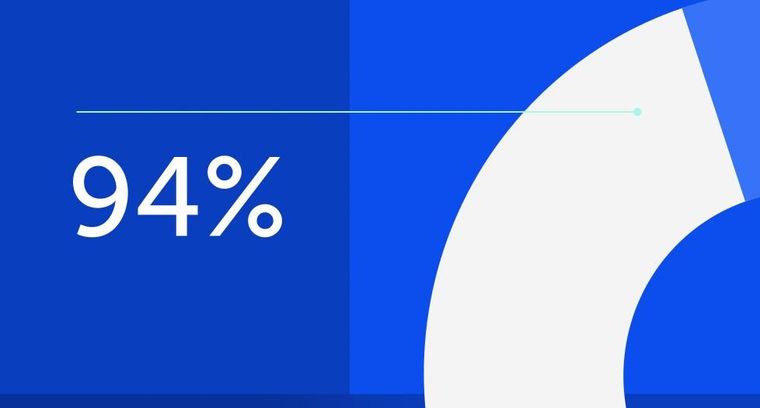
94% of researchers rate our articles as excellent or good
Learn more about the work of our research integrity team to safeguard the quality of each article we publish.
Find out more
PERSPECTIVE article
Front. Psychol., 16 November 2023
Sec. Emotion Science
Volume 14 - 2023 | https://doi.org/10.3389/fpsyg.2023.1254179
This article is part of the Research TopicInsights in Emotion ScienceView all 12 articles
Decision-making is an executive function, tapping into cognitive, emotional, and personality-based components. This complexity, and the varying operational definitions of the construct, is reflected in the rich array of behavioral decision-making tasks available for use in research and clinical settings. In many cases, these tasks are “subfield-specific,” with tasks developed by cognitive psychologists focusing on cognitive aspects of decision-making and tasks developed by clinical psychologists focusing on interactions between emotional and cognitive aspects. Critically, performance across different tasks does not consistently correlate, obfuscating the ability to compare scores between measures and detect changes over time. Differing theories as to what cognitive and/or emotional aspects affect decision-making likely contribute to this lack of consistency across measures. The low criterion-related validity among decision-making tasks and lack of consistent measurement of the construct presents challenges for emotion and decision-making scholars. In this perspective, we provide several recommendations for the field: (a) assess decision-making as a specific cognitive ability versus a taxonomy of cognitive abilities; (b) a renewed focus on convergent validity across tasks; (c) further assessment of test–retest reliability versus practice effects on tasks; and (d) reimagine future decision-making research to consider the research versus clinical implications. We discuss one example of decision-making research applied to clinical settings, acquired brain injury recovery, to demonstrate how some of these concerns and recommendations can affect the ability to track changes in decision-making across time.
Decision-making belongs to a group of higher-order, complex cognitive abilities linked to the prefrontal cortex (PFC) (Lezak et al., 2004) known as executive functions (EF). Decisions that are more emotion-based often activate ventromedial prefrontal cortex (VMPFC) (Ernst et al., 2002; Cho et al., 2012; Paulsen et al., 2012), whereas decisions that are more cognition-based activate dorsolateral prefrontal cortex (DLPFC) (Krain et al., 2006; Rolls and Grabenhorst, 2008). Yet both VMPFC and DLPFC can contribute to either type of decision-making. The brain’s reward pathway links the PFC with the limbic system and midbrain (Glimcher et al., 2009; Galvan, 2012), and decision-making activates this pathway (Jessor, 1991; Elliott et al., 2008; Curtis and Lee, 2010; Reyna, 2012). But aspects of the limbic system (Dalgleish, 2004; Fossati, 2012) and PFC (Baker et al., 1997) are linked to the processing of emotions, demonstrating that cognition and emotion utilize similar circuitry. Should we disentangle cognition and emotion in decision-making, or do we need to consider these two components together when defining the construct? It is possible that keeping emotion out of cognition (and vice versa) when defining decision-making has led to some of the current issues affecting the field. In the following sections, we provide a review of the current status of several important issues for decision-making as an individual differences variable and provide recommendations for the field moving forward. As applicable, we discuss where there may be different needs for those in the research versus clinical realms.
Although a full review of the history of decision-making, and all the relevant theories of it, is outside the scope of this perspective, several reviews and key pieces are available regarding signal detection theory (Stanislaw and Todorov, 1999), expected value- and utility-based theories (Von Neumann and Morgenstern, 1944; Machina, 1987), prospect theory (Kahneman and Tversky, 1979), and dual (Tversky and Kahneman, 1983; Metcalfe and Mischel, 1999; Reyna, 2004; Evans, 2008) and triple (Wood and Bechara, 2014) process system theories of decision-making. A key component of several of these theories is the extent to which utilizing cognitive factors (e.g., working memory, knowledge of probabilities, abstract reasoning; Reyna et al., 2009; Weber and Johnson, 2009; Curtis and Lee, 2010; Reyna and Brainerd, 2011; Brand et al., 2014) and emotional factors (Suhr and Tsanadis, 2007; Roiser et al., 2009; Buelow et al., 2013) can affect decisions. A differing emphasis on emotion versus cognition can lead to differences in the overall conceptualization of decision-making and, subsequently, in how it is assessed.
Is decision-making a specific cognitive ability, or does it instead represent a taxonomy of related cognitive abilities that are tied to the PFC, limbic system, and their subcortical connections? Is decision-making similar to the other executive functions, in that it is made up of different aspects of the construct rather than one overarching ability that is applied in every situation? To the extent that a decision is made in a consistent manner, across situations and across time, then decision-making may be more of a specific cognitive ability. Yet as many show (e.g., Schoemaker, 1993; Ert and Yechiam, 2010; Figner and Weber, 2011; Yechiam and Ert, 2011), individuals do not consistently weigh gains and losses (or risks and benefits) across situations. How questions are worded/framed (e.g., gain-framing versus loss-framing; Tversky and Kahneman, 1981; Yechiam and Ert, 2011) can lead to different decisions. These inconsistencies may also occur based on the specific type of risk, the extent to which cognitive and emotional factors come into play, and one’s own risk-taking propensity (e.g., Figner and Weber, 2011). These inconsistencies lead us to wonder if decision-making mimics executive functions, in that it serves as a taxonomy for a series of ‘subcomponents’ that make up the overarching construct. Several of these subcomponents may be activated in one situation but not in another, which could account for the inconsistencies when performance is assessed across tasks. Moving forward, the field should consider the overarching construct of decision making and whether it is a specific ability or a higher-order classification for a set of cognitive abilities.
If decision-making is a specific cognitive ability, and did not represent a taxonomy like the executive functions, then tasks assessing decision-making should show strong convergent validity. A task such as the Iowa Gambling Task (IGT; Bechara et al., 1994) should show strong correlations with the Balloon Analog Risk Task (BART; Lejuez et al., 2002), Game of Dice Task (GDT; Brand et al., 2007), and others. Some previous research finds such correlations (Brand et al., 2007; Henninger et al., 2010; Koritzky and Yechiam, 2010; Upton et al., 2011; MacKillop et al., 2014; Brown et al., 2015), but most instead show weak/small (Skeel et al., 2007; Mäntylä et al., 2012; Brunell and Buelow, 2017) or no (Mäntylä et al., 2012; Pletzer and Ortner, 2016) correlations between decision-making tasks designed to assess individual differences in the construct. If decision-making instead represents a taxonomy of cognitive abilities, then these inconsistencies make sense. Tasks that pull for more “cold” executive functions may correlate more with each other than with tasks that pull more for “hot” executive functions. We see this relationship when assessing correlations between the Wisconsin Card Sort Task, a measure of cold EF linked to the DLPFC (Lezak et al., 2004), as it correlates with decision-making tasks assessing more cold cognitions (e.g., Columbia Card Task, GDT; Brand et al., 2007, 2014; Buelow, 2015) but not with more hot/emotion-based tasks (e.g., IGT; Bechara et al., 2001; Reynolds et al., 2019). Factor analyses also demonstrate this lack of convergent validity (Buelow and Blaine, 2015).
Research investigating cognitive models of decisions on behavioral tasks point toward a better understanding of decision-making and convergent validity across tasks. Previous research points to factors such as sensitivity to gains/rewards (Yechiam and Busemeyer, 2008; Ert and Yechiam, 2010; Brevers et al., 2014), sensitivity to losses/risks (Bishara et al., 2009; Ert and Yechiam, 2010), frequency of gains and losses (Lin et al., 2009), choice consistency (Stout et al., 2004; Yechiam et al., 2005; Lin et al., 2016), discounting of versus learning from feedback (Yechiam et al., 2005; Prause and Lawyer, 2014; Byrne and Worthy, 2016), and individual differences in risk perception and acceptance (Wallsten et al., 2005; Ert and Yechiam, 2010; Figner and Weber, 2011; Yechiam and Ert, 2011) can affect decision-making across multiple tasks. It is possible that the relative lack of convergent validity across tasks to date is partly due to the nature of decision-making itself (e.g., specificity versus taxonomy) and partly due to the use of total/net scores to assess decision-making. Future research should continue to investigate these cognitive modeling-based commonalities across tasks, as there may be more convergence than it appears.
How does performance on decision-making tasks change across time? Is decision-making a relatively stable construct, or does it change across time or based on other factors? To assess the stability of decision-making, we can assess the test–retest reliability of various behavioral tasks. Estimates of test–retest reliability vary across specific tasks, samples, and time periods, but overall there are often moderate to strong correlations between tasks administered days (Johnson and Bruner, 2013; Weafer et al., 2013), weeks (Buelow and Barnhart, 2018), months (Forster et al., 2016; Peng et al., 2018), and years (Kirby, 2009; Yechiam and Ert, 2011) apart. Although these correlations are relatively high, interpretation of them as evidence in favor of strong test–retest reliability depends on the context. Portney and Watkins (2015)‘s guidelines for clinical measures are that correlations across time of 0.50–0.75 are poor/moderate while those over 0.75 are acceptable. Few—if any—of these correlations meet that criterion. In addition, there are some tasks that are single-use, as there is some element (e.g., learning the risks/benefits of the decks on the IGT) that cannot be ‘unlearned’ to allow for a second, future administration. On the IGT in particular, evidence of these learned practice effects is evident even years after the initial administration (e.g., Waters-Wood et al., 2012; see Buelow, 2020, for review). Although lab-based studies of healthy control or clinical participants may require a one-time assessment of current decision-making skills, real-world clinical evaluations can require multiple assessments over time. Tracking decision-making over time is difficult when (a) tasks lack test–retest reliability and convergent validity and (b) there are no clinically-available tasks (i.e., those that have been validated in clinical samples and for which normative data is available) that can be used in a longitudinal assessment. As we will discuss in the next section, concerns about reliability and validity may differ based on the implications of a research study.
As the field of decision science moves forward, the noted concerns about reliability and validity can point in different directions for new experimental designs, in part due to the basis for or reasoning behind a new research study. Is the intent to predict behavior? Document impaired decision-making based on a situational factor, individual differences factor, or psychological or medical/neurological diagnosis? Assess potential for change in the future? Understand decision-making or other cognitive/emotional process? Although in some cases it may be sufficient to document a one-time only assessment of decision-making impairment due to a diagnosis or other factor, in other cases the findings may have implications for treatments to improve decision-making skills among, for example, those with an acquired brain injury.
Acquired brain injury (ABI) is one example where more research is needed into both test–retest reliability and convergent validity, as tracking changes in cognitive difficulties across time is important for the patient with an ABI. ABI refers to any injury to the brain, such as what occurs in the course of traumatic (TBI; e.g., from accidents or falls) or nontraumatic (e.g., stroke) injury (Cullen et al., 2008). Although cognitive and emotional consequences of ABI vary due to the specific regions affected, the PFC is often negatively impacted (McAllister, 2011). Following ABI, patients may experience difficulties with attention, memory encoding and retrieval, processing speed, and EF (Allanson et al., 2017; Watson et al., 2020); however, these cited meta-analyses do not assess how hot EFs, such as decision-making, change post-ABI. Many clinically-available EF tasks focus on cold cognitive processes, even though OFC, VMPFC, and medial PFC may also be affected. Results of EF assessments predict long-term outcome for patients post-ABI (Allanson et al., 2017), yet this is often predicated on results of cold (non-emotion based) EF assessment. Individuals with ABI can also experience changes in emotional experience and expression (Gouick and Gentleman, 2004; de Sousa et al., 2012; with Phineas Gage as an early example).
In their Jose et al. (2020) review, Jose and colleagues examined the current understanding of EF in ABI. They divided decision-making into a set of skills that include value coding, social context, and emotional dysregulation, with moral reasoning and working memory as related cognitive functions. Research suggests that VMPFC, DLPFC, and OFC are involved in each of these decision-making components, despite the belief that each structure is specific to one cognitive (or emotional) function. They point out that focusing on a specific/small area of cognition can result in a loss of the bigger picture in ABI, such as by focusing on value coding one loses sight of the working memory and emotion regulation difficulties that could also affect decision-making.
Following ABI, individuals are typically assessed in the acute recovery phase to (a) predict pre-morbid functioning; (b) determine their strengths and weaknesses post-injury; and (c) develop a treatment plan. This gives a single snapshot of that individual’s cognitive history, yet it is often used for future comparison. Although important to identify cognitive deficits, it is also important to document if those deficits resolve. The IGT is currently the only behavioral task with available normative data to guide interpretation of task performance in clinical populations (Bechara, 2007), with a recent meta-analysis supporting its utility to assess decision-making impairments post-ABI (regardless of lesion location; Moore et al., 2023, under review)1. However, even 1+ years after initial assessment, practice effects remain (not specific to ABI samples; Tuvblad et al., 2013; Xiao et al., 2013). If there were additional tasks that demonstrated strong convergent validity with the IGT, they could instead be used to assess decision-making change over time. But there is a lack of convergent validity across decision-making tasks, creating difficulty showing improved decision-making as individuals recover from ABI. And while there is a typical recovery period post-ABI, especially from traumatic etiologies (Cullen et al., 2008), our inability to track decision-making changes across time limits what we can learn about EF recovery and how decision-making strategies may change post-ABI.
Outside of ABI and other clinical diagnoses where repeatable decision-making tasks can be of benefit, within-subjects study designs can also benefit from repeatable tasks. As just one example, multiple studies have shown that participants’ current mood or emotional state can affect decision-making (e.g., Mitchell and Phillips, 2007; Tamir and Robinson, 2007; Buelow et al., 2013; Forgas, 2013). In most of the lab-based manipulations of mood, decision-making was assessed post-manipulation only. If there was a repeatable decision-making task, it could be utilized in a pre-post manipulation, within-participant study design. This would additionally allow researchers to assess the extent to which the mood manipulation interacted with prior characteristics of the individual and their decision-making ability to affect subsequent performance.
The cognitive and emotional conceptualizations of decision-making need to be more precise: when should cognition and emotion be integrated, and when should they be assessed separately? Applications of dual process theory suggest cognition and emotion inform each other, but many current tasks rely on operational definitions of decision-making focused on only cognition or only emotion. Emotion can inform our understanding of cognition, and cognition can inform our understanding of emotion. VMPFC and DLPFC pathways are functionally connected, forming a prefrontal-cingulate cortex network that combines elements of hot and cold (“hot-cold”) decision-making (Jose et al., 2020; Salehinejad et al., 2021). Even tasks designed to examine emotion-based decision-making are correlated with measures of EFs and other cognitive abilities, indicating you cannot take cognition out of emotion either. Continuing to ‘silo’ cognitive and emotional theories of decision-making limits our ability to understand why individuals take risks and make suboptimal decisions. Utilizing an integrated conceptualization of decision-making and better centering it within other EFs may help researchers develop new tasks with adequate psychometrics that can be used to track recovery from an acute TBI, for example.
We believe the field of decision science still has much to offer our understanding of decision-making processes. However, the field’s impact is constrained by the lack of good psychometrics for many tasks and inconsistencies in conceptualizations of decision-making leading to differences in explaining task performance. The incorporation of cognitive and affective components of decision-making into a single taxonomy will methodologically increase reliability and validity of decision-making measures, as tasks born out of this conceptualization should, at their core, be assessing an overarching conceptualization that includes the assessment of both cognitive and affective components of decision-making.
MB: Conceptualization, Writing – original draft, Writing – review & editing. SM: Conceptualization, Writing – review & editing. JK: Conceptualization, Writing – review & editing. BO: Conceptualization, Writing – review & editing.
The authors declare that the research was conducted in the absence of any commercial or financial relationships that could be construed as a potential conflict of interest.
All claims expressed in this article are solely those of the authors and do not necessarily represent those of their affiliated organizations, or those of the publisher, the editors and the reviewers. Any product that may be evaluated in this article, or claim that may be made by its manufacturer, is not guaranteed or endorsed by the publisher.
1. ^Moore, S., Naragon-Gainey, K., Pestell, C.F., Becerra, R., Buelow, M.T., and Fynn, D.M. (2023). The level and nature of impairment on the Iowa gambling task following acquired brain injury: a meta-analysis. (under review).
Allanson, F., Pestell, C., Gignac, G. E., Yeo, Y. X., and Weinborn, M. (2017). Neuropsychological predictors of outcome following traumatic brain injury in adults: a meta-analysis. Neuropsyhol. Rev. 27, 187–201. doi: 10.1007/s11065-017-9353-5
Baker, S. C., Frith, C. D., and Dolan, R. J. (1997). The interaction between mood and cognitive function studied with PET. Psychol. Med. 27, 565–578. doi: 10.1017/S0033291797004856
Bechara, A. (2007). Iowa gambling task professional manual. Lutz, FL: Psychological Assessment Resources.
Bechara, A., Damasio, A. R., Damasio, H., and Anderson, S. W. (1994). Insensitivity to future consequences following damage to human prefrontal cortex. Cognition 50, 7–15. doi: 10.1016/0010-0277(94)90018-3
Bechara, A., Dolan, S., Denburg, N., Hindes, A., Anderson, S. W., and Nathan, P. E. (2001). Decision-making deficits, linked to a dysfunctional ventromedial prefrontal cortex, revealed in alcohol and stimulant abusers. Neuropsycholgia 39, 376–389. doi: 10.1016/S0028-3932(00)00136-6
Bishara, A. J., Pleskac, T. J., Fridberg, D. J., Yechiam, E., Lucas, J., Busemeyer, J. R., et al. (2009). Similar processes despite divergent behavior in two commonly used measures of risky decision making. J. Behav. Decis. Mak. 22, 435–454. doi: 10.1002/bdm.641
Brand, M., Recknor, E. C., Grabenhorst, F., and Bechara, A. (2007). Decisions under ambiguity and decisions under risk: correlations with executive functions and comparisons of two different gambling tasks with implicit and explicit rules. J. Clin. Exp. Neuropsychol. 29, 86–99. doi: 10.1080/13803390500507196
Brand, M., Schiebener, J., Pertl, M.-T., and Delazer, M. (2014). Know the risk, take the win: how executive functions and probability processing influence advantageous decision making under risk conditions. J. Clin. Exp. Neuropsychol. 36, 914–929. doi: 10.1080/13803395.2014.955783
Brevers, D., Koritzky, G., Bechara, A., and Noël, X. (2014). Cognitive processes underlying impaired decision-making under uncertainty in gambling disorder. Addict. Behav. 39, 1533–1536. doi: 10.1016/j.addbeh.2014.06.004
Brown, E. C., Hack, S. M., Gold, J. M., Carpenter, W. T., Fischer, B. A., Prentice, K. P., et al. (2015). Integrating frequency and magnitude information in decision-making in schizophrenia: an account of patient performance on the Iowa gambling task. J. Psychiatr. Res. 66-67, 16–23. doi: 10.1016/j.jpsychires.2015.04.007
Brunell, A. B., and Buelow, M. T. (2017). Narcissism and performance on behavioral decision-making tasks. J. Behav. Decis. Mak. 30, 3–14. doi: 10.1002/bdm.1900
Buelow, M. T. (2015). Predicting performance on the Columbia card task: effects of personality characteristics, mood, and executive functions. Assessment 22, 178–187. doi: 10.1177/1073191114539383
Buelow, M. T., and Barnhart, W. R. (2018). Test-retest reliability of common behavioral decision making tasks. Arch. Clin. Neuropsychol. 33, 125–129. doi: 10.1093/arclin/acx038
Buelow, M. T., and Blaine, A. L. (2015). The assessment of risky decision making: a factor analysis of performance on the Iowa gambling task, balloon analogue risk task, and Columbia card task. Psychol. Assess. 27, 777–785. doi: 10.1037/a0038622
Buelow, M. T., Okdie, B. M., and Blaine, A. L. (2013). Seeing the forest through the trees: improving decision making on the Iowa gambling task by shifting focus from short- to long-term outcomes. Front. Psychol. 4:773. doi: 10.3389/fpsyg.2013.00773
Byrne, K. A., and Worthy, D. A. (2016). Toward a mechanistic account of gender differences in reward-based decision-making. J. Neurosci. Psychol. Econ. 9, 157–168. doi: 10.1037/npe0000059
Cho, S. S., Pellecchia, G., Aminian, K., Ray, N., Segura, B., Obeso, I., et al. (2012). Morphometric correlation of impulsivity in medial prefrontal cortex. Brain Topogr. 26, 479–487. doi: 10.1007/s10548-012-0270-x
Cullen, N. K., Park, Y., and Bayley, M. T. (2008). Functional recovery following traumatic vs non-traumatic brain injury: a case-controlled study. Brain Inj. 22, 1013–1020. doi: 10.1080/02699050802530581
Curtis, C. E., and Lee, D. (2010). Beyond working memory: the role of persistent activity in decision making. Trends Cogn. Sci. 14, 216–222. doi: 10.1016/j.tics.2010.03.006
de Sousa, A., McDonald, S., and Rushby, J. (2012). Changes in emotional empathy, affective responsivity, and behavior following severe traumatic brain injury. J. Clin. Exp. Neuropsychol. 34, 606–623. doi: 10.1080/13803395.2012.667067
Elliott, R., Agnew, Z., and Deakin, F. W. (2008). Medial orbitofrontal cortex codes relative rather than absolute value of financial rewards in humans. Eur. J. Neurosci. 27, 2213–2218. doi: 10.1111/j.1460-9568.2008.06202.x
Ernst, M., Bolla, K., Mouratidis, M., Contoreggi, C., Matochik, J. A., Kurian, V., et al. (2002). Decision-making in a risk-taking task: A PET study. Neuropsychopharmacology 26, 682–691. doi: 10.1016/S0893-133X(01)00414-6
Ert, E., and Yechiam, E. (2010). Consistent constructs in individuals’ risk taking in decisions from experience. Acta Psychol. 134, 225–232. doi: 10.1016/j.actpsy.2010.02.003
Evans, J. S. B. T. (2008). Dual-processing accounts of reasoning, judgment, and social cognition. Annu. Rev. Psychol. 59, 255–278. doi: 10.1146/annurev.psych.59.103006.093629
Figner, B., and Weber, E. U. (2011). Who takes risks when and why?: determinants of risk taking. Curr. Dir. Psychol. Sci. 20, 211–216. doi: 10.1177/0963721411415790
Forgas, J. P. (2013). Don’t worry, be sad! On the cognitive, motivational, and interpersonal benefits of negative mood. Curr. Dir. Psychol. Sci. 22, 225–232. doi: 10.1177/0963721412474458
Forster, S. E., Finn, P. R., and Brown, J. W. (2016). A preliminary study of longitudinal neuroadaptation associated with recovery from addiction. Drug Alcohol Depend. 168, 52–60. doi: 10.1016/j.drugalcdep.2016.08.626
Fossati, P. (2012). Neural correlates of emotion processing: from emotional to social brain. Eur. Neuropsychopharmacol. 22, S487–S491. doi: 10.1016/j.euroneuro.2012.07.008
Galvan, A. (2012). “Risky behavior in adolescents: the role of the developing brain” in The adolescent brain: Learning, reasoning, and decision making. eds. V. F. Reyna, S. B. Chapman, M. R. Dougherty, and J. Confrey (Washington, DC: American Psychological Association), 267–289.
Glimcher, P.W., Camerer, C.F., Fehr, E., and Poldrack, R.A.. (2009). Neuroeconomics: Decision making and the brain. New York: Academic Press.
Gouick, J., and Gentleman, D. (2004). The emotional and behavioural consequences of traumatic brain injury. Trauma 6, 285–292. doi: 10.1191/1460408604ta323oa
Henninger, D. E., Madden, D. J., and Huettel, S. A. (2010). Processing speed and memory mediate age-related differences in decision making. Psychol. Aging 25, 262–270. doi: 10.1037/a0019096
Jessor, R. (1991). Risk behavior in adolescence: a psychosocial framework for understanding and action. J. Adolesc. Health 12, 597–605. doi: 10.1016/1054-139X(91)90007-K
Johnson, M. W., and Bruner, N. R. (2013). Test-retest reliability and gender differnces in the sexual discounting task among cocaine-dependent individuals. Exp. Clin. Psychopharmacol. 21, 277–286. doi: 10.1037/a0033071
Jose, R. G., Samuel, A. S., and Isabel, M. M. (2020). Neuropsychology of executive functions in patients with focal lesion in the prefrontal cortex: a systematic review. Brain Cogn. 146:105633. doi: 10.1016/j.bandc.2020.105633
Kahneman, D., and Tversky, A. (1979). Prospect theory: an analysis of decision under risk. Econometrica 47, 263–292. doi: 10.2307/1914185
Kirby, K. N. (2009). One-year temporal stability of delay-discount rates. Psychon. Bull. Rev. 16, 457–462. doi: 10.3758/PBR.16.3.457
Koritzky, G., and Yechiam, E. (2010). On the robustness of description and experience based decision tasks to social desirability. J. Behav. Decis. Mak. 23, 83–99. doi: 10.1002/bdm.660
Krain, A. L., Wilson, A. M., Arbuckle, R., Castellanos, F. X., and Milham, M. P. (2006). Distinct neural mechanisms of risk and ambiguity: a meta-analysis of decision-making. NeuroImage 32, 477–484. doi: 10.1016/j.neuroimage.2006.02.047
Lejuez, C. W., Richards, J. B., Read, J. P., Kahler, C. W., Ramsey, S. E., Stuart, G. L., et al. (2002). Evaluation of a behavioral measure of risk taking: the balloon analogue risk task (BART). J. Exp. Psychol. Appl. 8, 75–84. doi: 10.1037//1076-898x.8.2.75
Lezak, M.D., Howieson, D.B., and Loring, D.W. (2004). Neuropsychological Assessment. Oxford: Oxford University Press
Lin, C. H., Chiu, Y. C., and Huang, J. T. (2009). Gain-loss frequency and final outcome in the Soochow gambling task: a reassessment. Behav. Brain Funct. 5:45. doi: 10.1186/1744-9081-5-45
Lin, C. H., Lin, Y. K., Song, T. J., Huang, J. T., and Chiu, Y. C. (2016). A simplified model of choice behavior under uncertainty. Front. Psychol. 7:1201. doi: 10.3389/fpsyg.2016.01201
Machina, M. (1987). Choice under uncertainty: problems solved and unsolved. J. Econ. Perspect. 1, 121–154. doi: 10.1257/jep.1.1.121
MacKillop, J., Miller, J. D., Fortune, E., Maples, J., Lance, C. E., Campbell, W. K., et al. (2014). Multidimensional examination of impulsivity in relation to disordered gambling. Exp. Clin. Psychopharmacol. 22, 176–185. doi: 10.1037/a0035874
Mäntylä, T., Still, J., Gullberg, S., and Del Missier, F. (2012). Decision making in adults with ADHD. J. Atten. Disord. 16, 164–173. doi: 10.1177/1087054709360494
McAllister, T. W. (2011). Neurobiological consequences of traumatic brain injury. Dialogues Clin. Neurosci. 13, 287–300. doi: 10.31887/DCNS.2011.13.2/tmcallister
Metcalfe, J., and Mischel, W. (1999). A hot/cool-system analysis of delay of gratification: dynamics of willpower. Psychol. Rev. 106, 3–19. doi: 10.1037/0033-295X.106.1.3
Mitchell, R. L. C., and Phillips, L. H. (2007). The psychological, neurochemical and functional neuroanatomical mediators of the effects of positive and negative mood on executive functions. Neuropsychologia 45, 617–629. doi: 10.1016/j.neuropsychologia.2006.06.030
Paulsen, D. J., Carter, R. M., Platt, M. L., Huettel, S. A., and Brannon, E. M. (2012). Neurocognitive development of risk aversion from early childhood to adulthood. Front. Hum. Neurosci. 5:178. doi: 10.3389/fnhum.2011.00178
Peng, J., Feng, T., Zhang, J., Zhao, L., Zhang, Y., Chang, Y., et al. (2018). Measuring decision-making competence in Chinese adults. J. Behav. Decis. Mak. 32, 266–279. doi: 10.1002/bdm.2114
Pletzer, B., and Ortner, T. M. (2016). Neuroimaging supports behavioral personality assessment: overlapping activations during reflective and impulsive risk taking. Biol. Psychol. 119, 46–53. doi: 10.1016/j.biopsycho.2016.06.012
Portney, L.G., and Watkins, M.P. (2015). Foundations of clinical research: applications to practice. Pearson/Prentice Hall. Upper Saddle River.
Prause, N., and Lawyer, S. (2014). Specificity of reinforcement for risk behaviors of the balloon analogue risk task using math models of performance. J. Risk Res. 17, 317–335. doi: 10.1080/13669877.2013.808688
Reyna, V. F. (2004). How people make decisions that involve risk: a dual-processes approach. Curr. Dir. Psychol. Sci. 13, 60–66. doi: 10.1111/j.0963-7214.2004.00275.x
Reyna, V. F. (2012). A new intuitionism: meaning, memory, and development in fuzzy-trace theory. Judgm. Decis. Mak. 7, 332–359. doi: 10.1017/S1930297500002291
Reyna, V. F., and Brainerd, C. J. (2011). Dual processes in decision making and developmental neuroscience: A fuzzy-trace model. Dev. Rev. 31, 180–206. doi: 10.1016/j.dr.2011.07.004
Reyna, V. F., Nelson, W. L., Han, P. K., and Dieckmann, N. F. (2009). How numeracy influences risk comprehension and medical decision making. Psychol. Bull. 135, 943–973. doi: 10.1037/a0017327
Reynolds, B. W., Basso, M. R., Miller, A. K., Whiteside, D. M., and Combs, D. (2019). Executive function, impulsivity, and risky behaviors in young adults. Neuropsychology 33, 212–221. doi: 10.1037/neu0000510
Roiser, J., Farmer, A., Lam, D., Burke, A., O’Neill, N., Keating, S., et al. (2009). The effect of positive mood induction on emotional processing in euthymic individuals with bipolar disorder and controls. Psychol. Med. 39, 785–791. doi: 10.1017/S0033291708004200
Rolls, E. T., and Grabenhorst, F. (2008). The orbitofrontal cortex and beyond: from affect to decision-making. Prog. Neurobiol. 86, 216–244. doi: 10.1016/j.pneurobio.2008.09.001
Salehinejad, M. A., Ghanavati, E., Rashid, M. H. A., and Nitsche, M. A. (2021). Hot and cold executive functions in the brain: a prefrontal-cingular network. Brain Neurosci. Adv. 5, 239821282110077–239821282110019. doi: 10.1177/23982128211007769
Schoemaker, P. J. H. (1993). Determinants of risk-taking: behavioral and economic views. J. Risk Uncertain. 6, 49–73. doi: 10.1007/BF01065350
Skeel, R. L., Neudecker, J., Pilarski, C., and Pytlak, K. (2007). The utility of personality variables and behaviorally-based measures in the prediction of risk-taking behavior. Personal. Individ. Differ. 43, 203–214. doi: 10.1016/j.paid.2006.11.025
Stanislaw, H., and Todorov, N. (1999). Calculation of signal detection theory measures. Behav. Res. Methods Instrum. Comput. 31, 137–149. doi: 10.3758/BF03207704
Stout, J. C., Busemeyer, J. R., Lin, A., Grant, S. J., and Bonson, K. R. (2004). Cognitive modeling analysis of decision-making processes in cocaine abusers. Psychon. Bull. Rev. 11, 742–747. doi: 10.3758/BF03196629
Suhr, J. A., and Tsanadis, J. (2007). Affect and personality correlates of the Iowa gambling task. Personal. Individ. Differ. 43, 27–36. doi: 10.1016/j.paid.2006.11.004
Tamir, M., and Robinson, M. D. (2007). The happy spotlight: positive mood and selective attention to rewarding information. Personal. Soc. Psychol. Bull. 33, 1124–1136. doi: 10.1177/0146167207301030
Tuvblad, C., Gao, Y., Wang, P., Raine, A., Botwick, T., and Baker, L. A. (2013). The genetic and environmental etiology of decision-making: a longitudinal twin study. J. Adolesc. 36, 245–255. doi: 10.1016/j.adolescence.2012.10.006
Tversky, A., and Kahneman, D. (1981). The framing of decisions and the psychology of choice. Science 211, 453–458. doi: 10.1126/science.7455683
Tversky, A., and Kahneman, D. (1983). Extensional vs. intuitive reasoning: the conjunction fallacy in probability judgment. Psychol. Rev. 90, 293–315. doi: 10.1037/0033-295X.90.4.293
Upton, D. J., Bishara, A. J., Ahn, W.-Y., and Stout, J. C. (2011). Propensity for risk taking and trait impulsivity in the Iowa gambling task. Personal. Individ. Differ. 50, 492–495. doi: 10.1016/j.paid.2010.11.013
Von Neumann, J., and Morgenstern, O. (1944). Theory of games and economic behavior. Princeton University Press. Princeton.
Wallsten, T. S., Pleskac, T. J., and Lejuez, C. W. (2005). Modeling behavior in a clinically diagnostic sequential risk-taking task. Psychol. Rev. 112, 862–880. doi: 10.1037/0033-295X.112.4.862
Waters-Wood, S. M., Xiao, L., Denburg, N. L., Hernandez, M., and Bechara, A. (2012). Failure to learn from repeated mistakes: persistent decision-making impairment as measured by the Iowa gambling task in patients with ventromedial prefrontal cortex lesions. J. Int. Neuropsychol. Soc. 18, 927–930. doi: 10.1017/S135561771200063X
Watson, P. A., Gignac, G. E., Weinborn, M., Green, S., and Pestell, C. (2020). A meta-analysis of neuropsychological predictors of outcome following stroke and other non-traumatic acquired brain injuries in adults. Neuropsychol. Rev. 30, 194–223. doi: 10.1007/s11065-020-09433-9
Weafer, J., Baggott, M. J., and de Wit, H. (2013). Test-retest reliability of behavioral measures of impulsive choice, impulsive action, and inattention. Exp. Clin. Psychopharmacol. 21, 475–481. doi: 10.1037/a0033659
Weber, E. U., and Johnson, E. J. (2009). Mindful judgment and decision making. Annu. Rev. Psychol. 60, 53–85. doi: 10.1146/annurev.psych.60.110707.163633
Wood, S. M. W., and Bechara, A. (2014). “The neuroscience of dual (and triple) systems in decision making” in The neuroscience of risky decision making. eds. V. F. Reyna and V. Zayas (Washington, DC: American Psychological Association Press)
Xiao, L., Wood, S. M. W., Denburg, N. L., Moreno, G. L., Hernandez, M., and Bechara, A. (2013). Is there a recovery of decision-making function after frontal lobe damage? A study using alternative versions of the Iowa gambling task. J. Clin. Exp. Neuropsychol. 35, 518–529. doi: 10.1080/13803395.2013.789484
Yechiam, E., and Busemeyer, J. R. (2008). Evaluating generalizability and parameter consistency in learning models. Games Econ. Behav. 63, 370–394. doi: 10.1016/j.geb.2007.08.011
Yechiam, E., Busemeyer, J. R., Stout, J. C., and Bechara, A. (2005). Using cognitive models to map relations between neuropsychological disorders and human decision-making deficits. Psychol. Sci. 16, 973–978. doi: 10.1111/j.1467-9280.2005.01646.x
Keywords: decision-making, emotion, cognition, executive function, validity
Citation: Buelow MT, Moore S, Kowalsky JM and Okdie BM (2023) Cognitive chicken or the emotional egg? How reconceptualizing decision-making by integrating cognition and emotion can improve task psychometrics and clinical utility. Front. Psychol. 14:1254179. doi: 10.3389/fpsyg.2023.1254179
Received: 06 July 2023; Accepted: 31 October 2023;
Published: 16 November 2023.
Edited by:
Andrew Kemp, Swansea University, United KingdomReviewed by:
Eldad Yechiam, Technion Israel Institute of Technology, IsraelCopyright © 2023 Buelow, Moore, Kowalsky and Okdie. This is an open-access article distributed under the terms of the Creative Commons Attribution License (CC BY). The use, distribution or reproduction in other forums is permitted, provided the original author(s) and the copyright owner(s) are credited and that the original publication in this journal is cited, in accordance with accepted academic practice. No use, distribution or reproduction is permitted which does not comply with these terms.
*Correspondence: Melissa T. Buelow, YnVlbG93LjExQG9zdS5lZHU=
Disclaimer: All claims expressed in this article are solely those of the authors and do not necessarily represent those of their affiliated organizations, or those of the publisher, the editors and the reviewers. Any product that may be evaluated in this article or claim that may be made by its manufacturer is not guaranteed or endorsed by the publisher.
Research integrity at Frontiers
Learn more about the work of our research integrity team to safeguard the quality of each article we publish.