci. 9:386. doi: 10.3389/fnhum.2015.00386
Wang, P., Zhou, B., Yao, H., Zhan, Y., and Zhang, Z. (2015b). Aberrant intra-and inter-network connectivity architectures in Alzheimer’s disease and mild cognitive impairment. Sci. Rep. 5, 1–12. doi: 10.1038/srep14824
Wang, R., Liu, M., Cheng, X., Wu, Y., Hildebrandt, A., and Zhou, C. (2021a). Segregation, integration, and balance of large-scale resting brain networks configure different cognitive abilities. Proc. Natl. Acad. Sci. 118:e2022288118. doi: 10.1073/pnas.2022288118
Wang, X., Zhuang, K., Li, Z., and Qiu, J. (2022). The functional connectivity basis of creative achievement linked with openness to experience and divergent thinking. Biol. Psychol. 168:108260. doi: 10.1016/j.biopsycho.2021.108260
Watkins, E., and Baracaia, S. (2002). Rumination and social problem-solving in depression. Behav. Res. Ther. 40, 1179–1189. doi: 10.1016/S0005-7967(01)00098-5
Watkins, E., and Brown, R. (2002). Rumination and executive function in depression: an experimental study. J. Neurol. Neurosurg. Psychiatry 72, 400–402. doi: 10.1136/jnnp.72.3.400
Watkins, E., and Moulds, M. (2005). Distinct modes of ruminative self-focus: impact of abstract versus concrete rumination on problem solving in depression. Emotion 5, 319–328. doi: 10.1037/1528-3542.5.3.319
Watkins, E., and Teasdale, J. D. (2001). Rumination and overgeneral memory in depression: effects of self-focus and analytic thinking. J. Abnorm. Psychol. 110, 353–357. doi: 10.1037/0021-843X.110.2.333
Watkins, E. R., and Roberts, H. (2020). Reflecting on rumination: consequences, causes, mechanisms and treatment of rumination. Behav. Res. Ther. 127:103573. doi: 10.1016/j.brat.2020.103573
Wesselmann, E. D., Ren, D., Swim, E., and Williams, K. D. (2013). Rumination hinders recovery from ostracism. Int. J. Dev. Sci. 7, 33–39. doi: 10.3233/DEV-1312115
Whitfield-Gabrieli, S., and Nieto-Castanon, A. (2012). Conn: a functional connectivity toolbox for correlated and anticorrelated brain networks. Brain Connect. 2, 125–141. doi: 10.1089/brain.2012.0073
Whitmer, A. J., and Gotlib, I. H. (2013). An attentional scope model of rumination. Psychol. Bull. 139, 1036–1061. doi: 10.1037/a0030923
Williams, K. D. (2009). Ostracism: a temporal need-threat model. Adv. Exp. Soc. Psychol. 41, 275–314. doi: 10.1016/S0065-2601(08)00406-1
Wood, A., Rychlowska, M., Korb, S., and Niedenthal, P. (2016). Fashioning the face: sensorimotor simulation contributes to facial expression recognition. Trends Cogn. Sci. 20, 227–240. doi: 10.1016/j.tics.2015.12.010
Xie, X., Bratec, S. M., Schmid, G., Meng, C., Doll, A., Wohlschläger, A., et al. (2016). How do you make me feel better? Social cognitive emotion regulation and the default mode network. NeuroImage 134, 270–280. doi: 10.1016/j.neuroimage.2016.04.015
Yeo, B. T., Krienen, F. M., Sepulcre, J., Sabuncu, M. R., and Lashkari, D. (2011). The organization of the human cerebral cortex estimated by intrinsic functional connectivity. J. Neurophysiol. 106, 1125–1165. doi: 10.1152/jn.00338.2011
Zar, J. H. (1999). Biostatistical analysis. Pearson Education India. 4th edn. New Jersey, USA: Prentice-Hall.
Zhang, R., Geng, X., and Lee, T. M. (2017). Large-scale functional neural network correlates of response inhibition: an fMRI meta-analysis. Brain Struct. Funct. 222, 3973–3990. doi: 10.1007/s00429-017-1443-x
Zhang, R., Kranz, G. S., Zou, W., Deng, Y., Huang, X., Lin, K., et al. (2020). Rumination network dysfunction in major depression: a brain connectome study. Prog. Neuro-Psychopharmacol. Biol. Psychiatry 98:109819. doi: 10.1016/j.pnpbp.2019.109819
Zhang, Y., Zhang, R., and Feng, T. (2022). The functional connectivity between right middle temporal gyrus and right superior frontal gyrus impacted procrastination through neuroticism. Neuroscience 481, 12–20. doi: 10.1016/j.neuroscience.2021.11.036
Zhou, H.-X., Chen, X., Shen, Y.-Q., Li, L., Chen, N.-X., Zhu, Z.-C., et al. (2020). Rumination and the default mode network: meta-analysis of brain imaging studies and implications for depression. NeuroImage 206:116287. doi: 10.1016/j.neuroimage.2019.116287
Zhu, H., Zhou, P., Alcauter, S., Chen, Y., Cao, H., Tian, M., et al. (2016). Changes of intranetwork and internetwork functional connectivity in Alzheimer’s disease and mild cognitive impairment. J. Neural Eng. 13:046008. doi: 10.1088/1741-2560/13/4/046008
Zhu, W., Tang, W., Liang, Y., Jiang, X., Li, Y., Chen, Z., et al. (2022). Aberrant functional connectivity of sensorimotor network and its relationship with executive dysfunction in bipolar disorder type I. Front. Neurosci. 15:1859. doi: 10.3389/fnins.2021.823550
Keywords: rumination, social rejection, functional connectivity, prediction model, resting-state fMRI
Citation: Geng L, Feng Q, Wang X, Gao Y, Hao L and Qiu J (2023) Connectome-based modeling reveals a resting-state functional network that mediates the relationship between social rejection and rumination. Front. Psychol. 14:1264221. doi: 10.3389/fpsyg.2023.1264221
Edited by:
Martin Reuter, University of Bonn, GermanyReviewed by:
Taiyong Bi, Zunyi Medical University, ChinaVera Zamoscik, University of Mannheim, Germany
Copyright © 2023 Geng, Feng, Wang, Gao, Hao and Qiu. This is an open-access article distributed under the terms of the Creative Commons Attribution License (CC BY). The use, distribution or reproduction in other forums is permitted, provided the original author(s) and the copyright owner(s) are credited and that the original publication in this journal is cited, in accordance with accepted academic practice. No use, distribution or reproduction is permitted which does not comply with these terms.
*Correspondence: Lei Hao, aGFvbGxsQHN3dS5lZHUuY24=; Jiang Qiu, cWl1ajMxOEBzd3UuZWR1LmNu
†These authors have contributed equally to this work and share first authorship